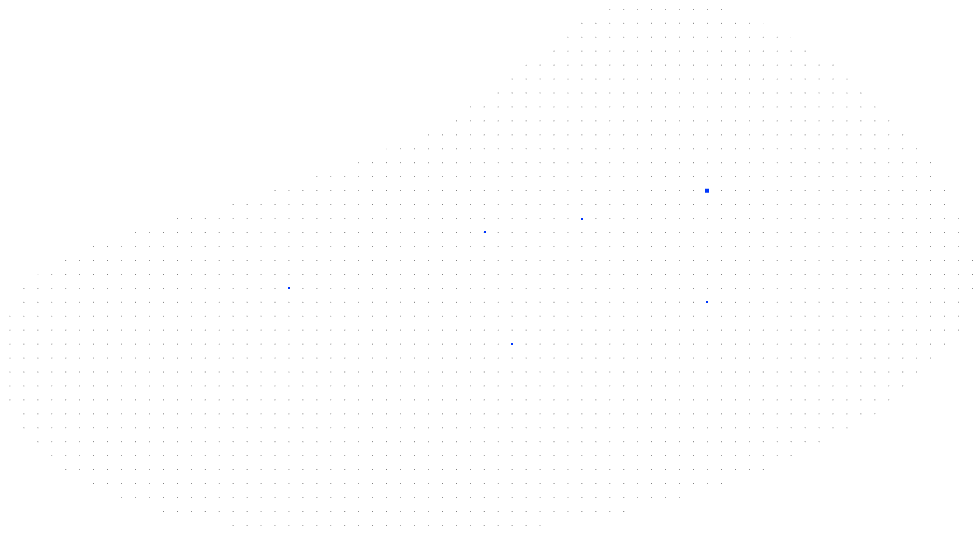
MAGNIFY
Machine learning Assisted larGe scale quaNtIfication of building energy FlexibilitY
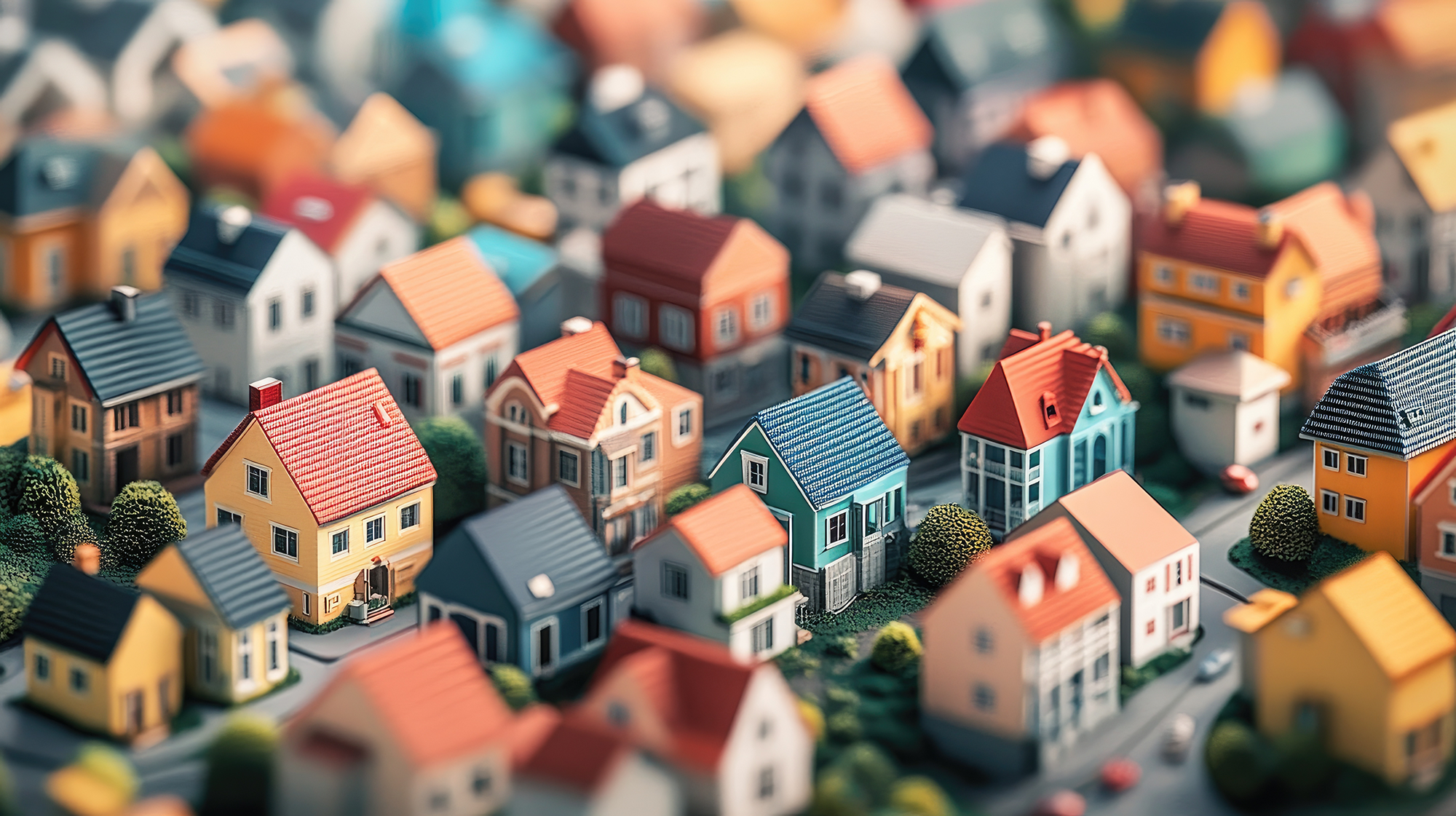
Abstract
With the increasing integration of intermittent renewable energy sources into power systems, there arises a significant challenge in energy system operation. Studies of Swiss and European energy transitions have shown that demand-side flexibility can greatly reduce electricity generation costs, cut down on wasted renewable energy, and lower CO2 emissions. This project aims to address this need for energy flexibility in large-scale energy infrastructure. Contrary to the traditional operation where energy supply follows demand, the increasing digitalization and building automation allows energy consumers to adjust consumption patterns. Such adjustments offer the much-needed flexibility, ensuring in continuous balance between supply and demand. However, flexibility quantification at the national level is needed to achieve an impact on the system, emphasizes the importance of scalable demand-side flexibility quantification. Achieving this in practice is challenging due to the absence of scalable pipelines, the complex flexibility quantification process, and the need for uncertainty-aware tools. Additionally, flexibility quantification needs to be periodically updated (e.g., 15 minutes for 2.3 million buildings), emphasizing the needs for computationally efficient methods. The role of SDSC is in model architecture selection, developing transferable machine learning pipelines and insights into practical deployment. The essential tasks include developing a scalable and uncertainty-aware time series forecasting model for energy flexibility, leveraging historical data (e.g., measured or simulated with in-house model repository).
People
Collaborators
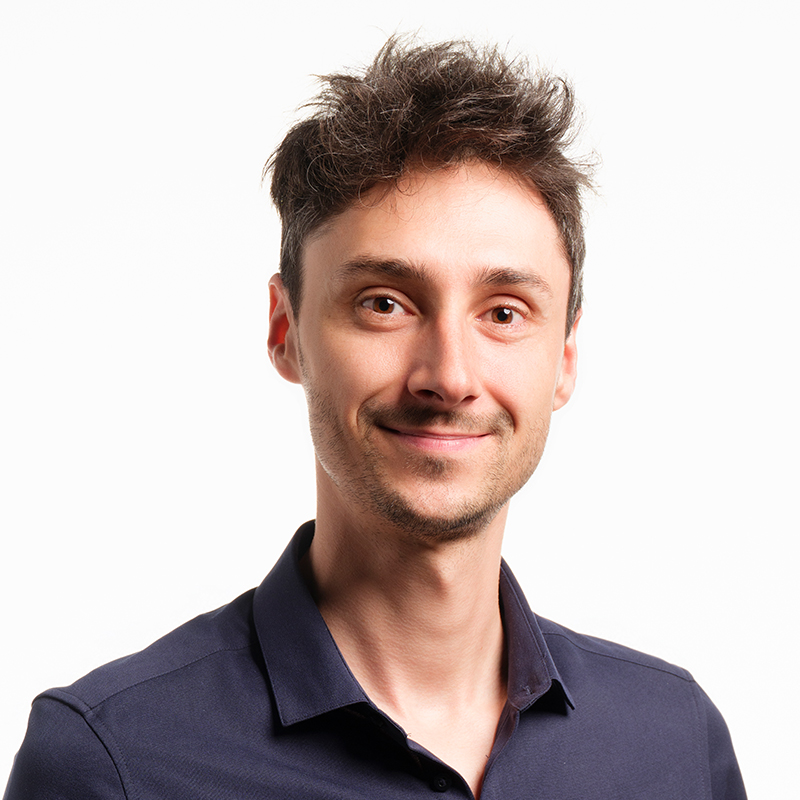
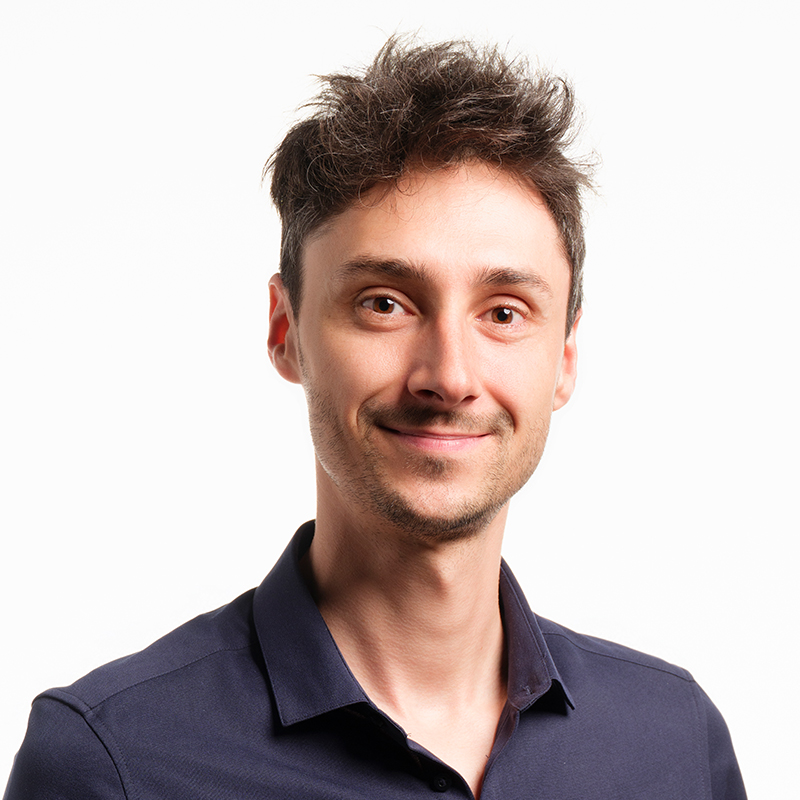
Carl holds a Ph.D in Mathematics from École des Ponts ParisTech and Université Gustave Eiffel in Paris. He has broad interests in statistics and stochastic control, and works on reinforcement learning, generative methods and time series forecasting, with applications in various domains such as energy, finance and physics. He worked with EDF R&D and Finance des Marchés de l’Energie (FiME) laboratory on applications of machine learning to risk management, including time series generation and deep hedging. He joined the SDSC in 2022 as a senior data scientist in the academic team at École Polytechnique Fédérale de Lausanne (EPFL).
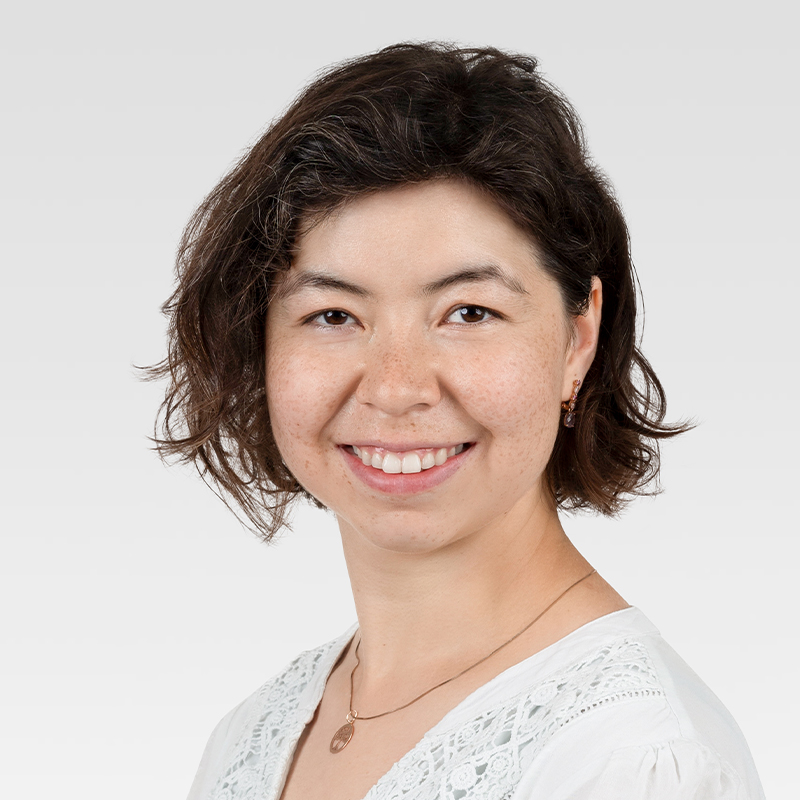
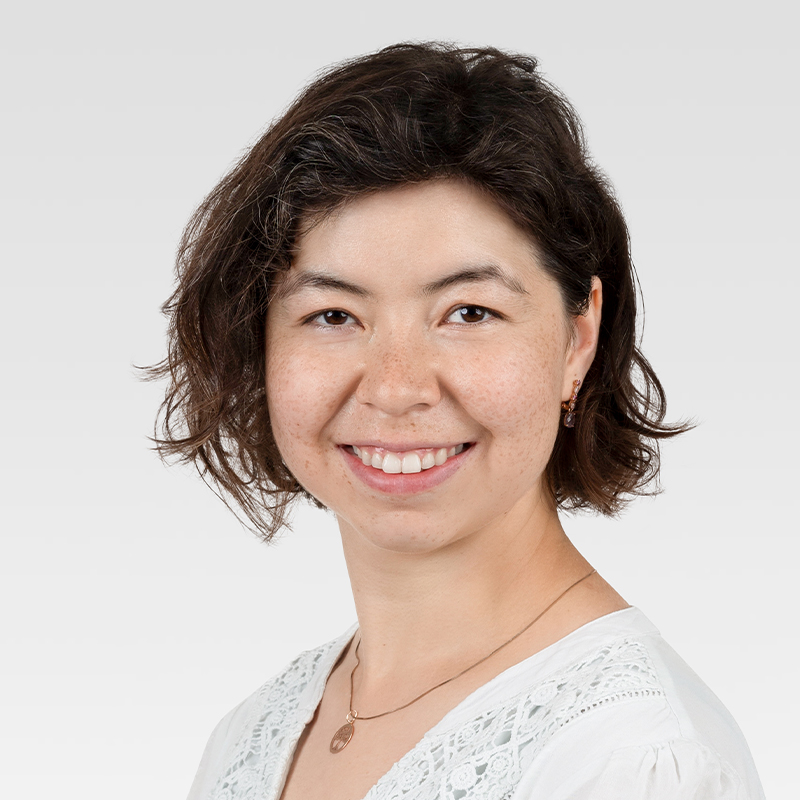
During 2023, Ilnura was doing a postdoc at the Technion, Israel, working with Prof. Dr. Kfir Levy. She received her PhD in September 2022 from the Automatic Control Laboratory (IfA) at ETH, Zürich. She joined IfA in 2017 to the Prof. Dr. Maryam Kamgarpour group and was co-supervised by Prof. Dr. Andreas Krause, working on safe learning methods. She obtained her Master's Degree jointly in Applied Mathematics at Institut Polytechnique de Grenoble (France) and Moscow Institute of Physics and Technology (Russia) in 2017. Her Master's thesis research was on stochastic optimization theory under the supervision of Prof. Dr. Anatoli Juditsky. Her Bachelor of Physics and Applied Math was obtained from the Moscow Institute of Physics and Technology (Russia) in 2015. Ilnura was born and finished high school in Almaty, Kazakhstan.
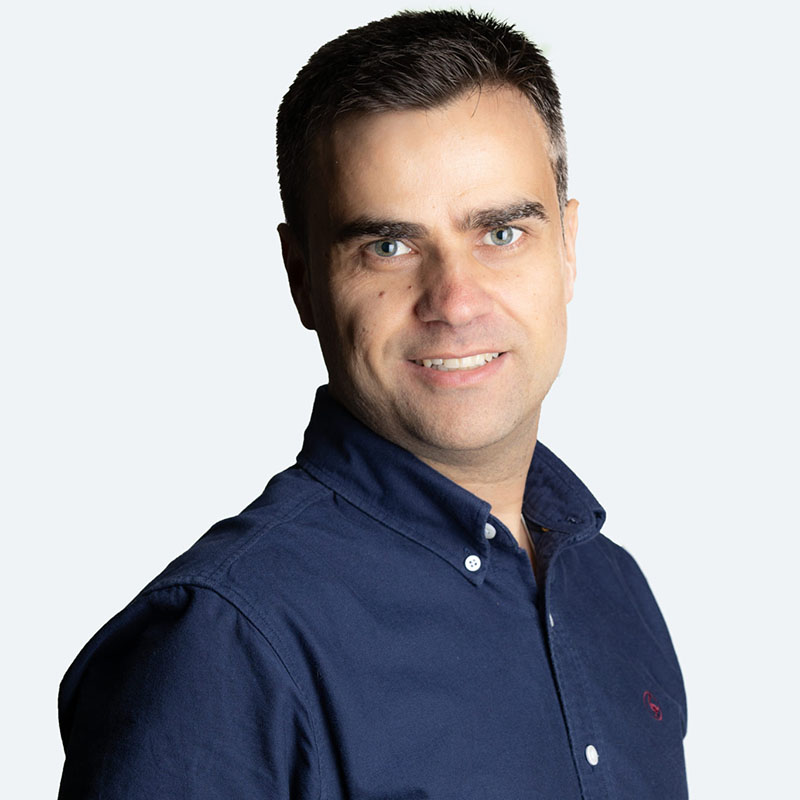
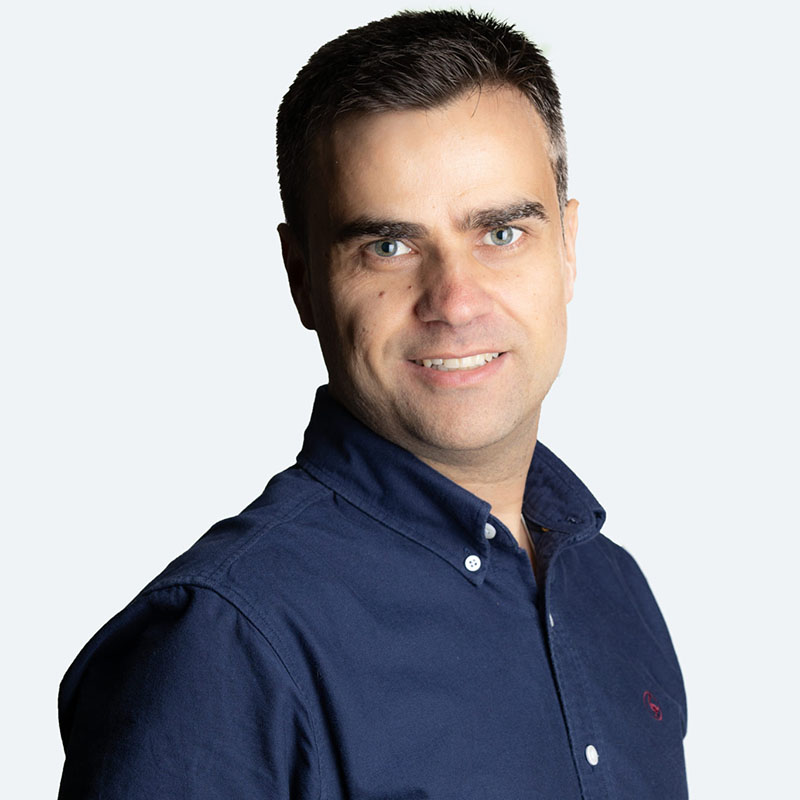
Benjamín Béjar received a PhD in Electrical Engineering from Universidad Politécnica de Madrid in 2012. He served as a postdoctoral fellow at École Polytechnique Fédérale de Lausanne until 2017, and then he moved to Johns Hopkins University where he held a Research Faculty position until Dec. 2019. His research interests lie at the intersection of signal processing and machine learning methods, and he has worked on topics such as sparse signal recovery, time-series analysis, and computer vision methods with special emphasis on biomedical applications. Since 2021, Benjamin leads the SDSC office at the Paul Scherrer Institute in Villigen.
PI | Partners:
EMPA, Urban Energy Systems Lab:
- Dr. Hanmin Cai
- Dr. Mina Montazeri
- Julie Rousseau
- Dr. Federica Bellizio
description
Motivation
The widespread use of renewable sources in power grids highlights the need for energy flexibility. One solution is leveraging flexibility through energy demand adjustments by consumers. Nationwide and scalable flexibility quantification becomes crucial for stable system operation. Although recent studies have advanced in their efforts to develop flexibility metrics, challenges remain in practical implementation. To this end, this project aims to develop a scalable and reliable data-driven pipeline for scalable demand-side flexibility quantification. More specifically, the pipeline involves advanced time series forecasting models. There are two main objectives:
- Objective 1: uncertainty-aware data-driven energy flexibility quantification.
- Objective 2: data-driven flexibility quantification on a large scale for practical deployment.
Proposed Approach / Solution
To address the scalability issue, one approach could be to replace the current optimization procedure by the data-driven deep-learning based predictions. Another approach could use the similarities between the consequent or similar optimization problems, and benefit from the warm starting, allowing for cheaper and faster updates.
To improve the uncertainty estimation, various uncertainty estimation approaches can be used, trained using various household datasets.
Impact
The outcomes of this project can support system operators to achieve a continuous balance between energy supply and demand. The topic treated in this project is strongly linked to several large-scale national research projects. Therefore, the outcome of this project can be integrated into a larger initiative and lead to more significant societal impacts.
Presentation
Gallery
Annexe
Cover image source: Adobe Stock
Additional resources
Bibliography
- Le Dréau, J. et al. Developing energy flexibility in clusters of buildings: A critical analysis of barriers from planning to operation. Energy and Buildings 300, 113608. issn: 0378-7788. https://www.sciencedirect.com/science/article/pii/S0378778823008381 (2023) (Dec. 1, 2023).
- Rousseau, J., Cai, H., Heer, P., Orehounig, K. & Hug, G. Uncertainty-aware energy flexibility quantification of a residential building in. ISGT 2023 (Belgrade, 2023).
Publications
Related Pages
More projects
News
Latest news
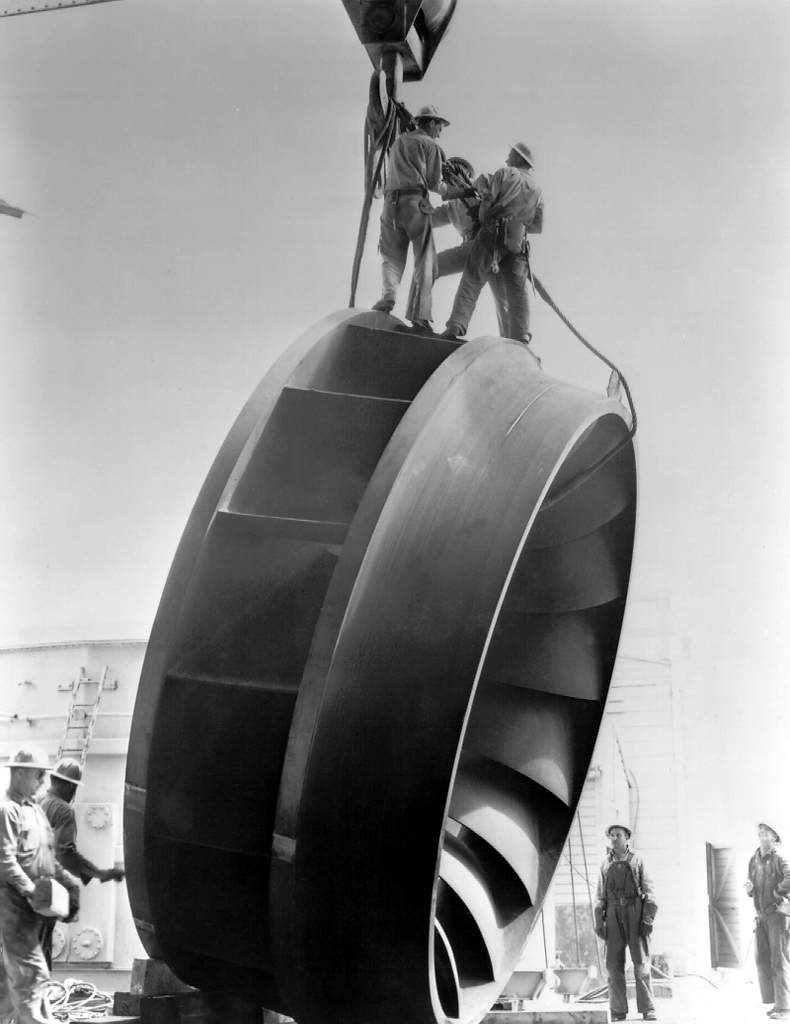
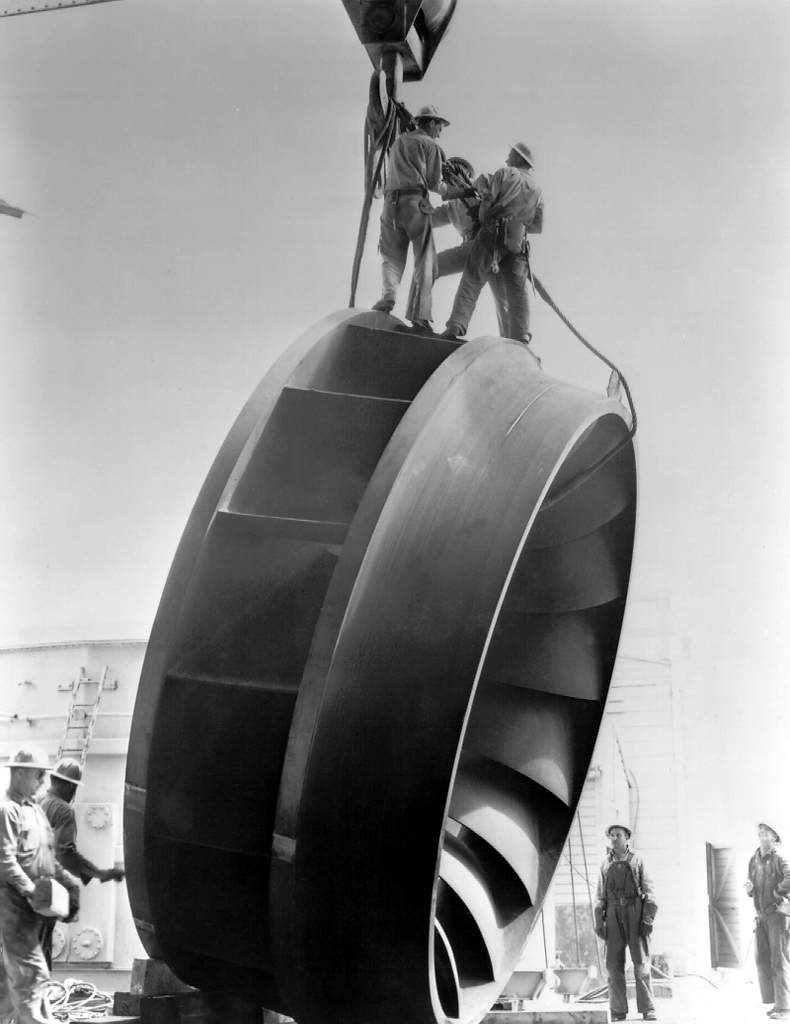
PAIRED-HYDRO | Increasing the Lifespan of Hydropower Turbines with Machine Learning
PAIRED-HYDRO | Increasing the Lifespan of Hydropower Turbines with Machine Learning


First National Calls: 50 selected projects to start in 2025
First National Calls: 50 selected projects to start in 2025
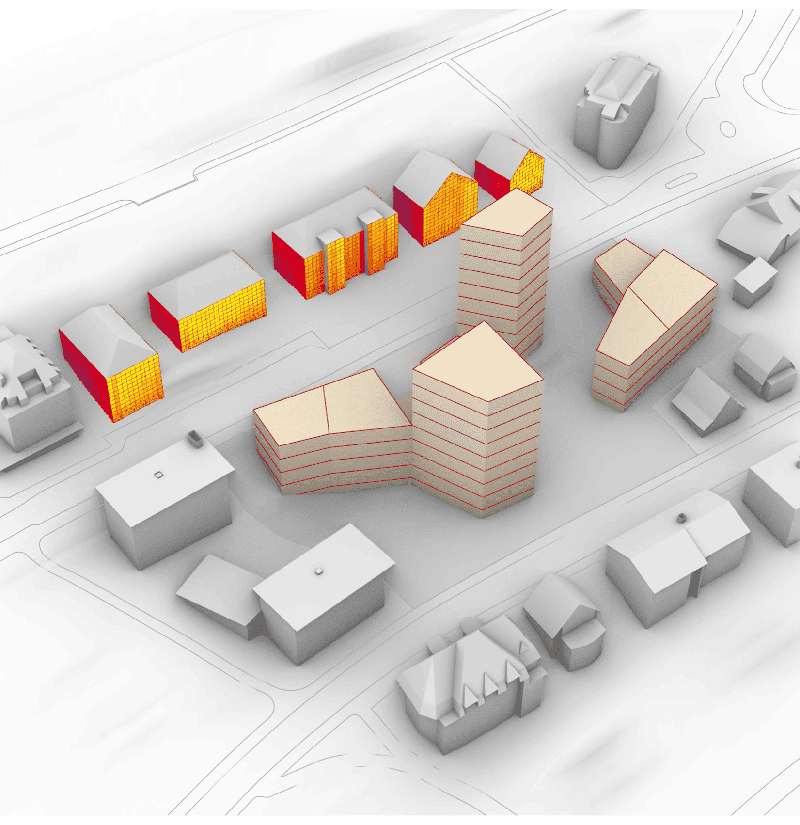
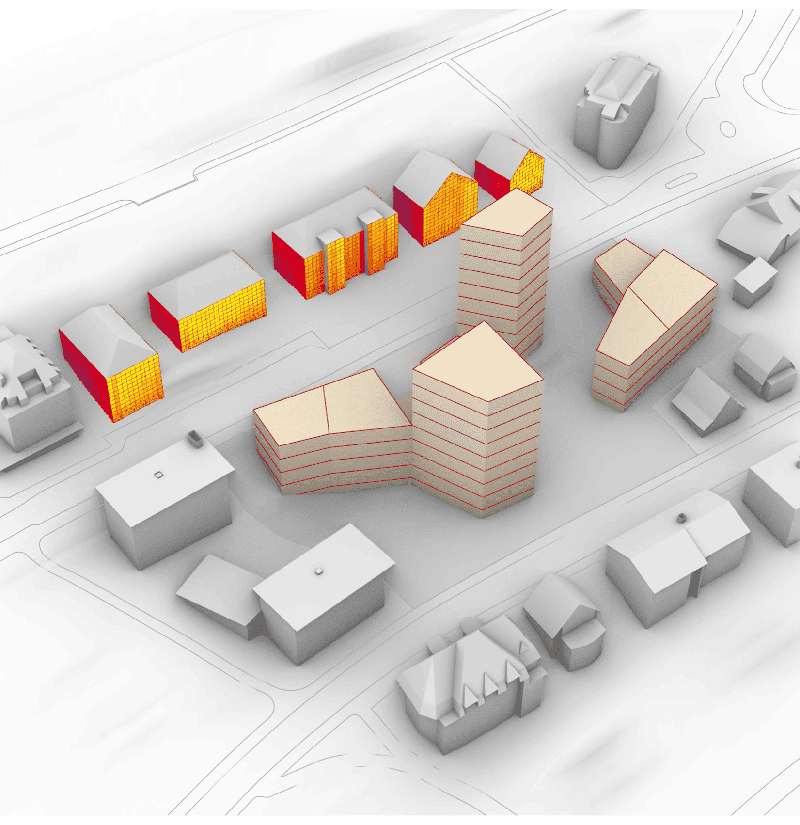
AIXD | Generative AI toolbox for architects and engineers
AIXD | Generative AI toolbox for architects and engineers
Contact us
Let’s talk Data Science
Do you need our services or expertise?
Contact us for your next Data Science project!