
DLOC
Deep Learning for Observational Cosmology
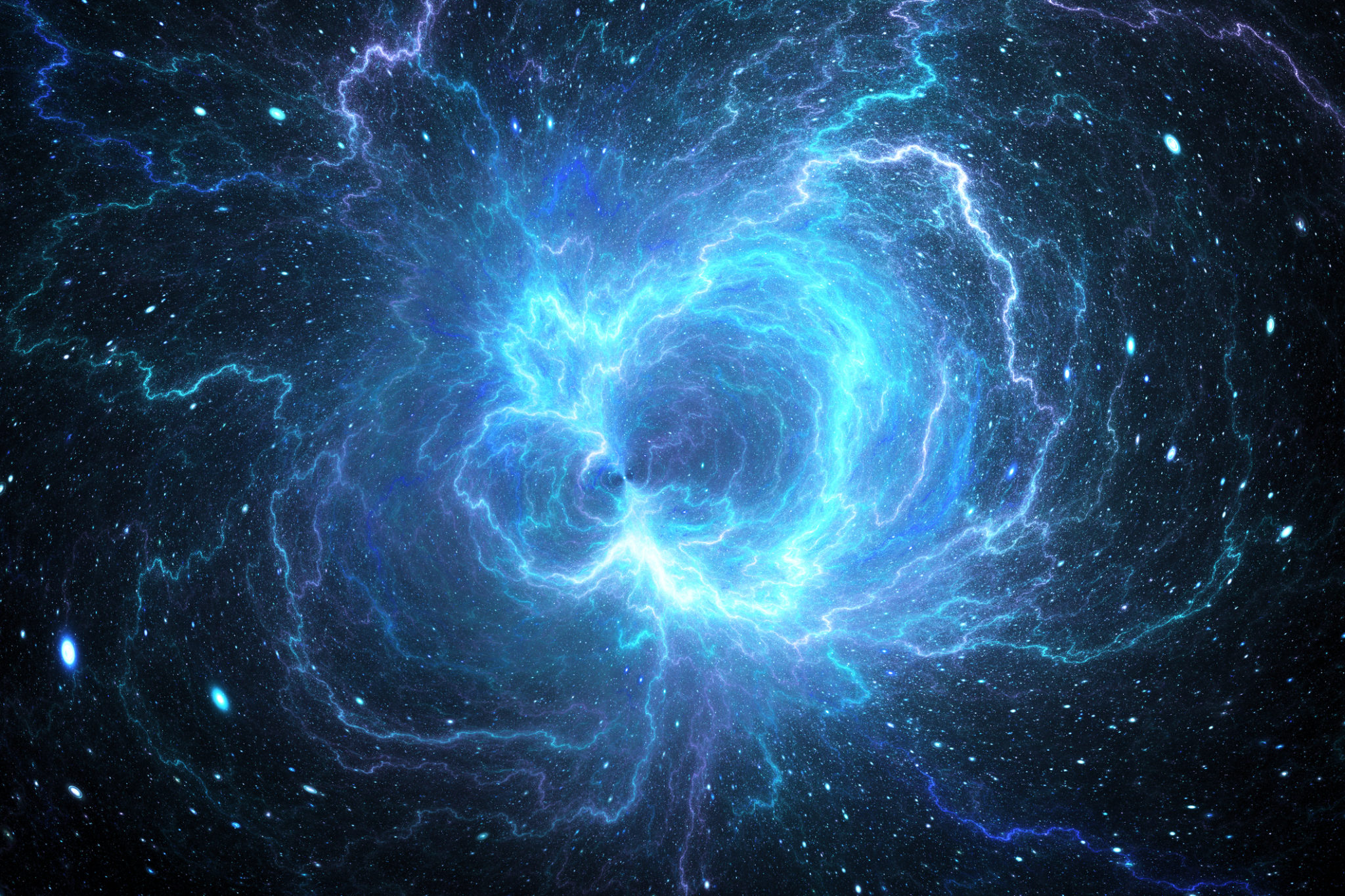
Abstract
We capitalise on the latest developments in the field of Deep Convolutional Neural Networks (CNN) and Generative Adversarial Networks (GAN) for solving some of the most important problems in the field of Observational Cosmology. The advantage of neural networks over classical statistical methods that are currently used in cosmology is that they are able to learn complex nonlinear relations present in the cosmological data. Applications of deep learning techniques have the potential to radically improve on existing data analysis methods in several areas of cosmology and astrophysics. In this proposed project, we plan to tackle three specific problems:
- Improving the precision of measurement of cosmological parameters from dark matter maps using CNNs.
- Generating cosmological N-body simulations using generative machine learning techniques.
- Creating deep learning algorithms to operate on the sphere.
People
Collaborators
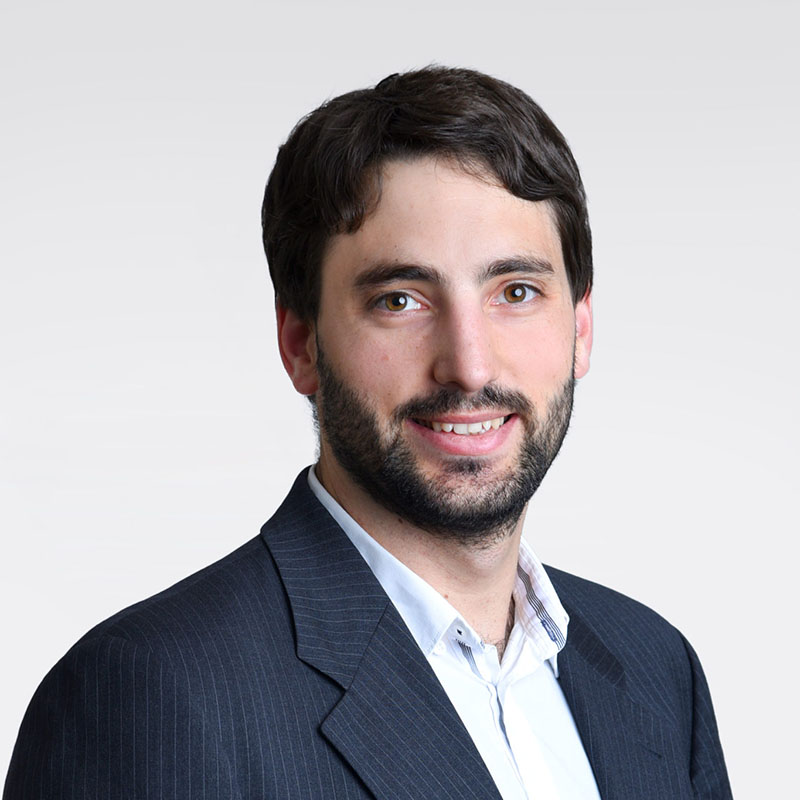
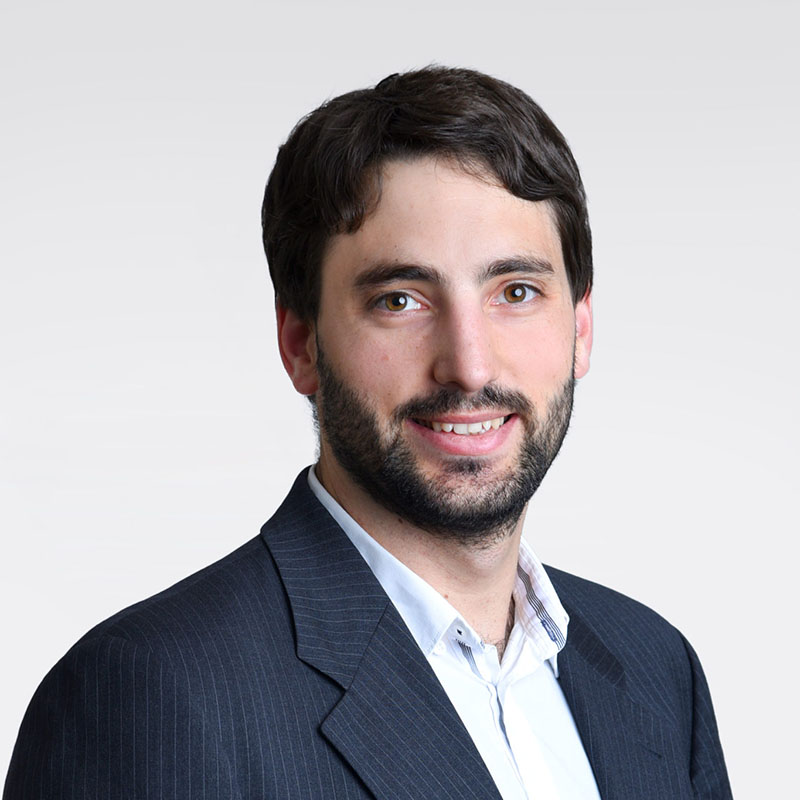
After finishing his Master in electrical engineering at the Ecole Fédérale de Lausanne (EPFL), Nathanaël worked as a researcher in the Acoustic Research Institute (ARI) in Vienna. In 2013, he returned to EPFL for a PhD, where he specialized himself in different fields of data science: signal processing, machine learning, graph theory and optimization. Furthermore, he created two open source libraries for optimization (UNLocBoX) and graph signal processing (GSPBOX). Since 2017, Nathanaël Perraudin is a Research Data Scientist at the Swiss Data Science Center in the ETH Zurich. He focuses on different aspects of deep learning in the area of generative models (VAE and GAN), recursive architectures and convolutional neural network for irregular domains. Outside office hours, he is passionate by tango dancing, tandem bike touring, skiing and rock climbing.
description
The main goal of this project is to capitalise on the latest developments in the field of Deep Convolutional Neural Networks (CNN) and Generative Adversarial Networks (GAN) for solving some of the most important problems in the field of Observational Cosmology. The advantage of neural networks over classical statistical methods that are currently used in cosmology is that they are able to learn complex nonlinear relations from the data. Applications of deep learning techniques have the potential to radically improve on existing data analysis methods in several areas of cosmology and astrophysics. In this proposed project, we plan to tackle three specific problems:
- At present the standard statistical measure in weak lensing, and most areas of cosmology, is the power spectrum. For gaussian random fields, it is known that this statistic captures all the available information. However, the fields that we measure in cosmology can be highly non-gaussian making the power spectrum measure suboptimal. We plan to develop a CNN-based method that will allow us to fully capture the non-gaussian features of cosmological data, which will allow us to make more precise measurements.
- Making theoretical predictions for the expected non-gaussian signals in cosmology currently requires extensive numerical simulations that are computationally expensive. In this part of the project we plan to use a GAN which will be trained on cosmological simulations to generate new instances of the cosmic web. This will have the advantage of being extremely fast compared to current existing methods. These fast simulations can be used in tandem with full physical numerical simulations to create a model of cosmological observables and uncertainties, both faster and more accurately.
- Developing algorithms to perform convolutional neural network analysis on the sphere, in the Healpix sampling scheme, which is most commonly used in cosmology. To date, the CNN-based analysis used only 2D image-like data. As the data available for these measurements will soon cover a significant part of the sky, the need of performing the analysis on the sphere will become pressing.
Solving these three problems benefits the machine learning community in several ways. For example, very few quantitative tests of the performance of GANs have been done to date in the field of machine learning. As the application to cosmological data would involve stringent quantitative testing, this project would allow for better evaluations of generative models. Another challenge that will push the limit of existing machine learning techniques is the need for processing large three-dimensional cosmological data. Most existing deep learning methods work on 2-dimensional data and although the extension to 3-dimensional data is straightforward, it nevertheless comes with additional constraints on the amount of memory required, especially when using GPUs. We here would like to exploit advanced analytic solutions to reduce the memory footprint of neural networks for 3-dimensional data. The development of such solutions is likely to benefit other scientific fields that have similar types of data to analyse, including the medical domain or material science.
An important part of the technology development process is taking a prototype algorithm and transforming it into a production-ready framework, which can benefit the community. A number of data science challenges will be present in that process, which would be very hard to address without the SDSC expertise. Such challenges may include: making the models work on 3- or higher- dimensional data, scaling the problem to use higher resolution images, building a platform for efficient training of the methods for multiple cosmological models, optimising network architectures and training strategies (optimisation engines, regularisation), creating a framework where experiments can be efficiently and systematically repeated to assure the quantitative accuracy of the methods, such that they meet the science requirements and can benefit the community of users.
Presentation
Gallery

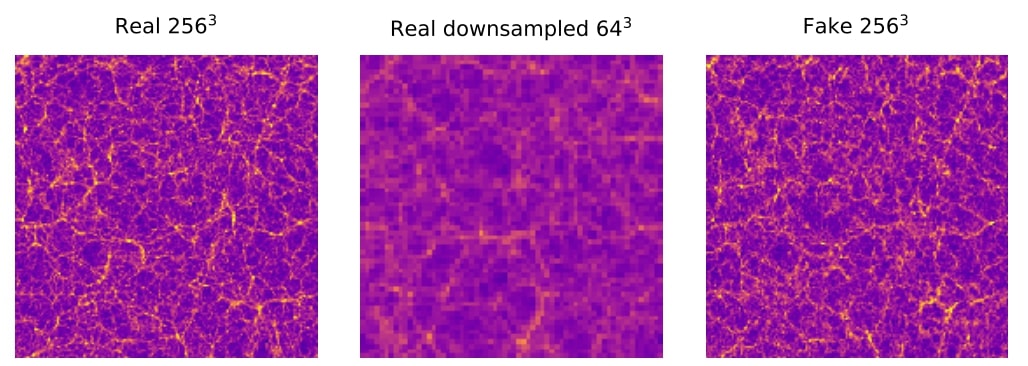
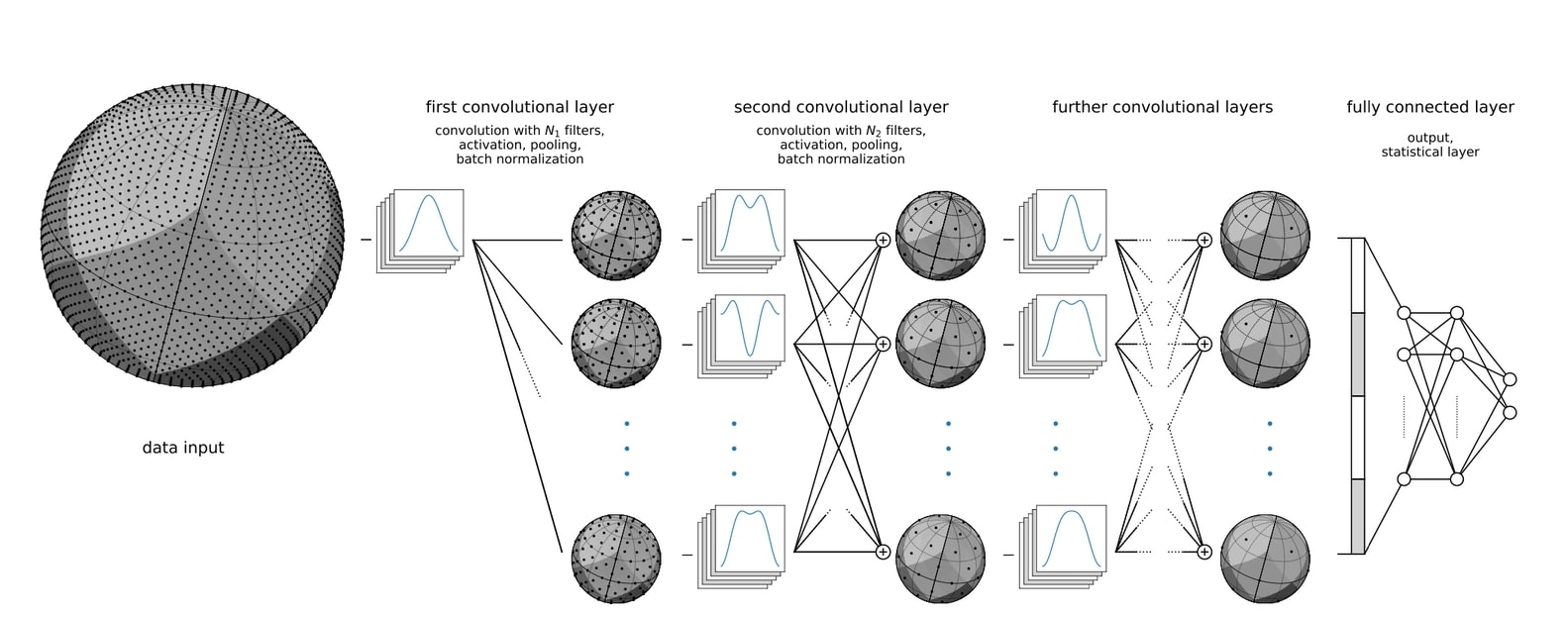
Annexe
Additionnal resources
Bibliography
- Dark Energy Survey Collaboration, 2016. Cosmology from cosmic shear with Dark Energy Survey Science Verification data, Physical Review D, Volume 94.
- Dietrich and Hartlap, 2010. Cosmology with the shear-peak statistics, Monthly Notices of the Royal Astronomical Society, Volume 402, Issue 2.
- Haider et al., 2015, Large-scale mass distribution in the Illustris simulation, monthly Notices of the Royal Astronomical Society, 457, 3, (2016), arXiv:1508.01525
- Howlett and Manera and Percival, 2015. L-PICOLA: A parallel code for fast dark matter simulation, Astronomy and Computing, Volume 12.
- Hubara, I., Courbariaux, M., Soudry, D., El-Yaniv, R., & Bengio, Y. (2016). Quantized neural networks: Training neural networks with low precision weights and activations. arXiv preprint arXiv:1609.07061.
- Kingma, Diederik Welling, 2014. Auto-encoding variational bayes, International Conference on Learning Representations.
- Kacprzak et al, Cosmology constraints from shear peak statistics in Dark Energy Survey Science Verification data, Monthly Notices of the Royal Astronomical Society, 463, 4 (2016)
- Krizhevsky, Alex, Ilya Sutskever, and Geoffrey E. Hinton. "Imagenet classification with deep convolutional neural networks." Advances in neural information processing systems. 2012.
- Goodfellow, I. et al, 2014, Generative adversarial nets, NIPS.
- Hildebrandt et al 2016, KiDS-450: cosmological parameter constraints from tomographic weak gravitational lensing, Monthly Notices of the Royal Astronomical Society, 465, 2 (2016)
- Lin, Chieh-An and Kilbinger, 2015. A new model to predict weak-lensing peak counts I. Comparison with N-body Simulations, Astronomy & Astrophysics, Volume 583.
- Petri et al, 2014. Cosmology with Minkowski functionals and moments of the weak lensing convergence field, Physical Review D, vol. 88, Issue 12.
- Potter, Douglas, Stadel, Teyssier, 2016. PKDGRAV3: Beyond Trillion Particle Cosmological Simulations for the Next Era of Galaxy Surveys, arXiv:1609.08621
- Refregier, 2003. Weak Gravitational Lensing by Large-Scale Structure, Annual Review of Astronomy and Astrophysics 41
- Rezende, Jimenez, Mohamed, Wierstra, 2014, Stochastic backpropagation and approximate inference in deep generative models, International Conference on Machine Learning.
- Schawinski, Kevin, et al. "GalaxyGAN: Generative Adversarial Networks for recovery of galaxy features." Astrophysics Source Code Library (2017).
- Scoville et al, 2007. The Cosmic Evolution Survey (COSMOS): Overview, The Astrophysical Journal Supplement Series, Volume 172, Issue 1.
- Taniguchi et al, 2007. The Cosmic Evolution Survey (COSMOS): Subaru Observations of the HST Cosmos Field, The Astrophysical Journal Supplement Series, Volume 172, Issue 1.
- Springel et al, 2005. Simulating the joint evolution of quasars, galaxies and their large-scale distribution, Nature. 435 (7042).
- Berge et al (+AA, AR), An Ultra Fast Image Generator (UFIG) for wide-field astronomy, Astronomy and Computing, Volume 1, p. 23-32. (2013) arXiv:1209.1200
- Mustafa et al. 2017, Creating Virtual Universes Using Generative Adversarial Networks, CosmoGAN: creating high-fidelity weak lensing convergence maps...
- Herbel et al. 2017, The redshift distribution of cosmological samples: a forward modeling approach, CosmoGAN: creating high-fidelity weak lensing convergence maps...
- Schmelzle, TK, AL, et al 2017 Cosmological model discrimination with Deep Learning
Publications
Related Pages
More projects
CLIMIS4AVAL
News
Latest news
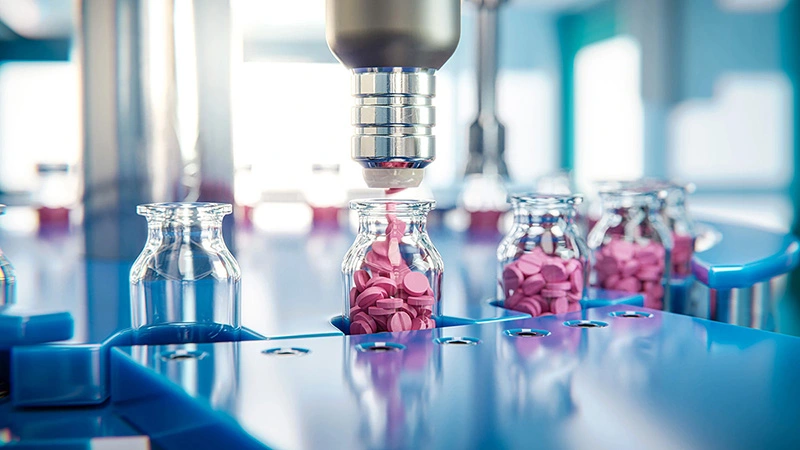
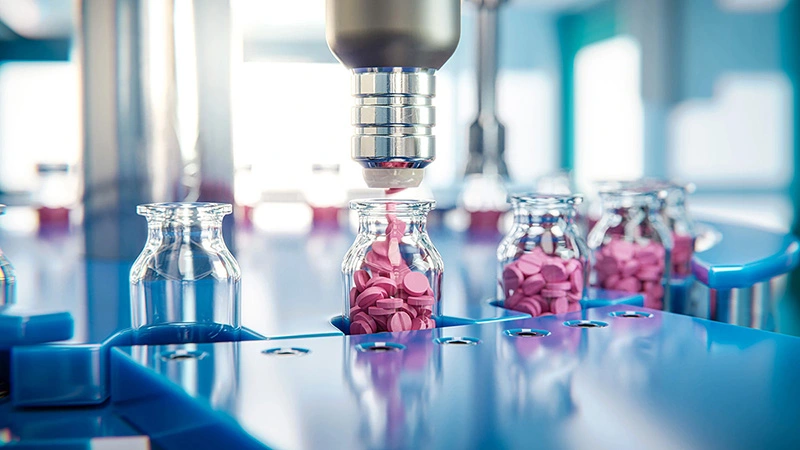
The Promise of AI in Pharmaceutical Manufacturing
The Promise of AI in Pharmaceutical Manufacturing
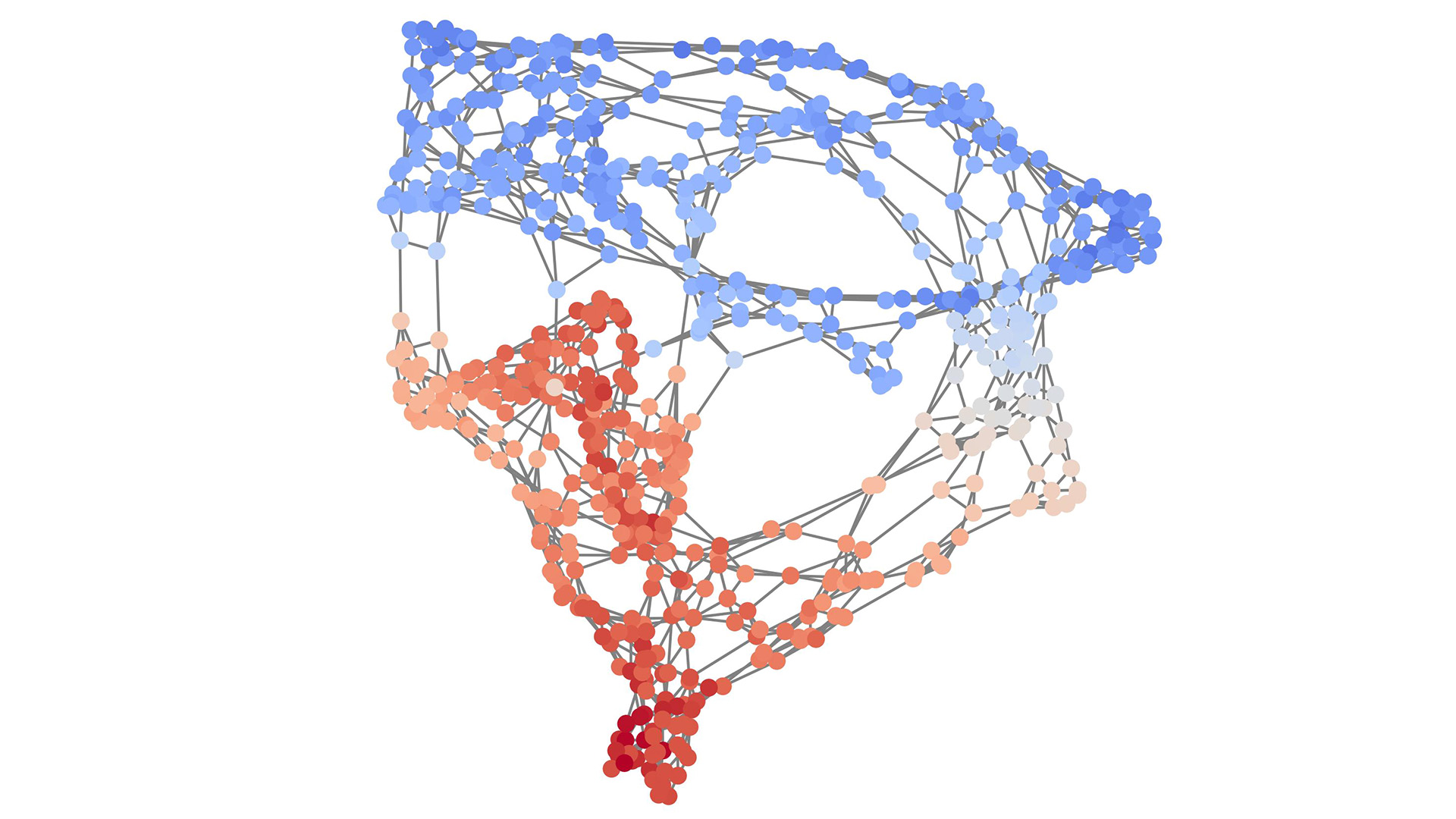
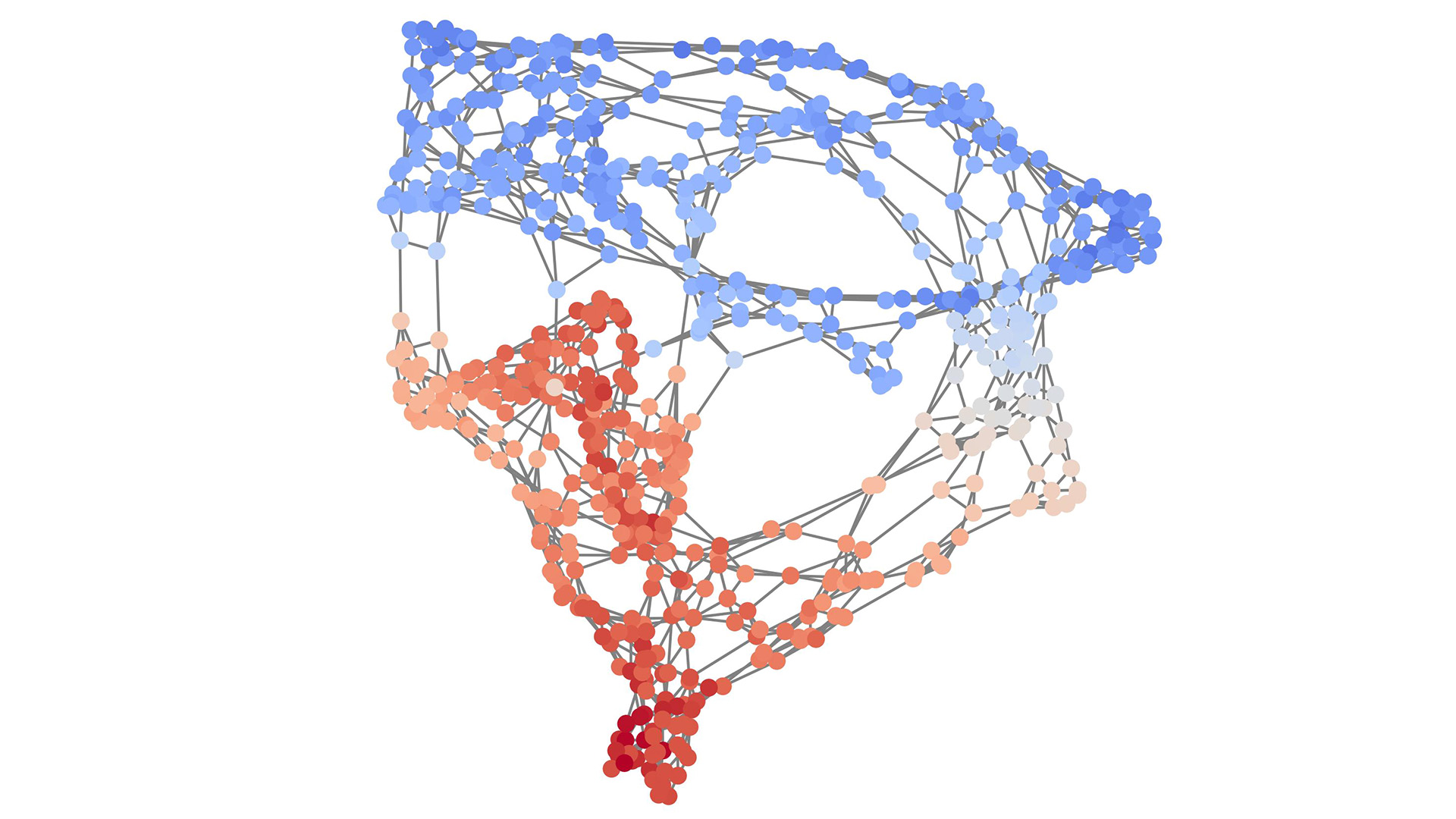
Efficient and scalable graph generation through iterative local expansion
Efficient and scalable graph generation through iterative local expansion


RAvaFcast | Automating regional avalanche danger prediction in Switzerland
RAvaFcast | Automating regional avalanche danger prediction in Switzerland
Contact us
Let’s talk Data Science
Do you need our services or expertise?
Contact us for your next Data Science project!