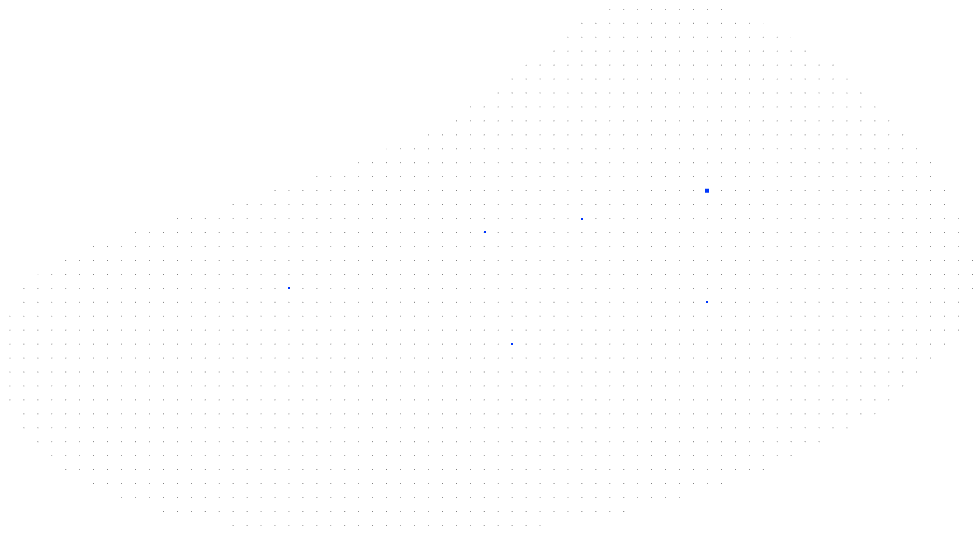
EXPECT
EXtending the PrEdiCTability of the Atmosphere over Europe
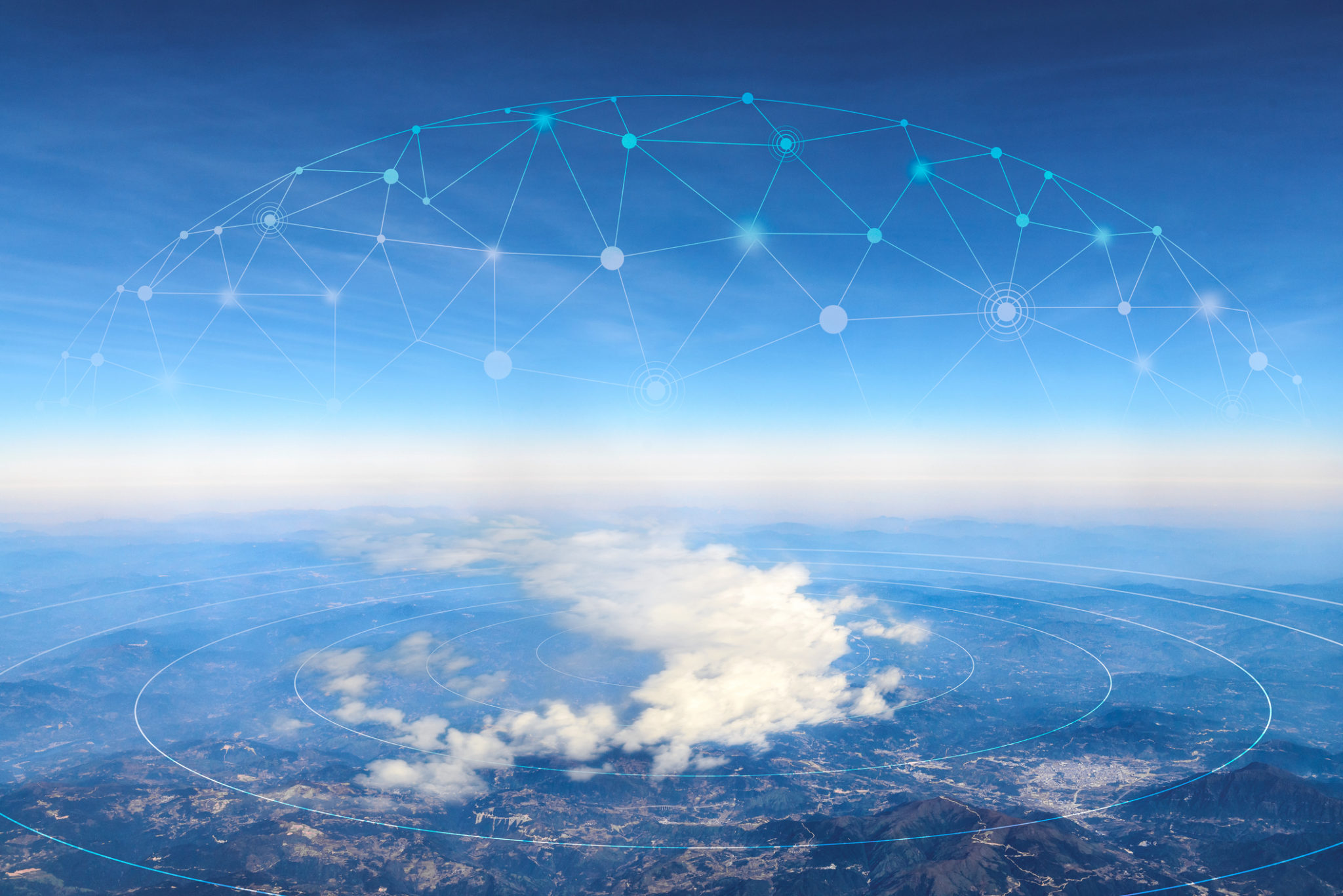
Abstract
Currently available weather forecasts over Europe tend to have skill up to about one week, although theoretical estimates indicate a theoretical limit of about 3 weeks (Buizza et al., 2015; Domeisen et al., 2018a). After that, predictability often decreases sharply. However, longer term forecasts are possible if information is available that provides an increased probability of a long-lasting event, or an influence from a region that has a slower variability – such as the ocean (e.g. Duchez et al., 2016) – or longer predictability – such as the tropics (e.g. Greatbatch et al., 2015; Scaife et al., 2017; Wulff et al., 2017). The upper atmosphere, i.e. the stratosphere at about 12 – 50km above the Earth’s surface, is such a region that provides increased predictability to Europe after extreme stratospheric events, so-called Sudden Stratospheric Warming (SSW) events (Scaife et al., 2016). These events can provide skill over Europe for up to several weeks to months (Sigmond et al., 2013), with persistently colder than usual weather over Northern and central Europe. One of these events occurred in February 2018 and led to persistent cold weather in Europe in late February and early March after an otherwise mild winter.
SSW events themselves are however only possible to predict several days in advance. An extended prediction of SSW events would therefore significantly benefit forecasts at the surface. While the stratosphere is not the only region with predictive potential for Europe, most other predictors exhibit a pathway through the stratosphere, such as e.g. predictability arising from the tropics (e.g. Domeisen et al., 2015; Butler et al., 2016). It is therefore crucial to understand the predictability of the stratosphere itself. However, SSW events are difficult to classify, and indeed there exists a range of methods and classifications. This project aims to find an automated classification of these events in addition to an automated analysis of predictability over Europe. The main objectives of this project are described here:
- Only recently have predictions on sub-seasonal to seasonal timescales, i.e. weeks to months, become publicly available. This project aims at extracting novel insights from this data using data science tools.
- A first step will be an improved classification of stratospheric events, allowing for a flexible definition that includes the predictability aspects of these events.
- In a second step, this project aims to classify remote predictors of long-term weather variability. In particular, known predictors for stratospheric and tropospheric variability will be evaluated using data science methods and possible new predictors will be identified. This knowledge is expected to lead to an improved predictability of the weather over Europe on weekly to monthly timescales.
People
Collaborators


Raphaël graduated in 2014 with an engineering degree from l’Ecole des Mines de Paris and holds since 2018 a Ph.D. in Statistics from l’Ecole Polytechnique Fédérale de Lausanne. Before joining the Swiss Data Science Center as senior data scientist, Raphaël was post-doctoral researcher at the Institute of Mathematics at EPFL working on quantitative risk modelling for natural hazards using extreme value theory. His research interests lie at the boundary of statistics and environmental sciences with a special focus on the analysis of spatio-temporal data.
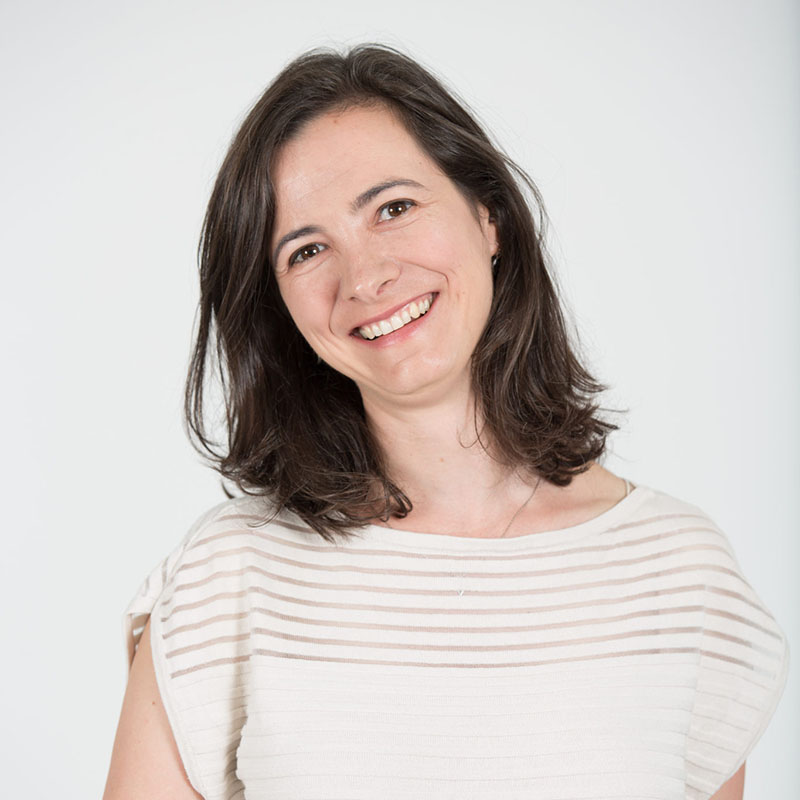
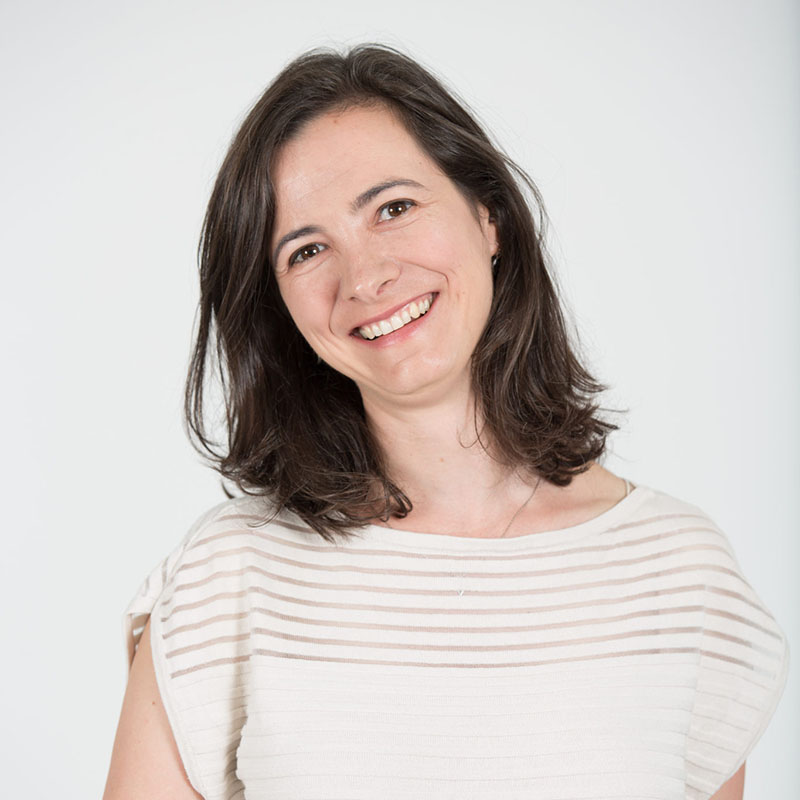
Eniko joined SDSC as a senior data scientist in September 2017. Previously, she was a postdoctoral researcher at the Courant Institute of Mathematical Sciences, New York University, working on machine learning for dynamical systems and climate science. She obtained her PhD in Computer Science from the University of Geneva, Switzerland (2011), her MSc from Telecom Bretagne, France (2006) and her BSc from the University of Timisoara, Romania (2005). Broadly she is interested in machine learning for nonlinear phenomena and high-dimensional data, and more recently she has been working on using machine learning approaches to advance our understanding of the climate.
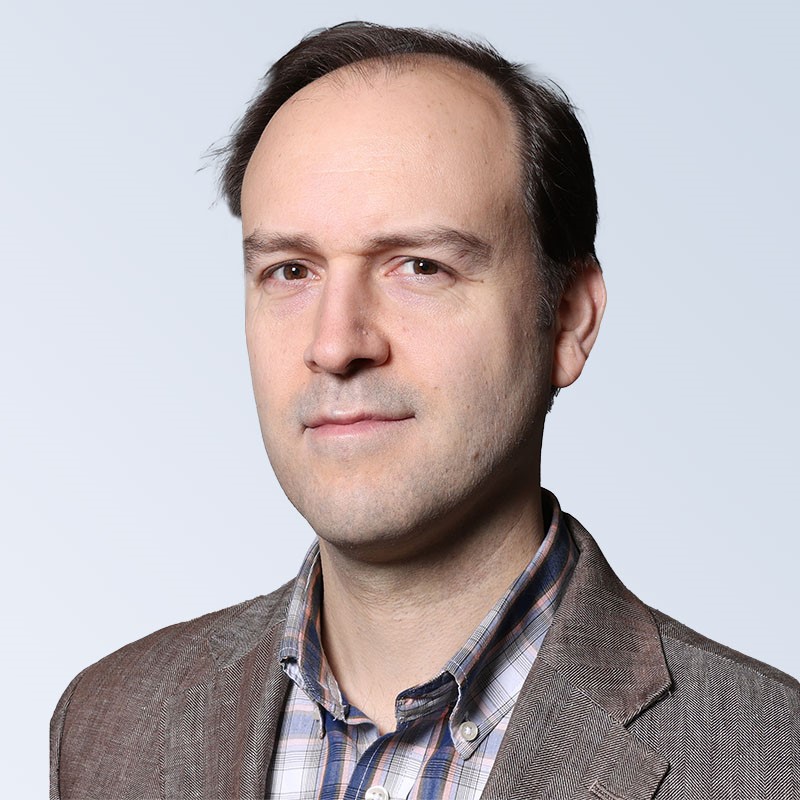
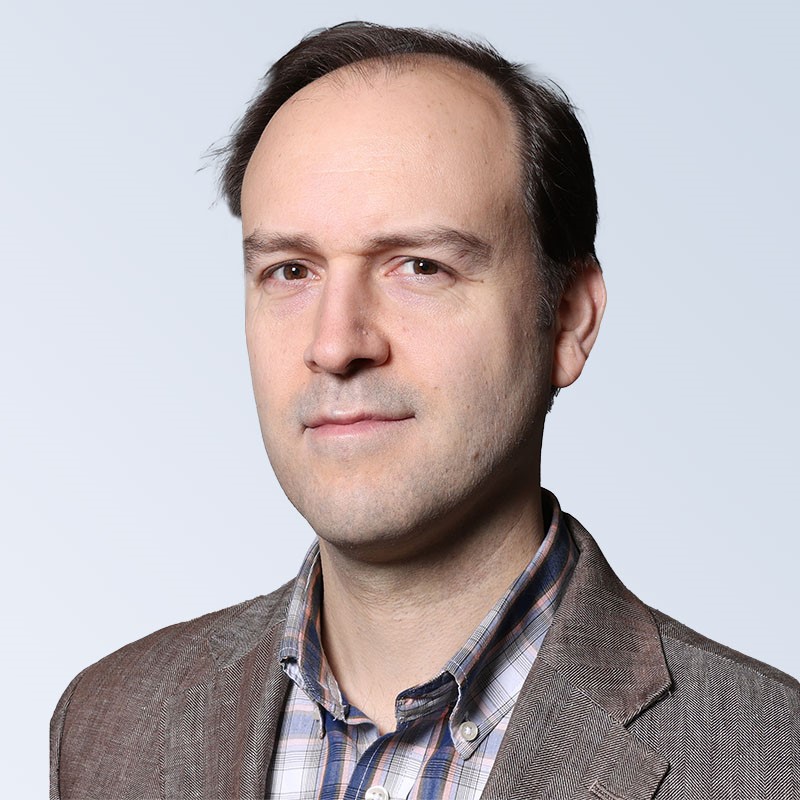
Guillaume Obozinski graduated with a PhD in Statistics from UC Berkeley in 2009. He did his postdoc and held until 2012 a researcher position in the Willow and Sierra teams at INRIA and Ecole Normale Supérieure in Paris. He was then Research Faculty at Ecole des Ponts ParisTech until 2018. Guillaume has broad interests in statistics and machine learning and worked over time on sparse modeling, optimization for large scale learning, graphical models, relational learning and semantic embeddings, with applications in various domains from computational biology to computer vision.
PI | Partners:
description
Problem:
From a climatological point of view, winter is characterized by the apparition in the stratosphere of a vortex of strong winds over the polar regions; this vortex is generally centered at the pole and have with a circular shape.
In average once every two years, sudden and rapid warmings of the stratosphere disturb the vortex causing displacements and deformations. The impact of such events, known as Sudden Stratospheric Warmings (SSW), are not limited to the stratosphere but also strongly influence the troposphere, i.e., weather at the sea level, during two to three months after an occurrence.
Thus a good prediction of SSW events would enable the production of more accurate weather forecasts at a monthly time scale. However, the stratosphere is a complex environment: the different states of the polar vortex and their relations with SSW events are still not well understood.
Solution
- Use Data science methodologies to better characterize the relationship between SSWs and the different states of the polar vortex.
- Improve the prediction of disturbed state of the stratosphere by combining data driven prediction algorithms with the knowledge extracted from the first point.
Impact:
- Contribute to a better understanding of the coupling between stratosphere and troposphere.
- Improve the predictability of weather at a seasonal time scale. Accurate seasonal forecasts are particularly important for agriculture planning, public health and natural resources management.
Presentation
Gallery

Annexe
Publications
- Raphaël de Fondeville, Zheng Wu, Enikő Székely, Guillaume Obozinski, and Daniela I. V. Domeisen Improved extended-range prediction of persistent stratospheric perturbations using machine learning, Weather and Climate Dynamics, 4, 287–307, 2023.
- Zheng Wu, Bernat Jiménez-Esteve, Raphaël de Fondeville, Enikő Székely, Guillaume Obozinski, William T. Ball, and Daniela I. V. Domeisen. Emergence of representative signals for sudden stratospheric warmings beyond current predictable lead times. Weather and Climate Dynamics, 2, 841–865, 2021.
Additional resources
Bibliography
- Baldwin, M. P. and Dunkerton, T. J. (2001). Stratospheric Harbingers of Anomalous Weather Regimes. Science, 294(5542):581-584.
- Butler, A. H., Seidel, D. J., Hardiman, S. C., Butchart, N., Birner, T., & Match, A. (2015). Defining sudden stratospheric warming. Bull. Amer. Meteor. Soc., 1–16.
- Blume, C., Matthes, K., and Horenko, I. (2012). Supervised Learning Approaches to Classify Sudden Stratospheric Warming Wvents. Journal of the Atmospheric Sciences, 69(6):1824-1840.
- Coughlin, K. and Gray, L. J. (2009). A Continuum of Sudden Stratospheric Warmings. Journal of the Atmospheric Sciences, 66(2):531-540.
Publications
Related Pages
More projects
ML-L3DNDT
BioDetect
News
Latest news
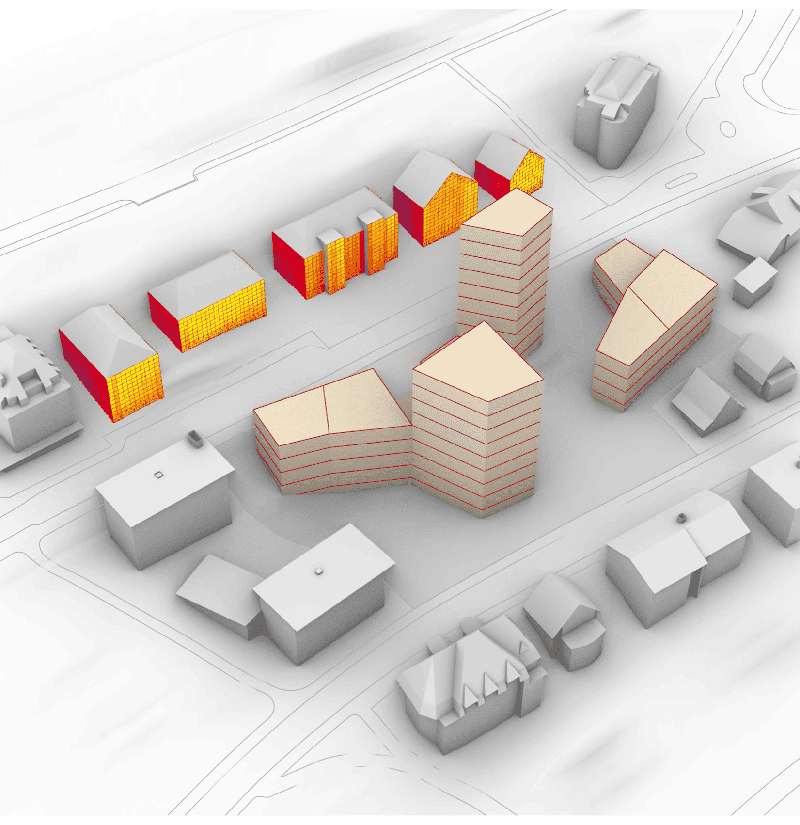
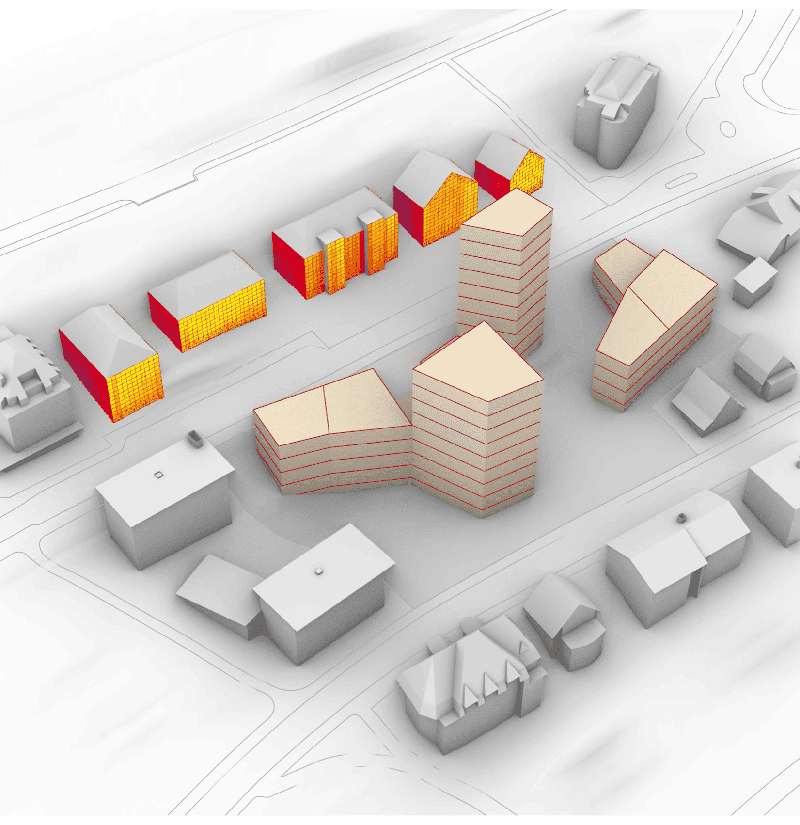
AIXD | Generative AI toolbox for architects and engineers
AIXD | Generative AI toolbox for architects and engineers


Smartair | An active learning algorithm for real-time acquisition and regression of flow field data
Smartair | An active learning algorithm for real-time acquisition and regression of flow field data
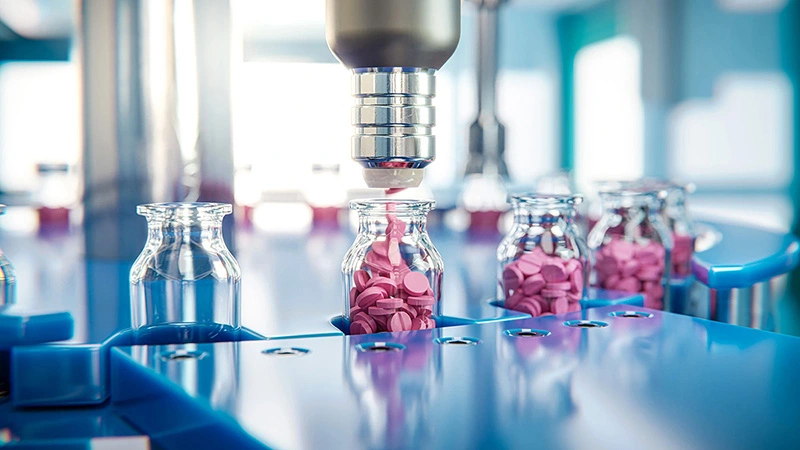
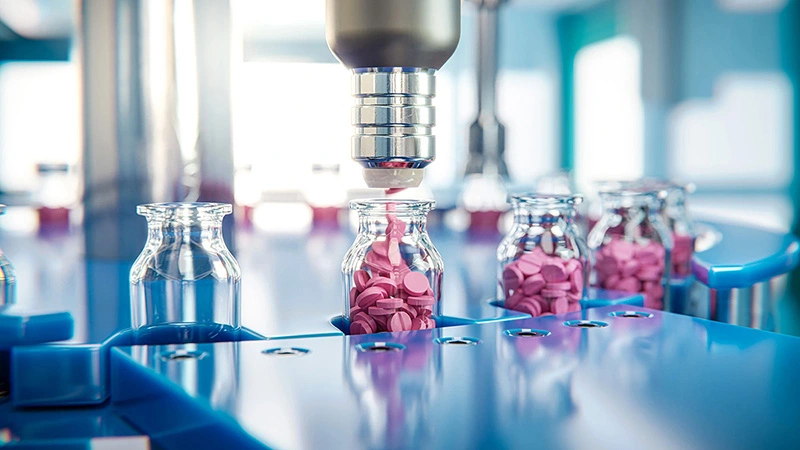
The Promise of AI in Pharmaceutical Manufacturing
The Promise of AI in Pharmaceutical Manufacturing
Contact us
Let’s talk Data Science
Do you need our services or expertise?
Contact us for your next Data Science project!