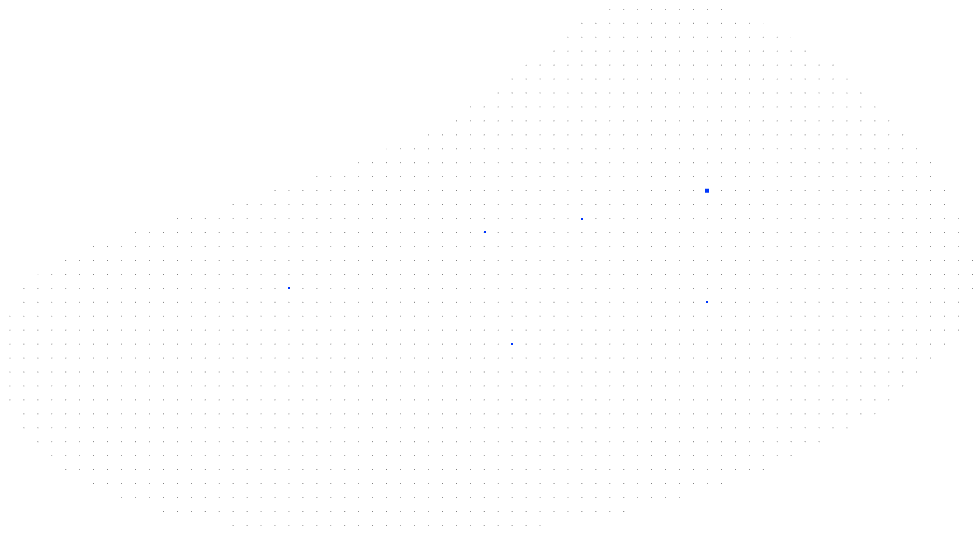
TE4med
Transposcriptome-based identifier for precision medicine

Abstract
People
Collaborators


Luis is originally from Spain, where he completed his bachelor's studies in Electrical engineering, and the Ms.C. on signal theory and communications, both at the University of Seville. During his Ph.D. he started focusing on machine learning methods, more specifically message passing techniques for channel coding, and Bayesian methods for channel equalization. He carried it out between the University of Seville and the University Carlos III in Madrid, also spending some time at the EPFL, Switzerland, and Bell Labs, USA, where he worked on advanced techniques for optical channel coding. When he completed his Ph.D. in 2013, he moved to the Luxembourg Center on Systems Biomedicine, where he switched his interest to neuroscience, neuroimaging, life sciences, etc., and the application of machine learning techniques to these fields. During his 4 and a half years there as a Postdoc, he worked on many different problems as a data scientist, encompassing topics such as microscopy image analysis, neuroimaging, single-cell gene expression analysis, etc. He joined the SDSC in April 2018. As Lead Data Scientist, Luis coordinates projects in various domains. Several projects focus on the application of natural language processing and knowledge graphs to the study of different phenomena in social and political sciences. In the domains of architecture and engineering, Luis is responsible for projects centered on the application of novel generative methods to parametric modeling. Finally, Luis also coordinates different projects in robotics, ranging from collaborative robotic construction to deformable object manipulation.
description
Problem:
Precision oncology relies on the characterization of molecular signatures of diagnostic and treatment selection value. An algorithm that ensures not only high sensitivity and specificity, but also extreme precision in the identification of tumor subtypes is key and missing.
Proposed approach:
Combine:
- Advanced ML techniques.
- The power of Transposable Elements as a highdensity barcode for cell identity.
- High-throughput sequencing big data. To obtain biomarkers that define specific, sensitive and robust classifiers.
Impact:
- Trace the origin of tumors.
- Predict their sensitivity to particular treatments.
- Monitor therapeutic responses.
- Detect relapses as early as possible.
- Identify new therapeutic targets.
Presentation
Gallery
Annexe
Additional resources
Bibliography
Publications
Related Pages
More projects
MAGNIFY
News
Latest news
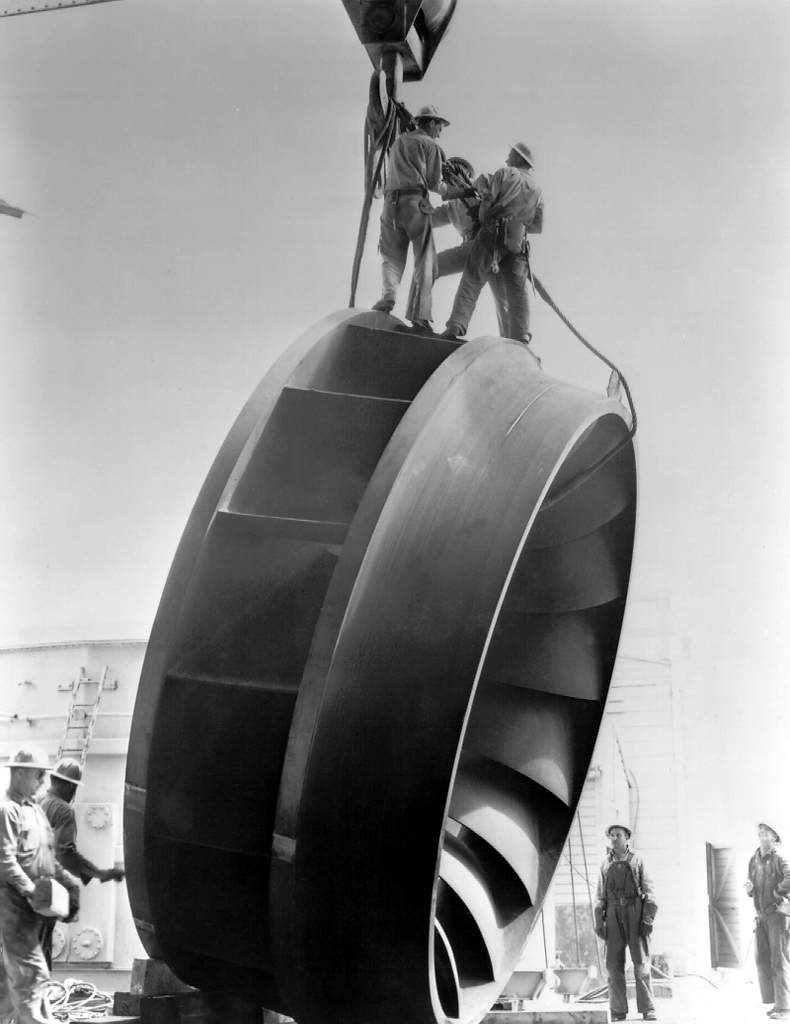
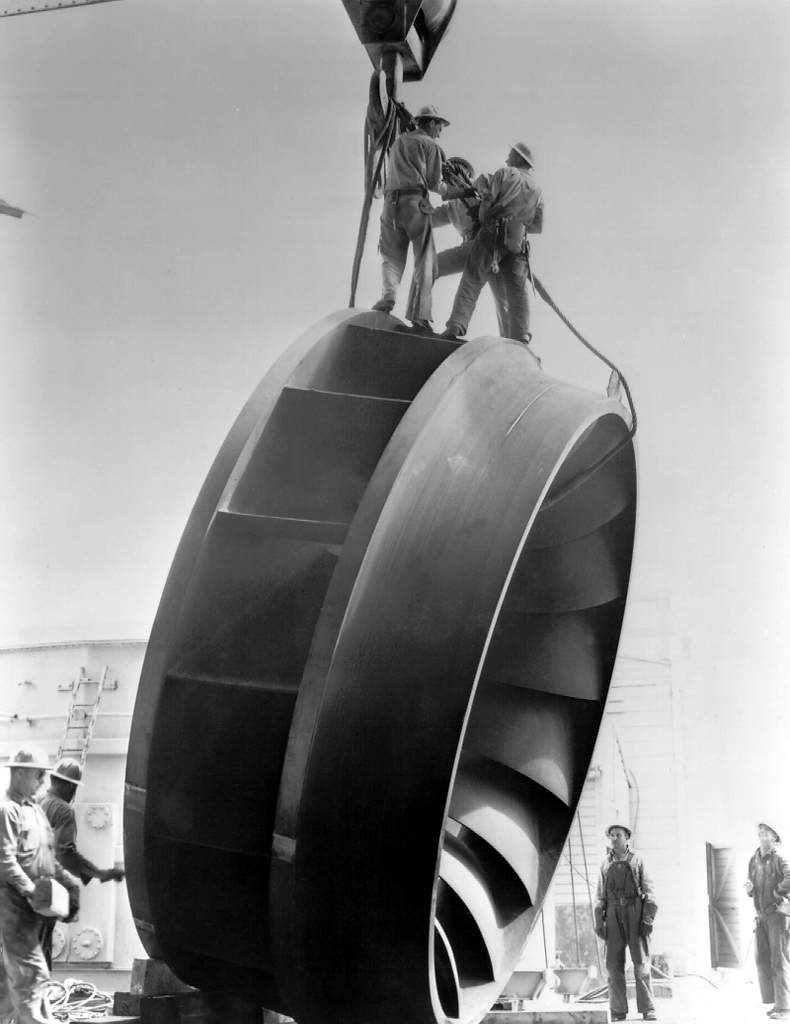
PAIRED-HYDRO | Increasing the Lifespan of Hydropower Turbines with Machine Learning
PAIRED-HYDRO | Increasing the Lifespan of Hydropower Turbines with Machine Learning


First National Calls: 50 selected projects to start in 2025
First National Calls: 50 selected projects to start in 2025
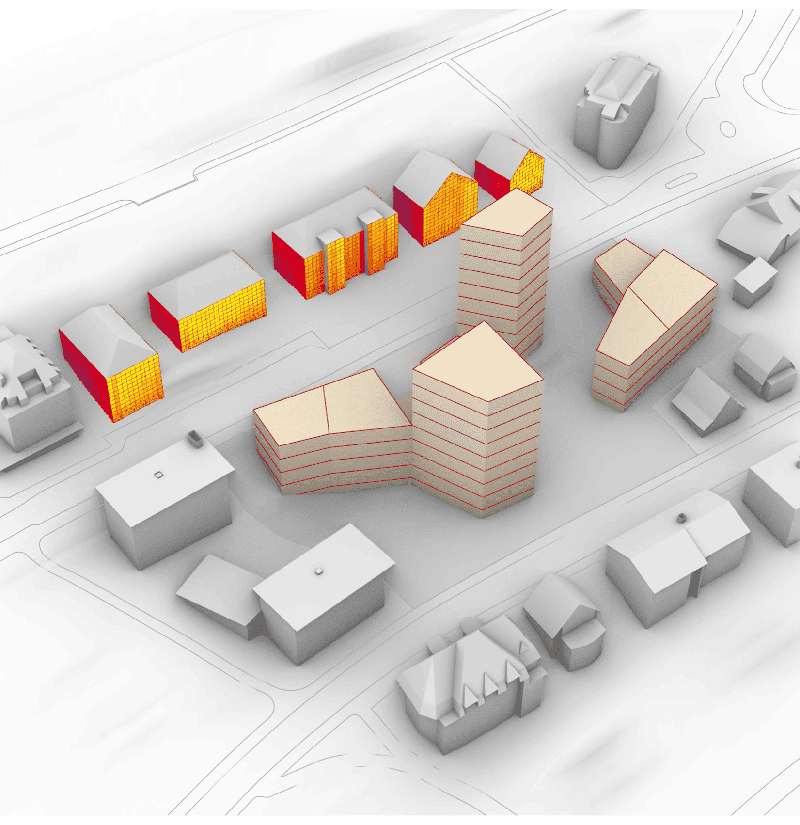
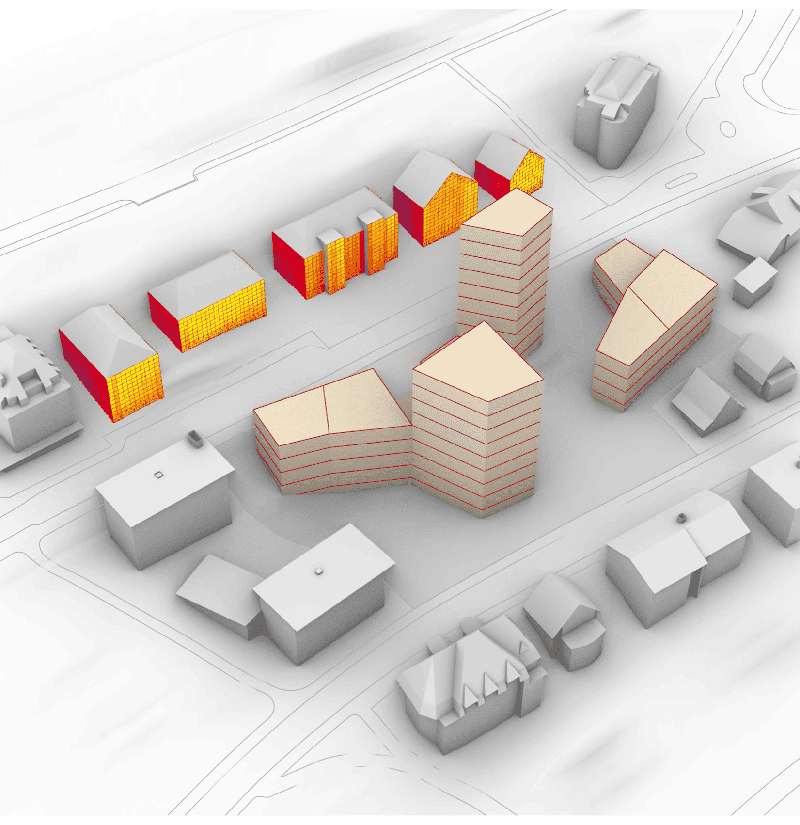
AIXD | Generative AI toolbox for architects and engineers
AIXD | Generative AI toolbox for architects and engineers
Contact us
Let’s talk Data Science
Do you need our services or expertise?
Contact us for your next Data Science project!