
Smart Waste Collection with AI-Empowered Planning


Christian joined the SDSC in July 2021 as a data scientist in the industry cell. Before that he worked at the Media Technology Center at ETH Zurich to develop new ML technology for their industry partners. He completed his Master's degree in Mathematics at ETH Zurich (2017) with focus on algorithmics and machine learning. After his studies he worked as a online marketing data analyst in the news publishing business. His expertise lies in statistics, NLP and software engineering.
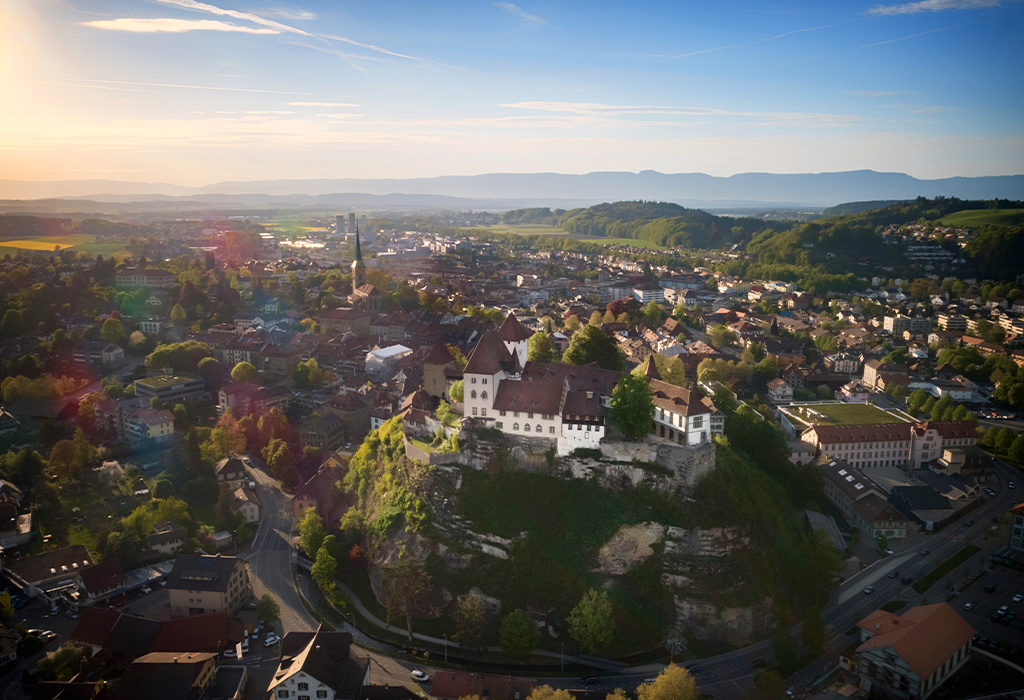
Context
Urban waste management faces inefficiencies from fixed collection schedules, often requiring unnecessary trips to half-full containers. Our collaboration focused on creating an AI-empowered routing system to help truck drivers dynamically adapt to real-time conditions, improving operational efficiency in the city of Burgdorf’s waste collection procedures.
Objectives
Working with the Swiss Data Science Center (SDSC) under an InnoSuisse mandate, the City of Burgdorf envisioned to gain efficiencies and resource-savings in their waste collection. The aim of the collaboration was to replace static schedules with adaptive algorithms that optimize waste collection based on container fill patterns, vehicle capacity, and other constraints. The goals included reducing distances, minimizing CO2 emissions, and enabling quick, reliable routes accessible to drivers with one click.
Solution
Based on existing schedule data, the team developed adaptive AI algorithms to address routing challenges. Adopting the Vehicle Routing Problem (VRP) to prioritizing full containers and incorporating partially filled ones emerged as a critical solution to increase efficiency. Using deep reinforcement learning (RL) further helped to minimize travel distances, container overfilling, and truck overloading. These innovative techniques produced optimal routes in under five seconds, ensuring real-time adaptability.
Simulations included diverse scenarios from Burgdorf, and for reasons of comparison were expanded to Emmental district, and Zurich City later in the project. Parameters like vehicle types, depot locations, and regional constraints were additionally integrated. As a result, the adaptive algorithms clearly outperformed static models, reducing collection distances by 24% in Burgdorf and up to 38% in Emmental.
The user-friendly platform Switzercloud by Brunata AG complemented the algorithms, offering real-time route planning via an app. Drivers could adjust routes dynamically, enhancing flexibility and resource optimization.
Impact
The dynamic routing system achieved a 24% reduction in collection distances (~500 km per year) in the City of Burgdorf, significantly lowering fuel consumption, CO2 emissions (~ 250 kg CO2 per year), and operational costs. The system’s real-time adaptability ensured efficient resource use without compromising service quality. A user-friendly interface allowed the operators and drivers to generate a dynamic schedule and route with a few clicks.
Collaboration
Next to their data, the city of Burgdorf team led by Andreas Rössler, Chief Digital Officer, provided valuable insights and testing grounds, ensuring that the solutions were aligned with practical needs. The collaboration between Brunata AG, Burgdorf, and SDSC played a central role in the project’s success: Brunata AG developed a user-friendly app to interface with the algorithms created by the SDSC. This partnership exemplifies how data-driven innovations can address real-world challenges, offering scalable and impactful solutions for urban waste management.
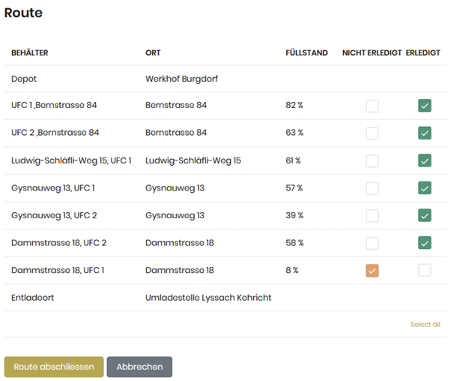
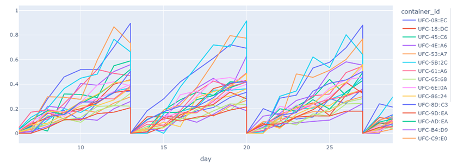
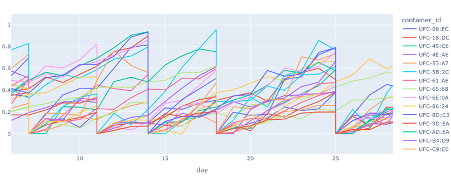
Cover image source: Stadtmarketing Burgdorf
More case studies
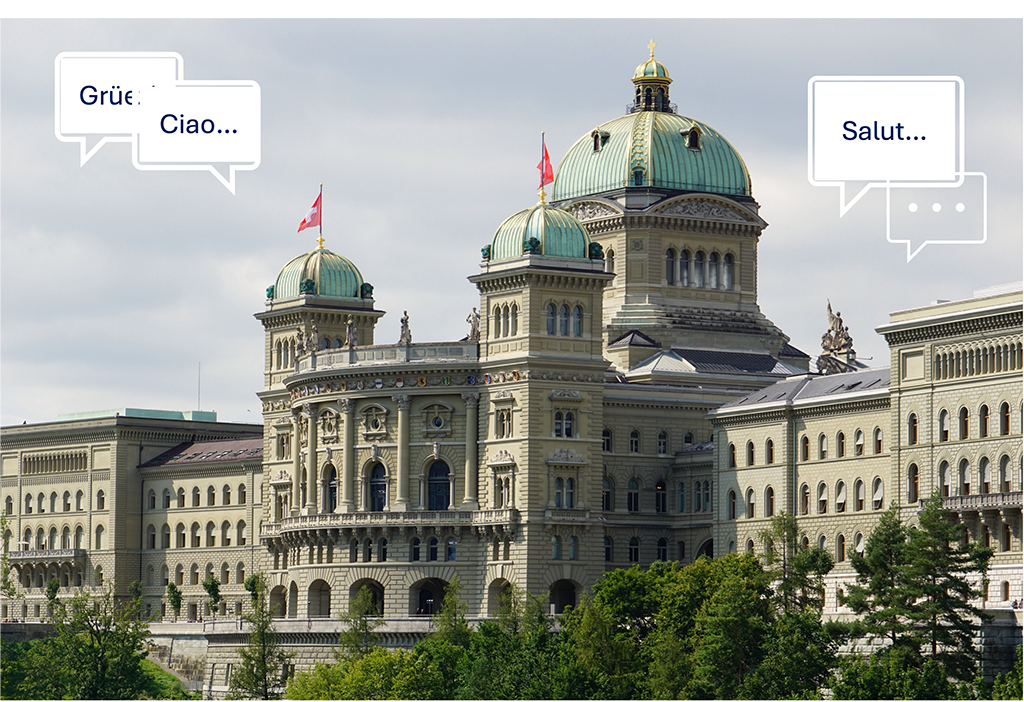
Enhancing Parliamentary Services with Generative AI

An artificial intelligence-based system for augmented cell & gene therapies
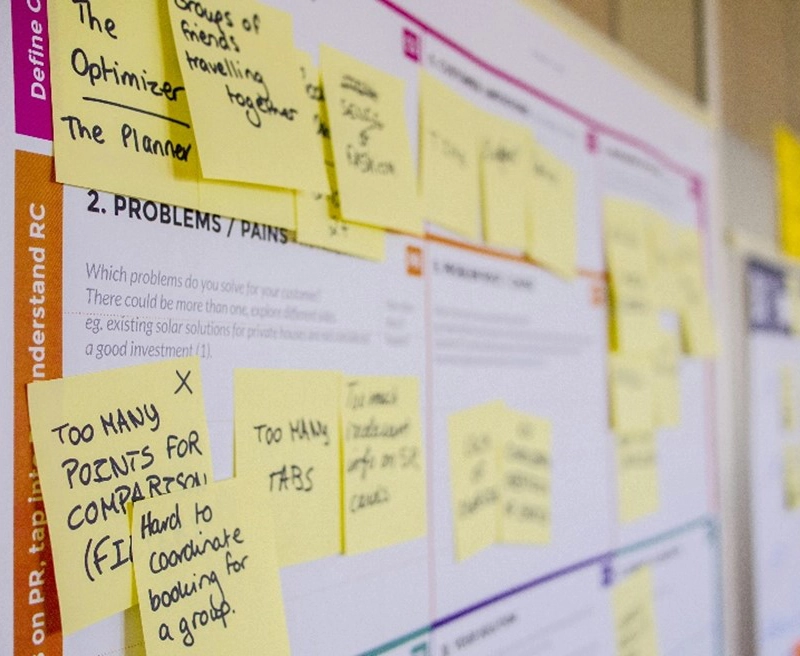
Qlaire: Enhance Quality Management with generative AI
Contact us
Let’s talk Data Science
Do you need our services or expertise?
Contact us for your next Data Science project!