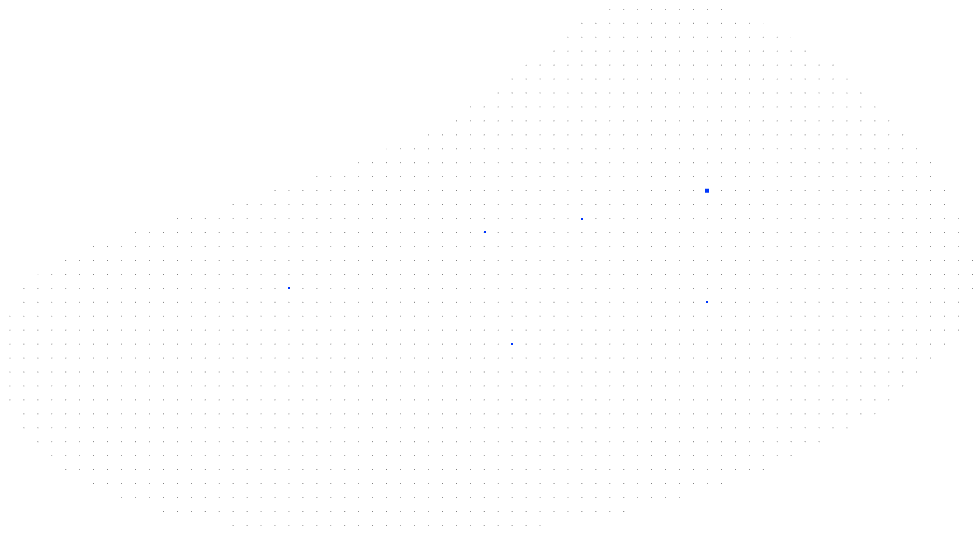
LAMP
Lensless Actinic Metrology for EUV Photomasks

Abstract
Extreme Ultraviolet (EUV) lithography is the current technology for semiconductor device manufacturing, and it will allow the industry to uphold Moore’s law in years to come. The use of the EUV wavelength (13.5 nm) allows a tremendous increase in the resolution but brings along several challenges that drive up the cost of the infrastructure for the metrology of various steps in the lithography process. At the XIL beamline of the SLS, PSI is pioneering the use of coherent diffraction imaging for the detection and the characterization of defects on EUV photomasks with the RESCAN microscope. RESCAN can detect defects as small as 50x50 nm² with an acquisition frame rate of 2 kHz. RESCAN is a demo tool, designed to inspect small samples with an area of 200x200 µm² , however the full photomask has an active area of about 100x100 mm² , which would require to collect at least 44M diffraction patterns to reconstruct the whole sample. Considering the required sampling and dynamic range required for this application, a complete diffraction data set will be of the order of 320 PB. The goal of the project is to develop efficient methods to reduce the data storage requirement, to optimize the image reconstruction procedure and avoid throughput throttling.
People
Collaborators
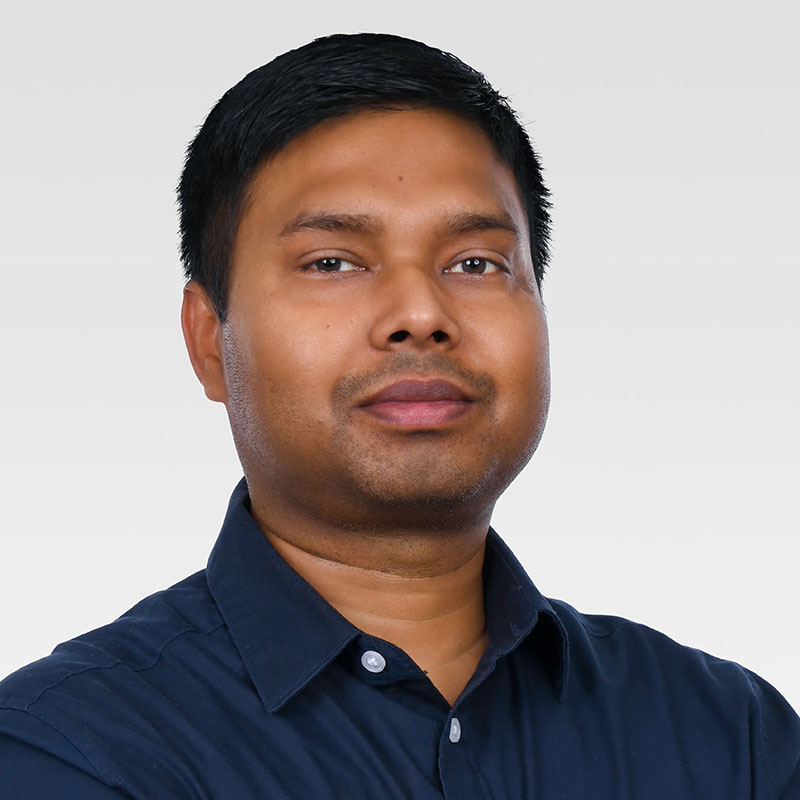
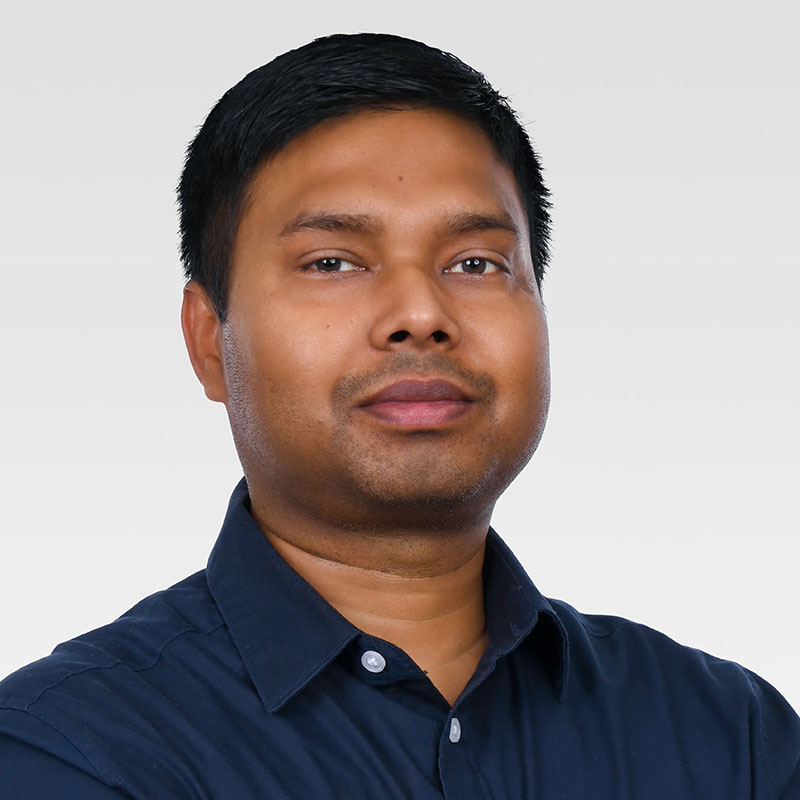
Suman pursued his doctoral studies in the Visual Artificial Intelligence Laboratory at Oxford Brookes University, United Kingdom, focusing on spatiotemporal human action localization using deep learning techniques. Suman received a Ph.D. in Computer Science and Mathematics from Oxford Brookes University in 2017. He served as a postdoctoral fellow at Oxford Brookes University between December 2017 and July 2018. Then he moved to CVL (Computer Vision Lab) at ETH Zurich, holding a postdoctoral researcher position until June 2023. Suman's research has centered around unsupervised domain adaptation (UDA) for visual scene understanding (semantic and panoptic segmentation), human behavior understanding, and vision-based biometrics (face anti-spoofing). He also worked on semi-supervised learning for semantic segmentation by leveraging self-supervised depth estimation. His internship at Disney Research Zurich involved designing deep generative models for unsupervised facial expression learning. Additionally, Suman tackled research problems in multi-task learning (MTL) by addressing two common challenges in developing multi-task models, incremental learning and task interference.
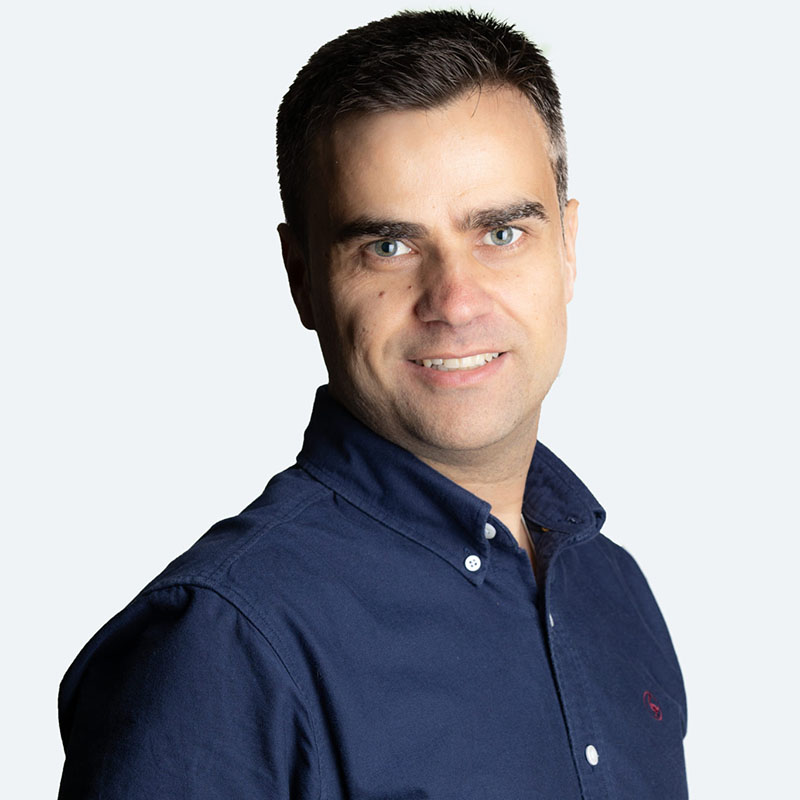
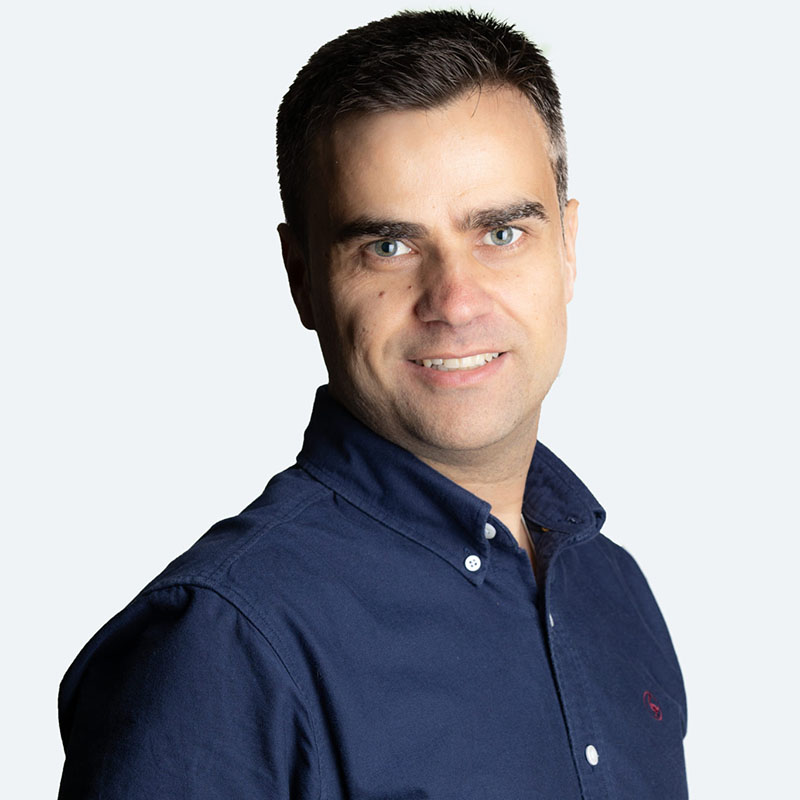
Benjamín Béjar received a PhD in Electrical Engineering from Universidad Politécnica de Madrid in 2012. He served as a postdoctoral fellow at École Polytechnique Fédérale de Lausanne until 2017, and then he moved to Johns Hopkins University where he held a Research Faculty position until Dec. 2019. His research interests lie at the intersection of signal processing and machine learning methods, and he has worked on topics such as sparse signal recovery, time-series analysis, and computer vision methods with special emphasis on biomedical applications. Since 2021, Benjamin leads the SDSC office at the Paul Scherrer Institute in Villigen.
PI | Partners:
description
Motivation
Extreme Ultraviolet (EUV) lithography is the current technology for semiconductor device manufacturing, and it allows to uphold Moore’s law in upcoming years. The use of EUV wavelength (13.5 nm) allows a tremendous increase in the resolution, but at the same time, it also increases the cost of the infrastructure for the lithography process. PSI is pioneering a lensless imaging approach - ptychography or coherent diffraction imaging (CDI) - for EUV mask inspection. A dedicated imaging tool, RESCAN, has been built and developed in recent years. With RESCAN, the main challenge is that a very large computational infrastructure is needed to achieve the desired reconstruction speed, which is highly impractical. The objective of the project is to explore alternative data processing approaches to achieve the required reconstruction speed with an affordable hardware infrastructure. The development of a cost-effective framework for mask inspection would have an enormous impact on future technology development in semiconductor device manufacturing.
Proposed Approach / Solution
We propose to optimize computational and data storage bottlenecks by designing efficient algorithms for solving the phase retrieval problem in ptychography. Ptychography is an imaging technique that allows the observation of samples at the nanometer resolution by successively probing small overlapping regions in the sample (see Fig. 1). In this project we are focusing on the inspection of semiconductor device-related samples like EUV photomasks. Usually, these samples are very well characterized and we can leverage the abundant amount of prior information in our possession to drive the image reconstruction process.
In this project, we employ deep generative models such as Generative Adversarial Networks (GANs), Denoising Diffusion Probabilistic Models (DDPMs), and Latent Diffusion Models (LDMs) to capture the data distribution of complex objects (photomasks in this case) in order to solve the phase retrieval problem. We consider image-to-image translation approaches, as well as inverse problem formulations coupled with conditional data generation from the generative models. Fig. 3 illustrates the qualitative phase reconstruction achieved by our proposed conditional latent diffusion model (LDM). It is organized into three rows for clear comparison. In the top row, we present the condition images obtained through a ptychography algorithm running for a minimal number of iterations, resulting in very noisy photomask images. The middle row displays the samples generated by our LDM model, showcasing its ability to produce high-quality photomasks. The bottom row contains the ground truth photomasks for reference. It is noteworthy that despite the high level of noise in the conditioning images, our LDM model is capable of generating photomasks of good quality. This demonstrates the efficacy of the LDM in handling noisy input data and accurately reconstructing the desired phase information.
Since deep generative models like GANs, DDPMs, and LDMs require large amounts of data for training, we have developed a synthetic photomask generation pipeline for data agumentation. This pipeline can generate a large number of synthetic photomasks (with or without defects) within a few hours on an HPC cluster (see Fig. 2).
Impact
The impact of this work is significant in advancing semiconductor manufacturing technologies. Firstly, by implementing deep generative models for phase retrieval in ptychography, this approach has the potential to enhance the accuracy and resolution of defect detection on EUV photomasks. This improvement directly supports the industry's ability to enable the production of smaller, more powerful semiconductor devices. Secondly, the use of such advanced models reduces the computational load and associated costs compared to traditional methods, making the process more economically viable and potentially lowering the barrier to adoption for manufacturers. Finally, the application of machine learning and computer vision techniques, such as deep generative modeling and image-to-image translation, provides a novel pathway to overcome the challenges of high data volumes and dynamic range in lithography, thus improving the efficiency and speed of the imaging process.

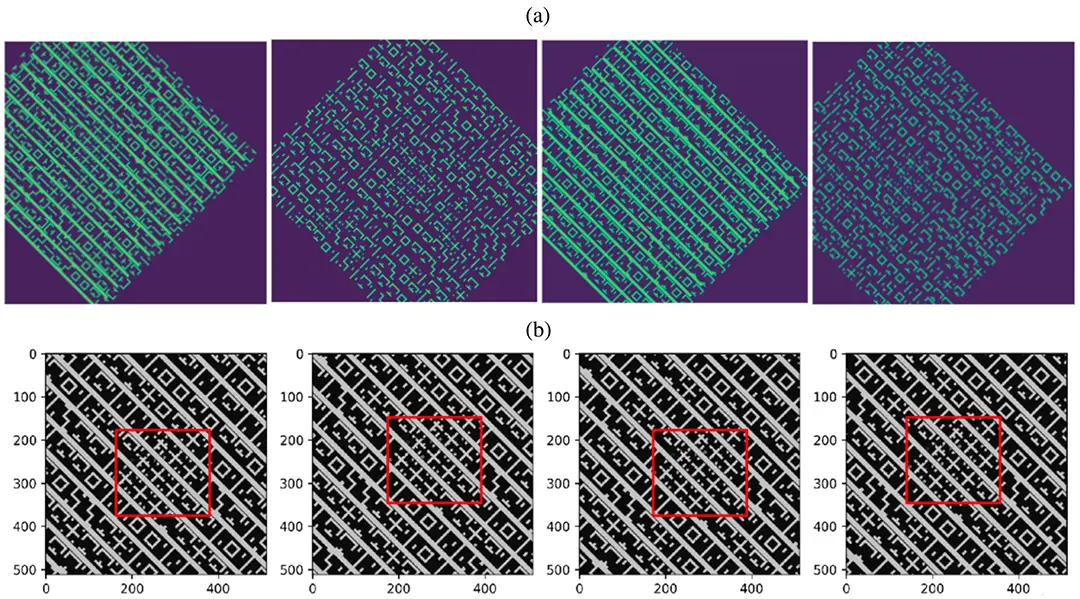

Presentation
Gallery
Annexe
Additional resources
Bibliography
- Bunday, B. D., Bello, A., Solecky, E. & Vaid, A. 7/5nm logic manufacturing capabilities and requirements of metrology in Metrology, Inspection, and Process Control for Microlithography XXXII (eds Adan, O. & Ukraintsev, V. A.) 10585 (SPIE, Mar. 2018), 17. isbn: 9781510616622. https://www.spiedigitallibrary.org/conference- proceedings- of- spie/10585/2296679/75nm- logic-
manufacturing-capabilities-and-requirements-of-metrology/10.1117/12.2296679.full. - Miyai, H., Kohyama, T., Suzuki, T., Takehisa, K. & Kusunose, H. Actinic patterned mask defect inspection for EUV lithography in Photomask Technology 2019 (eds Rankin, J. H. & Preil, M. E.) 11148 (SPIE, 2019), 162–170. https://doi.org/10.1117/12.2538001.
- Thibault, P., Dierolf, M., Bunk, O., Menzel, A. & Pfeiffer, F. Probe retrieval in ptychographic coherent diffractive imaging. Ultramicroscopy 109, 338–343 (2009).
- Gardner, D. F. et al. High numerical aperture reflection mode coherent diffraction microscopy using off-axis apertured illumination. Optics Express 20. issn: 1094-4087 (2012).
- Harada, T., Nakasuji, M., Nagata, Y., Watanabe, T. & Kinoshita, H. Phase imaging of EUV masks using a lensless EUV microscope in (ed Kato, K.) 8701 (International Society for Optics and
Photonics, June 2013), 870119. http : / / proceedings. spiedigitallibrary . org / proceeding . aspx?doi=10.1117/12.2027283. - RESCAN: an actinic lensless microscope for defect inspection of EUV reticles
I. Mochi, P. Helfenstein, I. Mohacsi, R. Rajeev, D. Kazazis, S. Yoshitake, and Y. Ekinci
J. Micro/Nanolith. MEMS MOEMS 16(4), 041003 (2017)
doi: 10.1117/1.JMM.16.4.041003 - Scanning coherent diffractive imaging methods for actinic EUV mask metrology
P. Helfenstein, I. Mohacsi, R. Rajendran, and Y. Ekinci
J. Micro/Nanolith. MEMS MOEMS 15(3), 034006 (2016)
doi: 10.1117/1.JMM.15.3.034006
Publications
Related Pages
More projects
ML-L3DNDT
BioDetect
News
Latest news


First National Calls: 50 selected projects to start in 2025
First National Calls: 50 selected projects to start in 2025
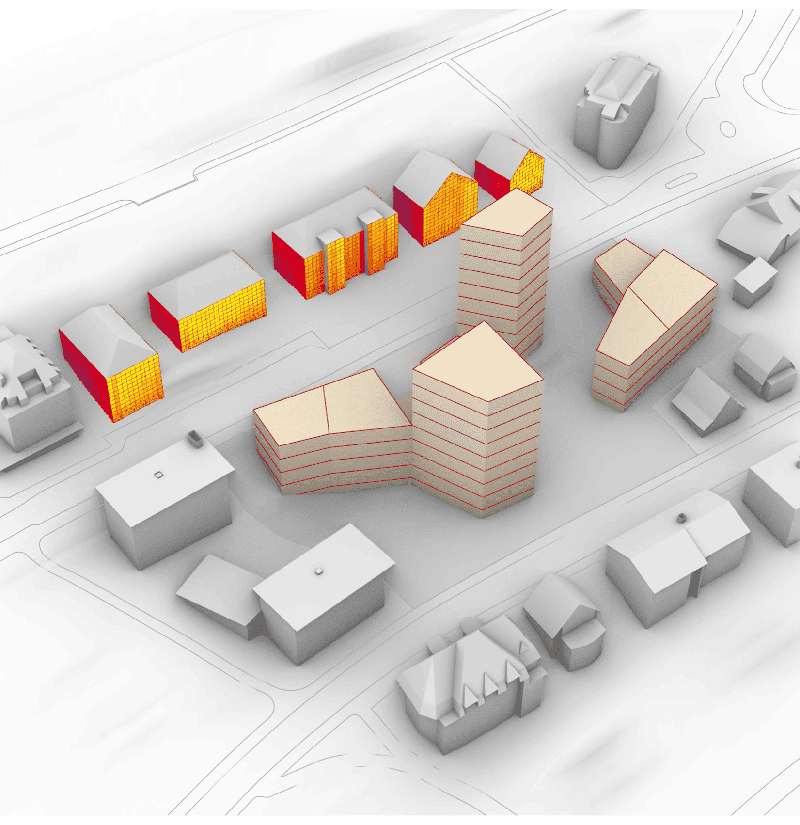
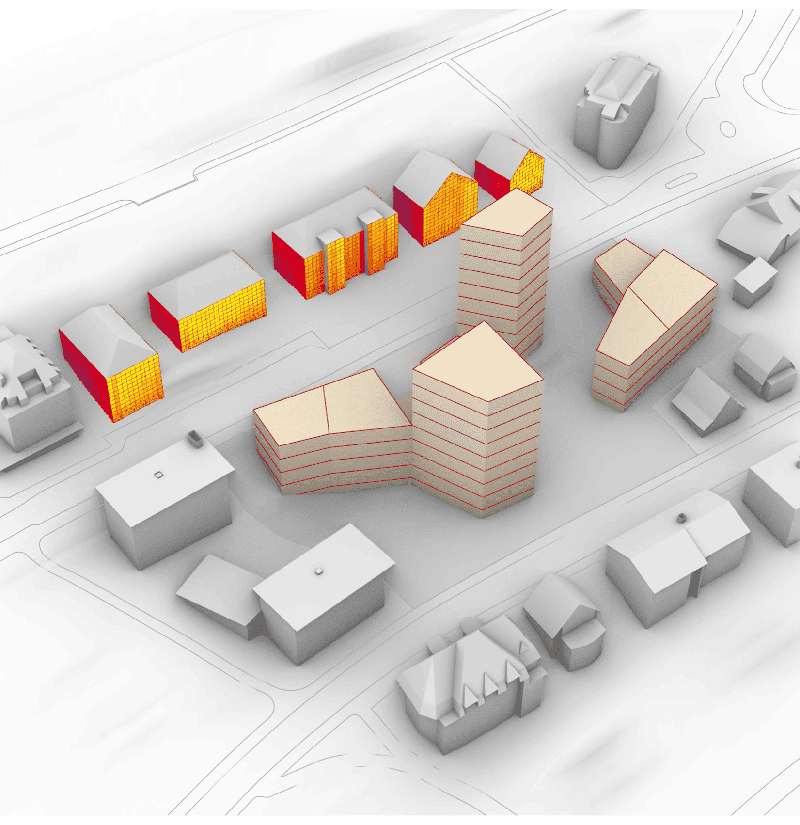
AIXD | Generative AI toolbox for architects and engineers
AIXD | Generative AI toolbox for architects and engineers


Smartair | An active learning algorithm for real-time acquisition and regression of flow field data
Smartair | An active learning algorithm for real-time acquisition and regression of flow field data
Contact us
Let’s talk Data Science
Do you need our services or expertise?
Contact us for your next Data Science project!