Introduction
Artificial Intelligence (AI) is not an alien word anymore nowadays. We see both academic and industrial institutions adopting AI topics as a part of their curriculum and use cases to accelerate existing processes. The pharmaceutical industry is one of them.
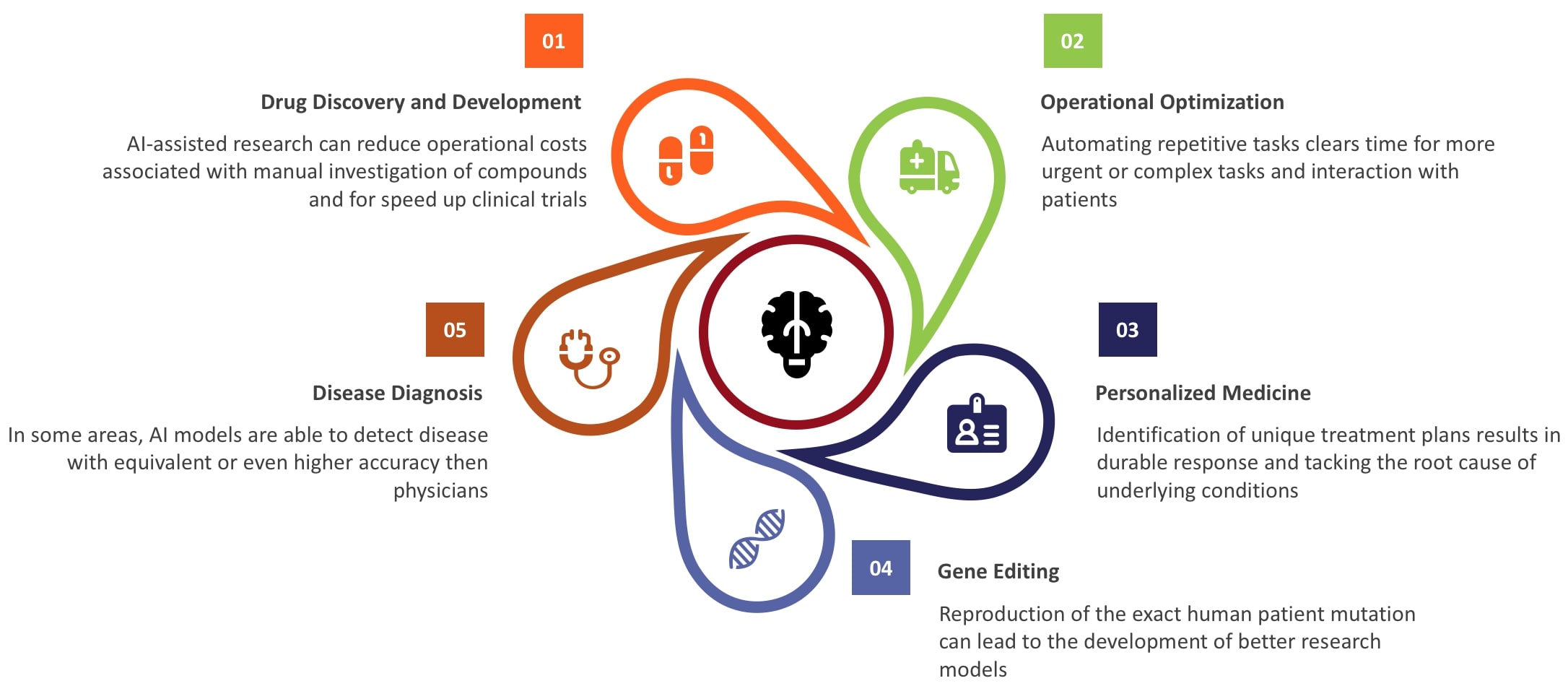
Having reviewed recent articles and corporate communications*, we identified 5 main areas in the pharmaceutical sector with an AI footprint (Figure 1). These included 60 use cases and 36 companies or institutions expanding their activities in different pharmaceutical areas. Each area has a starred case: (1) compound screening is a predominant use case in drug discovery area, (2) customer service “bots” have the most potential in operational optimization, (3) personalized medicine aims to design treatments by cross-referencing patients, treatments and outcomes, (4) insertions and deletions predictions are key in gene editing and (5) differentiation between healthy and disease conditions is the main trend in disease diagnosis area.
“… 60 use cases and 36 companies or institutions expanding their activities in different pharmaceutical areas.”
Not withstanding the challenge of data collection, cleaning and integration, Data Science still enabled a variety of use cases in the pharmaceutical domain [1]. In a nutshell, some processes are completely automated with AI, while a few would highly benefit from data-driven insights. Let’s look closer into these cases!
*Our review was limited to publications and corporate communications in 2019 and later, hence this is a non-exhaustive list of industry developments. If you would like to add a use case or company relevant to AI applications in the pharmaceutical domain, please do not hesitate to contact us at contact@datascience.ch
Drug discovery & development
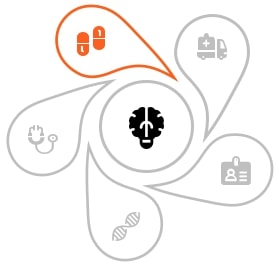
Computational and algorithmic advancements accelerated the domain of drug discovery and development. This resulted in a reduction of animal testing and time required for drug approval and market reach, consequently speeding up clinical trials. Another positive outcome is biomarker discovery with data science tools that can ensure their sensitivity, robustness and validate results with in-silico models.
“… biomarker discovery with data science tools that can ensure their sensitivity, robustness and validate results…”

Operational optimization
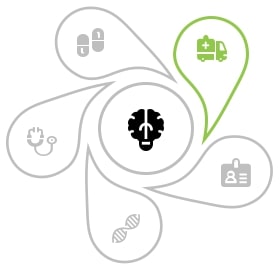
Smart automation of processes clears up time to focus on more urgent or complex tasks (see the visual below). A clear example is automating patients’ reminders and follow-ups. This remote monitoring increases chances for timely interventions in a treatment plan when drugs lack efficiency and addresses drug adherence issues. Another example of operational optimization is reading, cleaning and analysis of structured and unstructured data such as patients’ medical records, doctor notes, prescriptions and detected side effects, etc. A continuous medical follow up history generates a multitude of personalized data that, if harvested effectively, can bring us closer to the notion of the Big Data and greatly advance medical research.
“A continuous medical follow up history generates a multitude of personalized data…”
In brief, there are multiple benefits to invest into operations optimization that in turn can reduce unnecessary hospital visits, and thus lessen the burden on medical professionals and save the healthcare system money.
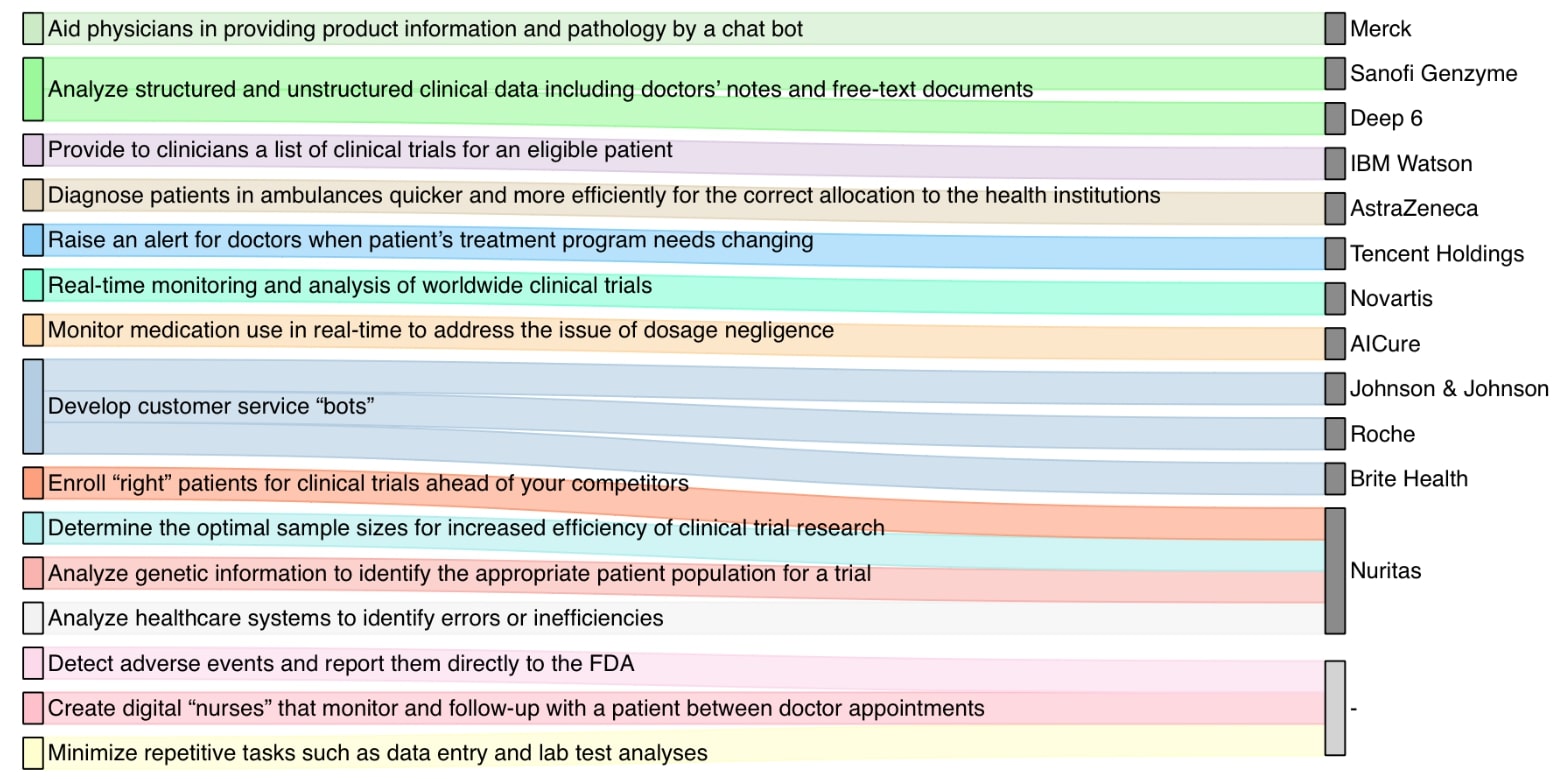
Personalized medicine
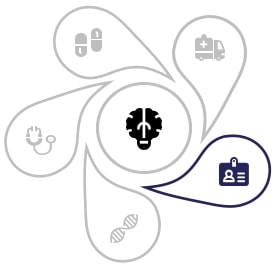
One of the greatest advantages of AI over a human is ability to process and integrate a vast amount of information from the multiple sources. This is a key requirement for personalized medicine, where multiple levels of information at an individual and global scale should be mined to provide effective individual-level treatments. An extent of this idea would be a continuous analysis of the treatment progress that can allow timely adjustment of the drug dosing, hence increasing the efficiency and safety of the treatment itself.
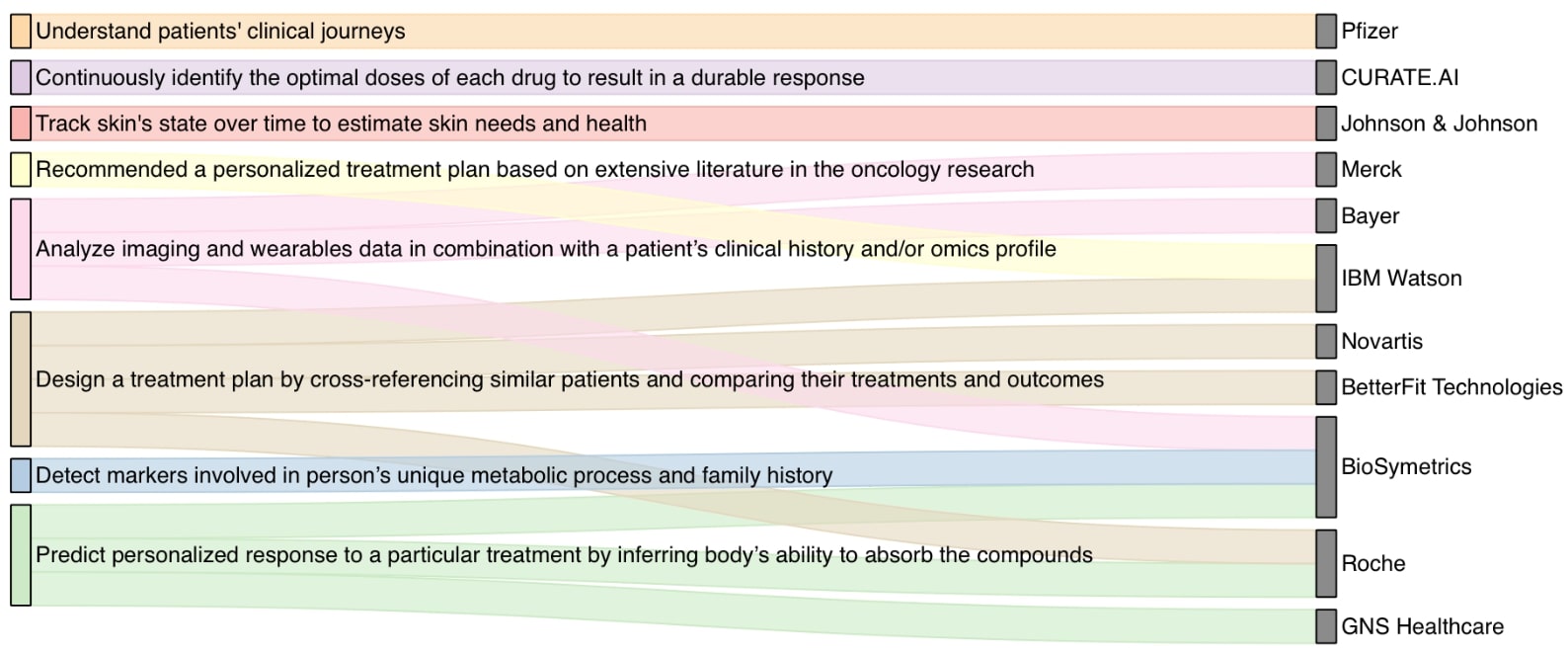
Gene editing
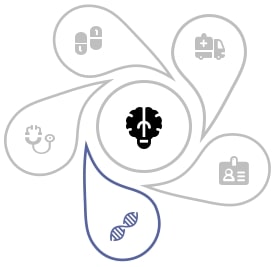
“AI can make gene editing initiatives more accurate, safer and cheaper.”
While genome editing is far from being translated into therapies, researchers should use AI to minimize potential damaging off-target effects. Primarily, research institutions such as Broad Institute, MIT and Wellcome Sanger Institute investigate advancements in gene editing technology. There are a few developments in this area, where AI can make gene editing initiatives more accurate, safer and cheaper (see the visual below).
Genome editing – a method that lets scientists change the DNA of many organisms, including plants, bacteria, and animals. Editing DNA can lead to changes in physical traits, like eye color, and disease risk. (Ref: National Human Genome Research Institute)

Disease Diagnosis
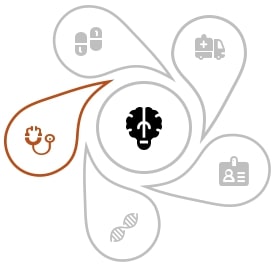
“Unsupervised learning could change the way diseases are classified, as current disease ontologies were created in a top-down fashion based on the observed symptoms.”
Limitations of current medicine – such as incomplete patients’ history records, limited root cause analysis or overlapping causal symptoms – can be compensated with supervised and unsupervised machine learning approaches. Unsupervised learning could change the way diseases are classified, as current disease ontologies were created in a top-down fashion based on the observed symptoms. The basis to challenge this concept is the fact that molecular profiles of the diseases might share common pathways that result in entirely different symptoms. Hence, harvesting the ability of AI to analyze multiple data sources and types (imaging, omics, clinical records and biological event) overcomes the limitations of the manual approach.
Omics – in cellular and molecular biology, omics is used to form nouns with the sense “all constituents considered collectively”. (Ref: Oxford English Dictionary)
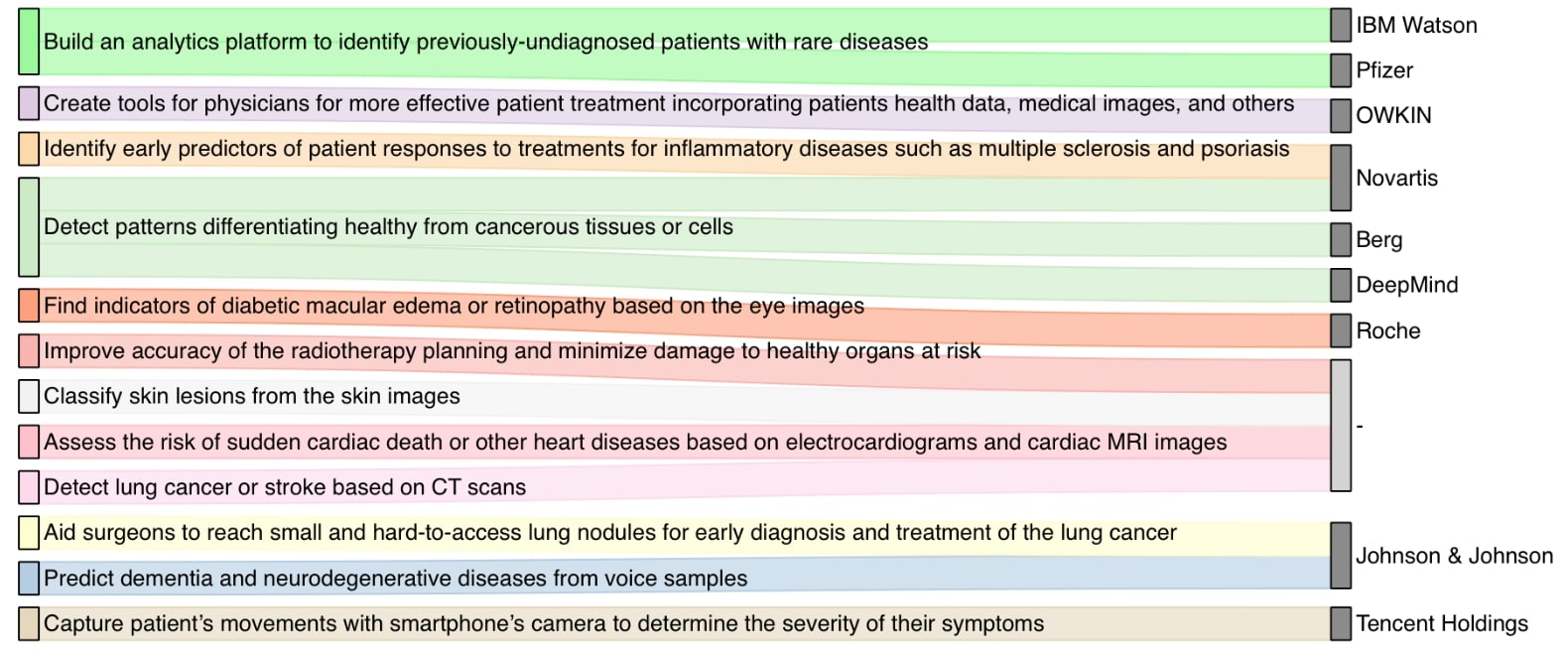
Conclusion
“The most common use case (based on the number of involved companies) is compound screening for their affinity and toxicity.”
In this article, we aimed to summarize the main pharmaceutical areas and showcase relevant use cases that take place in different companies (Figure 2). The most common use case (based on the number of involved companies) is compound screening for their affinity and toxicity. A high effort is also put into drug repurposing as well as structural and molecular analysis. Two companies – Nuritas and Novartis – have been highly attributed with different AI use cases. However, this does not mean that other pharmaceutical players have fewer advancements in Data Science but rather that projects could be confidential or could have a limited media coverage. In any case do not hesitate to reach out to us to add use cases that your company is pursuing!
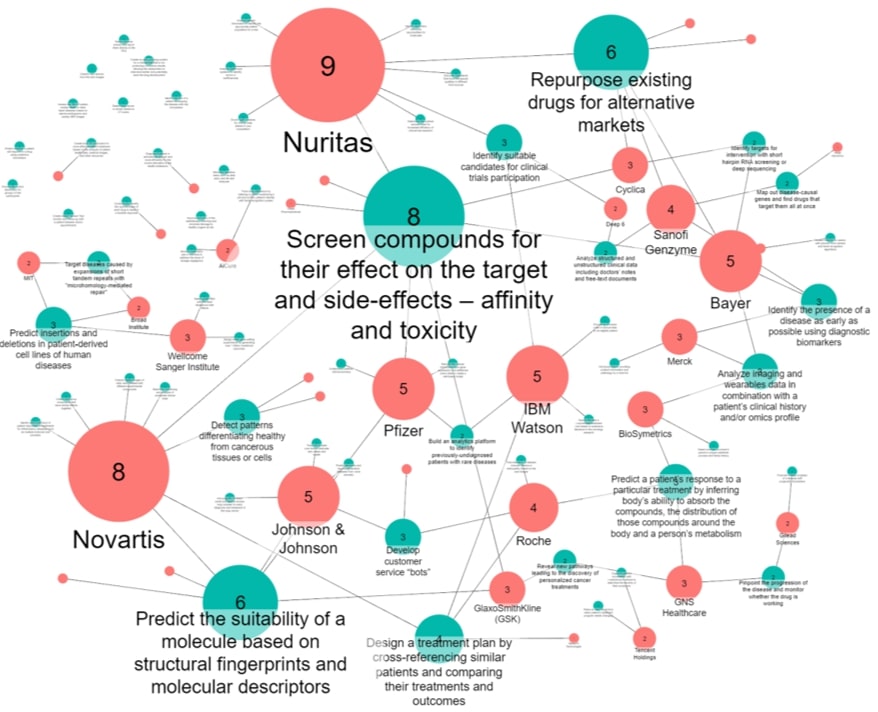
Disclaimer: The network depiction of the use cases and participating companies was limited to publications and corporate communications in 2019 and later, hence this is a non-exhaustive list of industry developments. If you would like to add a use case or company relevant to AI applications in the pharmaceutical domain, please do not hesitate to contact at contact@datascience.ch
References
- Shaywitz D. (2019) “Novartis CEO Who Wanted To Bring Tech Into Pharma Now Explains Why It’s So Hard”.
- Data Revenue (2019) “Artificial Intelligence in Medicine”.
- de Jesus A. (2019) “Artificial Intelligence in the Pharmaceutical Industry – An Overview of Innovations”.
- Yeadon N. (2019) “Artificial Intelligence in Pharma”.
- Conroy D., Conroy M. (2019) “AI in Pharmaceuticals”.
- Proffitt C. (2017) “Top 10 Artificial Intelligence Companies Disrupting the Pharmaceutical Industry”.
- Arsene C. (2019) “Artificial Intelligence & Pharma: What’s Next?”.
- PharmaTutor (2019) “TOP 10 Pharmaceutical industries using Artificial Intelligence (AI)”.
- Sennaar K. (2019) “AI in Pharma and Biomedicine – Analysis of the Top 5 Global Drug Companies”.
- Liu A. (2018) “Making CRISPR-Cas9 gene editing safer with artificial intelligence”.