
AURORA
from Air pollUtion souRces tO moRtAlity
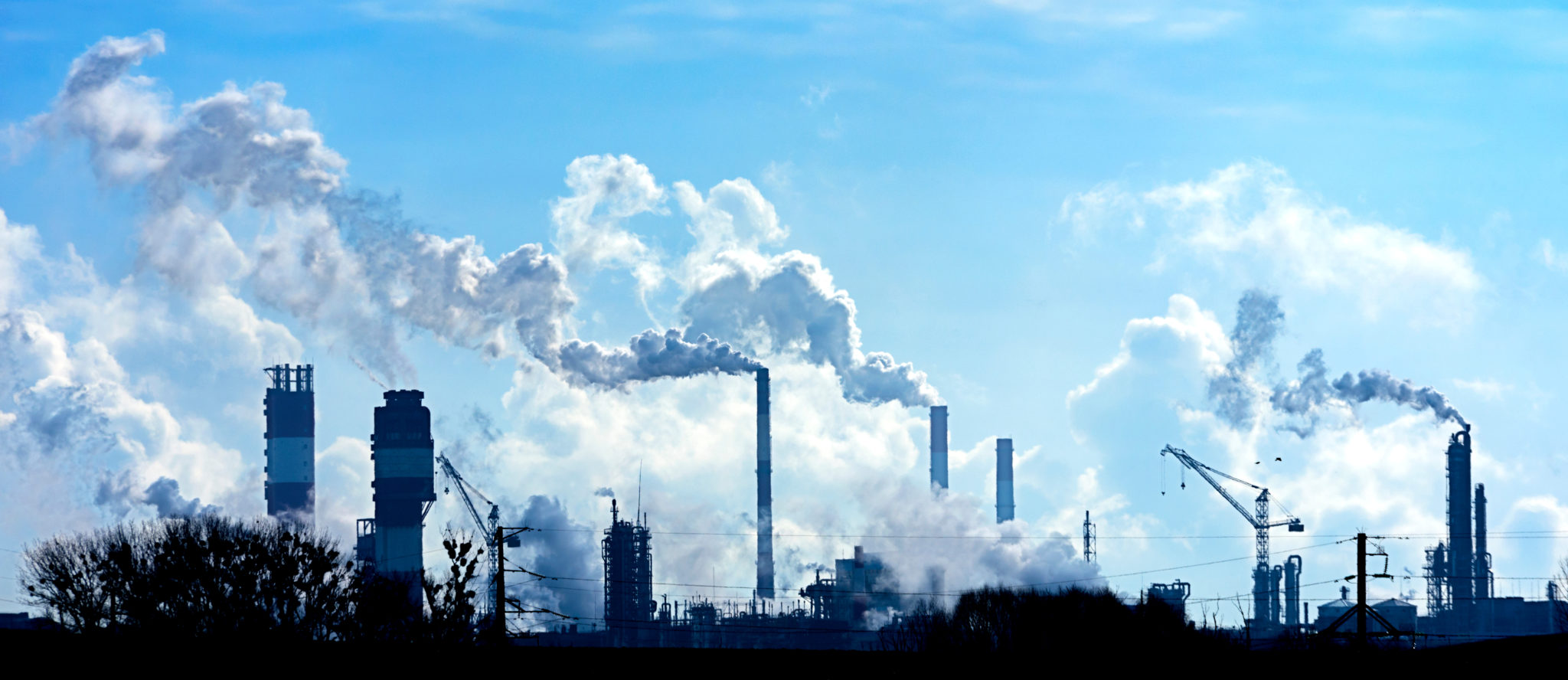
Abstract
Atmospheric aerosols (particulate matter, PM) are liquid or solid particles suspended in the air with diameters ranging from a few nanometers to tens of micrometers. Poor air quality associated with high levels of PM is a major public health problem, and is one of the five leading causes of premature deaths worldwide, alongside high blood pressure, smoking, diabetes, and obesity.
Human exposure to PM caused ~8.9 million deaths, or ~10% of the total global mortality burden in 2015, more than car accidents, HIV, and malaria combined. Without any action, these numbers are expected to double by 2050.
PM health effects can be acute and chronic and have been associated with cardiovascular diseases, respiratory symptoms, cancer, diabetes, sudden infant mortality, and neurodegenerative diseases (upon penetrating the blood-brain barrier). The magnitude of the association between PM exposure and the probability of death is based on the total PM mass, while PM’s health effects are strongly driven by its chemical composition and size, and hence its origin.
PM originates from natural (e.g. volcanos, pollen) or anthropogenic (e.g. combustion) sources, and can be primary from direct emissions (e.g. metals from vehicular wear) or secondary, formed in the atmosphere through complex oxidation mechanisms of gaseous precursors (e.g. from trees, car/industrial exhaust, residential heating).
Our ability to identify the major PM sources responsible for health outcomes is a two-fold challenge that requires (1) a fundamental understanding of PM emissions and formation processes and (2) the consideration of the high diversity and spatial heterogeneity of PM emissions, especially in urban settings where most of the population resides.
AURORA unifies the expertise from distinct fields of science, including analytical & atmospheric chemistry, numerical modeling, epidemiology, and medical science, to propose an innovative modeling framework, which integrates data science, geo-statistics, and process-based simulations to achieve a unique combination of source specificity, spatial and temporal coverage and resolution required for human exposure assessments. Model outputs will be combined with invaluable records of acute and chronic diseases developed and maintained over the course of 30 years to derive the pathogenicity of PM sources and their contribution to different health outcomes on a European scale.
People
Collaborators


Daniel worked as a postdoctoral researcher on critical event prediction for the University Hospital in Zurich. In addition, Daniel has worked as a postdoctoral researcher in Lausanne, delivering algorithms for Bayesian inference in big panel data. Previously in Paris, he developed models for automated scientific discovery. He obtained a Ph.D. from the University of Edinburgh, funded by a Microsoft Research scholarship. His interest relates primarily to attacking applied biomedicine problems from different angles, frequentist statistics, Bayesian statistics, and Machine Learning.
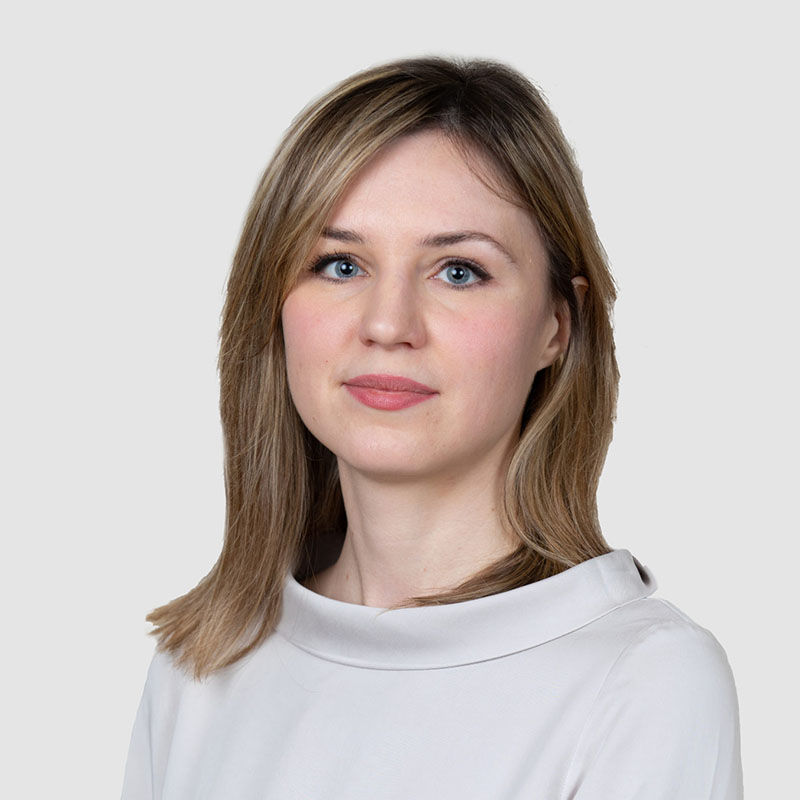
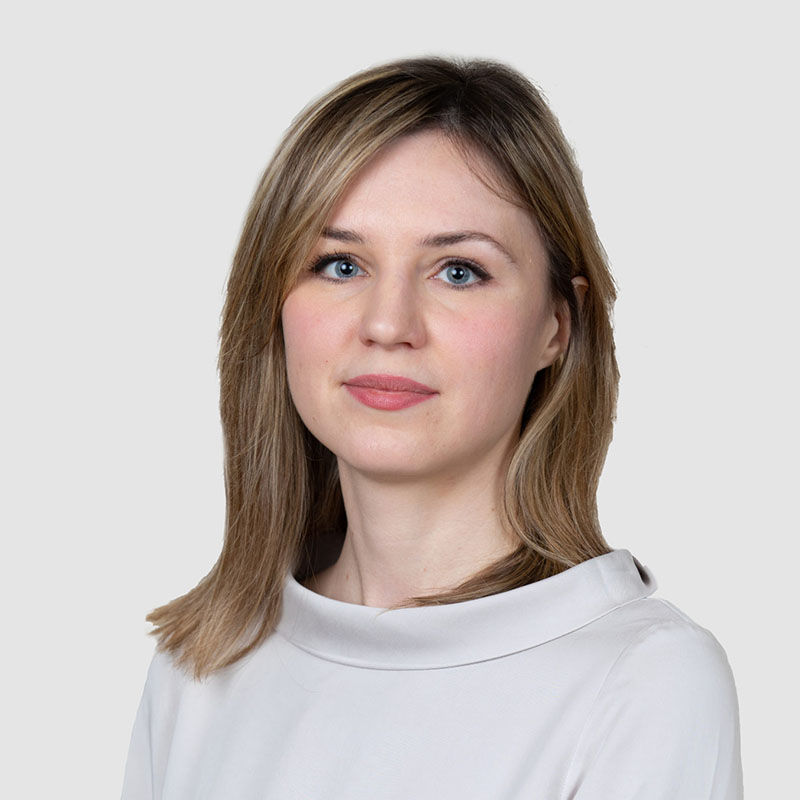
Ekaterina received her PhD in Computer Science from Moscow Institute for Physics and Technology, Russia. Afterwards, she worked as a researcher at the Institute for Information Transmission Problems in Moscow and later as a postdoctoral researcher in the Stochastic Group at the Faculty of Mathematics at University Duisburg-Essen, Germany. She has experience with various applied projects on signal processing, predictive modelling, macroeconomic modelling and forecasting, and social network analysis. She joined the SDSC in November 2019. Her interests include machine learning, non-parametric statistical estimation, structural adaptive inference, and Bayesian modelling.
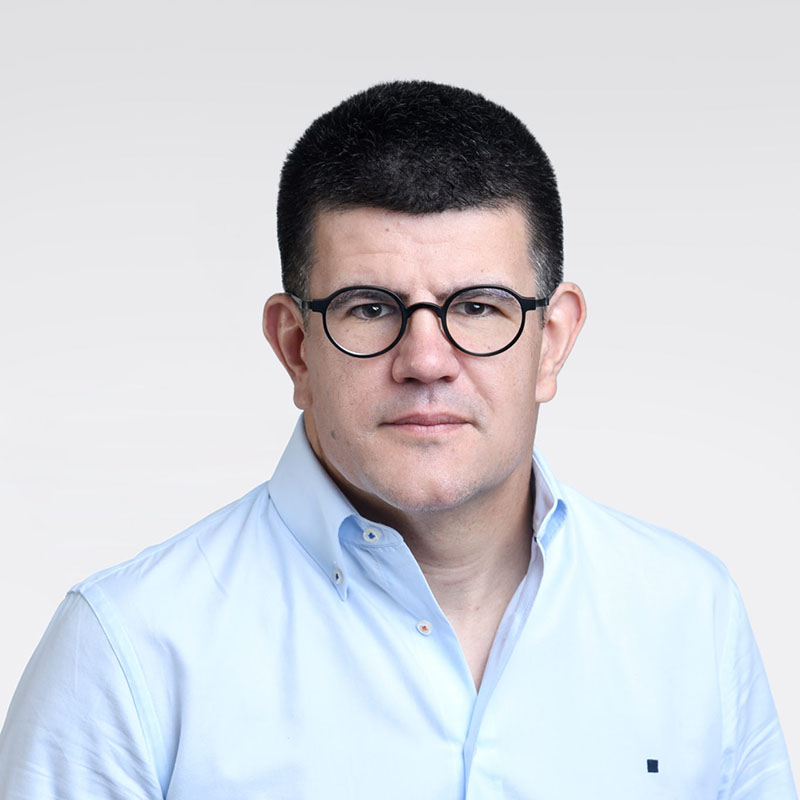
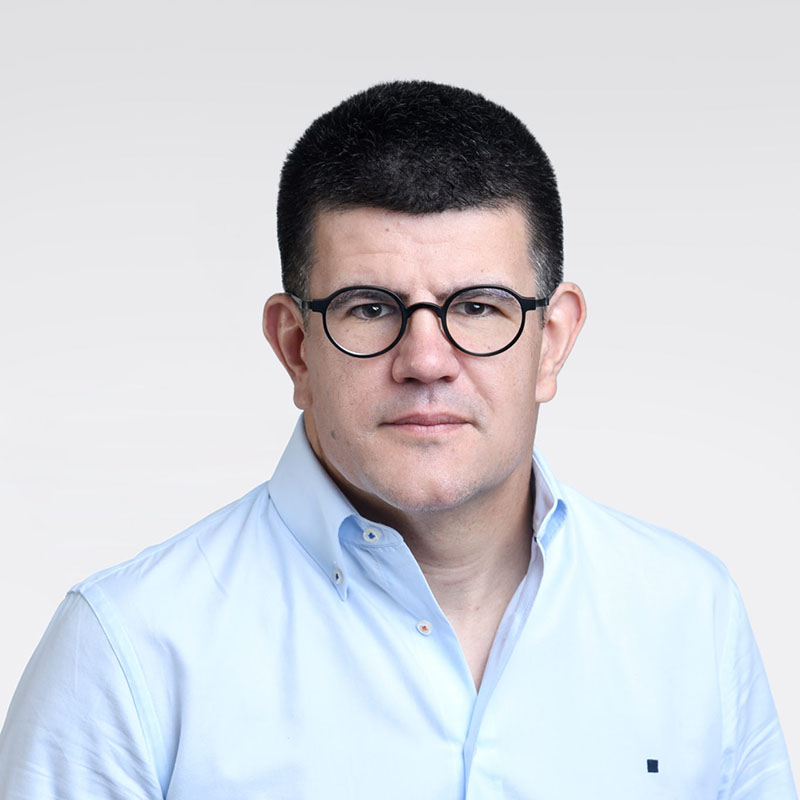
Fernando Perez-Cruz received a PhD. in Electrical Engineering from the Technical University of Madrid. He is Titular Professor in the Computer Science Department at ETH Zurich and Head of Machine Learning Research and AI at Spiden. He has been a member of the technical staff at Bell Labs and a Machine Learning Research Scientist at Amazon. Fernando has been a visiting professor at Princeton University under a Marie Curie Fellowship and an associate professor at University Carlos III in Madrid. He held positions at the Gatsby Unit (London), Max Planck Institute for Biological Cybernetics (Tuebingen), and BioWulf Technologies (New York). Fernando Perez-Cruz has served as Chief Data Scientist at the SDSC from 2018 to 2023, and Deputy Executive Director of the SDSC from 2022 to 2023
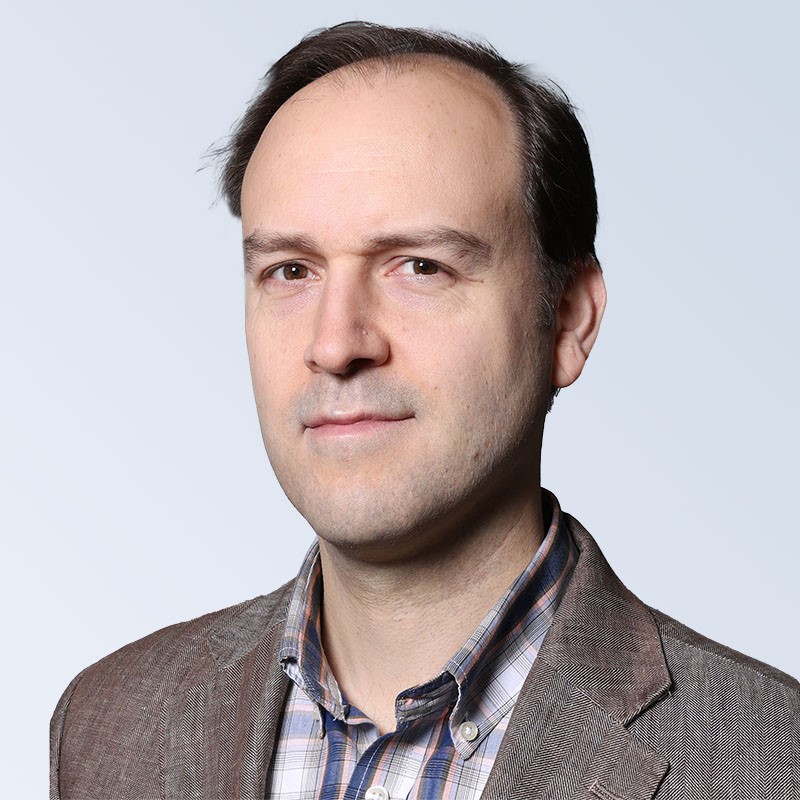
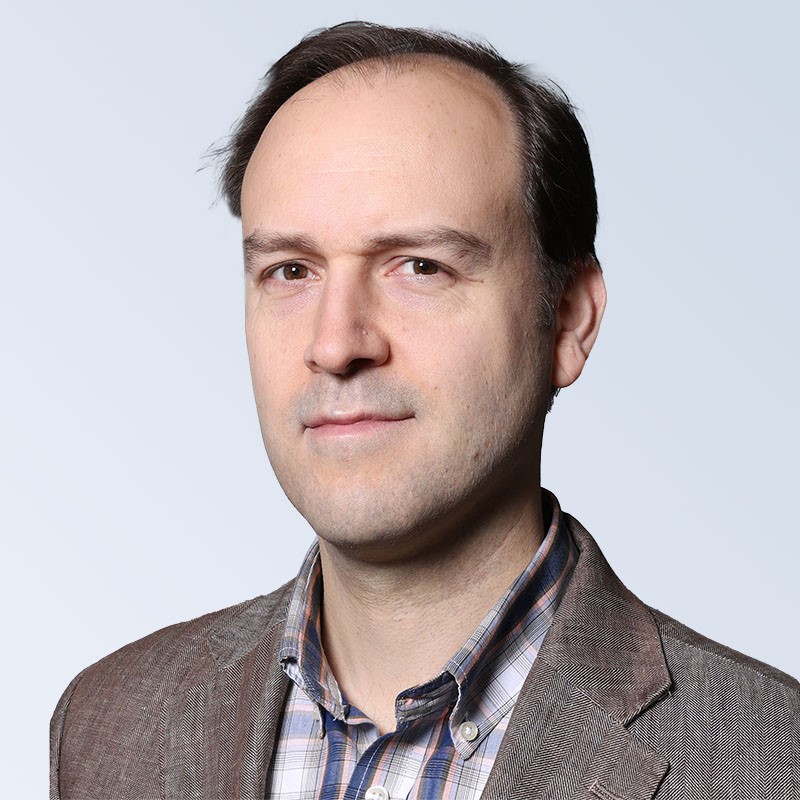
Guillaume Obozinski graduated with a PhD in Statistics from UC Berkeley in 2009. He did his postdoc and held until 2012 a researcher position in the Willow and Sierra teams at INRIA and Ecole Normale Supérieure in Paris. He was then Research Faculty at Ecole des Ponts ParisTech until 2018. Guillaume has broad interests in statistics and machine learning and worked over time on sparse modeling, optimization for large scale learning, graphical models, relational learning and semantic embeddings, with applications in various domains from computational biology to computer vision.
PI | Partners:
Paul Scherrer Institute:
- Prof. El Haddad, Imad
- Dr. Daellenbach, Kaspar Rudolf
- Dr. Upadhyay Abhishek Kumar
- Wu Jimeng
- Dr. Chen Ying
description
Problem:
AURORA will link for the first time single PM emission sources and formation processes to health effects. A data-science-based ensemble model will be developed combining both geostatistical data and CTM outputs to produce source-specific, fine-resolution, PM concentration fields for large scales and long-terms, suitable for assessing human exposure to source-specific PM.
Impact:
Aurora has tangible impacts on air quality, public health, and the economy. The identification of the sources of harmful components in PM air pollution is the Holy Grail for atmospheric scientists, air quality modelers, and epidemiologists. Aurora will enhance the fundamental understanding of key emissions and processes controlling PM concentrations, chemical composition and noxiousness, and their sensitivity to changes in energy and land use that cities are currently experiencing.
Proposed approach:
None of the existing approaches fulfills the requirements to relate PM sources and complex formation processes to its health effects. This is an opportune moment for atmospheric chemists, numerical modelers, data scientists, exposure scientists, and epidemiologists to work together to develop such an approach.
The goal is set to go beyond linear dimension reduction techniques currently used in the field to better represent the chemical complexity of the atmospheric system and the interdependence between sources.
We will implement new techniques to efficiently explore the parameter space of our numerical model and optimize them using field observations. The developed machine learning techniques will help to:
- generate fine-resolution PM concentration fields from different sources and processes using coarse resolution CTM outputs and geostatistical data, and
- infer relationships between source-specific PM concentrations and mortality or diseases.
If the model performance is satisfactory, we could predict the PM sources that contribute most to lung diseases and mortality in Europe.
Presentation
Gallery
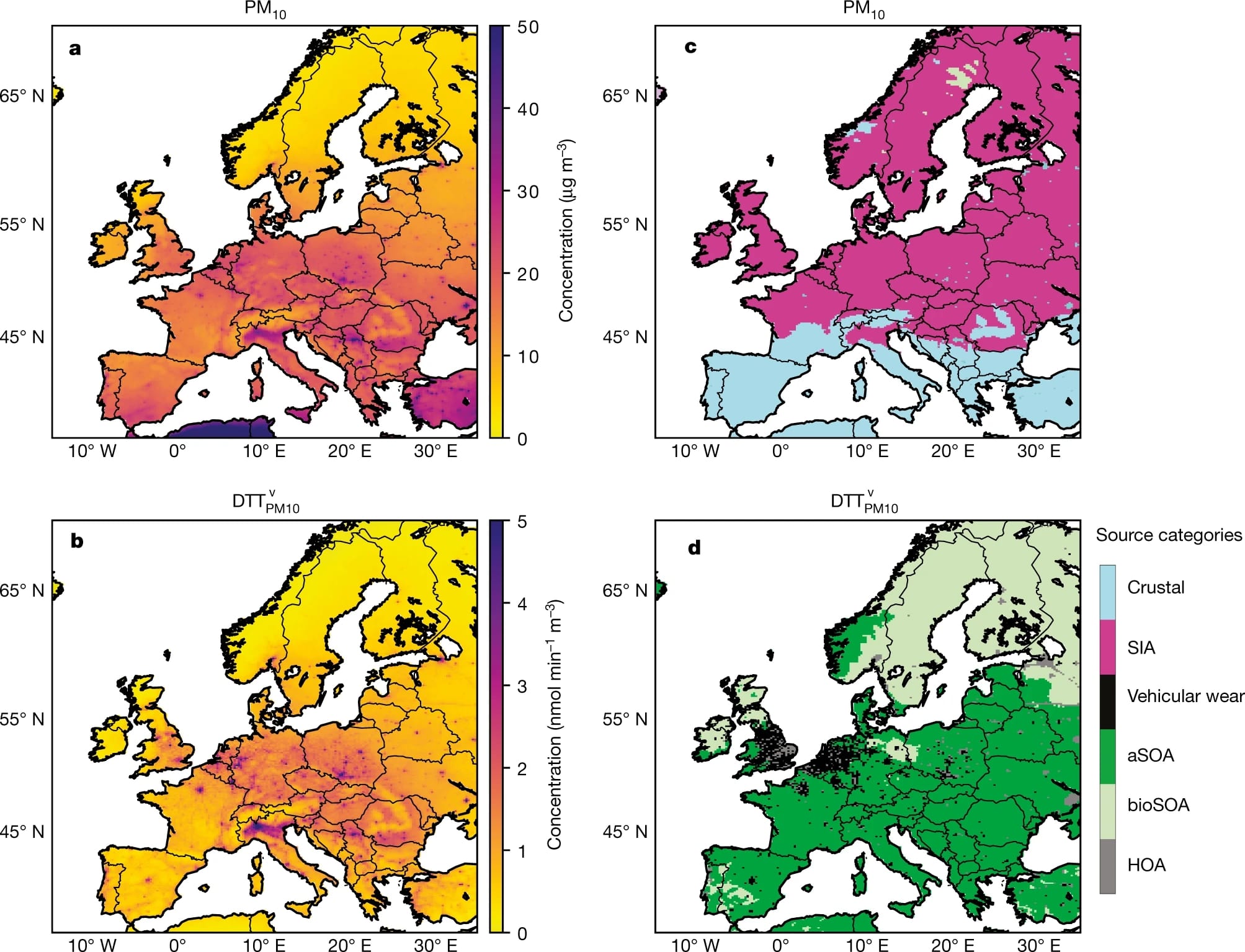
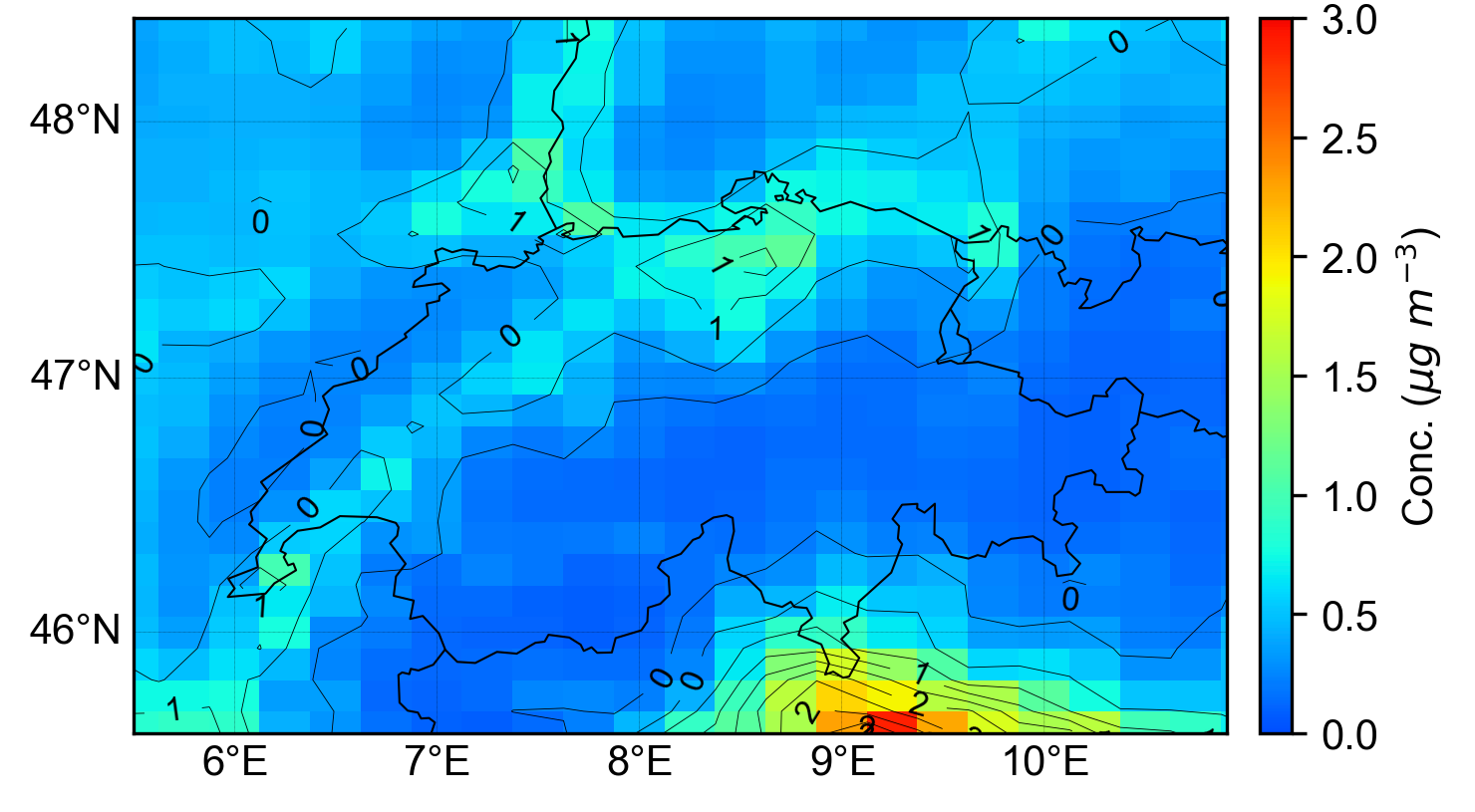
Annexe
Additionnal resources
Bibliography
- Daellenbach, Kaspar R., et al. “Sources of particulate-matter air pollution and its oxidative potential in Europe.” Nature587.7834 (2020): 414-419.
- Chen, Gang, et al. “European Aerosol Phenomenology-8: Harmonised Source Apportionment of Organic Aerosol using 22 Year-long ACSM/AMS Datasets.” Environment International (2022): 107325.
- Daellenbach, K. R.; Bozzetti, C.; Křepelová, A.; Canonaco, F.; Wolf, R.; Zotter, P.; Fermo, P.; Crippa, M.; Slowik, J. G.; Sosedova, Y.; Zhang, Y.; Huang, R. J.; Poulain, L.; Szidat, S.; Baltensperger, U.; El Haddad, I.; Prévôt, A. S. H., “Characterization and source apportionment of organic aerosol using offline aerosol mass spectrometry”. Atmos. Meas. Tech. 2016, 9 (1), 23-39.
Publications
Related Pages
More projects
CLIMIS4AVAL
News
Latest news
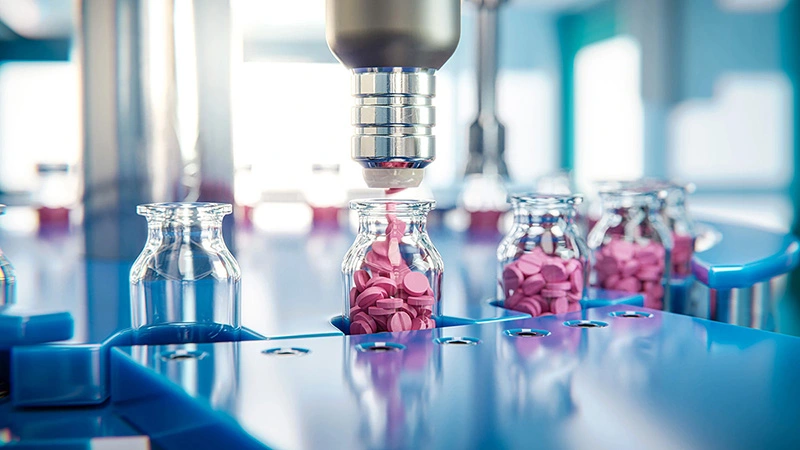
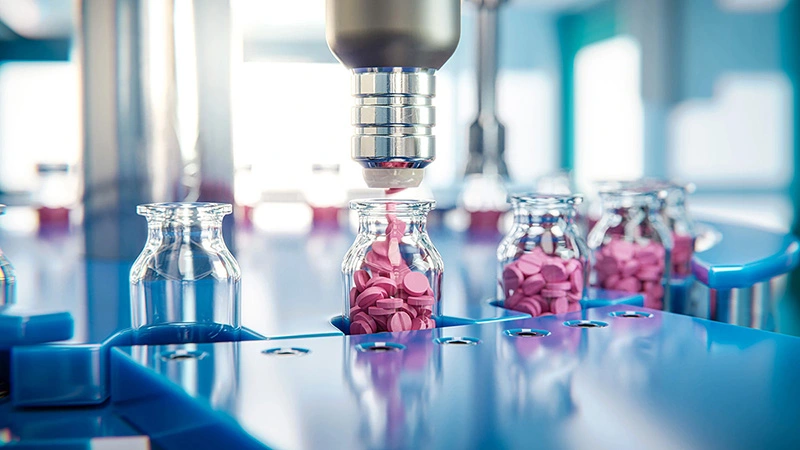
The Promise of AI in Pharmaceutical Manufacturing
The Promise of AI in Pharmaceutical Manufacturing
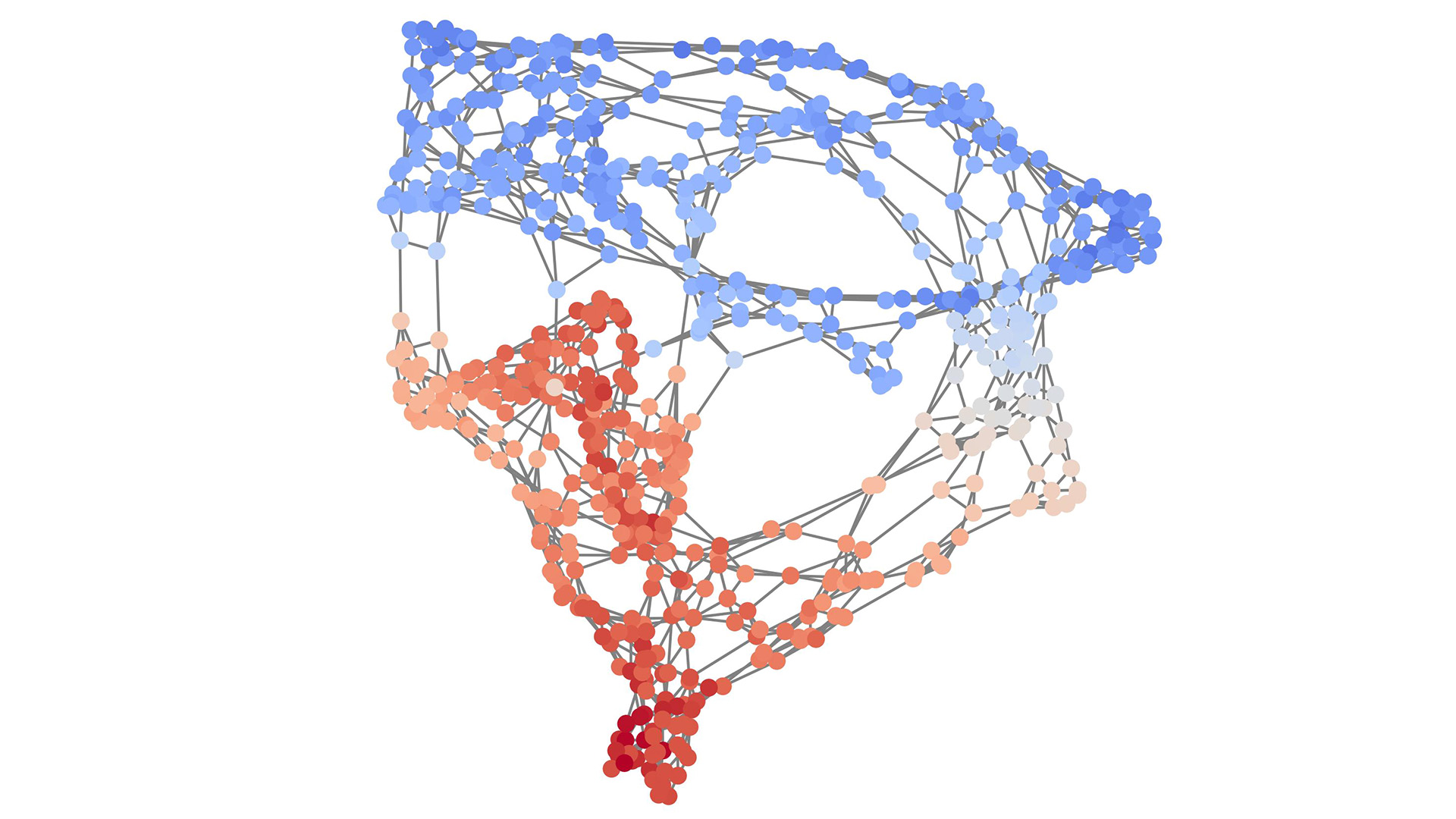
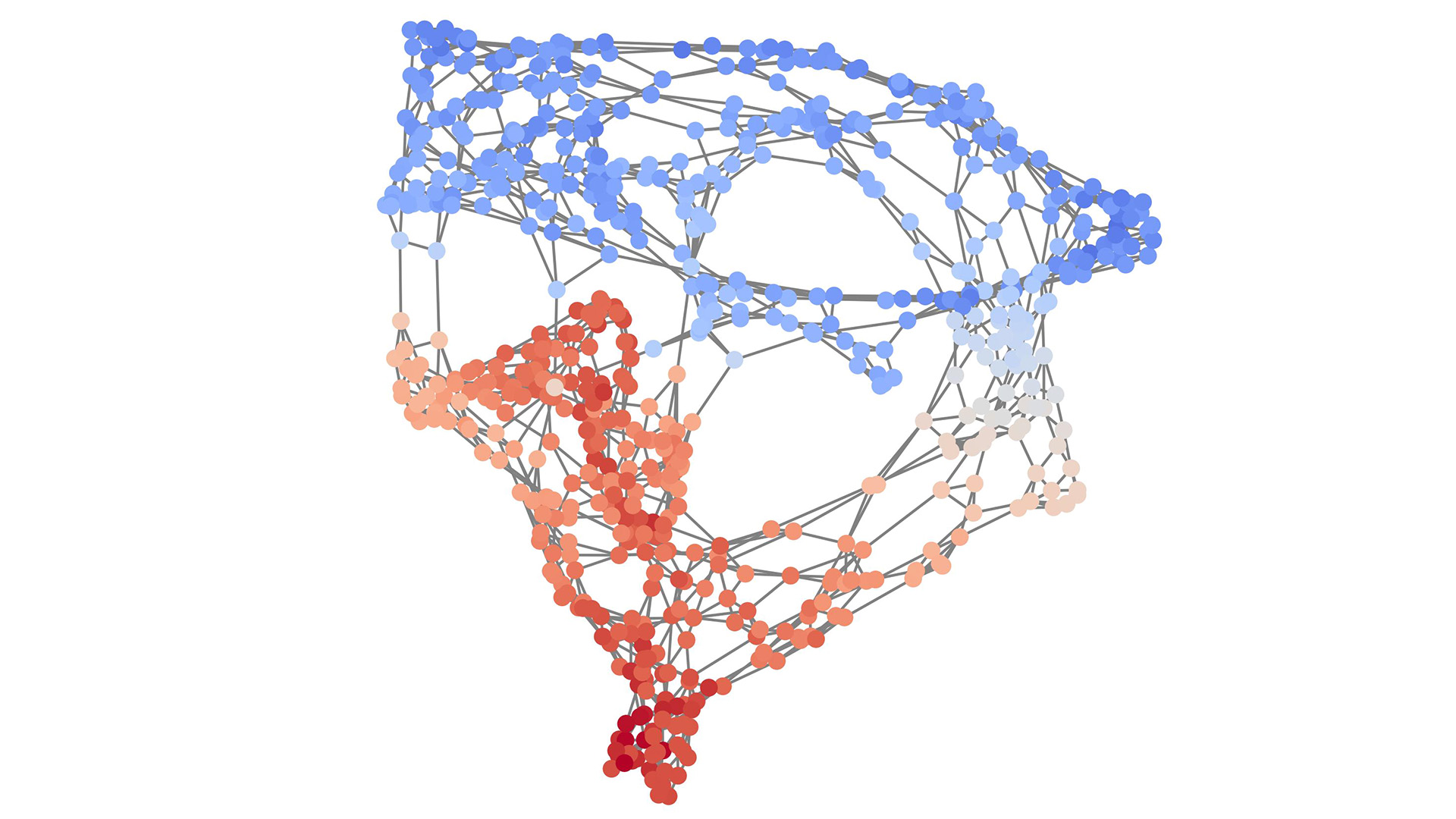
Efficient and scalable graph generation through iterative local expansion
Efficient and scalable graph generation through iterative local expansion


RAvaFcast | Automating regional avalanche danger prediction in Switzerland
RAvaFcast | Automating regional avalanche danger prediction in Switzerland
Contact us
Let’s talk Data Science
Do you need our services or expertise?
Contact us for your next Data Science project!