Innovation in pharmaceutical manufacturing raises key questions: How will AI change our operations? What does this mean for the skills of our workforce? How will it reshape our collaborative efforts? And crucially, how can we fully leverage these changes?
The Urgent Call for Transformation
A recent survey by BCG involving 1,400 industry leaders revealed a striking insight: 66% are either ambivalent or dissatisfied with their progress towards integrating AI and GenAI into their operations [1]. This sentiment underscores a pressing need for digitalisation, particularly within the realm of pharmaceutical manufacturing. Moving towards smart connected factories within this sector could indeed yield substantial benefits, potentially saving over 20% of total costs by addressing issues such as (i) poor product quality, (ii) inefficient conversion of raw materials into drugs, and (iii) unreliable delivery processes [2].
Embracing digital transformation within this sector goes beyond mere cost reduction, as it holds the promise of addressing the challenges posed by the modern healthcare landscape. Key factors propelling this shift include (1) sustainability requirements, (2) evolving market dynamics, (3) the rise of personalised medicine, and (4) intensified competition from generic drugs [2]. Indeed, regulatory adjustments, coupled with a growing emphasis on sustainable production practices, pose a risk to pharma profits. Concurrently, the trend towards more frequent, albeit smaller, drug launches adds layers of complexity and increases production cost. The move towards personalised medicine, offering treatments tailored to individual patients, requires more complex processes. Moreover, the widespread use of generic drugs amplifies competitive pressure, simultaneously driving down prices.
The core goal of pharmaceutical manufacturing is the consistent production of high-quality Active Pharmaceutical Ingredients (APIs). This involves a sequence of chemical transformations or purifications, each requiring precision and control over numerous unit operations. These operations span from the initial selection of the synthetic route for the drug substance to the final packaging and labelling of pharmaceuticals for distribution [3]. Efficiency and scalability at each step are crucial to ensure companies’ profitability and maintenance of safety standards.
However, the journey towards digitalisation has several challenges. 85% of pharma executives consider integration of new and existing data systems as the main obstacle [2]. The need for behavioural change required for digital manufacturing and lack of required skills among employees are also two critical challenges to overcome [2]. Additional obstacles to wider adoption of AI include uncertainty around return on investment (ROI) and availability of data for modelling and continuous learning [4].

AI Spectrum in Pharma Manufacturing
In the realm of pharmaceutical manufacturing, the strategic incorporation of AI should be approached not as a binary decision, but as a spectrum of options that align with the system's capabilities and the desired level of integration between human and machine intelligence for collaborative decision-making (see Figure 2).

Description and diagnostics AI models capable of analysing vast amounts of data to identify patterns and anomalies. These models provide a comprehensive overview of current conditions or past events. They help humans to understand the 'what' and the 'why' behind certain occurrences. Prediction and prescription AI systems are designed to not only predict future trends and potential outcomes based on historical and real-time data but also to suggest optimal courses of action to achieve desired objectives. They utilise advanced algorithms and machine learning techniques to forecast future scenarios with a certain degree of probability. Assistance systems support these capabilities by being foundational AI systems that aid humans in decision-making without the ability to learn from past interactions. They are specific, hard-wired systems designed to support, not replace, human expertise. Augmentation represents an advanced form of AI that works in tandem with human intelligence, enhancing decision-making processes and continually learning from interactions with both humans and the environment. Automation focuses on the automation of manual and cognitive tasks, streamlining and refining existing tasks through automation, rather than redefining how tasks are done. Lastly, autonomy marks the pinnacle of AI evolution, where systems are capable of adapting to various situations and operating independently, without the need for human oversight.
AI for Operational Excellence in Pharmaceutical Manufacturing
The main processes where AI has an impact in pharmaceutical manufacturing are summarised in Table 1. AI models for predictive maintenance, quality control, and supply chain management help to achieve operational excellence while meeting regulatory standards and sustainability goals.
Table 1. Where can AI fit pharmaceutical processes?
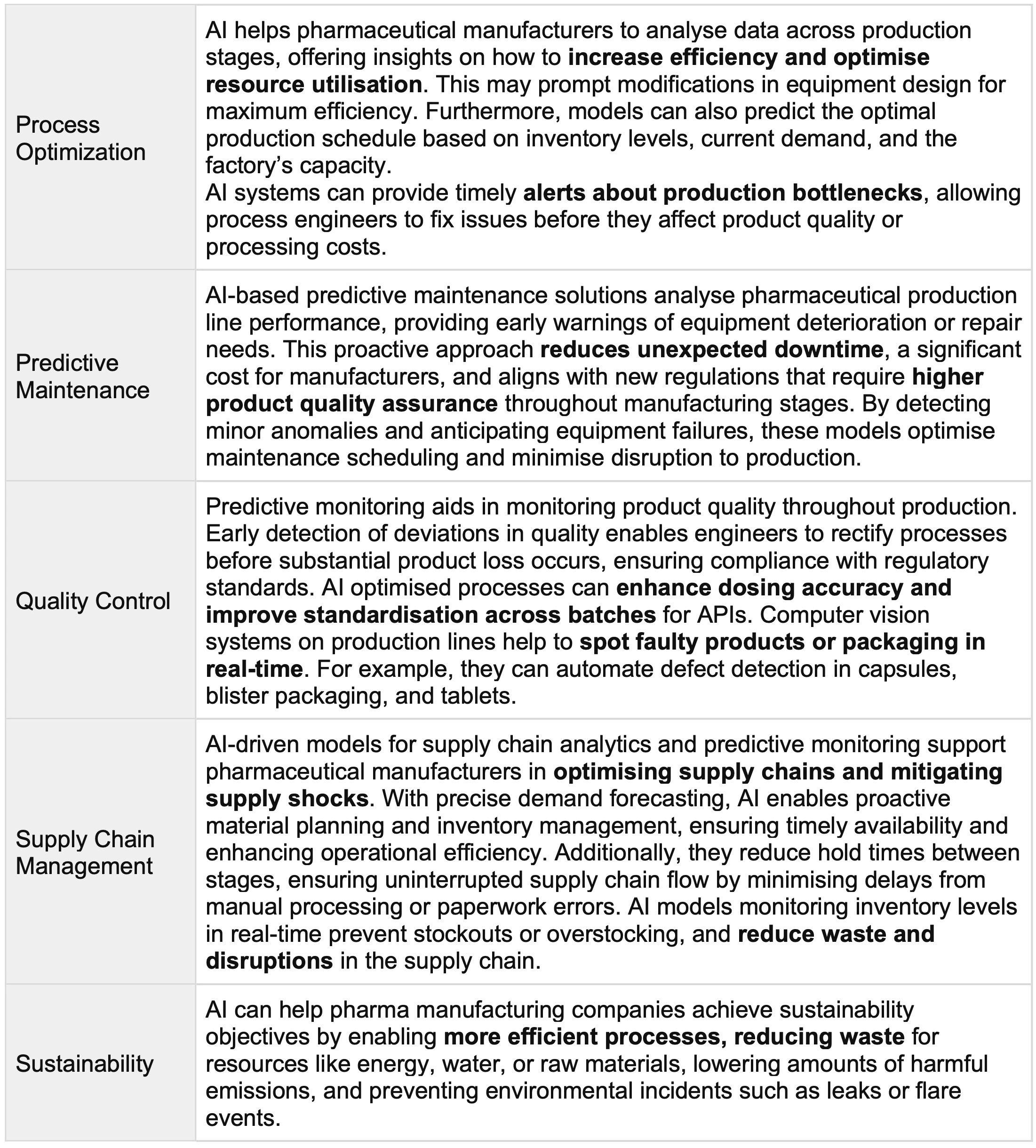
According to McKinsey [6], digitally enabled labs utilise real-time data analytics and process verification to enhance efficiency and prevent deviations (Figure 3). They employ digital tools like smart glasses for visual guidance and create digital twins to predict impact. Automated labs, on the other hand, rely on robotics and advanced automation for repeatable tasks and online testing. Distributed quality control represents a significant shift, enabling real-time release testing on production lines. However, the adoption of process analytical technology and real-time release testing has been slow due to regulatory hurdles.
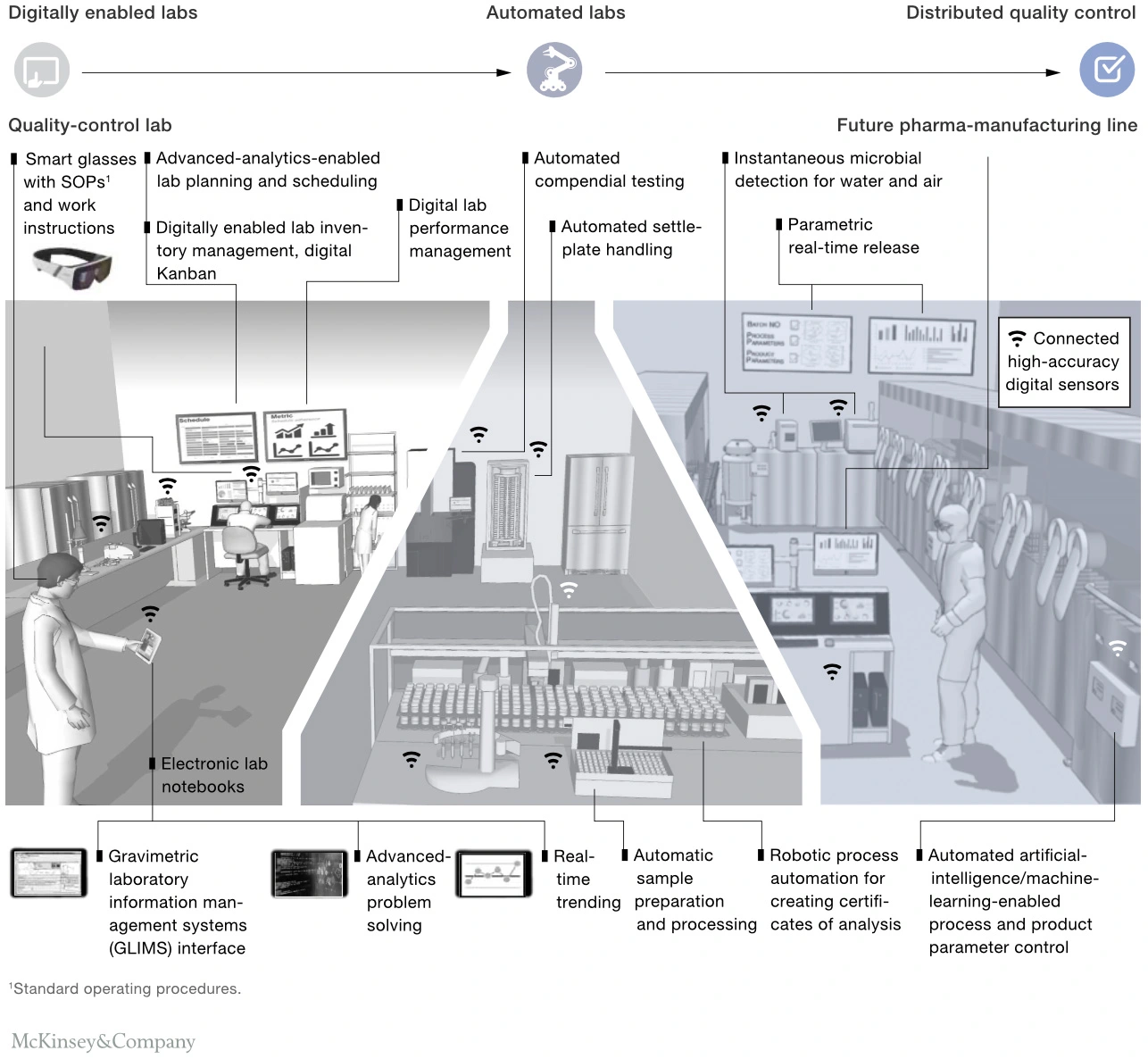
Use case: Automated visual inspection of capsules and tablets defects
In the realm of pharmaceutical manufacturing, ensuring the quality of capsules and tablets is of utmost importance. Both capsules and tablets are vulnerable to a range of imperfections arising from equipment malfunctions. These imperfections, spanning from dents to being missing or broken (Figure 4), present notable obstacles to the industry, resulting in revenue loss, increase in manufacturing and resource costs, and potential harm to a manufacturer's reputation [7]. Historically, quality assurance has relied on manual inspection - with inconsistency, cost, quality, and objectivity drawbacks.

To address these challenges, the industry has turned to automated quality inspection systems leveraging computer vision. Deep learning models and image segmentation are deployed to analyse digital video images captured by cameras, enabling real-time inspection of the product with high accuracy [10, 11]. These AI-driven systems offer a more objective, consistent, and cost-effective alternative to manual inspection.
The adoption of computer vision and machine learning technologies for quality inspection in pharmaceutical manufacturing has yielded significant benefits [10, 11]. In this use case, the image processing system meets the demand of inspecting 1000 capsules per minute with 95% accuracy [8]. This method not only maintains high throughput but also meets accuracy, cost, and speed requirements. Ultimately, these advancements contribute to the industry's competitiveness and reputation while ensuring the safety and efficacy of pharmaceutical products for consumers worldwide.
How to get started?
To embark on the path towards successful implementation of AI, strategic planning and decisive actions are essential. Organisations can benefit from AI's transformative potential by following four key practices during the adoption of innovation: (1) approaching the transition as business transformation, (2) adopting a practical strategy, (3) enhancing digital skills of the workforce, and (4) learning from diverse domains to foster cross-pollination of ideas (Figure 5). These practices set the foundation for successful AI adoption by aligning innovation with business goals and preparing the organisation for the transformative potential of AI.
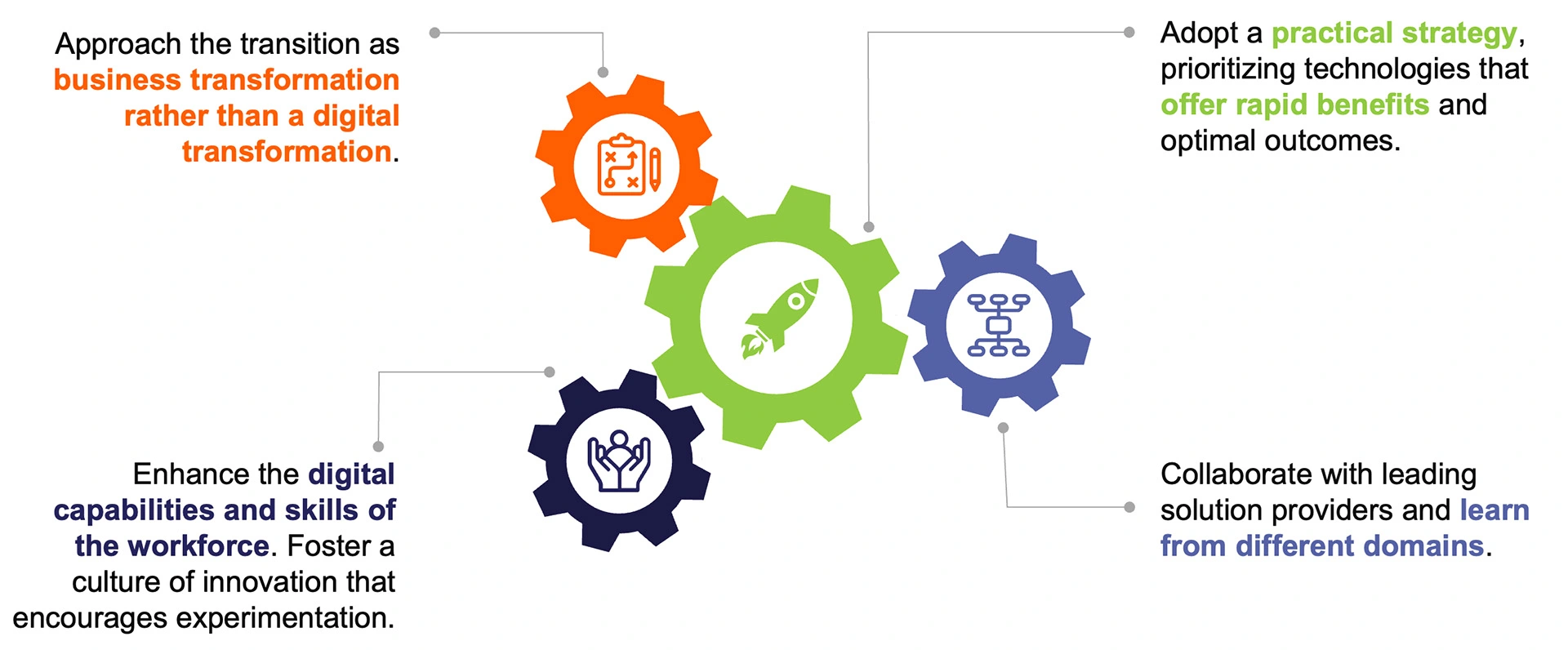
Building upon these practices, there are six pillars crucial for the successful implementation of AI (Figure 6):
- Business Application: Begin by exploring use cases and AI applications to identify those with the highest potential for impact within your organisation. Pilot projects can serve as valuable learning opportunities, providing insights into the feasibility and benefits of integrating AI into various business processes.
- Data: Invest in robust data collection, aggregation, and cleansing processes to build a high-quality dataset, foundational for AI-driven insights. Prioritise data security, privacy, and compliance measures to safeguard sensitive information and adhere to regulatory requirements. Embrace FAIR principles to ensure your data is Findable, Accessible, Interoperable, and Reusable, and consider leveraging external data sources to enrich your dataset.
- Technology-wise, implementing Manufacturing Execution Systems (MES) streamlines production processes, while Quality Management Systems (QMS) ensure compliance and product quality. Additionally, consider integrating electronic batch records to improve traceability in manufacturing operations.
- Talent and Organization: Cultivate a culture of innovation and continuous learning within your organisation. Leverage domain experts' expertise to guide AI implementation and engage data scientists, engineers, and architects to develop AI applications extracting actionable insights from data. Consider appointing a Chief Data Officer to oversee AI initiatives' strategic direction and governance.
- Process: Streamline and optimise existing processes to accommodate AI-driven workflows and methodologies. This involves defining clear roles and responsibilities, implementing agile methodologies, and integrating AI into existing business processes to maximise efficiency and effectiveness.
- Culture: Embrace an experimental mindset to foster innovation and commit to continuous improvement, emphasising data-driven decision-making. Build trust in data and algorithms by ensuring transparency, interpretability, and robustness in AI systems, enhancing confidence in AI-driven insights and outcomes.
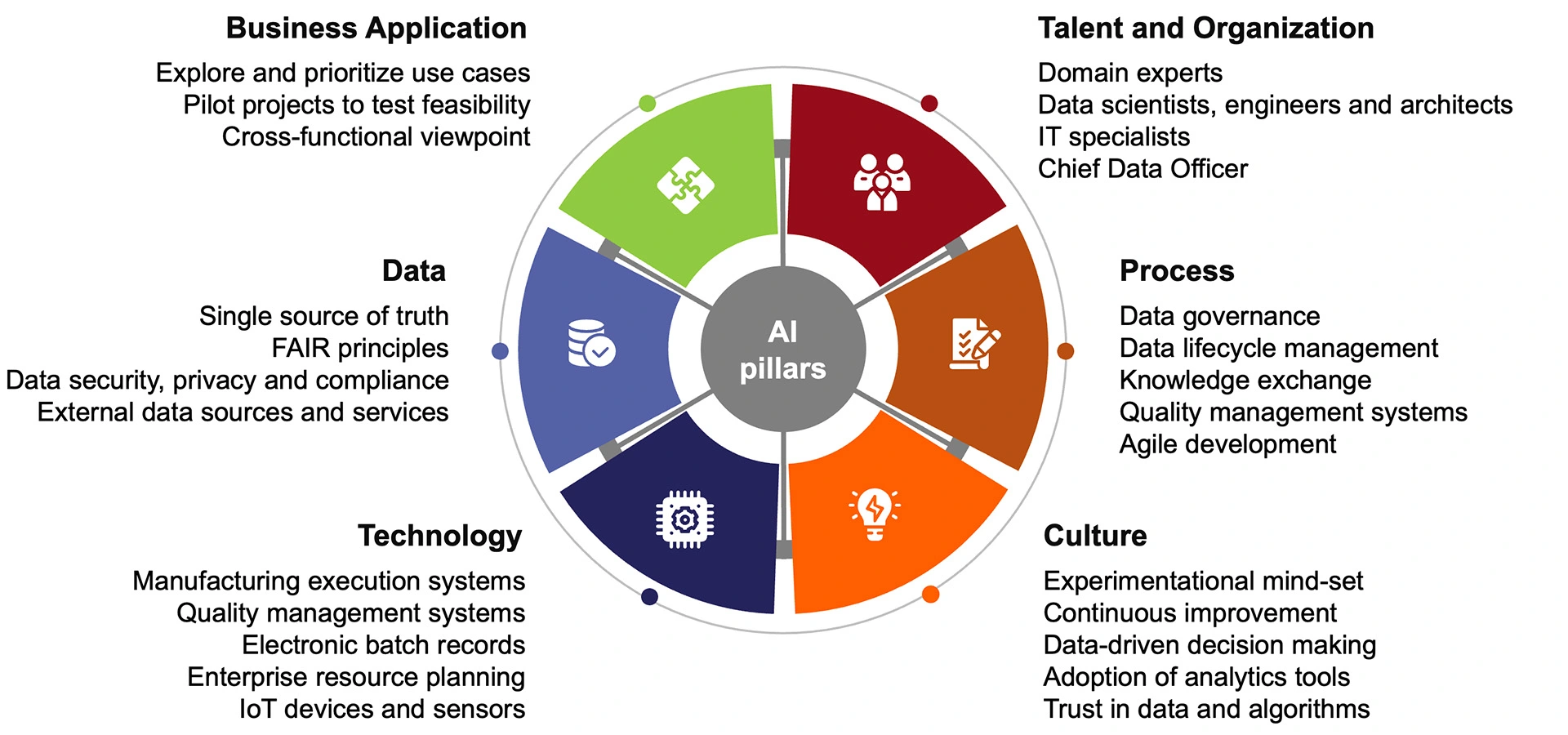
Adhering to these fundamental pillars, organisations can lay the groundwork for successful AI implementation, positioning themselves as leaders in leveraging AI to drive innovation and efficiency.
References
- Apotheker J. et al. (2024) “From Potential to Profit with GenAI” BCG AI Radar, https://www.bcg.com/publications/2024/from-potential-to-profit-with-genai
- Lesmeister F. and Ghosh P. (2020) "Digital Manufacturing Is (Finally) Coming to Pharma” Bain & Company BRIEF, https://www.bain.com/insights/digital-manufacturing-is-finally-coming-to-pharma/
- Owczarek D. (2021) “The Future of Pharmaceutical Manufacturing Process: Artificial Intelligence” Nexocode article, https://nexocode.com/blog/posts/ai-in-pharmaceutical-manufacturing/
- PwC’s technical report on AI (2018) “The macroeconomic impact of artificial intelligence” https://www.pwc.co.uk/economic-services/assets/macroeconomic-impact-of-ai-technical-report-feb-18.pdf
- PwC’s AI analysis (2017) “Sizing the prize – What’s the real value of AI for your business and how can you capitalize” https://www.pwc.com/gx/en/issues/analytics/assets/pwc-ai-analysis-sizing-the-prize-report.pdf
- Makarova E., Ringel M., Telpis V. (2019) “Digitization, automation, and online testing: The future of pharma quality control” McKinsey & Company Article, https://www.mckinsey.com/industries/life-sciences/our-insights/digitization-automation-and-online-testing-the-future-of-pharma-quality-control#/
- Boesch G. (2023) “Applications of Computer Vision in the Pharmaceutical Industry” viso.ai applications, https://viso.ai/applications/computer-vision-in-the-pharmaceutical-industry/
- Islam M. J., Basalamah S. M., Ahmadi M. & Sid-Ahmed M. A. (2012) “Computer Vision-Based Quality Inspection System of Transparent Gelatin Capsules in Pharmaceutical Applications” AJIS 2, 14–22. doi:10.5923/j.ajis.20120201.03
- Patgiri R. (2023) “Quality Assurance of Blister Package Dataset” Roboflow Universe, https://universe.roboflow.com/ripon-patgiri-hvvxc/quality-assurance-of-blister-package
- Galata D. L. et al. (2021) “Applications of machine vision in pharmaceutical technology: A review” European Journal of Pharmaceutical Sciences 159, 105717. doi:10.1016/j.ejps.2021.105717
- Zhao X. (2021) “A Computer Vision System for Missing Tablets Detection” J. Phys.: Conf. Ser. 1827, 012029. doi:10.1088/1742-6596/1827/1/012029