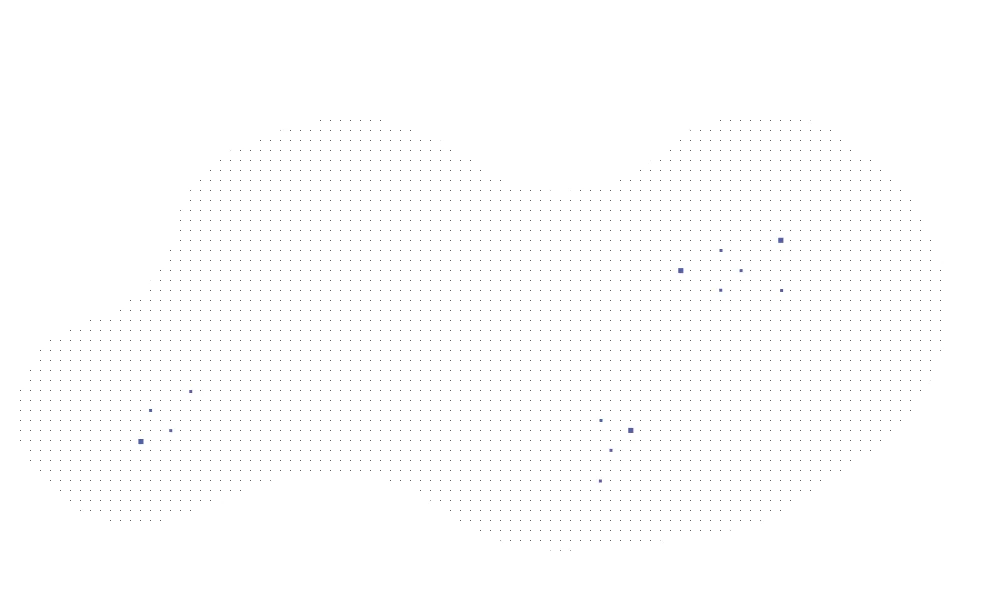
Job title standardization through entity alignment of knowledge graphs
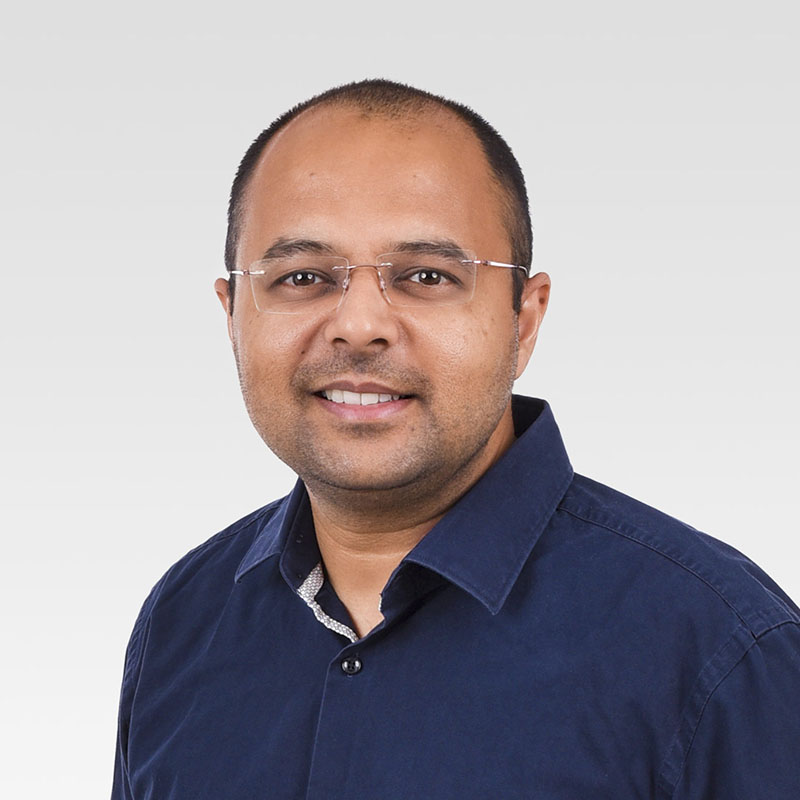
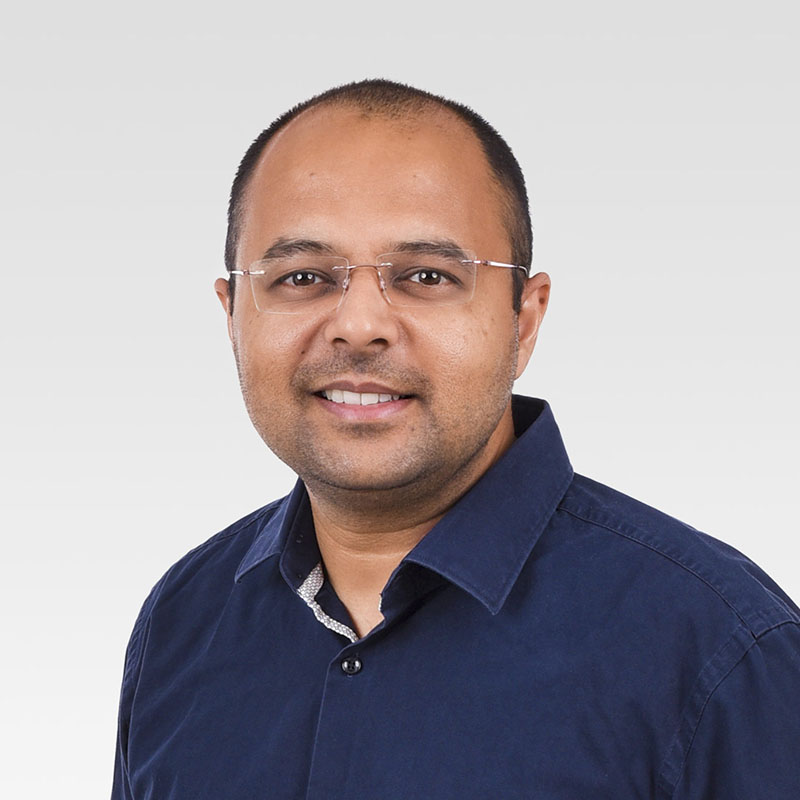
Saurabh Bhargava, joined the SDSC as a Principal Data Scientist in the Industry Cell at the Zürich office in 2022. Saurabh previously worked in the retail sector and the advertising industry in Germany. He lead and built various data products for customers using state of the art machine learning methods and industrializing them thereby adding value for the customers. He completed his PhD from ETH Zürich in June 2017 specializing in machine learning applications on Audio data. He obtained his Master’s and Bachelor’s degrees from EPFL and Indian Institute of Technology (IIT), Roorkee, India in 2011 and 2009 respectively. His interests and expertise are in combining state of the art data science and data engineering tools for building scalable data products.
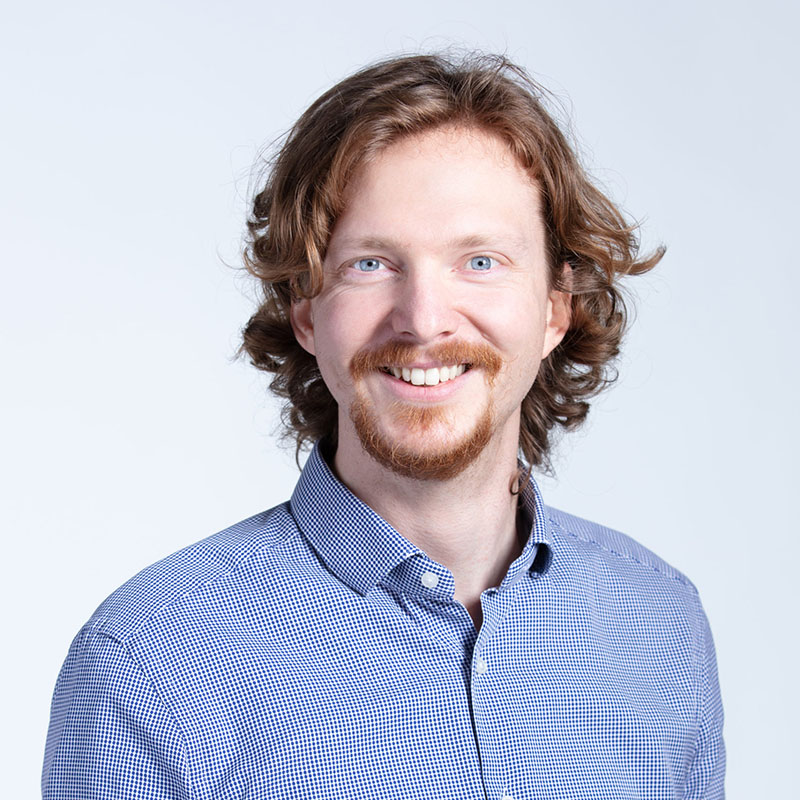
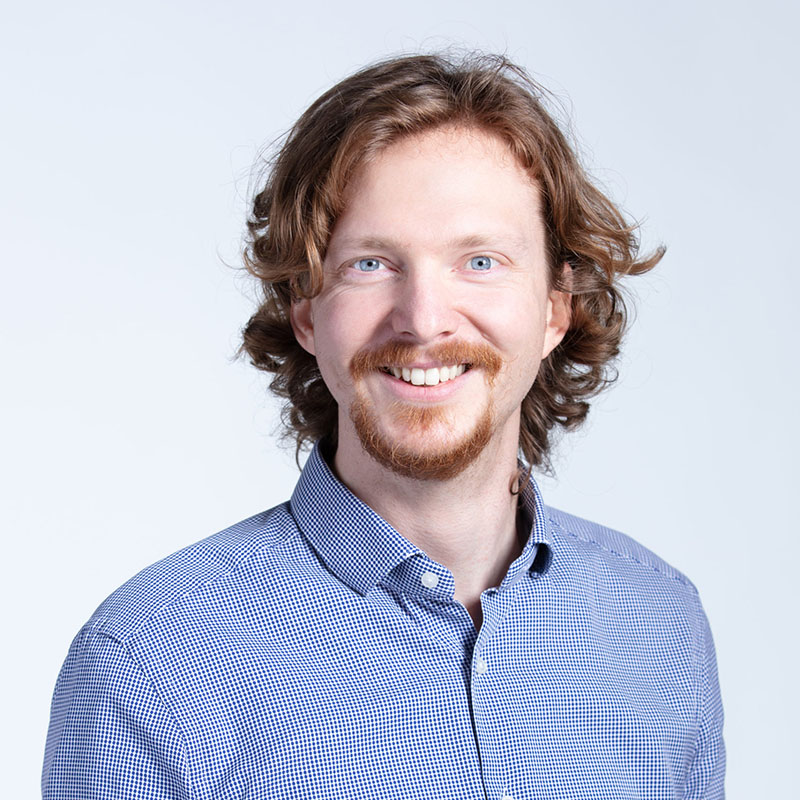
Lucas joined the SDSC's industry cell as a Data Scientist in November 2020, having previously worked in data related roles at the New York State Attorney and at Ericsson. He holds a BSc in Economics from Bocconi University, a MSc in Urban Science and Informatics from New York University as well as a MSc in Machine Learning from KTH Royal Institute of Technology. Over the course of his academic and professional career he has worked on a variety of topics, from computer vision tasks for automated driving to financial fraud detection to generating data driven insights to inform urban policy decisions.
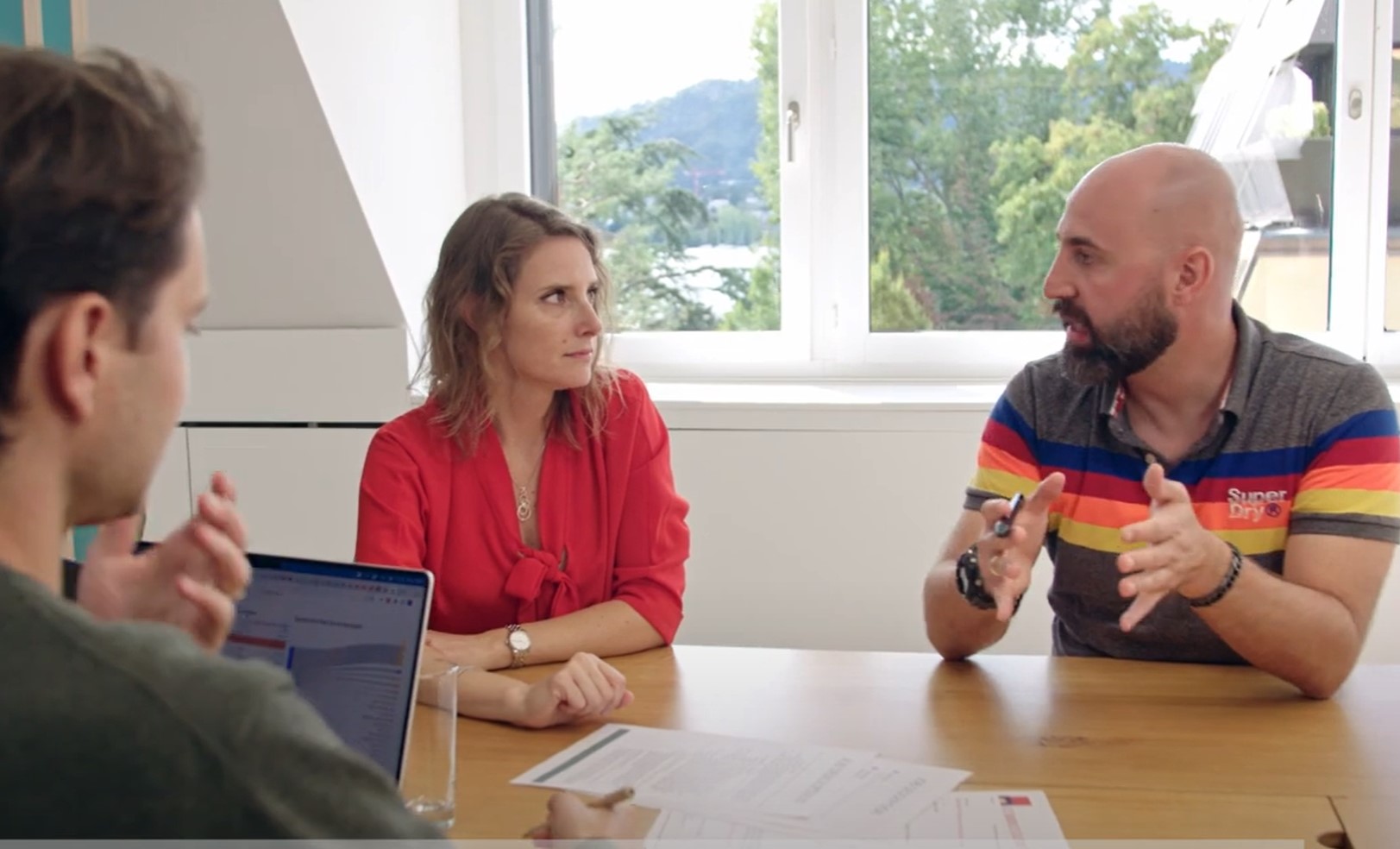
Context
The Adecco Group is one of the largest HR providers and staffing firms in the world. In order to find the best candidate for a given job vacancy, it is necessary to write precise job descriptions and to identify successful candidate profiles. Achieving this relies on curating unified and standardized job information. The focus of this project is on standardizing the terminology of job titles.
Objectives
Job information is scattered across various homogeneous sources, such as ESCO or O*NET, that differ in the use of terminology and data completeness. To optimally leverage information from these sources, they must be unified and standardized.
One approach to achieving this is by representing data sources as knowledge graphs (KG) and applying a technique named “entity alignment”, which identifies nodes in different KGs that refer to the same entity (i.e. concept). KGs generally contain different types of relationships (edges) and different types of entities (nodes). Crucially though, all constructed Knowledge Graphs have one type of entity in common, namely job titles.
Examples of relationships are those identifying alternative titles (e.g. Software Architect vs Application Architect), job categories (e.g. IT professionals) or skill requirements (e.g. Python). Considering node connectivity and embeddings of job titles and their descriptions obtained from fine-tuned Natural Language Processing models, a Deep Learning model was trained to identify nodes that refer to the same job title. This hybrid approach allows to incorporate both semantic and graph-based similarity of job titles.
Benefits
Aligned job titles as identified by the developed Deep Learning model are merged and represented by a single, standardized job title. Having a standardized terminology of job titles and their descriptions allows recruiters to describe job postings and assess candidate profiles more efficiently. This ensures faster and more accurate staffing, thereby raising labor productivity.
.jpg)
Notes
The SDSC would like to thanks the following people at Adecco Group: Pencho Yordanov, Riccardo Menoli, Sarah Mathews, Giovanna Favia, Helmi Boussetta, Marco Totolo.
Links
- The Adecco Group | Website
More case studies
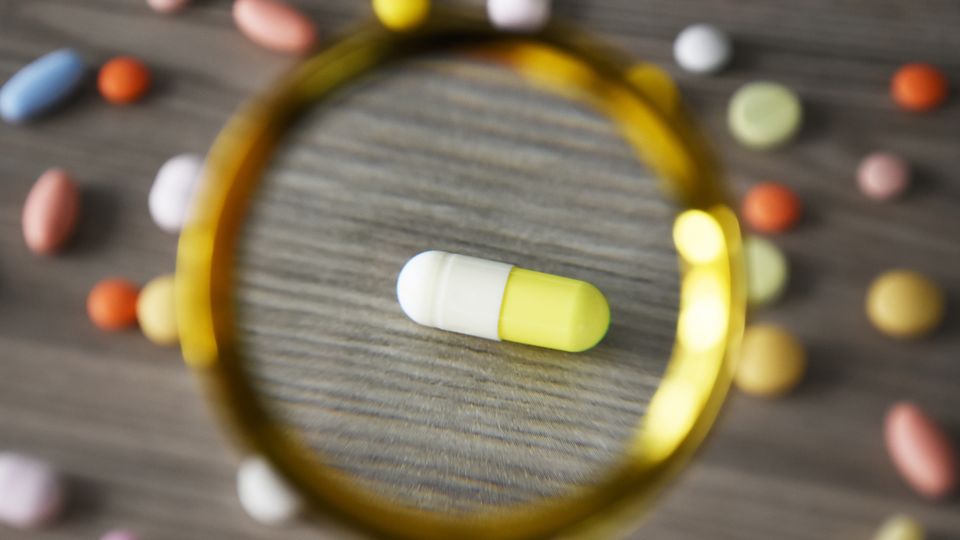
Repositioning drugs for a rare disease
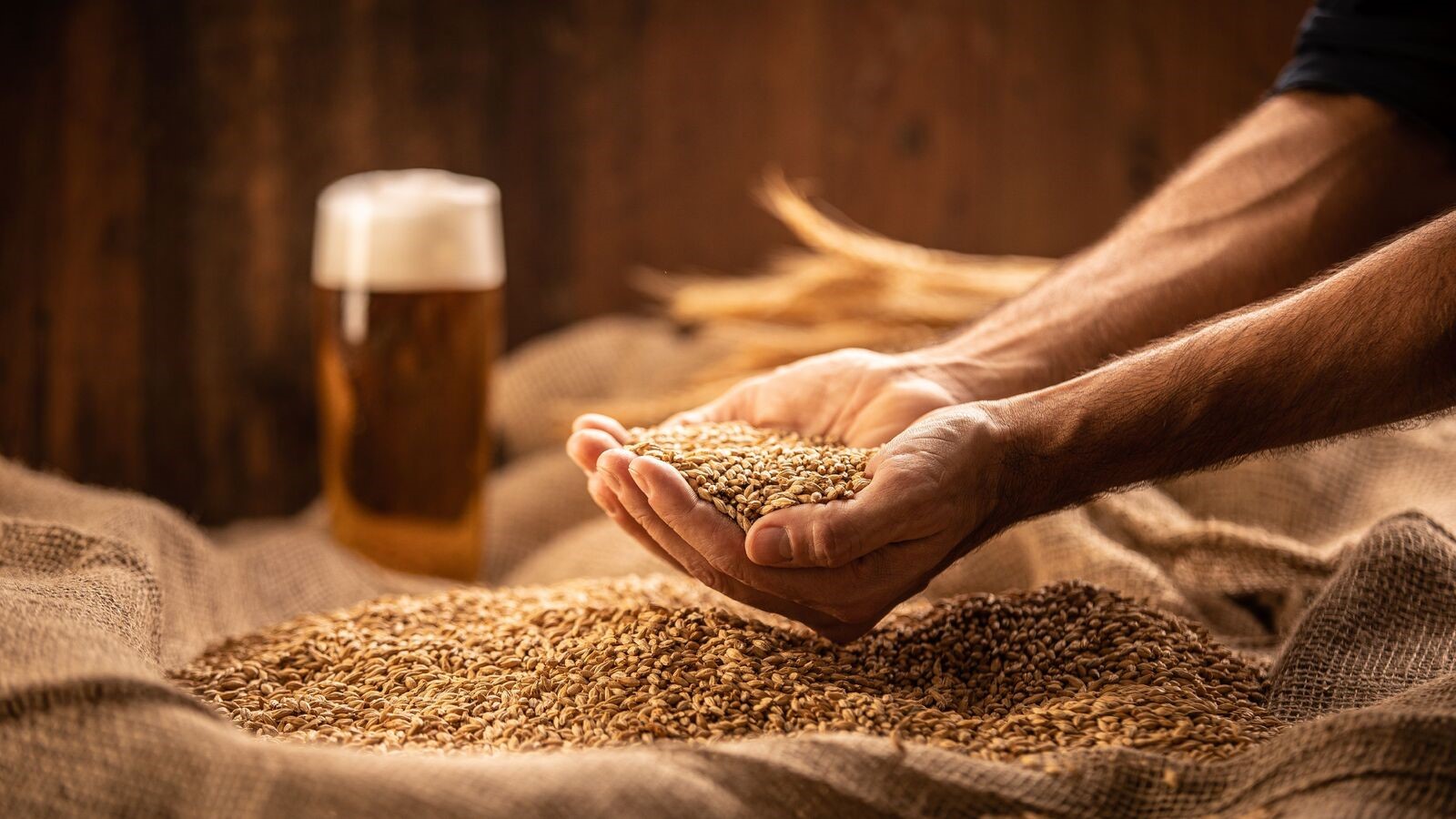
Data science for enhancing resource efficiency in manufacturing processes
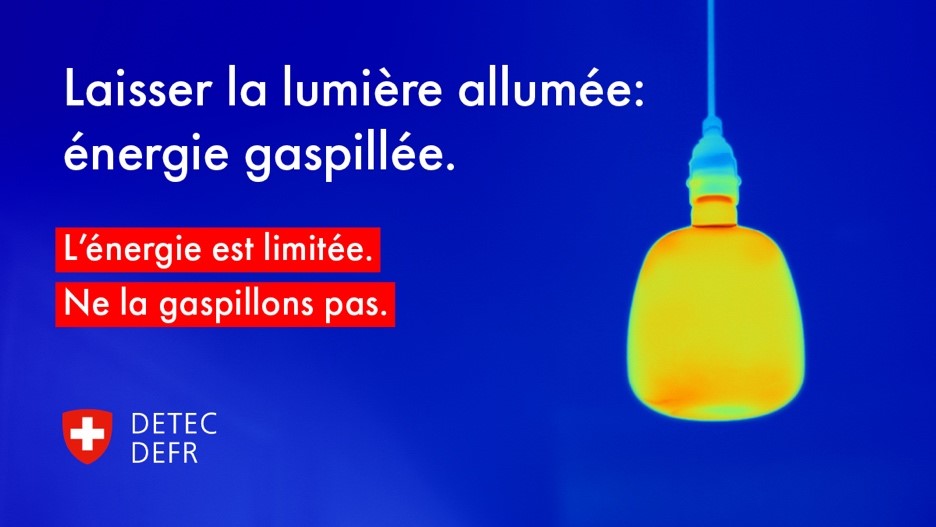
Modelling the end-user Swiss electricity consumption
Contact us
Let’s talk Data Science
Do you need our services or expertise?
Contact us for your next Data Science project!