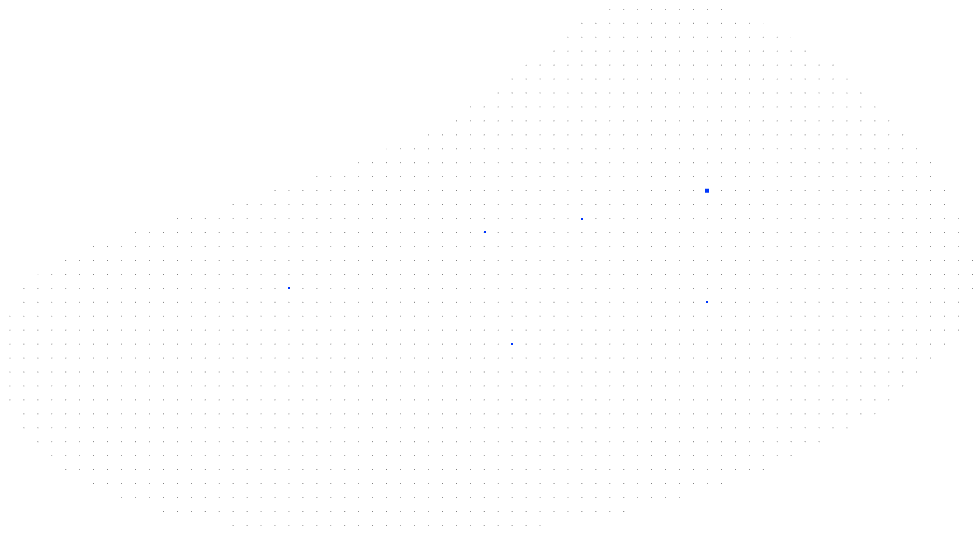
deepLNAfrica
Mapping changes in urban informal settlements in sub-Saharan Africa using deep learning and open-access satellite imagery

Abstract
Tackling inequalities and reducing poverty require timely measurements of socioeconomic development at high spatial resolution. This is a substantial challenge, even in developed parts of the world, due to costly and infrequent data collection methods used for socioeconomic measurements. Availability of such data is even more challenging in low- and middle-income countries (LMICs), particularly in sub-Saharan Africa (SSA). Over the past decade, researchers have investigated the potential for using emerging sources of large-scale data, such as satellite imagery and deep statistical learning, for advancing how we make measurements in data- poor contexts. Those studies, however, consist of cross-sectional proof-of-concepts at low spatial resolution that are not readily available to be used as input to development research or practice. This project aims to widen the adoption of deep learning methods and the use of imagery data in development economics. In this project, we will build on existing computer vision, machine and deep learning methods for extracting socio-economical measurements from Earth observation imagery data at high spatial resolution for multiple years in SSA. These data will then be used in ETH-DEC’s ongoing and future research, as well as shared with the wider community.
description
Motivation
In sub-Saharan Africa, more than 50% of urban residents reside in informal settlements, and are projected to number 1.3 billion people by 2050. Identifying and mapping the locations of informal settlements at scale and tracking their development over time is thus crucial for adequate policies to alleviate urban poverty and inequality. However, given the rapid and often unplanned urbanization dynamics in African cities, existing monitoring systems are insufficient for tracking the development of informal settlements timely and accurately. This project aims to alleviate these problems by introducing a cost-effective data-driven solution based on open-access Earth observation data that allows to accurately map informal settlements across space and time.
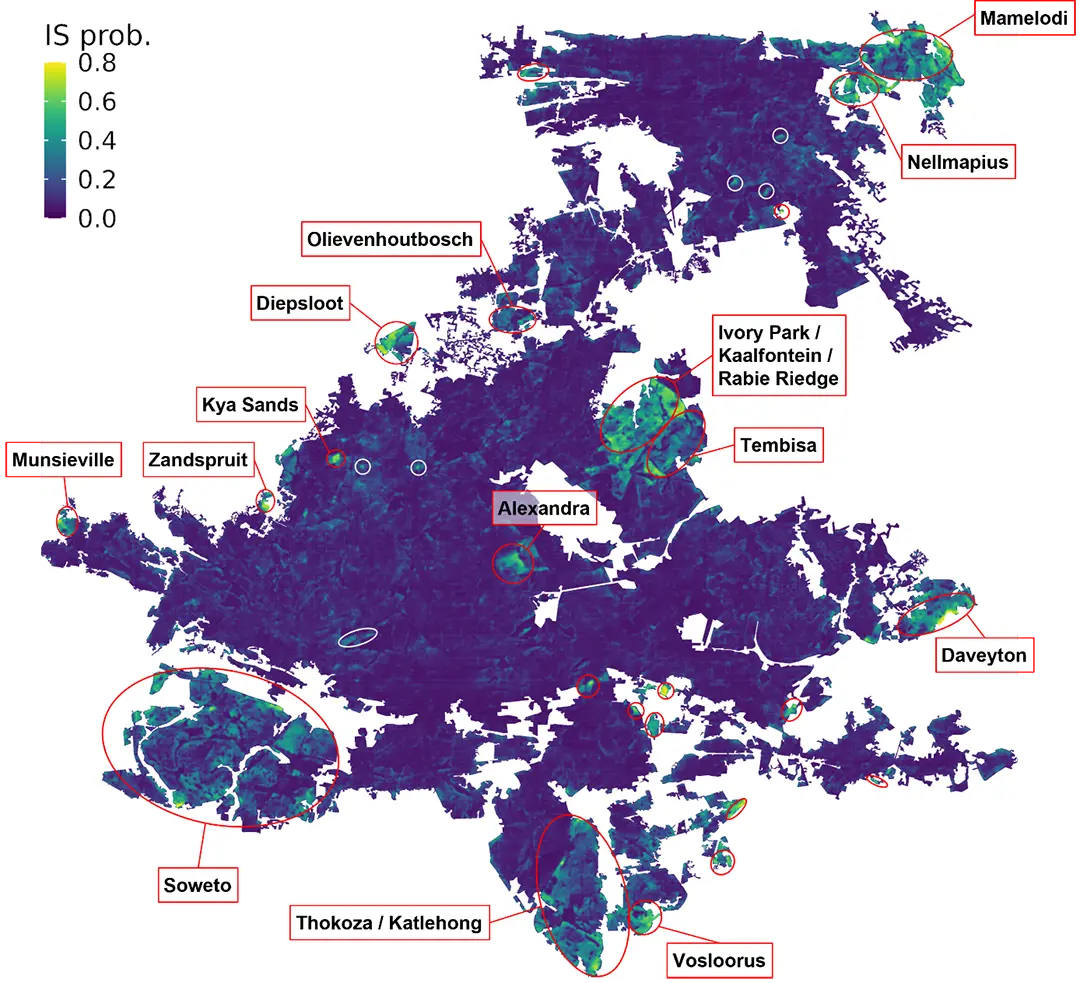
Proposed Approach / Solution
We use open-access Sentinel-2 satellite imagery with machine learning in order to learn the visual characteristics of informal settlements, as seen from above. First, unlabeled satellite images are used to pretrain a semantic segmentation neural network to become invariant to visual effects irrelevant to the task of informal settlement detection, such as those caused by atmospheric distortions, sun angle and acquisition geometry, weather conditions, and seasonality. In a second step, this model is fine-tuned on ground truth data from 16 cities within 6 different sub-Saharan countries that spatially describe informal settlements. To tackle the difficult challenge of mapping informal settlements in cities and countries where no training data was available, many different models are trained on subsets of the data and their predictions ensembled for increased robustness and decreased bias during inference. Using this setup, informal settlements were mapped in all 529 sub-Saharan African cities with a population of at least 100’000 for the years 2016 and 2022. See Figure 1 for an example prediction in a single location and point in time, and Figure 2 for our aggregated predictions for the share of the urban population living in informal settlements across all sub-Saharan African countries.
Impact
The solutions proposed in this project bring great improvements in spatial and temporal coverage of urban informal settlements when compared to established methods. Furthermore, it provides first-time estimates of the share and number of people living in informal settlements across all sub-Saharan African cities exceeding a population count of 100’000. This project demonstrates how the mapping of urban informal settlements could be revolutionized in SSA and across the world but also underlines a great need for standardized high-quality ground truth data.

Presentation
Gallery
Annexe
Conferences
- Büttner, N., Harttgen, K., Stalder, S., Suel, E. & Volpi, M. (2023). Mapping informal settlements in sub-Saharan Africa: The scope of applying statistical deep learning to satellite imagery. VfS (Verein für Socialpolitik) Annual Conference 2023, 24 - 27 September 2023, Regensburg.
- Büttner, N., Stalder, S., Volpi, M., Suel, E. & Harttgen, K. (2024). Mapping changes in urban slums in sub-Saharan Africa from 2016 to 2022. CSAE (Center for the Study of African Economies) Conference 2024, 17 - 19 March 2024, Oxford.
Additional resources
Bibliography
- UN (2015). Transforming Our World: The 2030 Agenda for Sustainable Development. Resolution Adopted by the General Assembly on 25 September 2015, 42809, 1-13. https://doi.org/10.1891/9780826190123.ap02
- Gram-Hansen, B. J., Helber, P., Varatharajan, I., Azam, F., Coca-Castro, A., Kopackova, V., & Bilinski, P. (2019, January). Mapping informal settlements in developing countries using machine learning and low resolution multi-spectral data. In Proceedings of the 2019 AAAI/ACM Conference on AI, Ethics, and Society (pp. 361-368). https://doi.org/10.1145/3306618.3314253
- Helber, P., Gram-Hansen, B., Varatharajan, I., Azam, F., Coca-Castro, A., Kopackova, V., & Bilinski, P. (2018). Generating material maps to map informal settlements. arXiv preprint arXiv:1812.00786. https://doi.org/10.48550/arXiv.1812.00786
- Jean, N., Wang, S., Samar, A., Azzari, G., Lobell, D., & Ermon, S. (2019, July). Tile2vec: Unsupervised representation learning for spatially distributed data. In Proceedings of the AAAI Conference on Artificial Intelligence (Vol. 33, No. 01, pp. 3967-3974). https://doi.org/10.1609/aaai.v33i01.33013967
- Zbontar, J., Jing, L., Misra, I., LeCun, Y., & Deny, S. (2021, July). Barlow twins: Self-supervised learning via redundancy reduction. In International conference on machine learning (pp. 12310-12320). PMLR. https://doi.org/10.48550/arXiv.2103.03230
Publications
Related Pages
As part of DeepLNAfrica, we have also worked on a project in which we mapped urban structural change in London, utilizing 15 million unlabeled street-level images with our adaption of the self-supervised Barlow Twins method:
News
Latest news


First National Calls: 50 selected projects to start in 2025
First National Calls: 50 selected projects to start in 2025
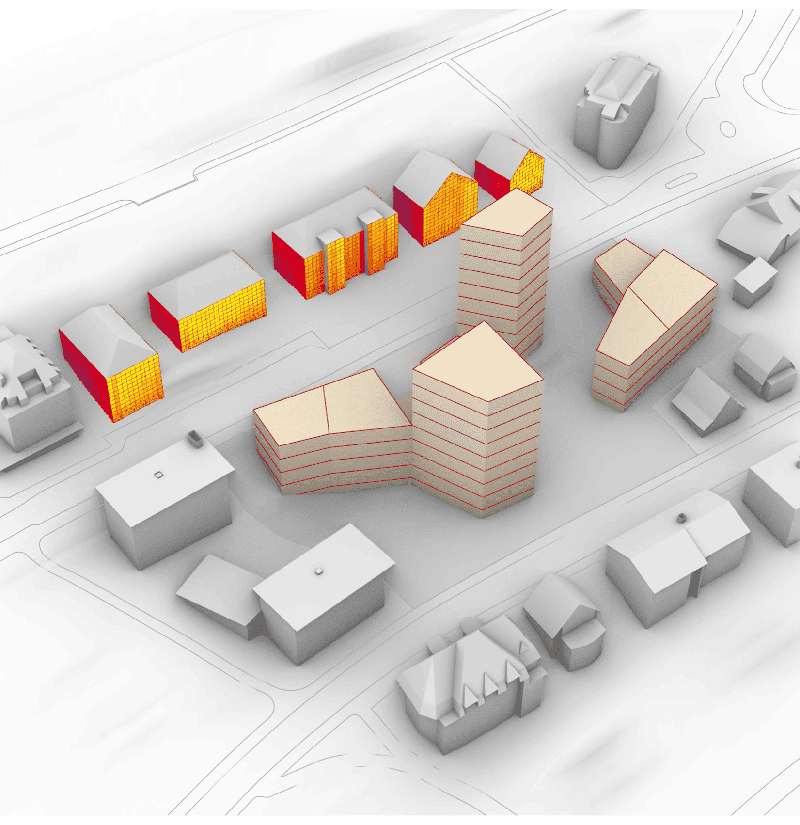
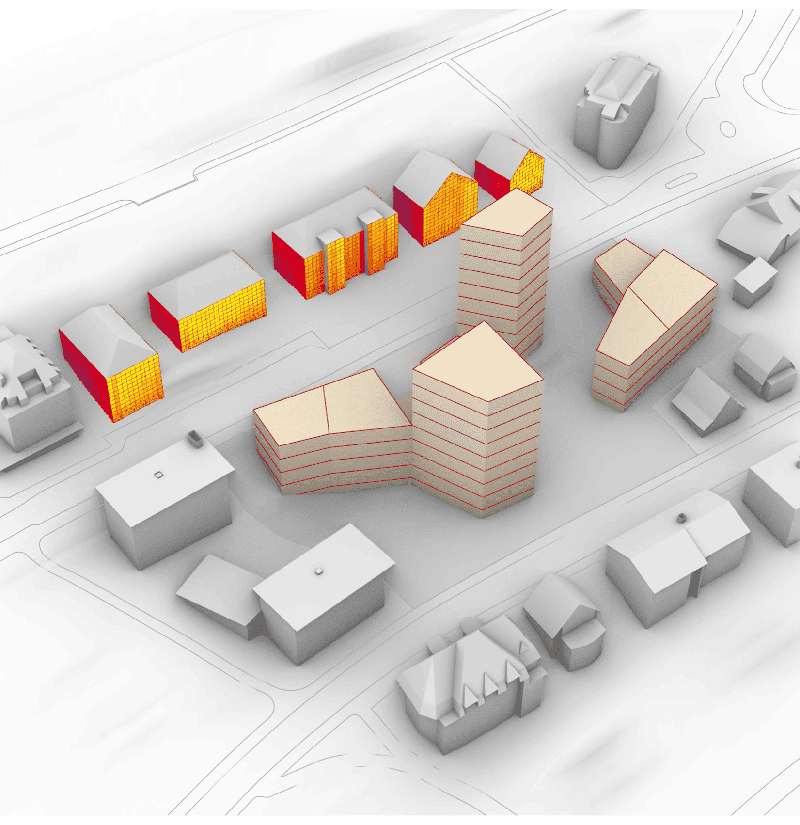
AIXD | Generative AI toolbox for architects and engineers
AIXD | Generative AI toolbox for architects and engineers


Smartair | An active learning algorithm for real-time acquisition and regression of flow field data
Smartair | An active learning algorithm for real-time acquisition and regression of flow field data
Contact us
Let’s talk Data Science
Do you need our services or expertise?
Contact us for your next Data Science project!