The beamline for TOmographic Microscopy and Coherent rAdiology experimentTs (TOMCAT) at the Paul Scherrer Institut (PSI) allows to do tomographic microscopy (SRXTM) using the X-rays from a synchrotron. This makes it possible to capture the three-dimensional structure of animal tissue from organs like, but not limited to, heart, brain, lungs, and bones at a high speed and high resolution in a non-destructive manner.
Such three-dimensional imaging can help clinicians, pathologists, and biomedical researchers in obtaining a deeper understanding of tissue and its functioning without going through the cumbersome conventional process of tissue fixing, drying, staining, paraffin blocking, and slicing, which in the end only provides two-dimensional images.
PSI, ETH Zurich, and the Swiss Data Science Center (SDSC) are jointly working on automatically analysing such three-dimensional images to ease the burden of the clinicians and pathologists in identifying healthy tissue from sick ones. But in addition, the three-dimensional imaging and its automatic analysis reveals new insights into such functioning of organs and their constitution. Together, both the accelerated analysis of tissue as well as a better understanding of the organs is going to benefit patients suffering from maladies of vital organs.
The current focus is on heart tissue, in particular that of rodents. The goal is to assess the health of the tissue by estimating the amount and nature of collagen fibers. This calls for a pixel-wise segmentation of the three-dimensional image volumes. Doing this segmentation manually is tedious and error-prone. Automatic methods are therefore of paramount importance.
Two approaches were developed to automatize the analysis of image volumes. One of them is a signal processing approach while the other is a deep learning approach. There are advantages and disadvantages to both approaches. A signal processing approach can be more general, applicable to many similar problem settings. It is also be computationally more efficient. In addition, it can avoid the need for training data, which is often difficult to obtain because it requires tedius manual labeling over thousands of images. On the other hand, the machine learning approach shows higher accuracy than the signal processing approach as long as there are sufficient groundtruth labels (obtained manually) to train the deep network.
While the currently developed solutions can be used for a limited set of tasks, in order to be used by clinicians and pathologists, the goal for the next one year are to combine the advantages of the two approaches, namely, require less training data, use fewer manual labels, and possess the capacity to learn from noisy labels. This will lead to drastically reduced times for tissue health analysis and will pave the way for a better understanding of human organs and their functioning.
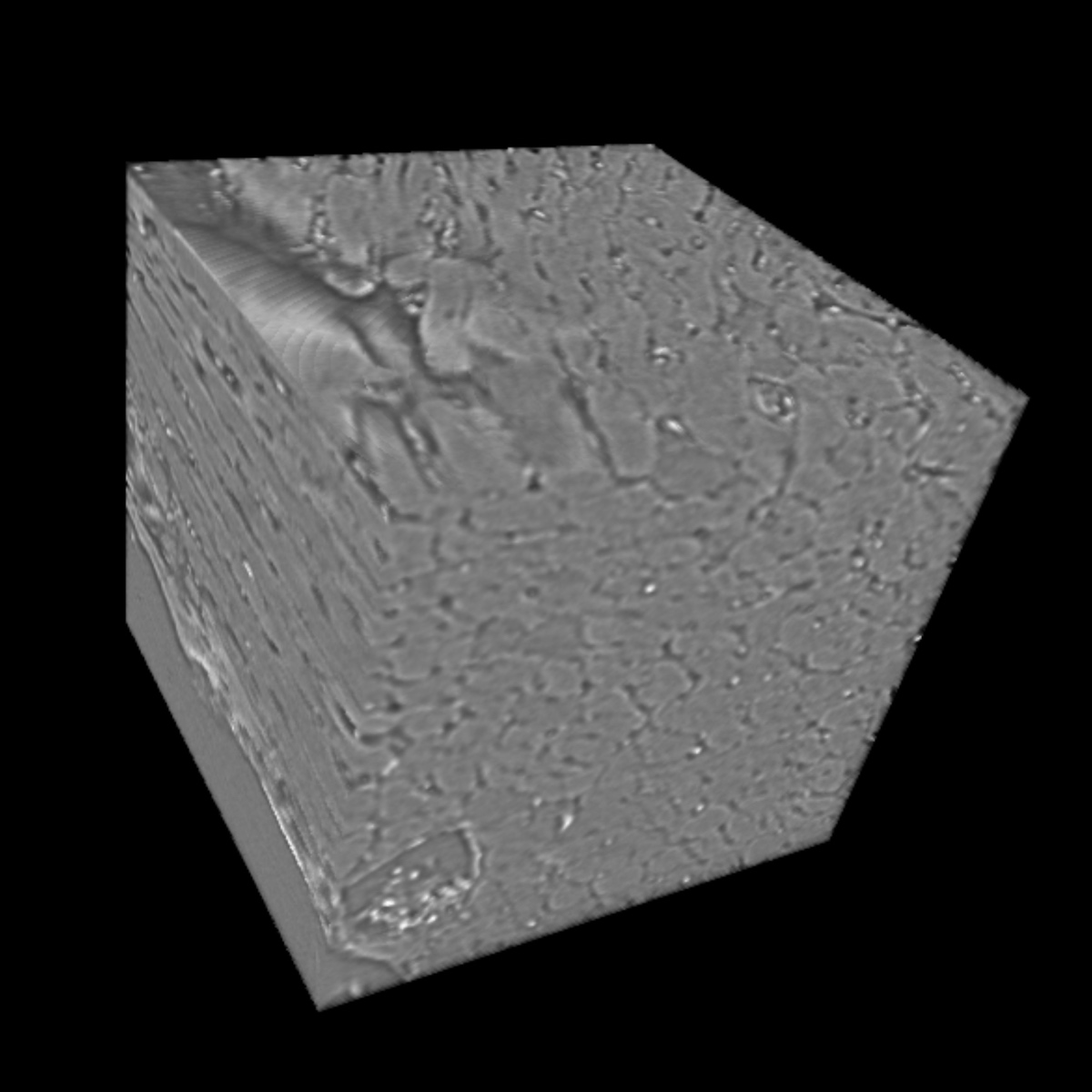
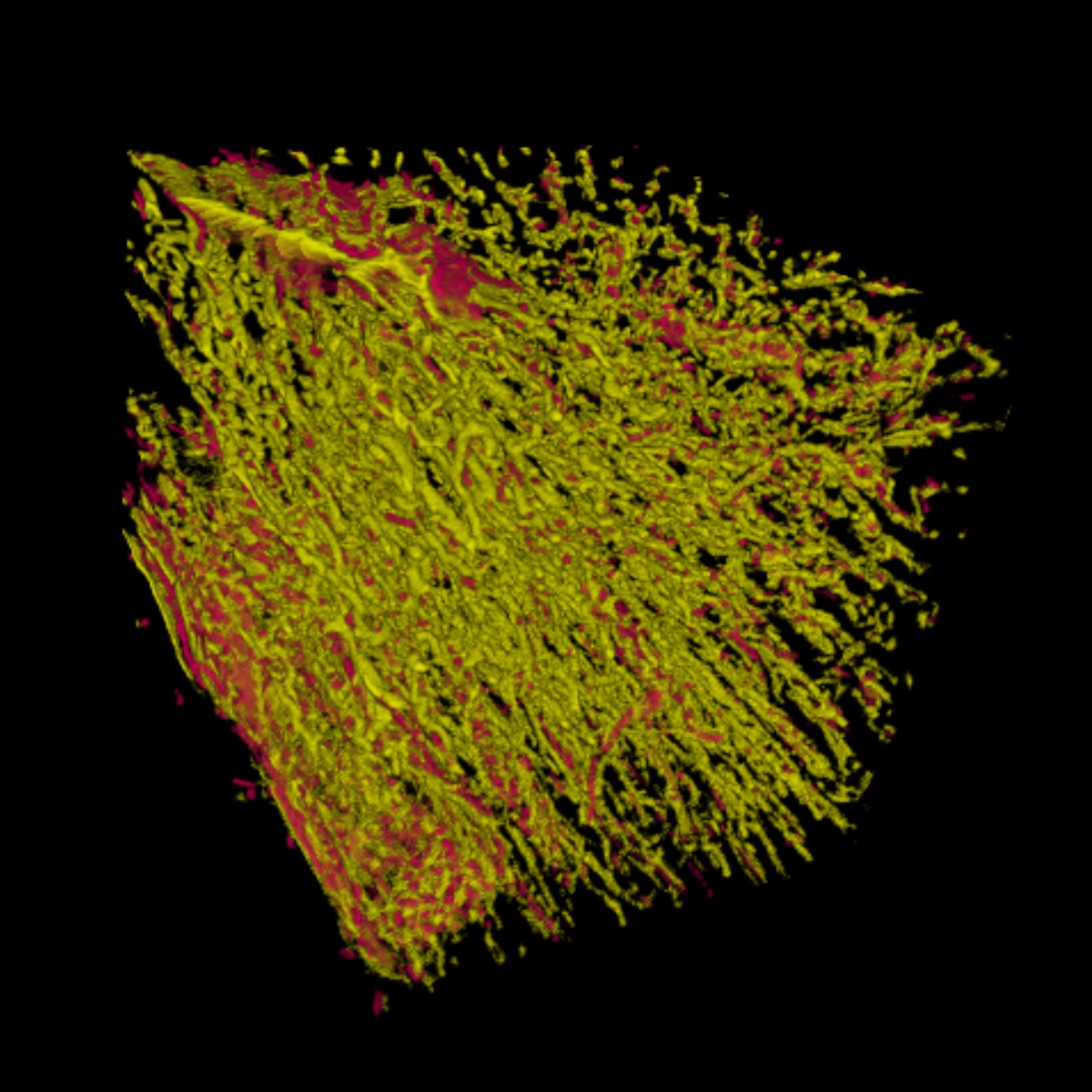