What factors are driving changes in climate and how can we reduce the uncertainties?
Weather, the short-term conditions of the atmosphere, is not climate; but climate, the “envelope” or distribution of weather over time and space, influences the weather. If we want to better understand the weather and have more accurate weather predictions, we need to better understand what is happening to the climate under current changes.
A lot is known about the physical impacts of a warming climate: more moisture in the air and warmer temperatures lead to increased heavy precipitation events, and in combination with warmer sea surface temperatures may lead to stronger hurricanes; increased temperatures lead to higher probabilities of heatwaves and more fires.
While we know for certain that global temperature is rising, other questions still remain surrounded by uncertainty. How strongly will the Earth’s temperature respond to increasing CO2 levels? What changes will happen on regional scales and how strong will they be?
These questions are difficult to answer because of the highly complex and unknown feedbacks between the components of the climate – atmosphere, ocean, land and ice, and because regionally internal climate variability is large enough to mask climate changes for possibly many decades to come . This is precisely because regional variability allows for seemingly contradictory events to occur on short-time scales, such as cold winter days in a warming climate.
Changes are happening. To what or whom do we attribute them ?
The Earth’s climate is constantly changing under the influence of both internal and external drivers. Internal drivers, such as the well-known El-Niño or the lesser-known Pacific Decadal Oscillation and North Atlantic Oscillation, lead to variability in the climate that spans over a few years up to multiple decades.
These are processes that occur naturally within the climate and constitute the so-called internal (unforced) climate variability. External drivers, also called external forcings, such as the influence of the sun, volcanic activity or human (anthropogenic) influence, externally shape the climate of the Earth and constitute the so-called forced climate variability.
When studying changes in the climate, it is relevant to both predict these changes and to identify their causes. Is the increase in global mean temperature that we are witnessing due to internal or external forcing? If it is external forcing, is it due to solar forcing, volcanic activity and/or human influence, i.e., anthropogenic greenhouse gases (GHG)?
While climate change is often used in the media to denote human-induced global warming, more broadly, forced climate change is any change caused by one or multiple of the external drivers.
A subfield of climate science, called detection and attribution (D&A), provides answers to these questions by studying the causal links between the external drivers of climate change and observed changes in climate variables, such as temperature, precipitation, or moisture.
How does detection & attribution of climate change work ?
Detection & attribution is one of the chapters of the Intergovernmental Panel on Climate Change (IPCC) report that comes out every few years. IPCC published its last report, the Fifth Assessment Report (AR5), in 2014 and the next one, the Sixth Assessment Report (AR6), is due in 2022.
According to the IPCC’s definition, detection aims to find if there is a change in the observations that cannot be explained by internal climate variability alone, and attribution tries to assign the detected change to a particular external forcing or a combination of forcings. Observations here represent for example maps of temperature or precipitation records covering the whole Earth or large regions.
Climate models are complex numerical models that simulate the behavior of the different components of the climate - atmosphere, ocean, land and ice, and allow scientists to simulate "possible" climates, climates that could possibly exist, in order to study their dynamics and their responses to internal or external forcings.
One such "possible" climate is a climate with only volcanic and solar forcings but no anthropogenic forcing (blue line in Figure 1), or a climate with only anthropogenic forcing but no solar or volcanic forcing (orange line), or even a climate with a solar forcing ten times stronger than what is observed in nature.
D&A methods use these simulations to explain the observations from nature (dots in Figure 1 – the climate trajectory that Earth has been on since the late 19th century) using regression analysis. The observations are regressed on a wide range of "possible" climates, and the climates that receive the largest scaling factors (regression coefficients) are the ones that best explain the underlying causes of climate change.
In Figure 1, starting around 1980, the solar and volcanic forcings alone (blue line) are not sufficient to explain the warming in the temperature (dots), which is more consistent with the anthropogenic-only forcing (orange line).
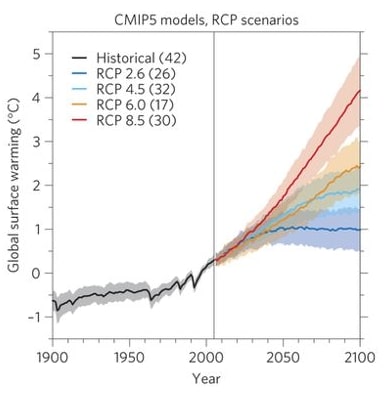
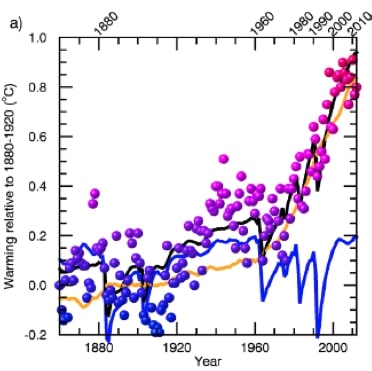
Besides simulating "possible" climates, climate models are also used to compute projections for future climates (for example, the evolution of temperature) in response to different trajectories for GHGs throughout the 21th century adopted by the IPCC, called Representative Concentration Pathways (RCPs).
There are four RCP scenarios considered in IPCC – RCP 2.6, RCP 4.5, RCP 6.0 and RCP 8.5. Figure 2 from shows the global mean temperature for the historical period (black line) and for the four different RCP scenarios along with their uncertainties estimated using an ensemble of climate models (the number of models is given in brackets) from the last Coupled Model Intercomparison Project (CMIP5).
Under RCP 8.5 the global mean temperature (the temperature averaged over all locations on the Earth) will increase by 3.7°C on average compared to the current climate, which has warmed by 1°C compared to the historical (preindustrial) period (1870-1920). The uncertainty around this average is of about 1.5°C among the different climate models.
Advancing the state-of-the-art of detection & attribution using statistical learning
The Institute for Atmospheric and Climate Science at ETH, the Seminar for Statistics at ETH and the Swiss Data Science Center (SDSC) are actively working towards advancing the state-of-the-art of D&A to improve the robustness of the methods and to reduce the uncertainties.
Standard D&A methods aim to explain the observations directly as a combination of climate model’s responses to different external forcings. However, this is a difficult problem because of the high-dimensionality of climate input data. Currently, we are working on incorporating supervised statistical learning techniques into the framework of D&A where we explain directly the external forcing from climate variables, and subsequently use the predicted forcing as a test statistic for D&A .
One aspect that we need to take into account is that these climate variables are influenced by multiple external forcings, and therefore the predictions that we make for one external forcing may also be sensitive to changes in the other external forcings.
The causal diagram in Figure 3 shows for example the temperature influenced by the solar, volcanic and anthropogenic forcings. If we want to predict the anthropogenic forcing, but in the future the radiation from the sun becomes stronger or the Earth experiences stronger volcanoes than previously seen, our predictions might fail. Because the “predicted forcing” is used as a test statistic for D&A, a wrong “prediction” may then translate into a wrong attribution statement.
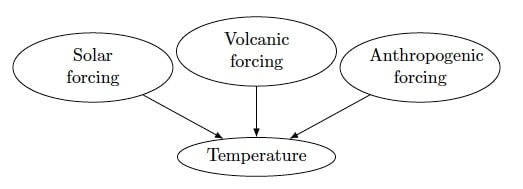
In the causal inference literature, such changes are called interventions or perturbations. They lead to distributional changes in the external forcings and therefore possibly to instability in the prediction which tends to overfit to the distribution of the only data that it has seen, that is, our current climate.
How can we guarantee that our predictions will work well even if these changes occur? We are currently studying these questions from a climate perspective, as well as a statistical perspective.
The climate is constantly changing and we need to continue developing robust methods to identify more and more accurately the causes and drivers of the changes that we are observing.
Co-authors
- Sebastian Sippel, Postdoctoral Researcher, ETH Zürich
References
- C. Deser, R. Knutti, S. Solomon, and A. S. Phillips. Communication of the role of natural variability in future North American climate. Nature Climate Change, 2, 775-779, DOI: https://doi.org/10.1038/nclimate1562 (2012).
- R. Knutti, J. Sedláček. Robustness and uncertainties in the new CMIP5 climate model projections. Nature Climate Change, 3, 369–373, DOI: https://doi.org/10.1038/nclimate1716 (2013)
- E. Székely, S. Sippel, R. Knutti, G. Obozinski, N. Meinshausen (2019). A direct approach to detection and attribution of climate change. Proceedings of the 9th International Workshop on Climate Informatics: CI2019 (No. NCAR/TN-561+PROC), 119-124, doi:10.5065/y82j-f154
- S. Sippel, N. Meinshausen, E. M. Fischer, E. Székely, R. Knutti (2020). Climate change now detectable from any single day of weather at global scale. Nature Climate Change, 10, 35-41, doi:10.1038/s41558-019-0666-7. See also News & Views
- C. Deser, R. Knutti, S. Solomon, and A. S. Phillips. Communication of the role of natural variability in future North American climate. Nature Climate Change, 2, 775-779, DOI: https://doi.org/10.1038/nclimate1562 (2012). R. Knutti, J. Sedláček. Robustness and uncertainties in the new CMIP5 climate model projections. Nature Climate Change,3, 369–373, DOI: https://doi.org/10.1038/nclimate1716 (2013) E. Székely, S. Sippel, R. Knutti, G. Obozinski, N. Meinshausen. A direct approach to detection and attribution of climate change. To appear in Proceedings of the 9th International Workshop on Climate Informatics (2019).