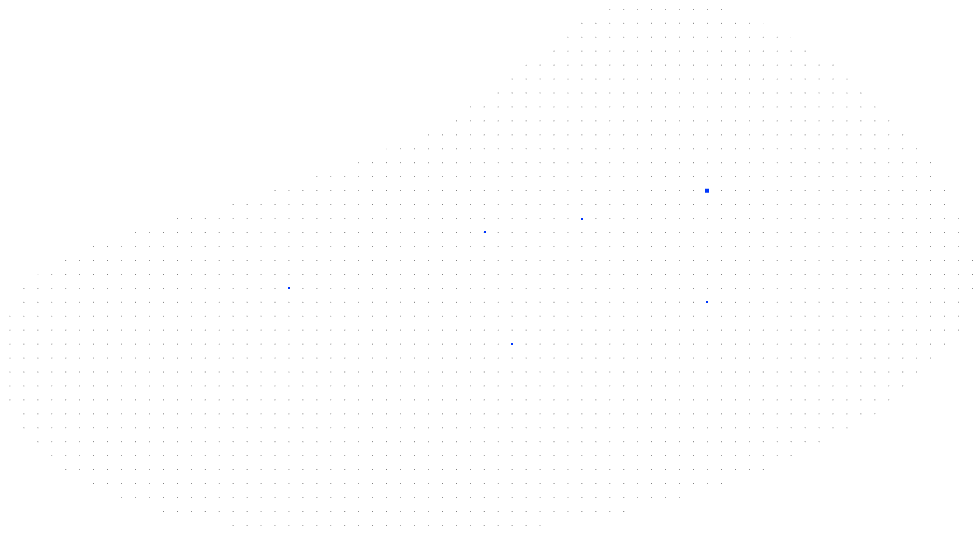
ML4FCC
Machine Learning for the Future Circular Collider Design

Abstract
The design and operation of the Future Circular Collider at the Council of the European Organization for Nuclear Research as a precision instrument for particle physics is an exciting Big Data and Machine Learning opportunity. Accelerator performance is characterized by the dynamic aperture (DA), which represents the size of the area in phase space where the beam particles feature stable behavior under long-term tracking. Particles located in the chaotic and unstable areas will be lost from the beam and will reduce its lifetime, so measuring the DA is vital. However, this is currently done with particle tracking simulations, which are computationally slow and expensive. A key aspect of accelerator design is to maximize the DA by properly adjusting thousands of control parameters. This requires the development of data-driven algorithms for predicting the DA, and efficiently sampling at fine resolution around its boundary when the predictive uncertainty is high.
People
Collaborators
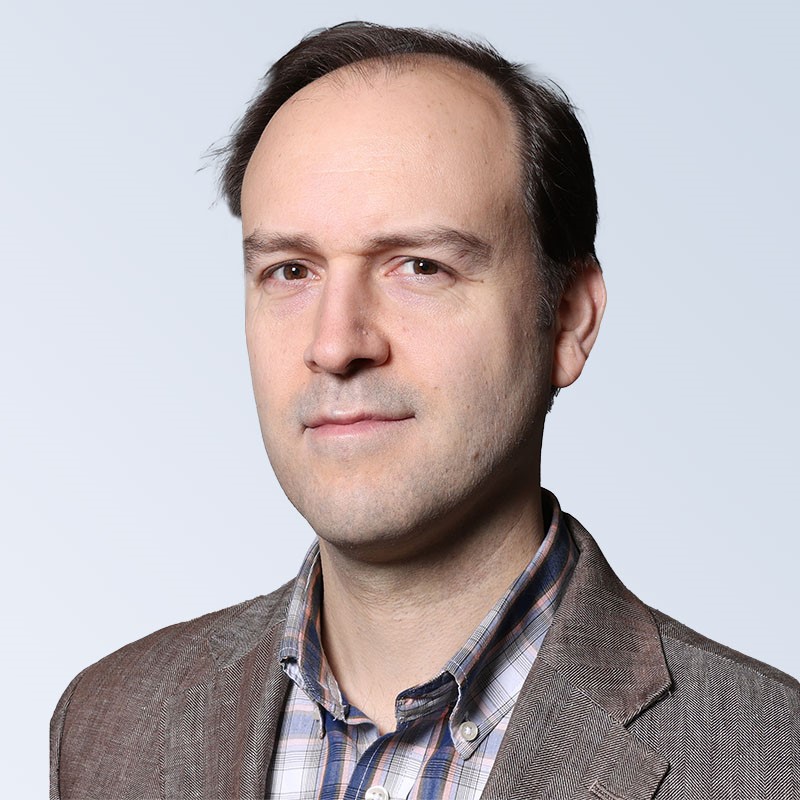
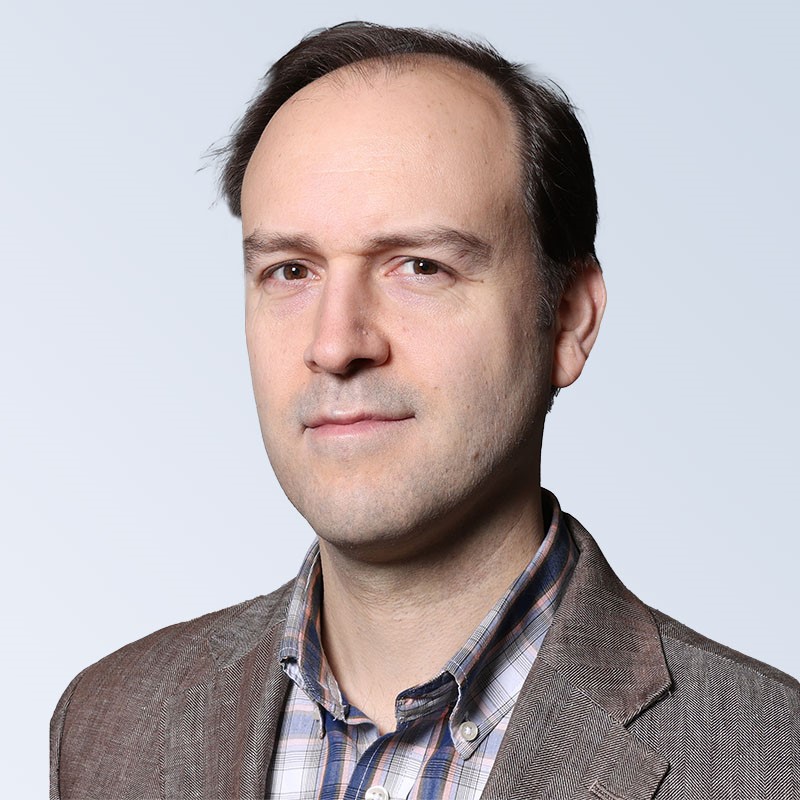
Guillaume Obozinski graduated with a PhD in Statistics from UC Berkeley in 2009. He did his postdoc and held until 2012 a researcher position in the Willow and Sierra teams at INRIA and Ecole Normale Supérieure in Paris. He was then Research Faculty at Ecole des Ponts ParisTech until 2018. Guillaume has broad interests in statistics and machine learning and worked over time on sparse modeling, optimization for large scale learning, graphical models, relational learning and semantic embeddings, with applications in various domains from computational biology to computer vision.
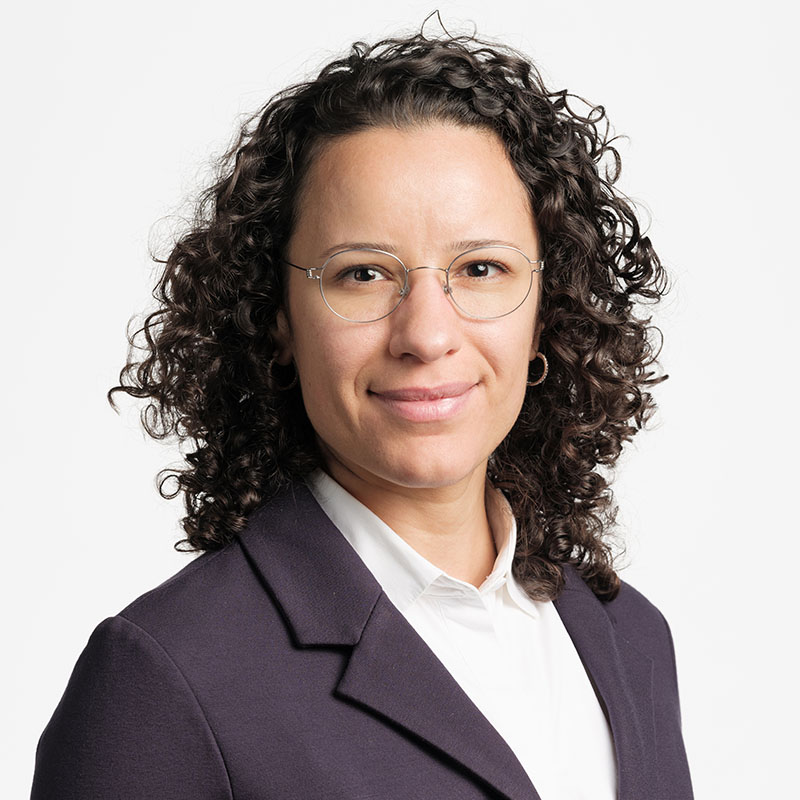
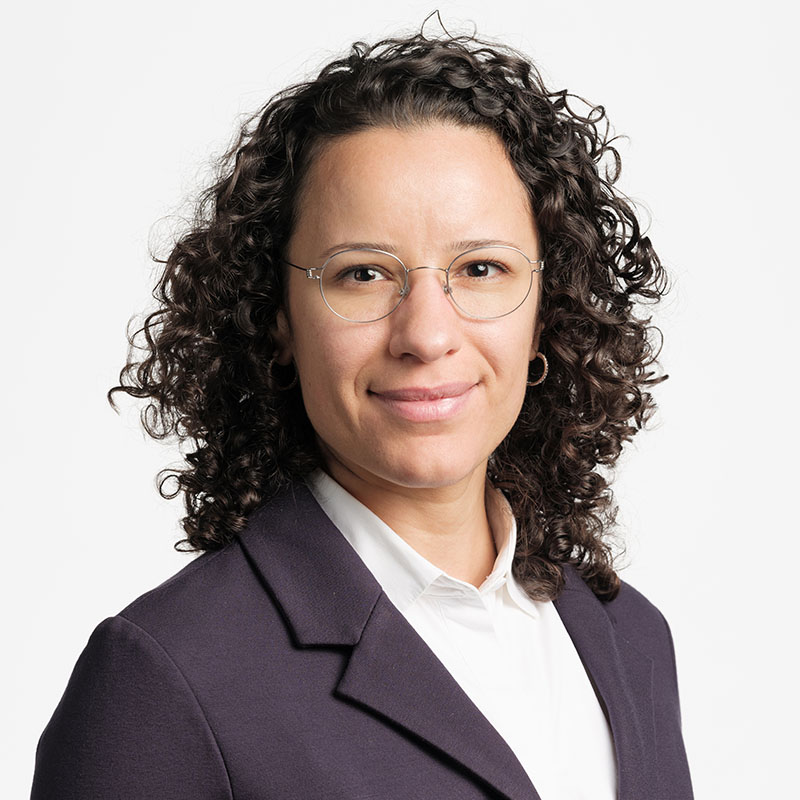
Yousra studied Mathematics and Computational Statistics. She did a PhD in Statistical Learning followed by a post-doc both at EPFL, where she developed empirical Bayes methods for automatic L2 regularization problems in smooth regression for big data. Before joining SDSC, she worked at SIB/UNIL, where she developed a statistical optimization solution to the parent-of-origin identification problem in human genetics. Her technical expertise includes supervised learning, numerical optimization for machine learning, statistical modeling and methodology, high-performance and distributed computing for big data, Bayesian computation and time series analysis. She worked on applied problems in quantitative finance and environmental sciences.
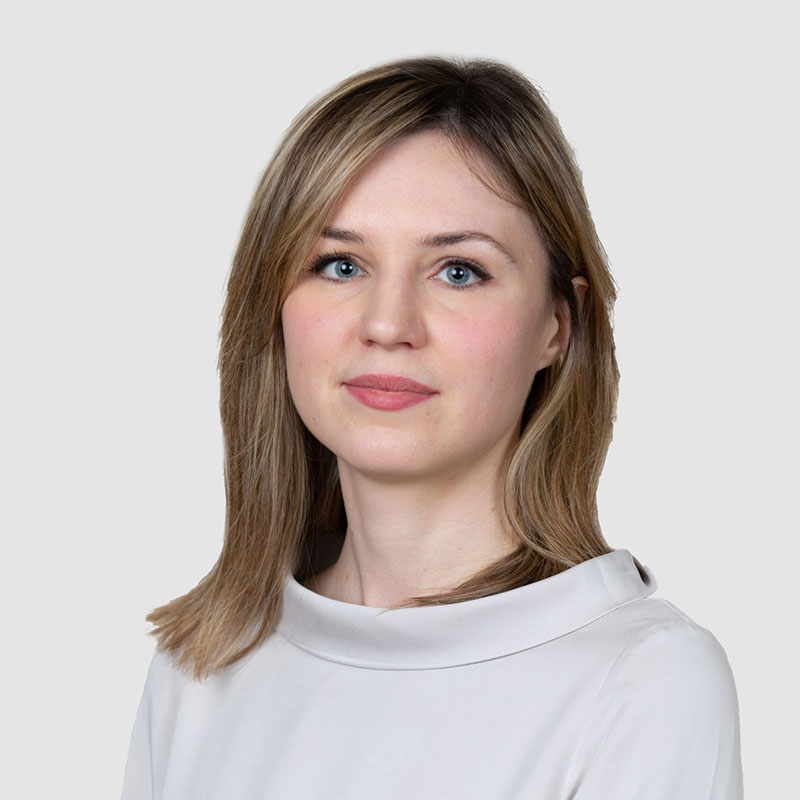
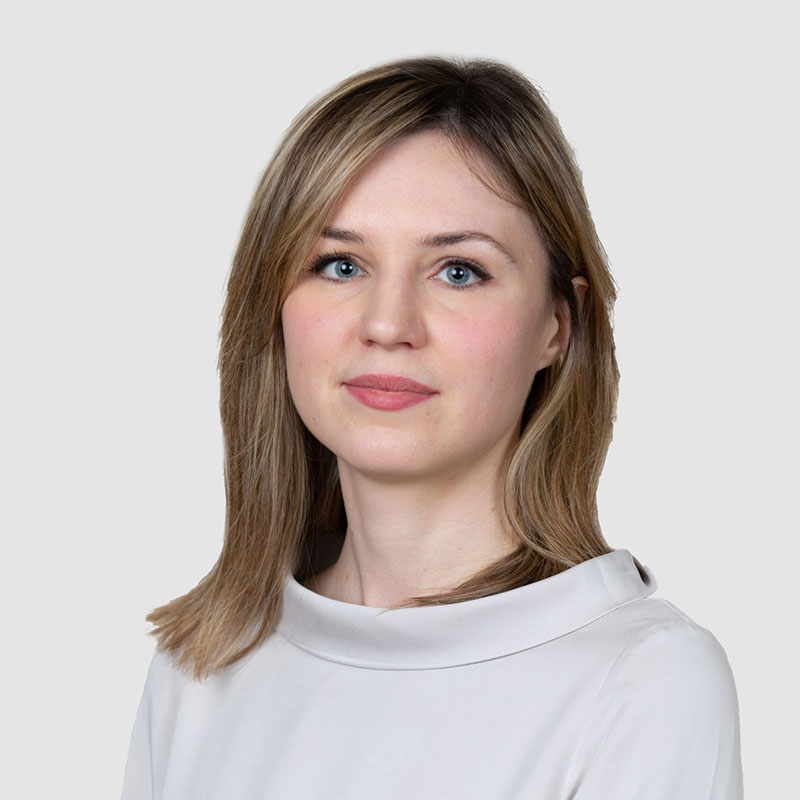
Ekaterina received her PhD in Computer Science from Moscow Institute for Physics and Technology, Russia. Afterwards, she worked as a researcher at the Institute for Information Transmission Problems in Moscow and later as a postdoctoral researcher in the Stochastic Group at the Faculty of Mathematics at University Duisburg-Essen, Germany. She has experience with various applied projects on signal processing, predictive modelling, macroeconomic modelling and forecasting, and social network analysis. She joined the SDSC in November 2019. Her interests include machine learning, non-parametric statistical estimation, structural adaptive inference, and Bayesian modelling.
PI | Partners:
description
Motivation
The dynamic aperture is currently measured with particle tracking simulations, which are computationally slow and expensive. Moreover, different sets of control parameter values may lead to different representations of the DA as illustrated below with three examples in Figure 1. Parametric representations of the DA are therefore difficult to define, raising thus two challenging objectives: i) to develop a machine learning model of the stable phase space area as a function of the accelerator control parameters; and ii) to develop an active learning algorithm that provides a targeted and efficient search for optimal machine configurations that lead to the largest DA.
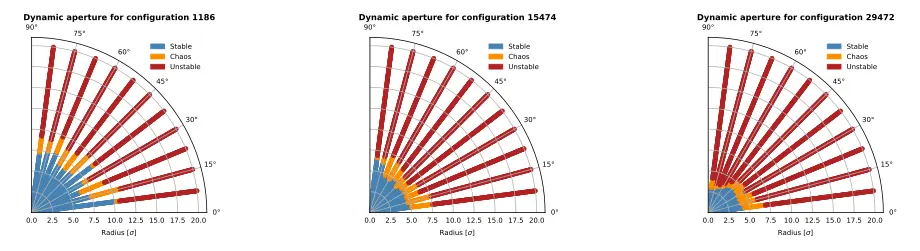
Proposed Approach / Solution
We predict the stable and unstable regions in the accelerator by using a residual neural network (ResNet) with a feature selection layer, and blocks of fully connected layers whose weights are constrained by spectral normalization. Figure 2 shows the predictive performance of the best model.

Figure 3 illustrates the predictive performance on three test configurations.

Impact
The results obtained in the project have the potential to revolutionize accelerator design by focusing the automated tracking of particles only on stable areas of the phase space since particles in the unstable areas will anyway be lost. This will optimize the performance of an expensive research infrastructure, and will enable faster scientific discoveries.
Presentation
Gallery
Annexe
Additional resources
Bibliography
Publications
Related Pages
More projects
ML-L3DNDT
BioDetect
News
Latest news
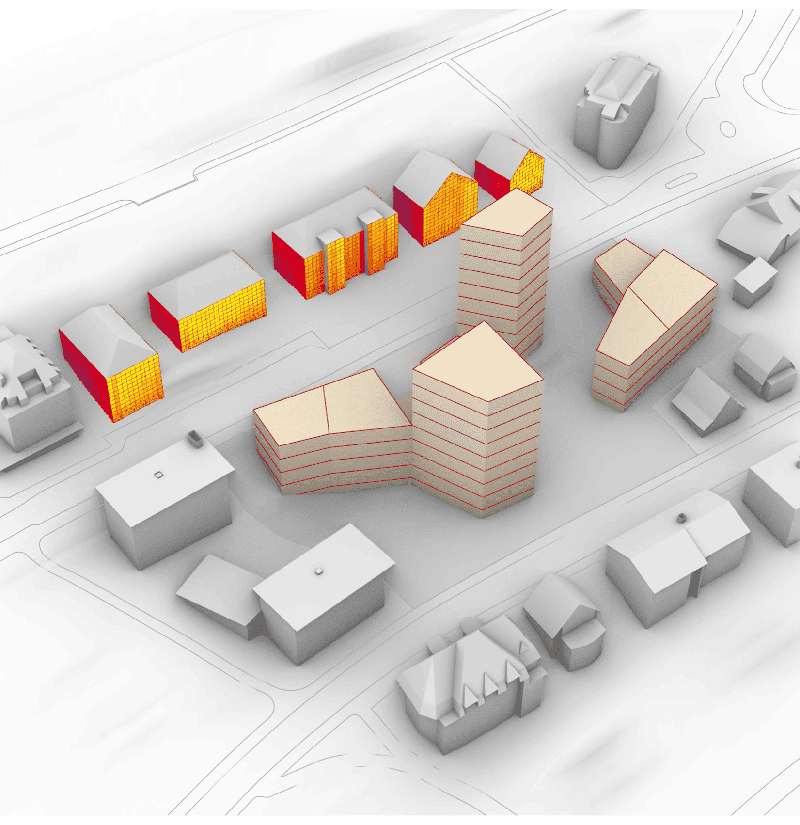
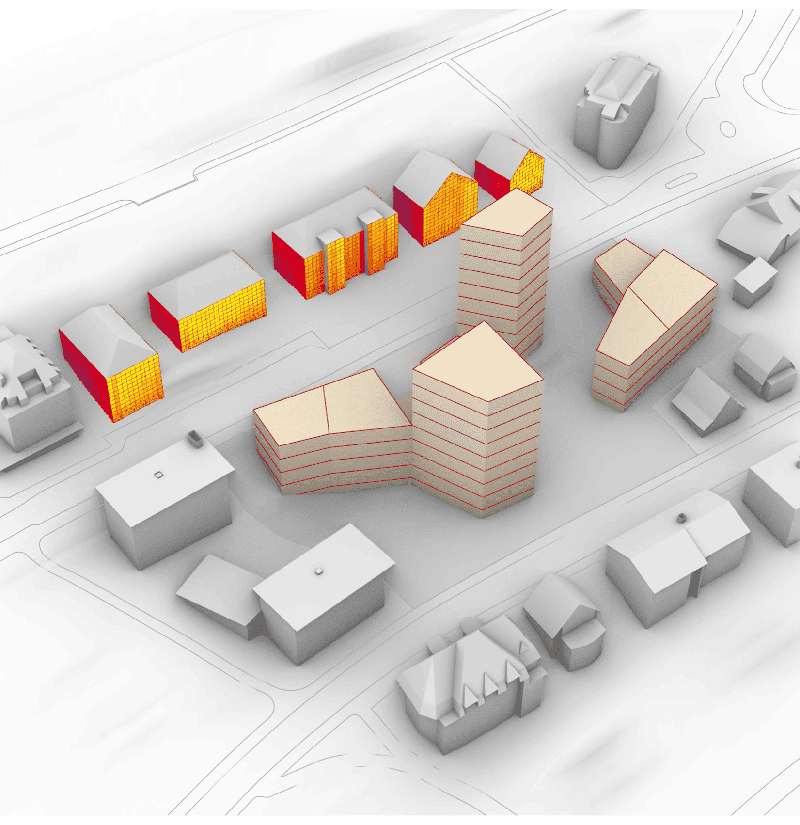
AIXD | Generative AI toolbox for architects and engineers
AIXD | Generative AI toolbox for architects and engineers


Smartair | An active learning algorithm for real-time acquisition and regression of flow field data
Smartair | An active learning algorithm for real-time acquisition and regression of flow field data
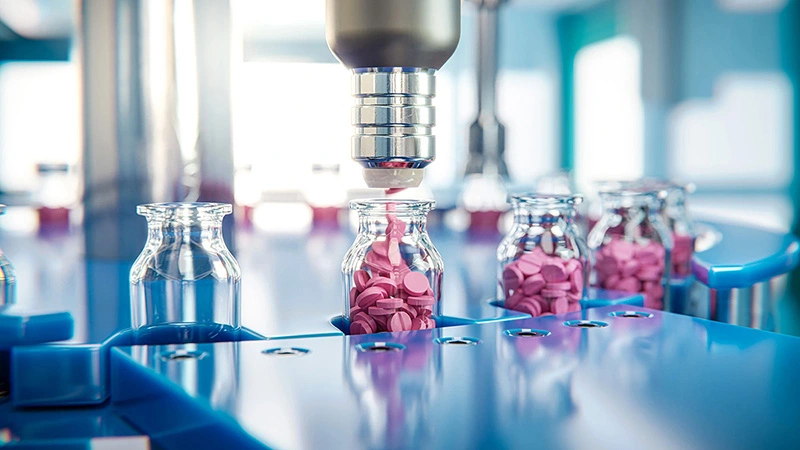
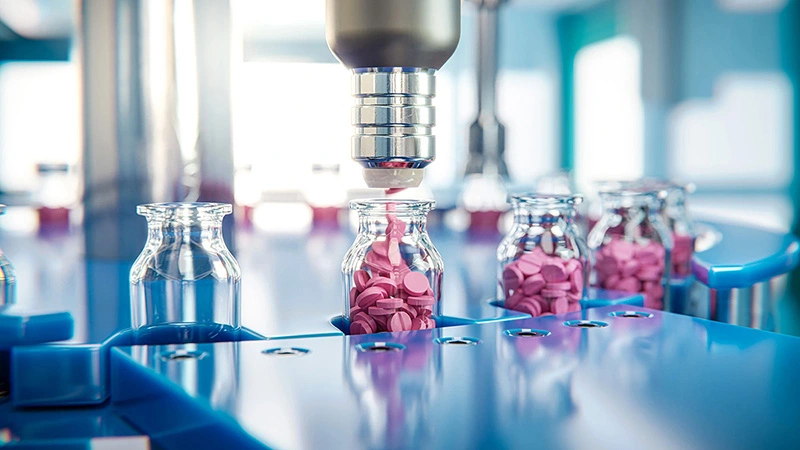
The Promise of AI in Pharmaceutical Manufacturing
The Promise of AI in Pharmaceutical Manufacturing
Contact us
Let’s talk Data Science
Do you need our services or expertise?
Contact us for your next Data Science project!