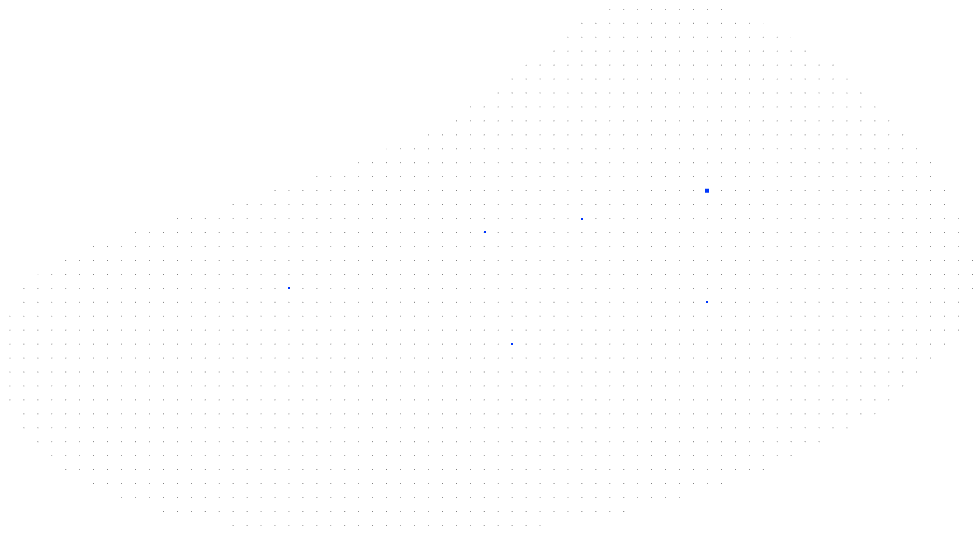
DATSSFLOW
Data Science and Mass Movement Seismology: Towards the Next Generation of Debris Flow Warning
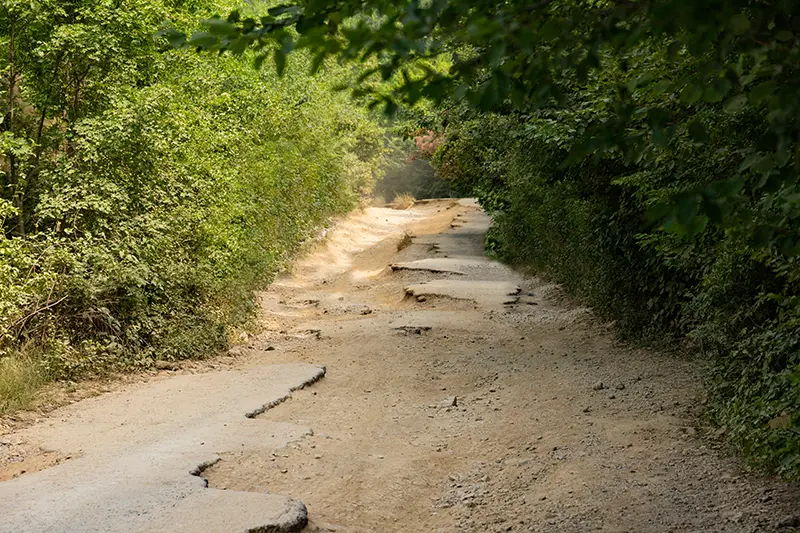
Abstract
Mobilized during heavy precipitation, debris flows are sediment-water mixtures moving down steep mountain torrents in an uncontrolled fashion. The triggering mechanisms of this severe natural hazard are difficult to quantify, because sediment mobilization occurs in high catchment areas with limited access. In this project we propose to monitor debris flow formation and preparatory mass
movements like rockfalls and landslides using seismic data. To tackle the longstanding challenge of automatic and reliable mass movement detection with real-time seismic data, we propose innovative machine learning approaches. A specific goal is to develop transferable algorithms to detect debris flows at arbitrary sites and thus to exploit existing seismic infrastructure in Alpine terrain.
The project focuses on seismic records from Illgraben, an active debris flow torrent in Switzerland. Using a four-year seismic record we aim to monitor sediment production and deposition, which precondition the catchment for debris flow occurrence. This will allow for a better understanding of debris flow triggering by precipitation, snow melt, temperature variations and other environmental
forcings. The next step of transferable debris flow detection requires unsupervised machine learning algorithms and thus significant improvement to existing supervised approaches.
Debris flow hazard is expected to grow during future climate change and as a result of increasing population pressure in mountain regions. Our project addresses the need to warn against this threat. The possibility to detect events remotely via seismic techniques that do not require instrumentation in poorly accessible terrain aims to significantly increase warning time and thus our capabilities to protect human lives and infrastructure.
People
Collaborators
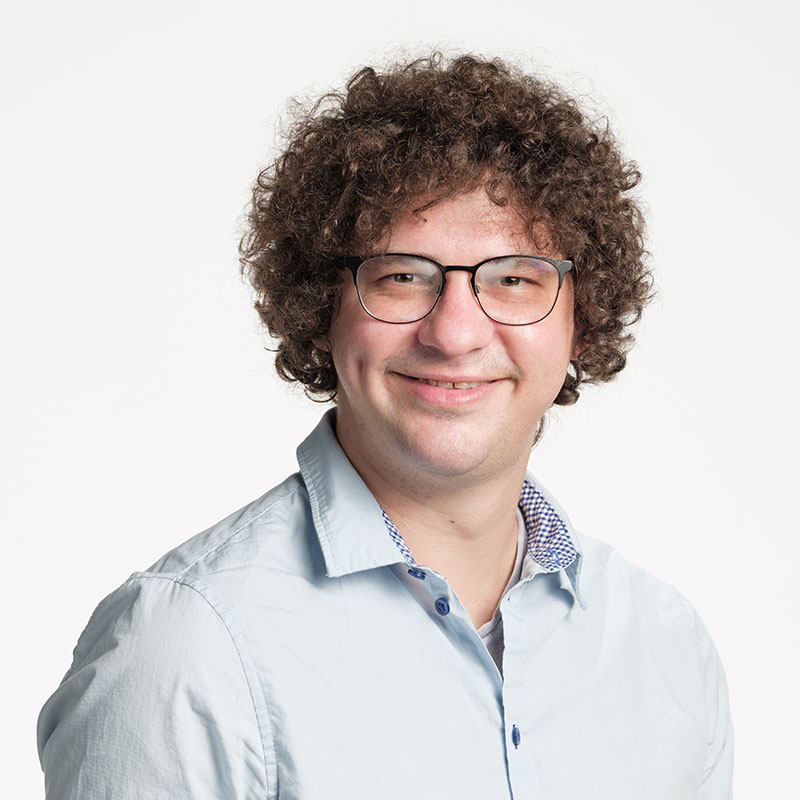
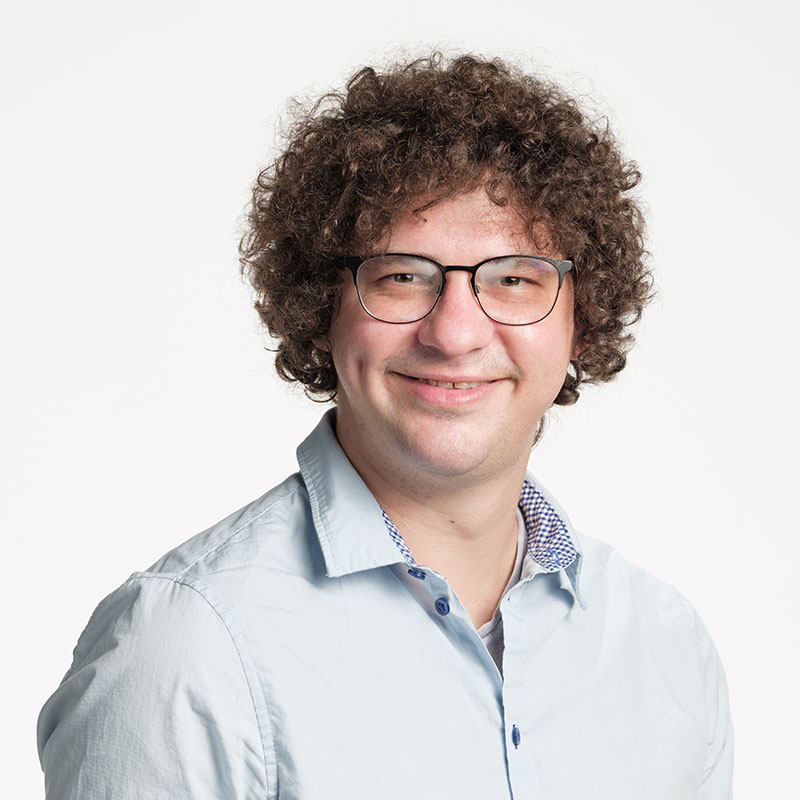
Francois received his PhD in December 2018 from Stellenbosch University (SU) in South Africa where he focused on improving inference algorithms for probabilistic graphical models. Before joining the SDSC, Francois worked as a senior lecturer at the Department of Statistics and Actuarial Science at SU. His research interests include probabilistic graphical models, time series forecasting, causal inference and discovery, extreme value theory, and machine learning in general.
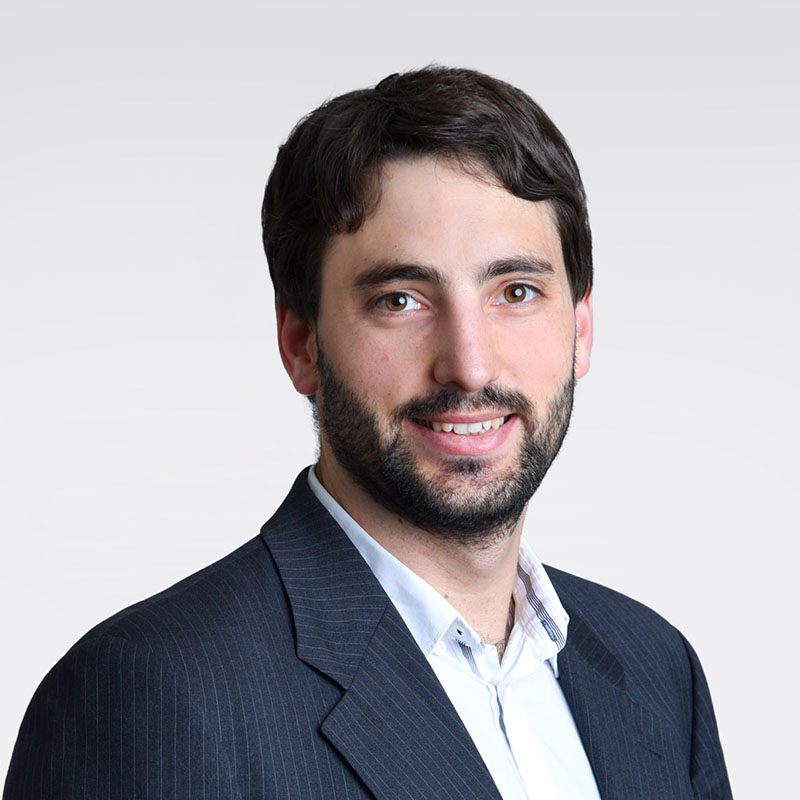
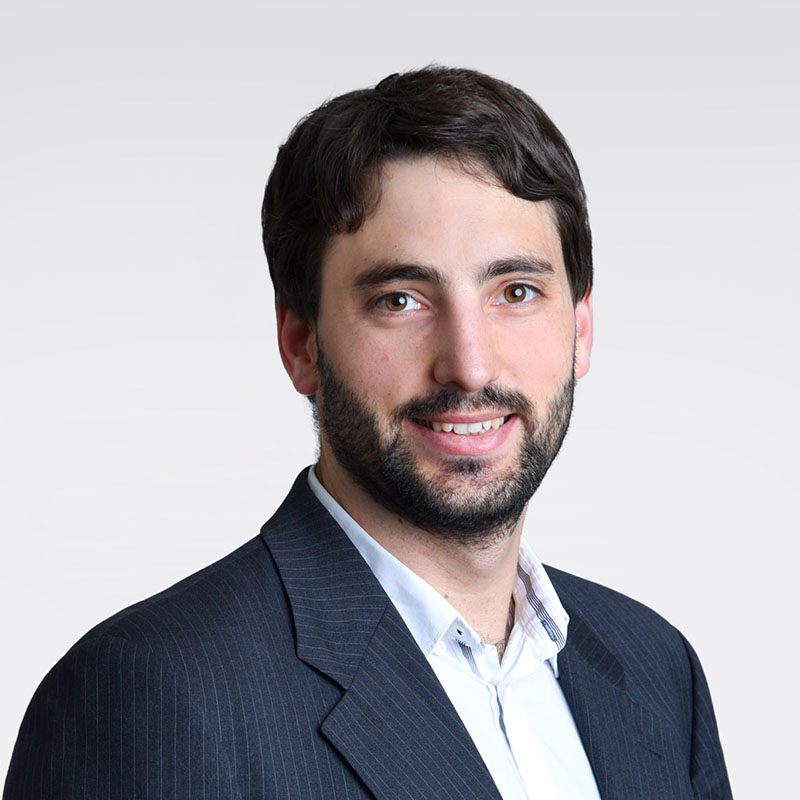
After finishing his Master in electrical engineering at the Ecole Fédérale de Lausanne (EPFL), Nathanaël worked as a researcher in the Acoustic Research Institute (ARI) in Vienna. In 2013, he returned to EPFL for a PhD, where he specialized himself in different fields of data science: signal processing, machine learning, graph theory and optimization. Furthermore, he created two open source libraries for optimization (UNLocBoX) and graph signal processing (GSPBOX). Since 2017, Nathanaël Perraudin is a Research Data Scientist at the Swiss Data Science Center in the ETH Zurich. He focuses on different aspects of deep learning in the area of generative models (VAE and GAN), recursive architectures and convolutional neural network for irregular domains. Outside office hours, he is passionate by tango dancing, tandem bike touring, skiing and rock climbing.
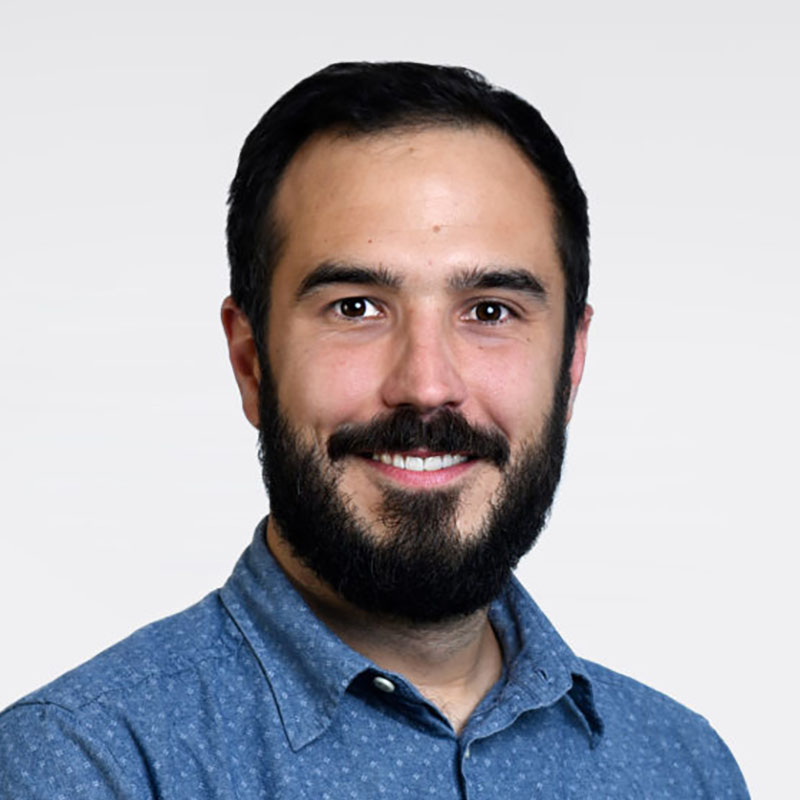
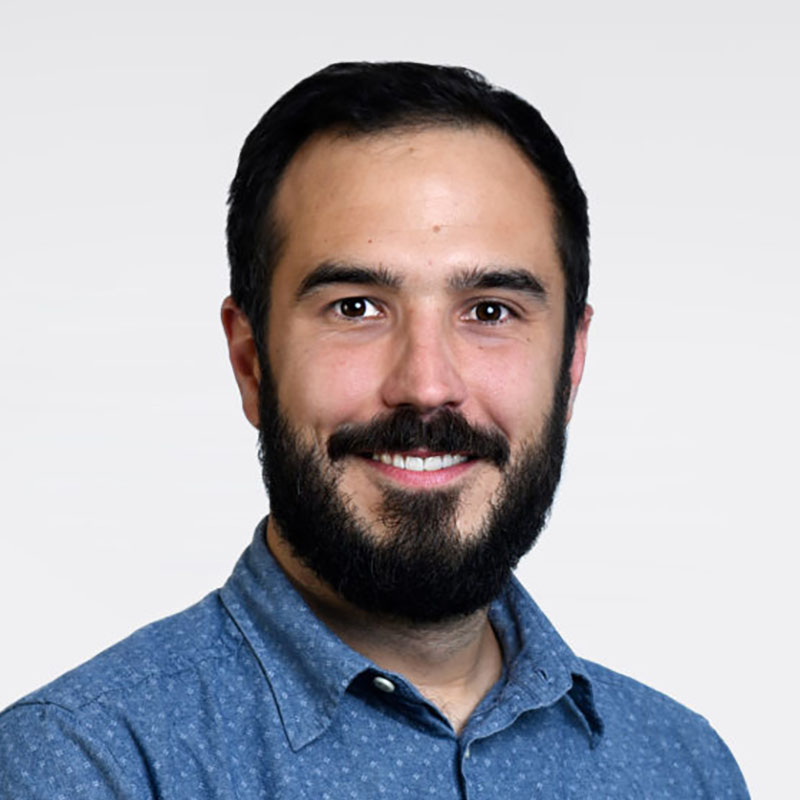
Michele received a Ph.D. in Environmental Sciences from the University of Lausanne (Switzerland) in 2013. He was then a visiting postdoc in the CALVIN group, Institute of Perception, Action and Behaviour of the School of Informatics at the University of Edinburgh, Scotland (2014-2016). He then joined the Multimodal Remote Sensing and the Geocomputation groups at the Geography department of the University of Zurich, Switzerland (2016-2017). His main research activities were at the interface of computer vision, machine and deep learning for the extraction of information from aerial photos, satellite optical images and geospatial data in general.


Mathieu Salzmann is the Deputy Chief Data Scientist of the Swiss Data Science Center and a Senior Scientist and Lecturer at EPFL. He received his PhD from EPFL in 2009 and, between 2009 and 2015, has held post-doctoral and researcher positions at UC Berkeley, TTI-Chicago, and NICTA and the Australian National University. From May 2020 to February 2024, he was also a part-time Senior GNC Engineer at ClearSpace. Mathieu Salzmann has broad interests in machine learning and deep learning, with a particular focus on computer vision. He has published over 100 articles at top-tier peer-reviewed machine learning and computer vision venues and is a strong believer in collaborative work.
description
Motivation
The main goal of the project is the development of a machine learning model capable of detecting debris-flows from seismic data. A major motivating factor is that such models can be used in the design (or aid) of early-warning systems, helping to protect human lives and infrastructure. Furthermore, since seismic coverage is growing at an accelerated rate, these models can be applied to a variety of locations without the need for installing additional infrastructure.
Proposed Approach
A random forest has been shown to be successful in predicting debris-flows from seismic data in the Illgraben region, however this model does not generalize well to data from other seismic networks. Our approach is to train several machine-learning based debris-flow detectors to the Illgraben data and utilize domain adaption techniques to improve generalization to other seismic networks. For this purpose we are investigating ideas from supervised, positive-unlabeled, semi-supervised and unsupervised learning,
Impact
With the advent new technologies, seismometer coverage is growing at an accelerated pace. The proposed project breaks the ground for turning already installed seismometers into mass movement detectors. While we focus on the analysis of seismic data, future extensions with real-time data of meteorological conditions influencing debris flow formation will improve detection accuracy.
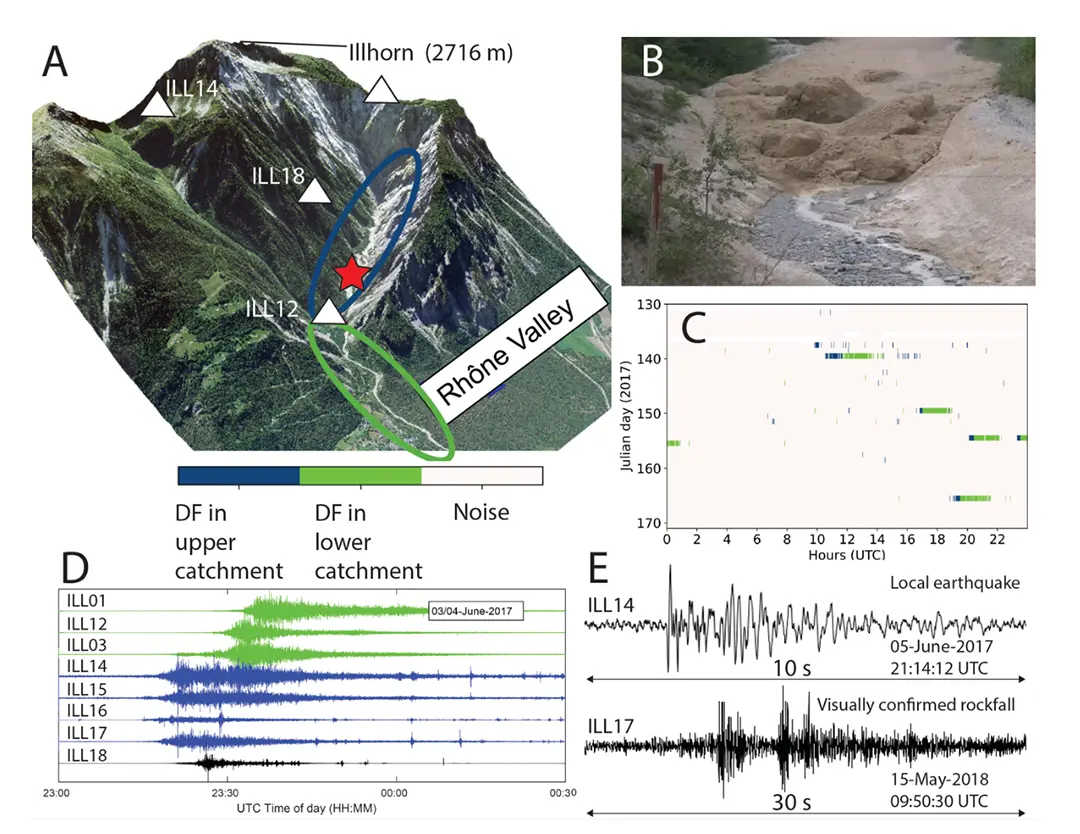
Presentation
Gallery
Annexe
Publications
- Paitz, P., Chmiel, M., Husmann, L., Volpi, M., Kamper, F., and Walter, F.: Towards an unsupervised generic seismic detector for hazardous mass-movements: a data-driven approach, EGU General Assembly 2023, Vienna, Austria, 24–28 Apr 2023, EGU23-6300, https://doi.org/10.5194/egusphere-egu23-6300, 2023.
- Paitz, P., Chmiel, M., Husmann, L., Volpi, M., Kamper, F., and Walter, F.: Generic seismic mass-movement detection leveraging unsupervised statistical learning methods. Machine Learning in Geo-, Ocean and Space Sciences. IUGG General Assembly 2023. Berlin, Germany, 11-20 July 2023, IUGG23-0742.
Additional resources
Bibliography
- Coussot, P., & Meunier, M. (1996). Recognition, classification and mechanical description of debris flows. Earth-Science Reviews, 40(3-4), 209-227.
- Bahavar, M., Allstadt, K. E., Van Fossen, M., Malone, S. D., & Trabant, C. (2019). Exotic seismic events catalog (ESEC) data product. Seismological Research Letters, 90(3), 1355-1363
- Wenner, M., Hibert, C., van Herwijnen, A., Meier, L., and Walter, F.: Near-real-time automated classification of seismic signals of slope failures with continuous random forests, Natural Hazards and Earth System Sciences, 21, 339–361, 2021
- Chmiel, M., Walter, F., Wenner, M., Zhang, Z., McArdell, B. W., & Hibert, C. (2021). Machine Learning
improves debris flow warning. Geophysical Research Letters, 48(3), e2020GL090874.
Publications
Related Pages
More projects
ML-L3DNDT
BioDetect
News
Latest news


Smartair | An active learning algorithm for real-time acquisition and regression of flow field data
Smartair | An active learning algorithm for real-time acquisition and regression of flow field data
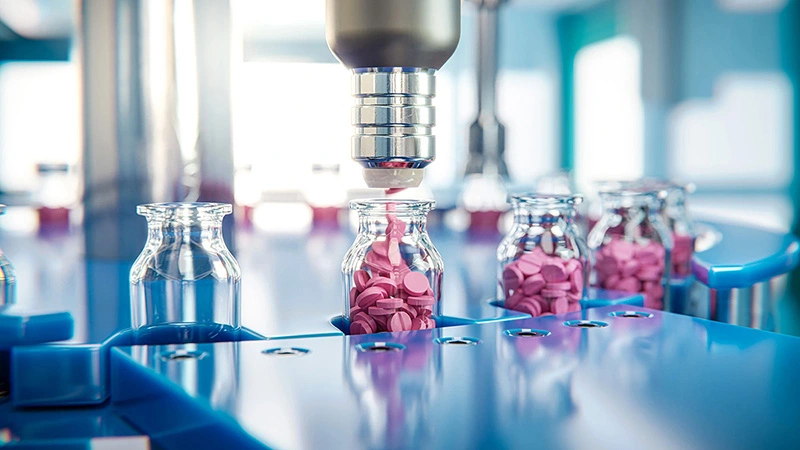
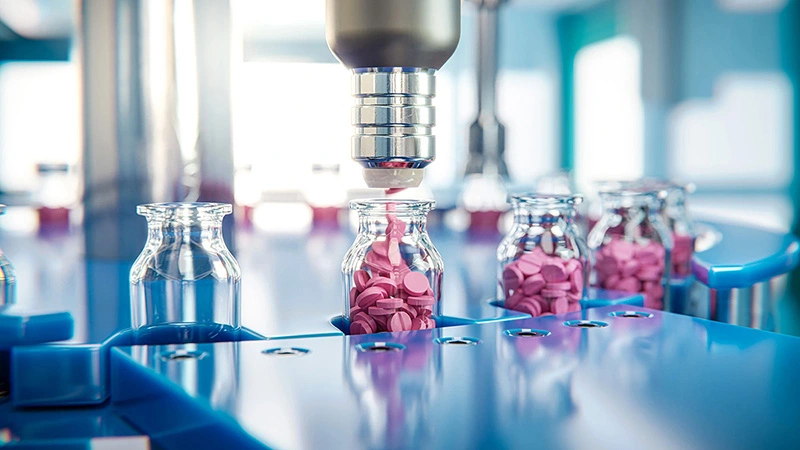
The Promise of AI in Pharmaceutical Manufacturing
The Promise of AI in Pharmaceutical Manufacturing


Efficient and scalable graph generation through iterative local expansion
Efficient and scalable graph generation through iterative local expansion
Contact us
Let’s talk Data Science
Do you need our services or expertise?
Contact us for your next Data Science project!