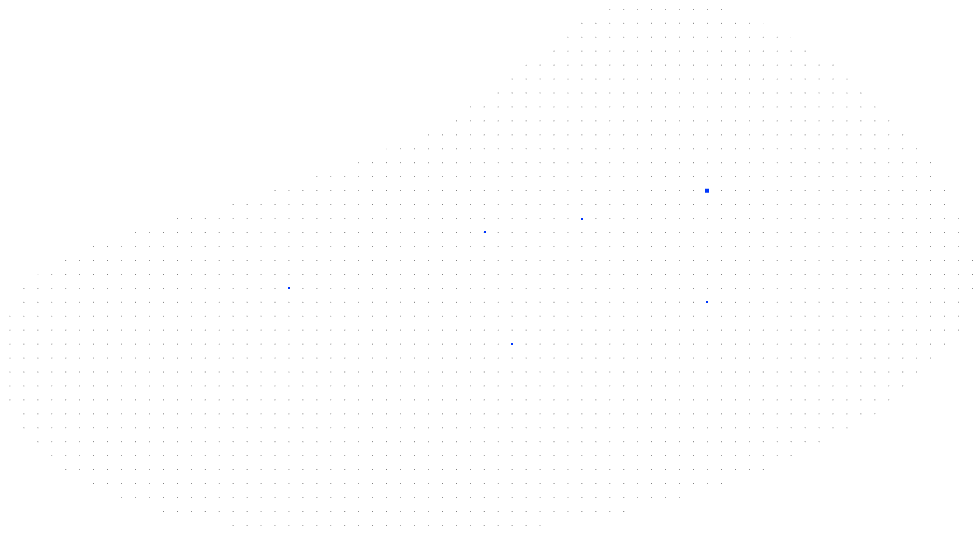
PAIRED-HYDRO
Machine learning for the components fatigue prediction in hydropower generation

Abstract
The contribution of renewable energy has increased dramatically in Europe, now estimated to be greater than 33%. As part of the European Green Deal, the European Commission is currently raising its targets still further with significant contribution from intermittent renewables, such as wind and solar sources, and disconnection of the so-called conventional units, as greenhouse gases emitters. This drastic change in the power system calls for a strong support from sources to guarantee the frequency and voltage stability by providing ancillary services for a suitable power regulation in both production and consumption. Hydropower easily provides active and reactive power regulation, but the need of ancillary services provision is expected to grow considerably in the next decades. Facing the increasing demand in flexibility, the hydroelectrical technologies employed in the power plants have to be ready to tackle the foreseen challenges in following fast dynamics. This often requires hydraulic machines to operate in off-design conditions and to increase the number of transitions to ensure high flexibility, but also availability and reliability of the power system.
PAIRED-HYDRO aims at developing a methodology to improve the operational safety and the condition-based maintenance asset of the hydroelectric unit. In particular, the study will be focused on the prediction of the damage due to fatigue and the optimization of the operational sequences to minimize the lifetime reduction of runner blades and guide vanes of a Francis-type hydraulic machine (see Figure 2).
People
Collaborators
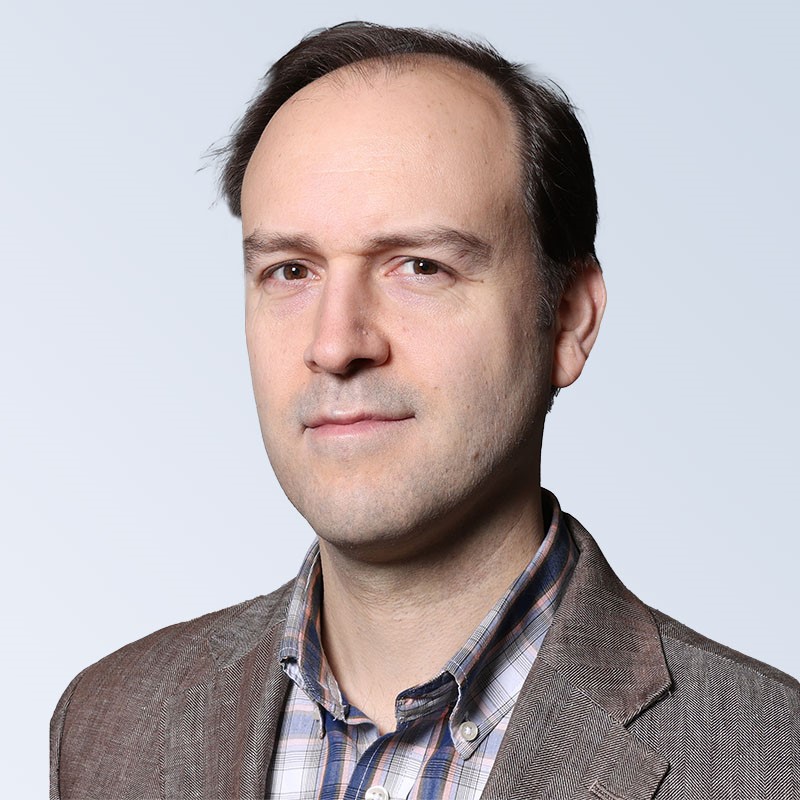
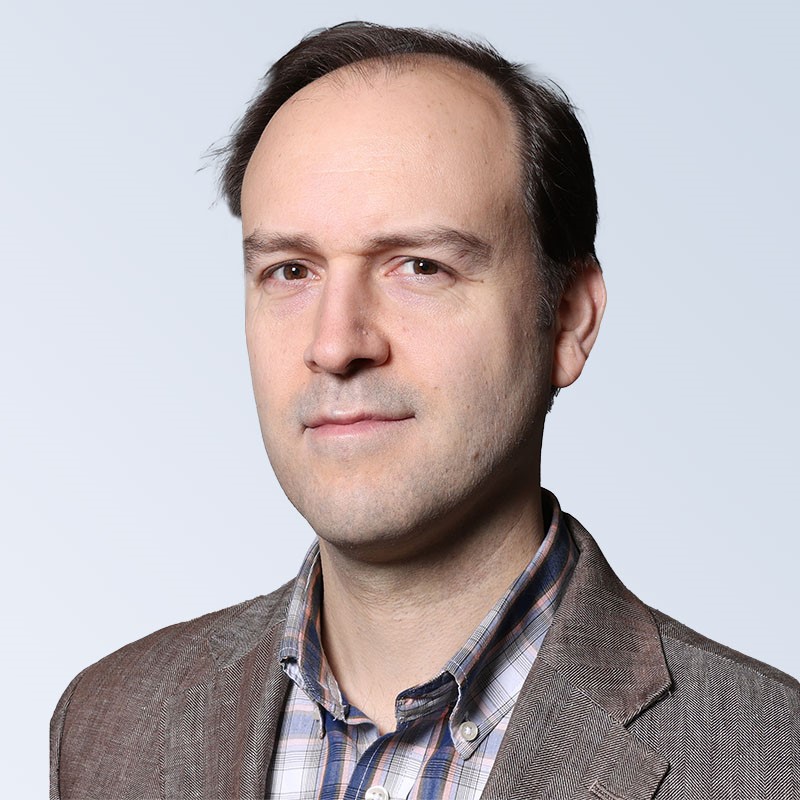
Guillaume Obozinski graduated with a PhD in Statistics from UC Berkeley in 2009. He did his postdoc and held until 2012 a researcher position in the Willow and Sierra teams at INRIA and Ecole Normale Supérieure in Paris. He was then Research Faculty at Ecole des Ponts ParisTech until 2018. Guillaume has broad interests in statistics and machine learning and worked over time on sparse modeling, optimization for large scale learning, graphical models, relational learning and semantic embeddings, with applications in various domains from computational biology to computer vision.
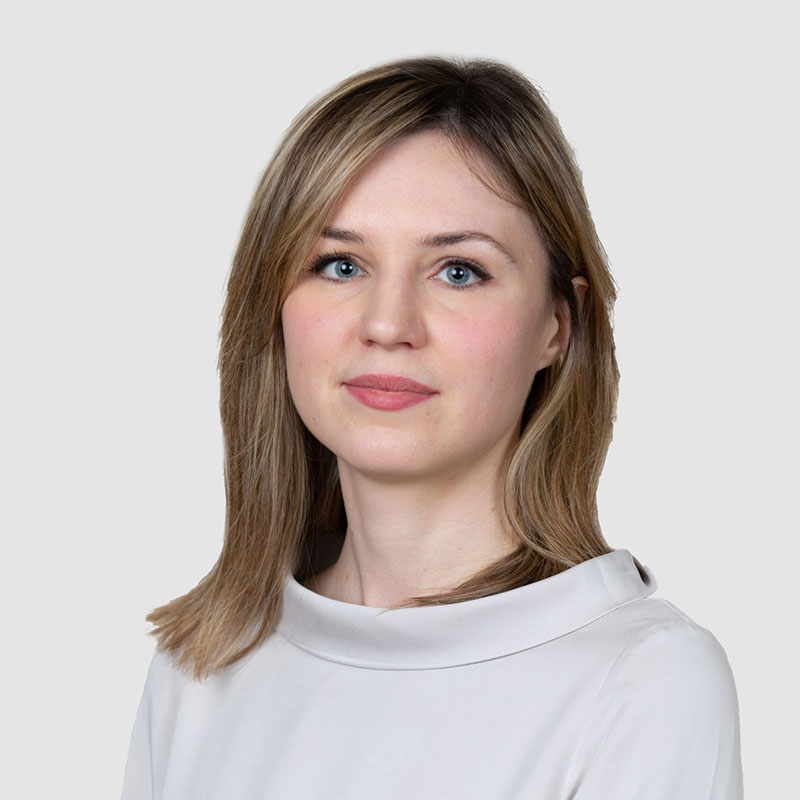
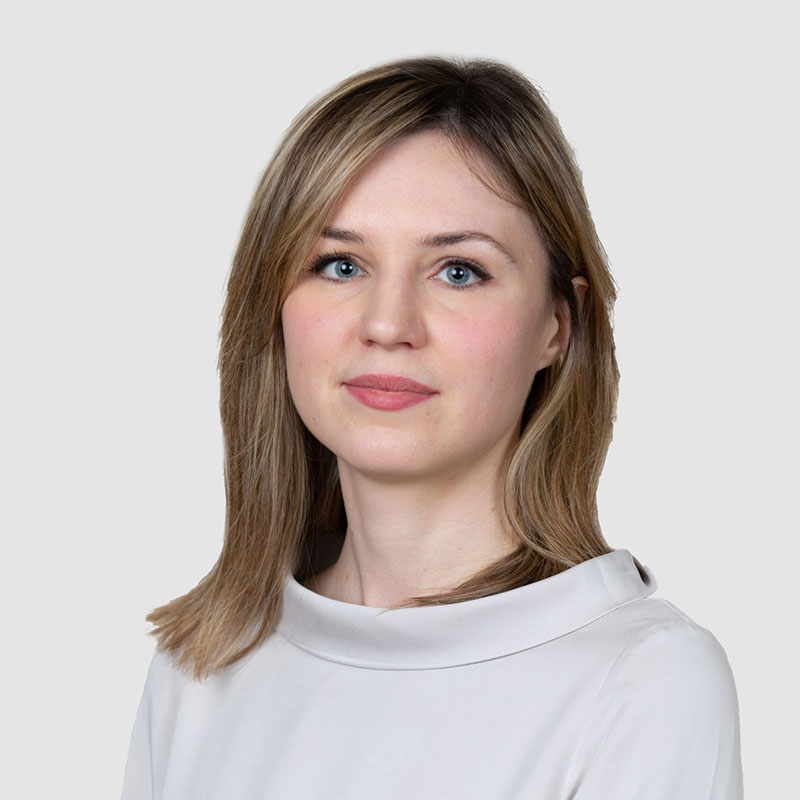
Ekaterina received her PhD in Computer Science from Moscow Institute for Physics and Technology, Russia. Afterwards, she worked as a researcher at the Institute for Information Transmission Problems in Moscow and later as a postdoctoral researcher in the Stochastic Group at the Faculty of Mathematics at University Duisburg-Essen, Germany. She has experience with various applied projects on signal processing, predictive modelling, macroeconomic modelling and forecasting, and social network analysis. She joined the SDSC in November 2019. Her interests include machine learning, non-parametric statistical estimation, structural adaptive inference, and Bayesian modelling.


Till obtained his Bachelor's and his Master's degrees in Physics at ETH Zurich in 2020 and 2022 respectively. Over the course of his studies and by applying computational methods to problems in physics, he developed a fascination with data science and machine learning. Having joined the SDSC in July 2022, Till seeks to gain an impression of academia outside the physics domain, as well as a deeper understanding of data-driven problem-solving. In his work, Till is using Deep Learning methods to lengthen the lifespan of hydropower turbines and to better understand the effect of mutations in non-coding regions of the DNA.
PI | Partners:
EPFL, Technology Platform for Hydraulic Machines:
- Dr. Elena Vagnoni
- Dr. Alessandro Morabito
- Prof. Mario Paolone
description
Motivation
Currently, there exists no modelling of the stress or damage incurred in turbines, thus predictive maintenance is used: At regular intervals, the hydroelectric unit is stopped for inspection and reparis. The goal of the project is to a) provide an accurate model of damage incurred both in the steady-state and transient regime of hydraulic turbines; and b) to provide a method that produces favourable (i.e. least damaging) start-up trajectory, allowing for a more judicious maintenance schedule and less frequent repairs.
Proposed Approach / Solution
The SDSC models the stress inflicted on different parts of the turbine based on operating conditions. The stress signal can be converted into a quantification of damage using standard mechanical engineering techniques such as rainflow counting and Miner’s rule. As oscillations are non-negligible contributors to the damage, we take care to model oscillations as well as an overall trend estimate (see Figure 1). After training and validating the stress model, we investigate optimized controls sequences to produce less-damaging start-up trajectories. These trajectories have been tested using a reduced-scale model at the PTMH (see Figure 3) to verify their performance and incure less damage than previously investigated trajectories.
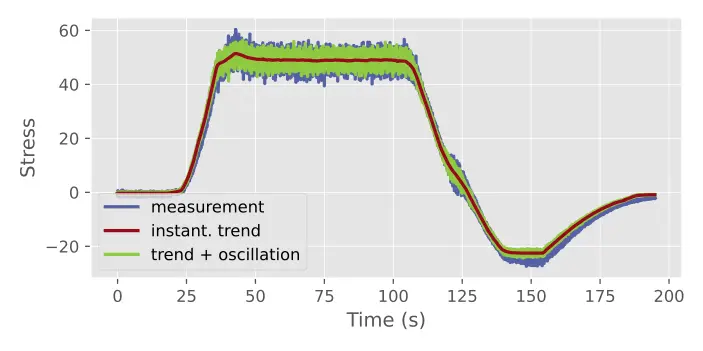


Impact
Hydropower already accounts for almost 60% of Swiss energy production, which is likely to increase with a shift to renewable energy in the future. By improving the life expectancy of hydraulic turbines, this project will lower the cost of maintenance and replacement of hydropower plants. Through collaboration with hydropower plant utilities such as EDF Hydro, the results of this project could find direct application in a full-size hydropower plant.
Presentation
Gallery
Annexe
Additional resources
Bibliography
- S.W. Gregg, J.P.H. Steele, and D. L. Van Bossuyt, 2017, Machine Learning: A Tool for Predicting Cavitation Erosion Rates on Turbine Runners, Hydro Reviews, 36(3).
- A. Lyutov, A. Kryukov, S. Cherny, D. Chirkov, A. Salienko, V. Skorospelov and P. Turuk, 2016, Modelling of a Francis Turbine Runner Fatigue Failure Process Caused by Fluid-Structure Interaction, IOP Conf. Series: Earth and Environmental Science, 49.
- A. Heng, S. Zhang, A.C.C. Tan, J. Mathew, 2009, Rotating machinery prognostics: State of the art, challenges and opportunities, Mechanical Systems and Signal Processing, 23(3) 724 – 739.
- M. A. Miner, 1954, Cumulative damage in fatigue. J. Applied Mechanics, 12 159 – 64.
- M Seydoux et al, Assessments of hydropower plants start-up sequences and equivalent runner damage under transient operation, IOP Conference Series: Earth and Environmental Science, 2022
Publications
Related Pages
More projects
ML-L3DNDT
BioDetect
News
Latest news
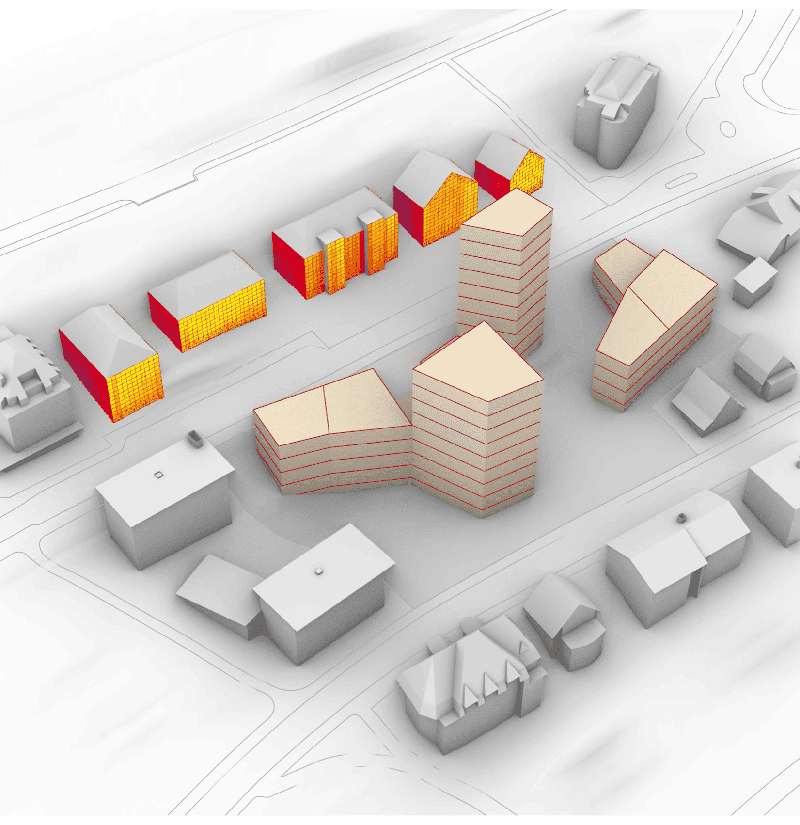
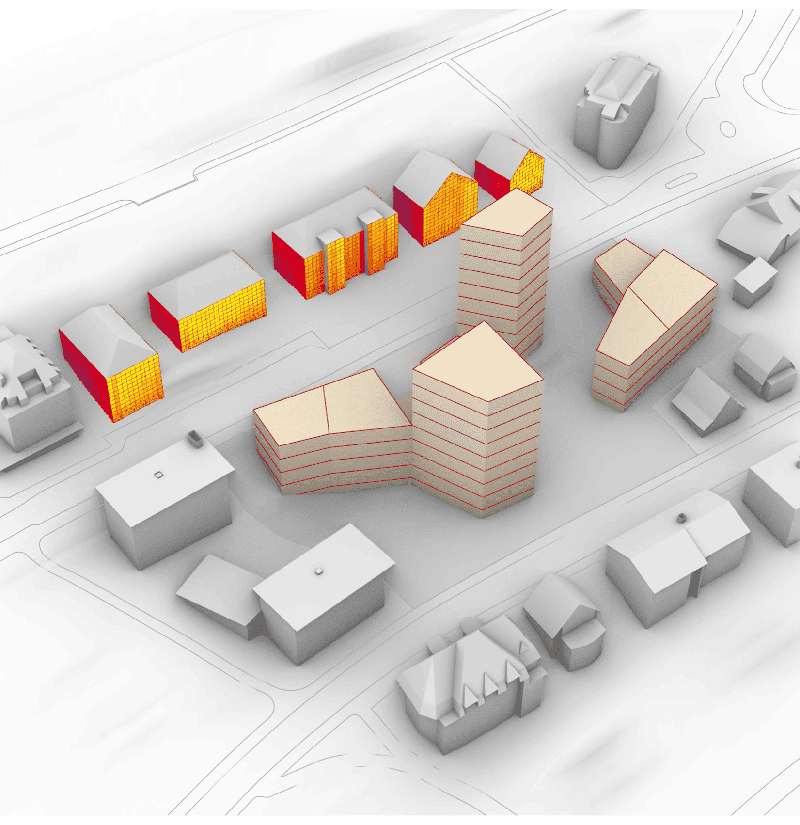
AIXD | Generative AI toolbox for architects and engineers
AIXD | Generative AI toolbox for architects and engineers


Smartair | An active learning algorithm for real-time acquisition and regression of flow field data
Smartair | An active learning algorithm for real-time acquisition and regression of flow field data
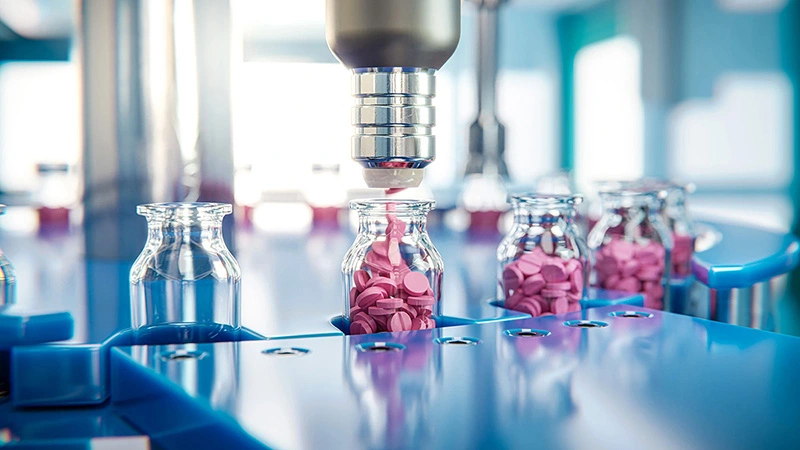
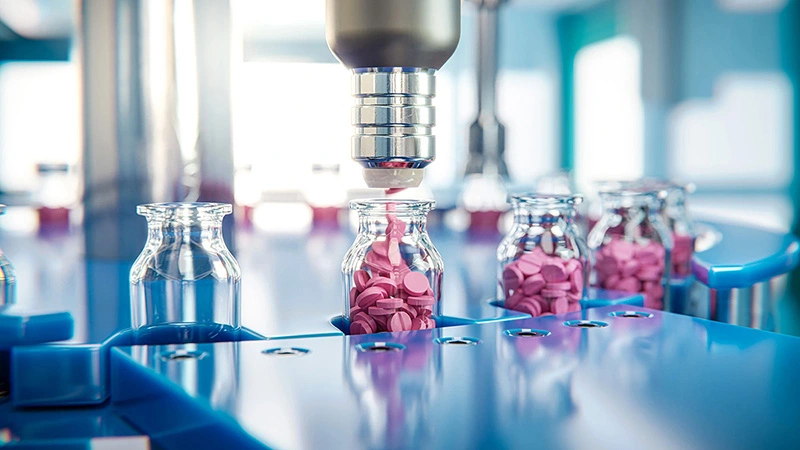
The Promise of AI in Pharmaceutical Manufacturing
The Promise of AI in Pharmaceutical Manufacturing
Contact us
Let’s talk Data Science
Do you need our services or expertise?
Contact us for your next Data Science project!