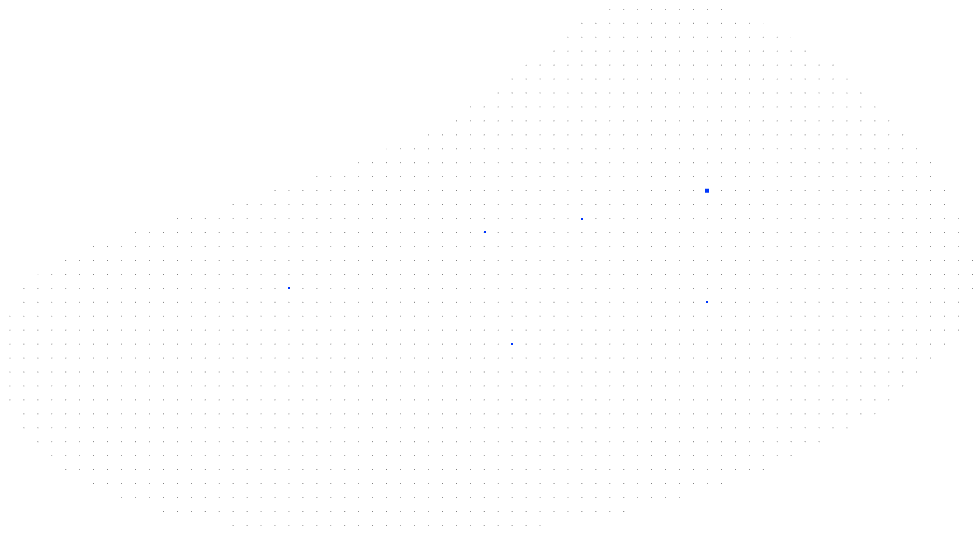
PHENO-MINE
Pheno-Mine: Extracting dynamic ideotypes from seasonal image time series of wheat taken in the field

Abstract
Adapting field crops to a changing climate requires a profound understanding of growth dynamics in relation to the environment. Recent advances in field phenotyping promised to facilitate the collection of essential data for such analyses. Indeed, in the last seven years, the field phenotyping platform (FIP) at ETH constantly collected image time series of more than 350 wheat genotypes. Analyzing these data involves a significant number of steps, most importantly the extraction of low-level features from images, the modeling of the dynamics of such low-level features, and relating these dynamics to target traits such as yield, protein content, earliness, and drought tolerance. The complexity of the involved steps prevents researcher from systematically “mining” image time series without previously defining a set of growth dynamics’ parameters to optimize. Consequently, one may not expect to find unforeseeable associations between growth dynamics and target traits. To overcome this limitation, this project aims to combine contemporary deep learning such as convolutional and recurrent neural network, together with generative image models. A neural network will be trained on image time series of genotypes with the task to predict their performance regarding a target trait, e.g., yield. The visualized response buildup in an a priori information constrained latent space will then be treated as new trait, a so-called “dynamic ideotype”, that represents a characteristic growth trajectory. Analyzing these ideotypes will enable researcher to identify favorable growth dynamics. Consequently, the newly gained insights will result in a better understanding of growth dynamics and responses to the environment. In the long term, such methods will hopefully allow plant physiologists and breeders to mine their datasets with less bias towards their initial hypothesis, allowing better mitigating future climate scenarios, and consequently contribute to ensure global food supply.
People
Collaborators


Xiaoran Chen joined SDSC as a senior data scientist in July 2022. Prior to this, she received her PhD at ETH Zurich in 2021. Her research was focused on unsupervised learning and anomaly detection on magnetic resonance imaging (MRI) scans. She also holds a master’s degree in bioinformatics and bachelor’s degree in biological science. Her research interest includes self-supervised learning, representation learning and general applications using machine learning methods.
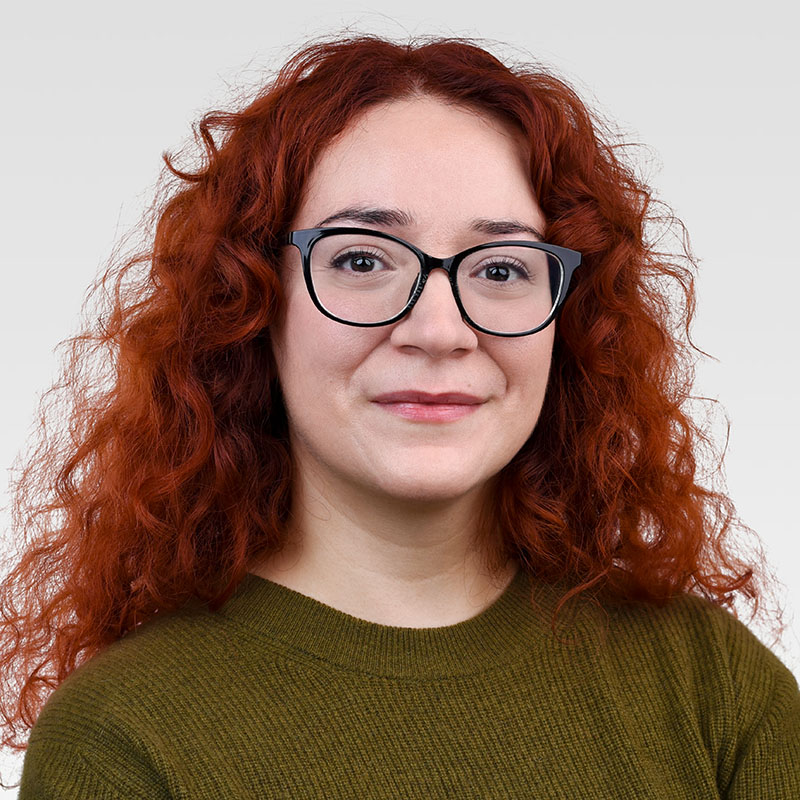
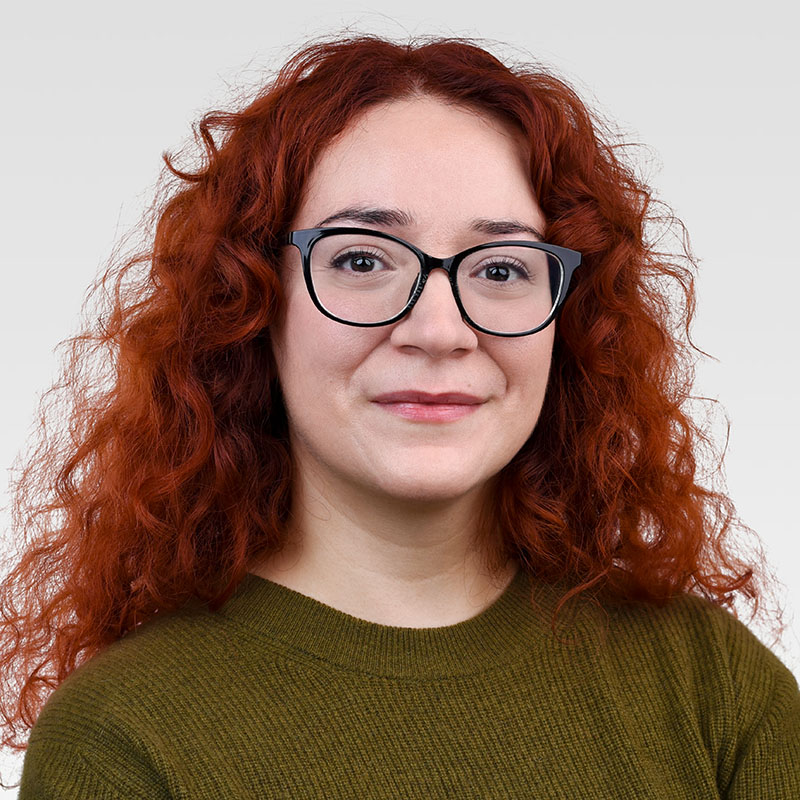
Paraskevi holds a Bachelor's degree and a PhD in Computer Science from Aristotle University of Thessaloniki, obtained in 2014 and 2021 respectively. Her thesis was focused on supervised and unsupervised Deep Learning methodologies with applications in computer and robotic vision as well as timeseries analysis. Her research interests include deep learning for computer vision, robotics, timeseries forecasting and gravitational waves analysis.
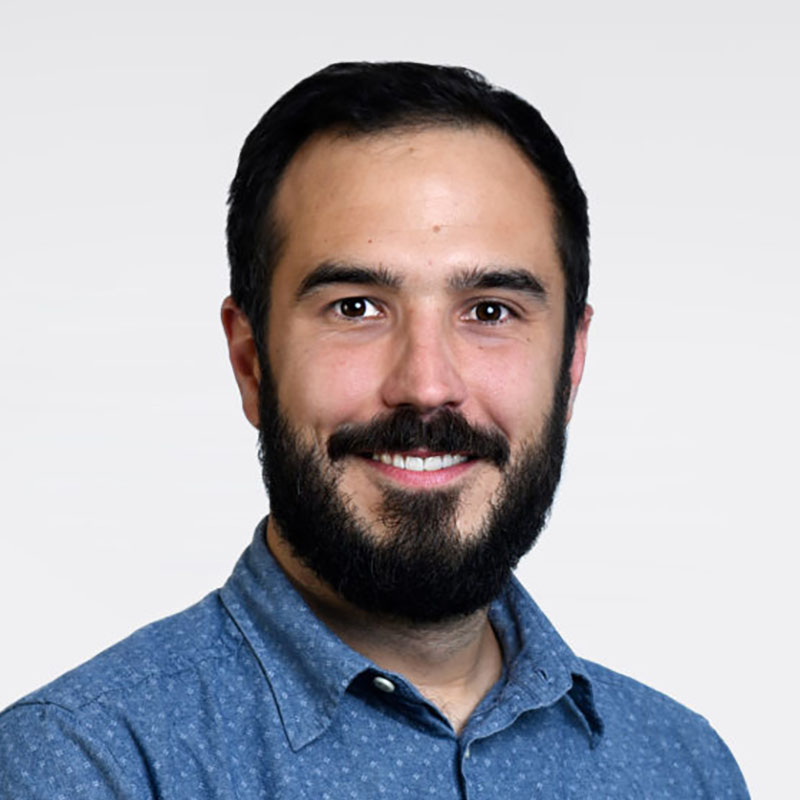
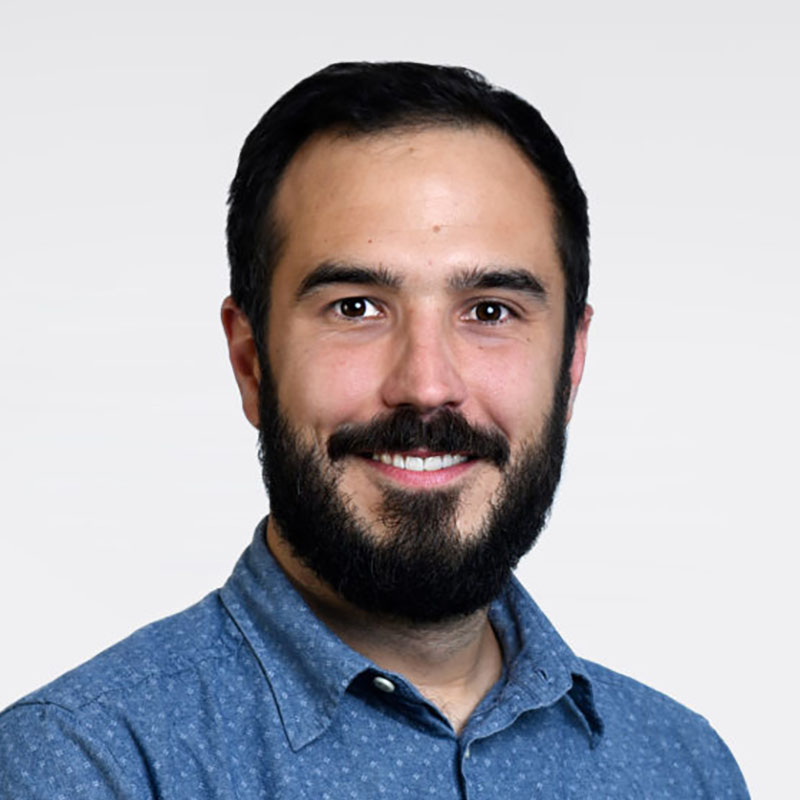
Michele received a Ph.D. in Environmental Sciences from the University of Lausanne (Switzerland) in 2013. He was then a visiting postdoc in the CALVIN group, Institute of Perception, Action and Behaviour of the School of Informatics at the University of Edinburgh, Scotland (2014-2016). He then joined the Multimodal Remote Sensing and the Geocomputation groups at the Geography department of the University of Zurich, Switzerland (2016-2017). His main research activities were at the interface of computer vision, machine and deep learning for the extraction of information from aerial photos, satellite optical images and geospatial data in general.
description
Motivation
The aim of the project is to learn latent phenotypes, or ideotypes, for crops given their temporal information of growth, weather data and measurements, to discover new traits and couple them with genotypes. Specifically, using both temporal imagery data and numeric measurements, a latent description can be learned to embed both information and also indicate genetic similarity, while traits of the crops can be disentangled to ensure interpretability.

Proposed Approach / Solution
Within the scope of the project, the problem is approached by encoding image and height sequences into a common vector representation before mapping the latter to the plant traits. Separate models for images and height sequences have been investigated before fusing the two into a single approach. Furthermore, genomic selection approaches, which have been studied in recent literature, are investigated within the purposes of the project, i.e., using genotype marker data in combination with environmental data to predict plant traits.
One major challenge in this task is to accurately learn to predict the traits of different wheat plants in unseen environments (e.g., different years) and of unseen genotypes in unseen environments, which relies very heavily on the phenotypic behavior captured by the input data. The discrepancy between the training and test data is highlighted in Figure 2, where FIP.2019 is used as the test set and split into two subsets: unseen environments and unseen genotypes in unseen environments. Despite learning the training set well, this baseline model does not generalize well to the test subsets; although it performs marginally better in the 'unseen environments' subset.
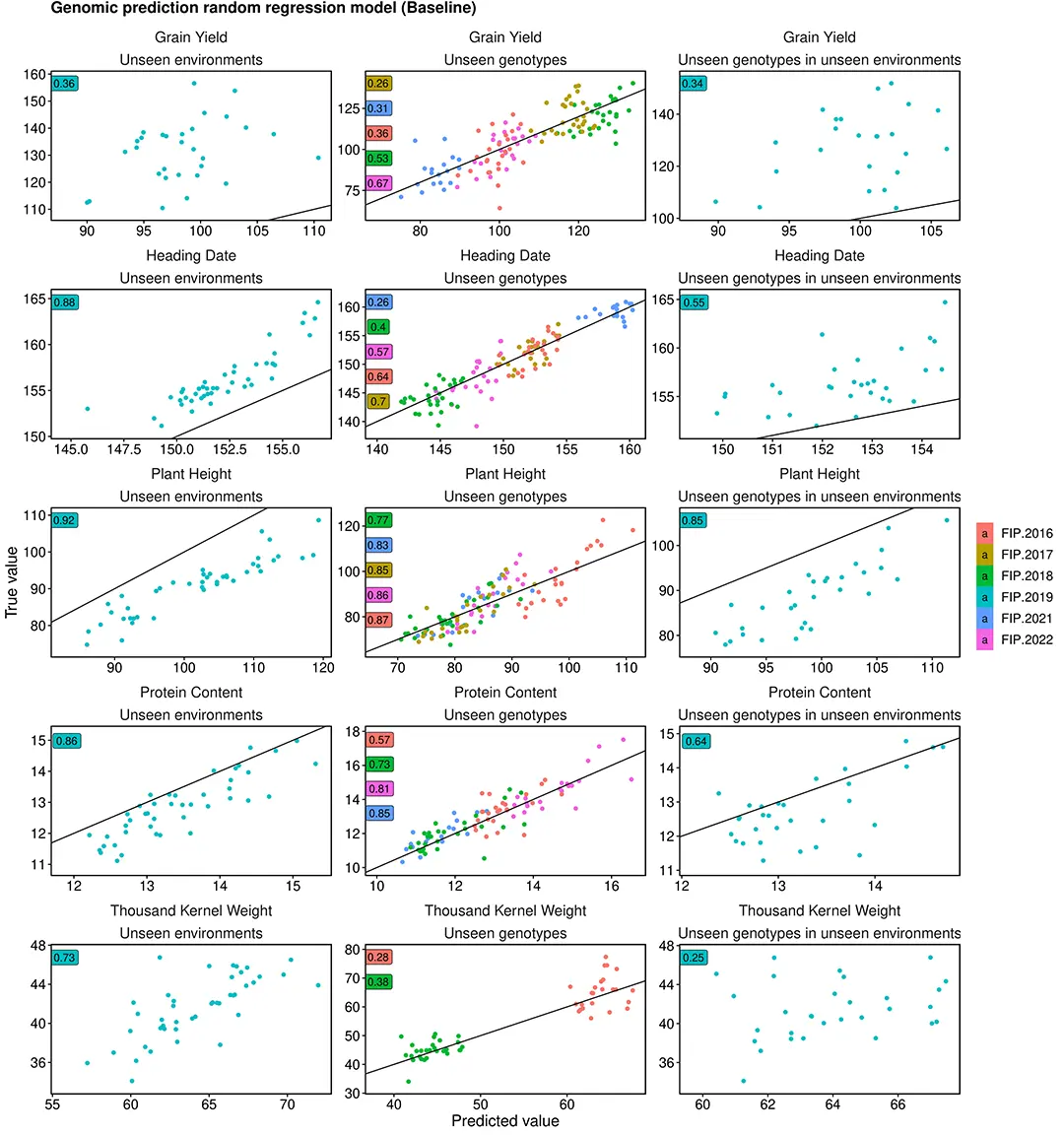
Impact
Growth of plants is a complicated and dynamic process that is difficult to measure and quantify manually. The field phenotyping platform (FIP) at ETH Zurich captures image time series at high image and temporal resolution and aims to analyze the data and extract ideotypes and help breeders better adapt their plants to future foreseeable environment conditions.
Presentation
Gallery
Annexe
Additional resources
Bibliography
- Ubbens, Jordan, et al. "Latent space phenotyping: automatic image-based phenotyping for treatment studies." Plant Phenomics 2020 (2020).
- Dosovitskiy, Alexey, et al. "An image is worth 16x16 words: Transformers for image recognition at scale." arXiv preprint arXiv:2010.11929 (2020).
- Caron, Mathilde, et al. "Emerging properties in self-supervised vision transformers." Proceedings of the IEEE/CVF international conference on computer vision. 2021.
- Kim, Wonjae, Bokyung Son, and Ildoo Kim. "Vilt: Vision-and-language transformer without convolution or region supervision." International conference on machine learning. PMLR, 2021.
Publications
Related Pages
More projects
ML-L3DNDT
BioDetect
News
Latest news


Smartair | An active learning algorithm for real-time acquisition and regression of flow field data
Smartair | An active learning algorithm for real-time acquisition and regression of flow field data
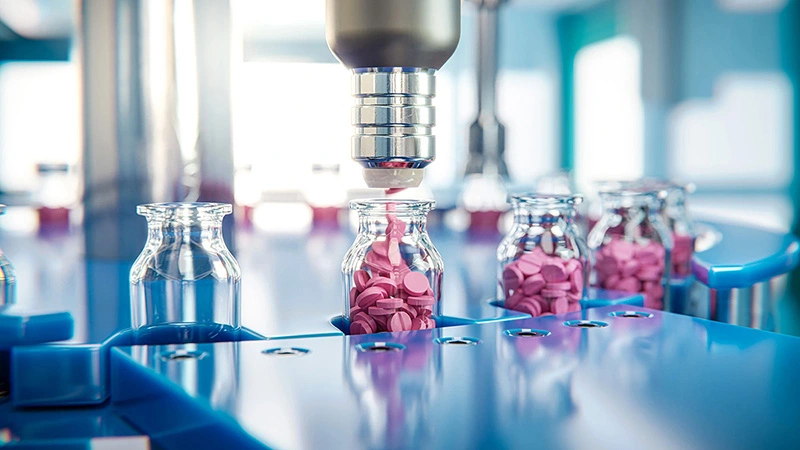
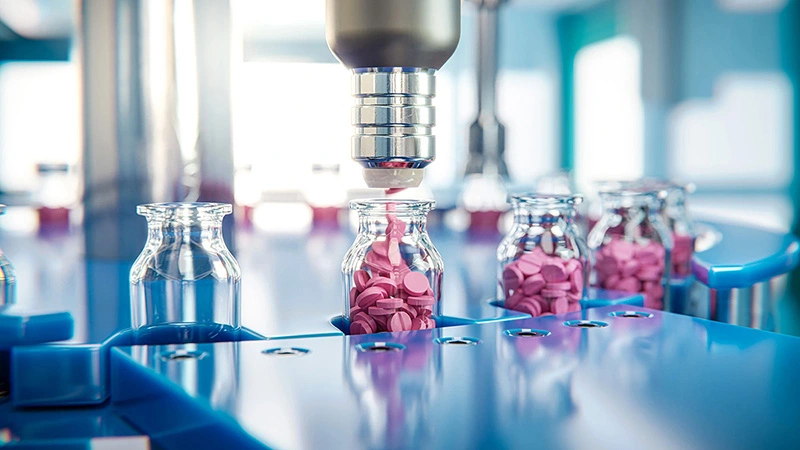
The Promise of AI in Pharmaceutical Manufacturing
The Promise of AI in Pharmaceutical Manufacturing


Efficient and scalable graph generation through iterative local expansion
Efficient and scalable graph generation through iterative local expansion
Contact us
Let’s talk Data Science
Do you need our services or expertise?
Contact us for your next Data Science project!