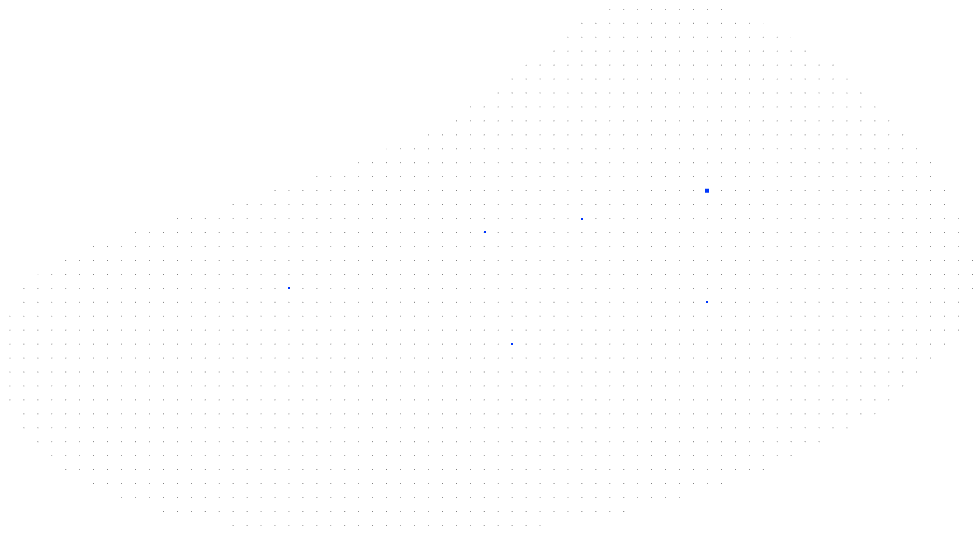
DDForms
Data driven fast optimization of resonant metamaterial structures

Abstract
In recent years, resonant metamaterials have garnered much interest because of their potential to break with traditional design principles in noise and vibration management. By virtue of a carefully designed micro- or mesostructure, metamaterials display exotic macroscopic properties such as vibrational band gaps, which do not exist in known bulk materials. However, modeling and optimizing these emergent properties is a challenging multiscale problem. The process remains computationally intractable in many cases due to the heavy reliance on expensive finite-element analyses and/or topology optimizations.To address this challenge, the present project focuses on applying data science techniques in the design of resonant metamaterial structures. In particular, we target vibration reduction in finite-sized elastic plates by integrating 3D-printed mechanical resonators, which is treated as an optimization problem in both the arrangement and the dynamics of the resonators. Where possible, costly high-fidelity simulations and optimizations will be replaced with data-driven surrogates to drastically reduce the computational load. On the one hand, the inverse design problem of creating resonators with a desired dynamic response will be addressed, for instance by applying tandem neural networks. On the other hand, we will investigate the optimal arrangement of resonators for maximum vibration reduction. Here, Bayesian optimization techniques may offer an efficient solution. The range of material and geometric properties obtainable via the 3D-printing process will be identified and taken into account.
People
Collaborators


Christian joined SDSC in 2019 to design data-driven solutions to support academic research projects. His research interests comprise inference of probabilistic models, with a focus on nonparametric models, and stochastic processes. He holds a PhD in Computational Neuroscience from the Technical University Berlin, Germany, a MSc from the Bernstein Center for Computational Neuroscience Berlin, and a BSc in Cognitive Science from the University of Osnabrück, Germany. During the course of his studies, he additionally conducted research at Khalifa University (UAE), Strathclyde University (UK), and the RIKEN Brain Science Institute and the Kyoto University in Japan. Before joining SDSC, he was an intern at Amazon CoreAI in Berlin.
description
Motivation
In this project, we aim to reduce the vibration level of finite-size elastic plates by integrating mechanical resonators. This approach - based on locally-resonant metamaterials design - offers lightweight, economical, and flexible vibration reduction for high-end applications such as precision machinery and aerospace components. By using machine learning, we wish to bypass the costly design procedure used normally.
Proposed Approach / Solution
We tackle two problems in this project: 1) Positioning and designing multiple resonators on a host structure such that response to vibrations is minimized (Figure 1). 2) Designing a resonator, that dampens predefined frequencies (Figure 2). For the first problem, we use a sampling technique called simulated annealing to find the optimal positioning. This is a stochastic optimization algorithm, that adds, removes, and moves resonators on the host-structure randomly. Changes that dampen the vibrations are more likely to be accepted. In this way, the configuration gets incrementally improved, while still allowing the exploration of many different configurations. For the second problem, we use a conditional variational autoencoder (cVAE). A cVAE is composed of an encoder and a decoder (Figure 3). The encoder maps the resonator design into a latent space. The decoder takes this mapping, and additionally specific user-specified resonant properties, and outputs a new design. With the trained model, the decoder can be used to propose many new designs with on-demand resonant properties, that can be 3D printed and tested for their actual resonant properties (Figure 4).
Impact
We find data-driven techniques that allow us to design materials that are less prone to be damaged by resonant frequencies. This can help with several problems, including manufacturing materials with certain on-demand properties.




Presentation
Gallery
Annexe
Publications
- Dedoncker, S., Donner, C., Taenzer, L., & Van Damme, B. (2023). Generative inverse design of multimodal resonant structures for locally resonant metamaterials. arXiv preprint arXiv:2309.04177. DOI: 10.48550/arXiv.2309.04177
- Dedoncker, S., Donner, C., Taenzer, L., & Van Damme, B. (2022). Optimization of resonant absorbers for passive vibration control: a numerical approach and its experimental validation. Available at SSRN 4266071. DOI: 10.2139/ssrn.4266071
Additional resources
Bibliography
Publications
Related Pages
More projects
ML-L3DNDT
BioDetect
News
Latest news


Smartair | An active learning algorithm for real-time acquisition and regression of flow field data
Smartair | An active learning algorithm for real-time acquisition and regression of flow field data
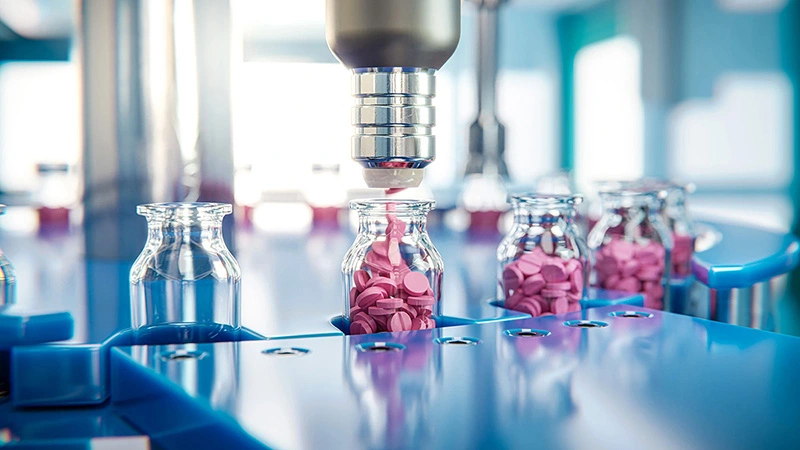
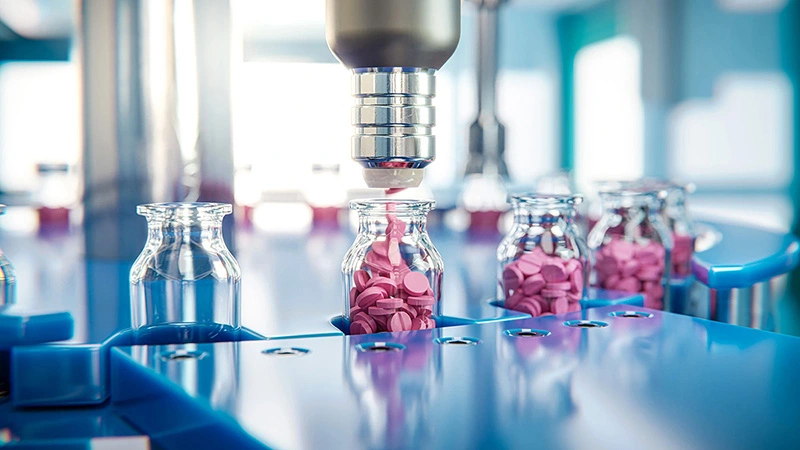
The Promise of AI in Pharmaceutical Manufacturing
The Promise of AI in Pharmaceutical Manufacturing


Efficient and scalable graph generation through iterative local expansion
Efficient and scalable graph generation through iterative local expansion
Contact us
Let’s talk Data Science
Do you need our services or expertise?
Contact us for your next Data Science project!