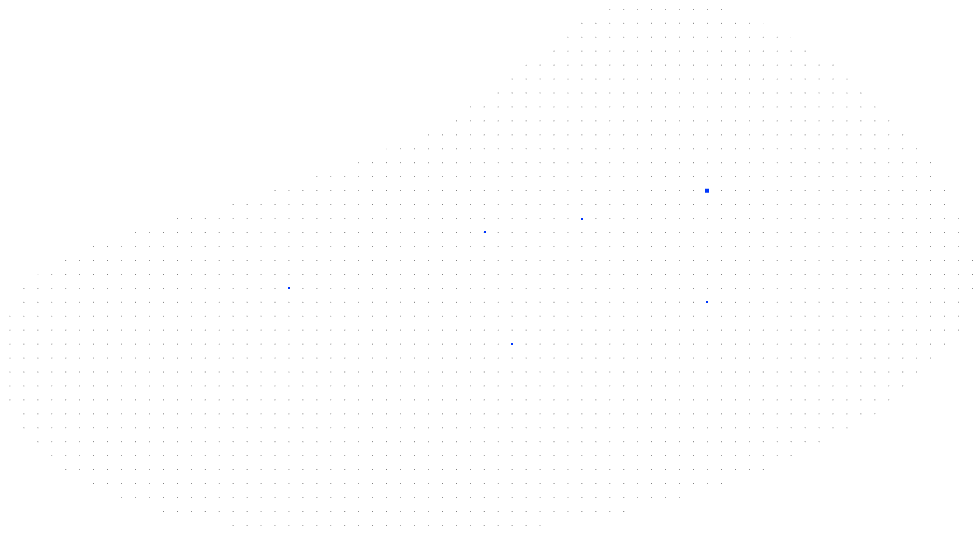
MLIBRA
Mouse LIpid Brain Atlas

Abstract
Lipids are fundamental constituents of all living beings: they participate in energy metabolism, account for the assembly of biological membranes, and act as signaling molecules. Brain cells produce thousands of different lipids, each with a distinct role in these biological processes.
With the development of lipidomics, it is possible to study the lipid compositional complexity of different brain parts. Nonetheless, a systematic and fine-grained characterization of lipid metabolism in the different brain regions is not available.
In this project a large MALDI Mass Spectrometry Imaging (MALDI-MSI) data of the spatial distribution (at the micrometric scale) of hundreds of lipids across the entire adult mouse brain is available. The goal of the project is to develop a tool that make use of the latest advances in statistical learning and deep neural networks to combine, integrate and analyze lipid distribution images of the brain from our data.
These computational procedures will be instrumental for assembling the lipid metabolic atlas of the mouse brain, a resource with a tremendous impact on neurochemical research and the understanding of brain metabolism in health and disease.
People
Collaborators
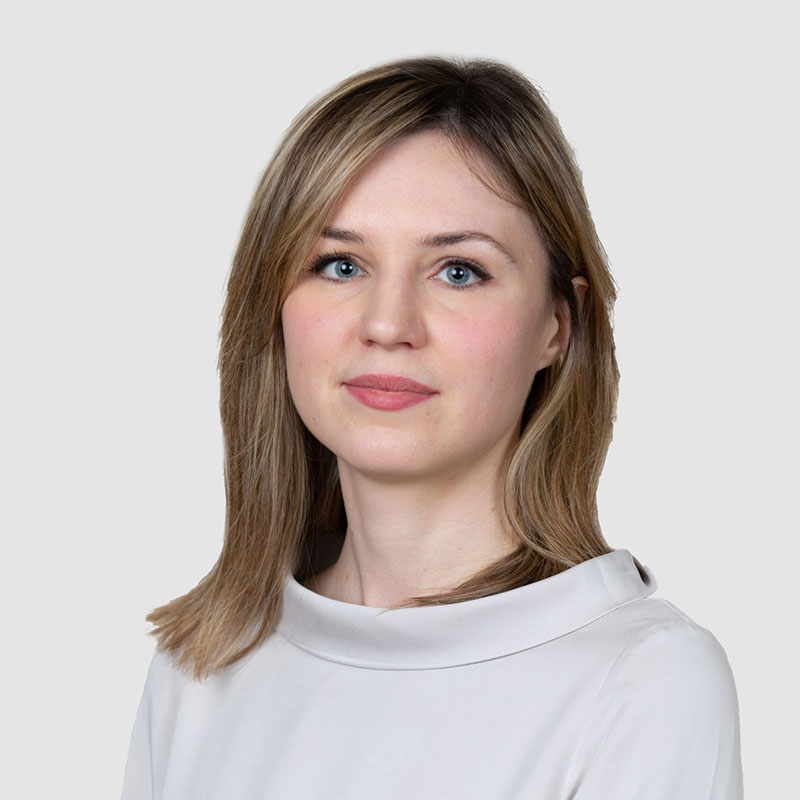
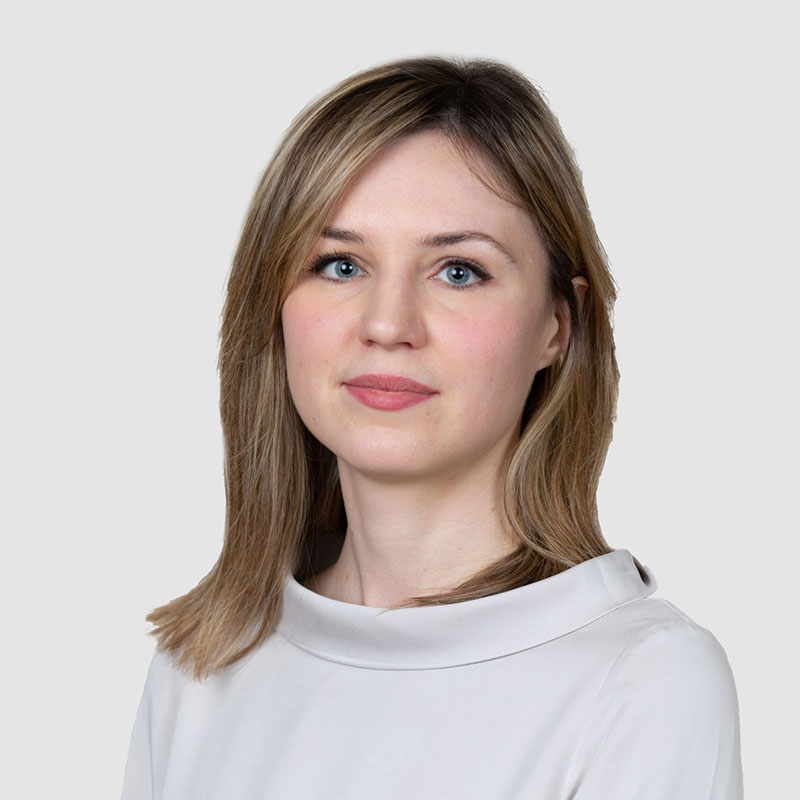
Ekaterina received her PhD in Computer Science from Moscow Institute for Physics and Technology, Russia. Afterwards, she worked as a researcher at the Institute for Information Transmission Problems in Moscow and later as a postdoctoral researcher in the Stochastic Group at the Faculty of Mathematics at University Duisburg-Essen, Germany. She has experience with various applied projects on signal processing, predictive modelling, macroeconomic modelling and forecasting, and social network analysis. She joined the SDSC in November 2019. Her interests include machine learning, non-parametric statistical estimation, structural adaptive inference, and Bayesian modelling.
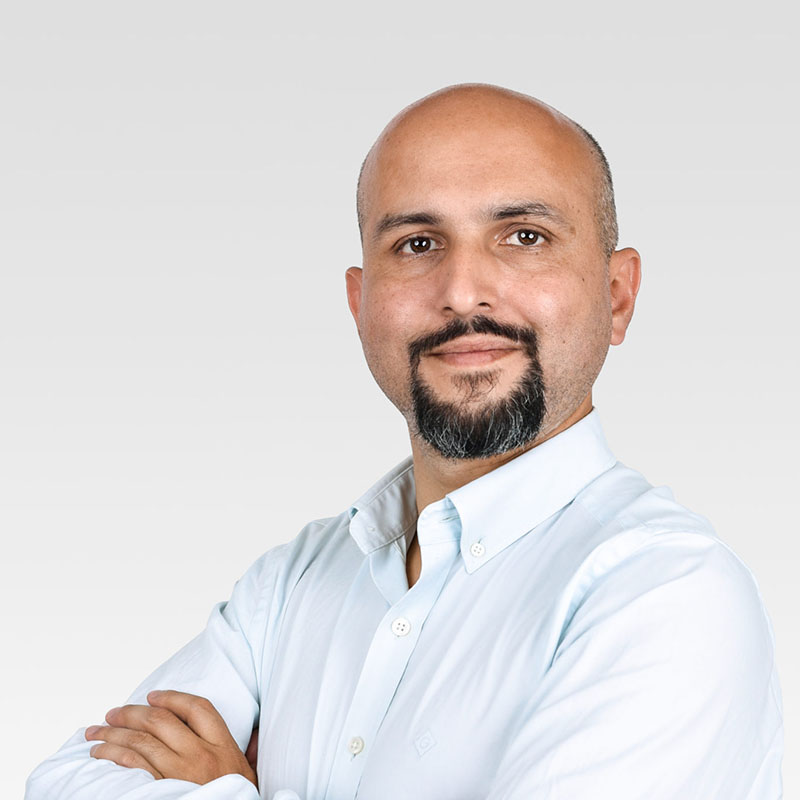
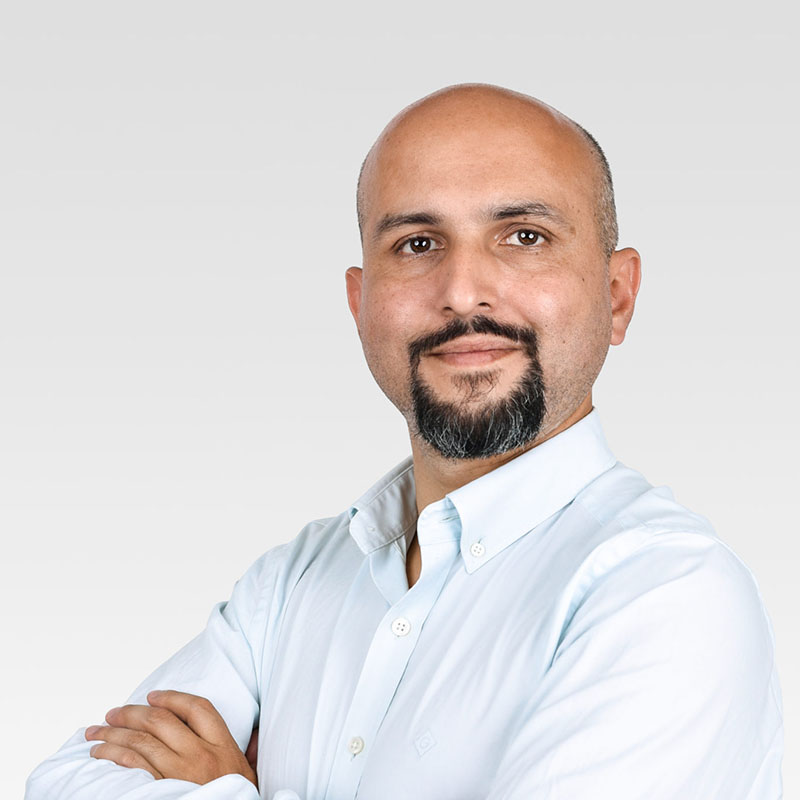
Daniel worked as a postdoctoral researcher on critical event prediction for the University Hospital in Zurich. In addition, Daniel has worked as a postdoctoral researcher in Lausanne, delivering algorithms for Bayesian inference in big panel data. Previously in Paris, he developed models for automated scientific discovery. He obtained a Ph.D. from the University of Edinburgh, funded by a Microsoft Research scholarship. His interest relates primarily to attacking applied biomedicine problems from different angles, frequentist statistics, Bayesian statistics, and Machine Learning.
PI | Partners:
EPFL, Lab of Brain Development and Biological Data Science:
- Prof. Gioele La Manno
- Prof. Giovanni D’Angelo
- Hannah Schede
- Luca Fusar Bassini
- Leila Alieh
description
Motivation
Brain atlases are an invaluable resource for the interpretation of structure-function relationships, provide a reference to the study of molecular heterogeneity among various brain cells, and a framework to formulate hypotheses about brain functioning and development. Although there exists today a wealth of knowledge regarding the different neuroanatomical regions, their precise chemical composition remains relatively unclear. This is unfortunate as brain operation largely relies on the electrochemical activity of neural cells. Lipids are a major component of the mammalian brain as they constitute half of the brain’s dry weight and are key components of every cell. Lipids are a chemically diverse class of molecules that participate in central metabolism, constitute the fabric of biomembranes and act as first and second messengers in signal transduction events. Different neural cell types produce different lipids, which impact many biological processes, including budding and fusion of synaptic vesicles, propagation of action potential, and insulation of axons as components of the myelin sheets. However, a systematic and fine-grained characterization of lipid metabolism in the different brain regions is currently unavailable. In this project, we propose compiling the first whole mammalian organ’s 3D metabolic atlas: the mouse Lipid Brain Atlas. We aim at identifying homogenous regions of lipid expression across the mouse brain. The further goal is to study these regions and get insights about differences in lipid distributions within different sexes, during pregnancy, and differences defined via gene expression change.
Proposed Approach / Solution
We developed an automatic hierarchical segmentation of brain slice MALDI readings. This approach uses iterative bipartitioning and a generative probabilistic model. We then aim at identifying differences in brains of different types and relate these regions to gene expression and chemical pathways.

Impact
All research results will be collected in an interactive web atlas, in which researchers can explore the data and compare with their readings in their own experiments. Given the importance of mice brain for biomedicine, this could open venues for new lines of research or make relations between lipid distribution and other biological processes.
Presentation
Gallery
Annexe
Additional resources
Bibliography
- La Manno, Gioele, Kimberly Siletti, Alessandro Furlan, Daniel Gyllborg, Elin Vinsland, Alejandro Mossi Albiach, Christoffer Mattsson Langseth, et al. 2021. “Molecular Architecture of the Developing Mouse Brain.” Nature 596 (7870): 92–96.
- Bruce, Kimberley D., Andrea Zsombok, and Robert H. Eckel. 2017. “Lipid Processing in the Brain: A Key Regulator of Systemic Metabolism.” Frontiers in Endocrinology 8 (April): 60.
- Dawson, Glyn. 2015. “Measuring Brain Lipids.” Biochimica et Biophysica Acta 1851 (8): 1026–39.
- Jungmann, Julia H., and Ron M. A. Heeren. 2012. “Emerging Technologies in Mass Spectrometry Imaging.” Journal of Proteomics 75 (16): 5077–92.
Publications
Related Pages
More projects
ML-L3DNDT
BioDetect
News
Latest news
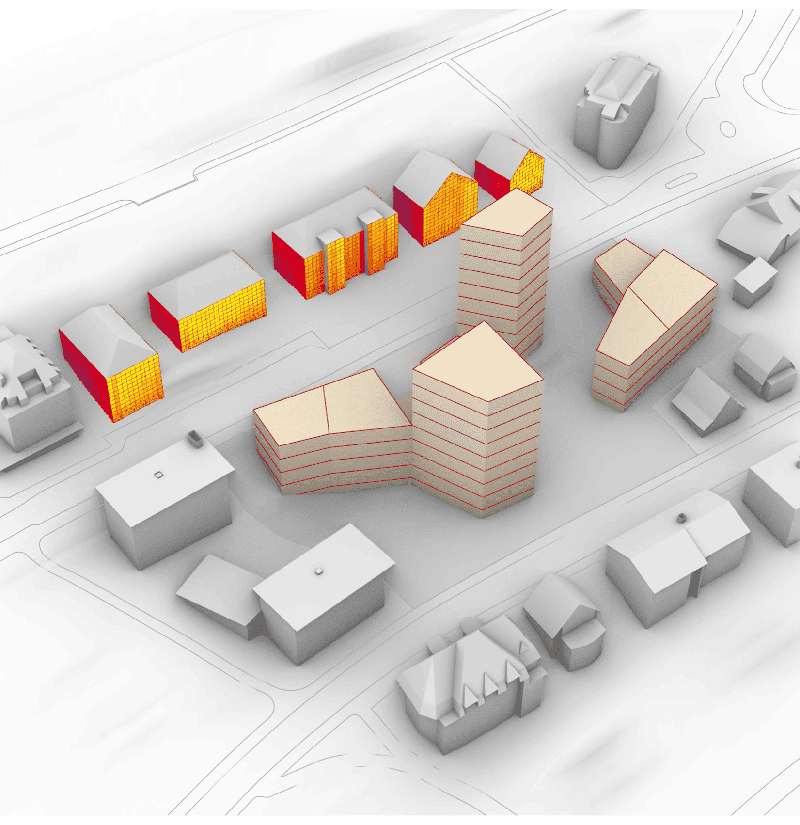
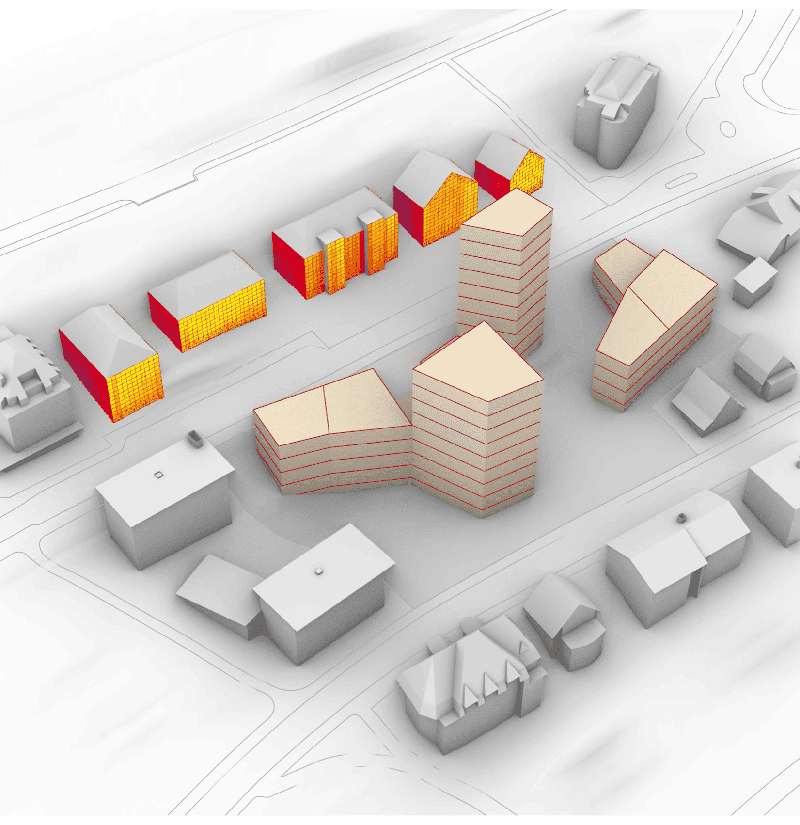
AIXD | Generative AI toolbox for architects and engineers
AIXD | Generative AI toolbox for architects and engineers


Smartair | An active learning algorithm for real-time acquisition and regression of flow field data
Smartair | An active learning algorithm for real-time acquisition and regression of flow field data
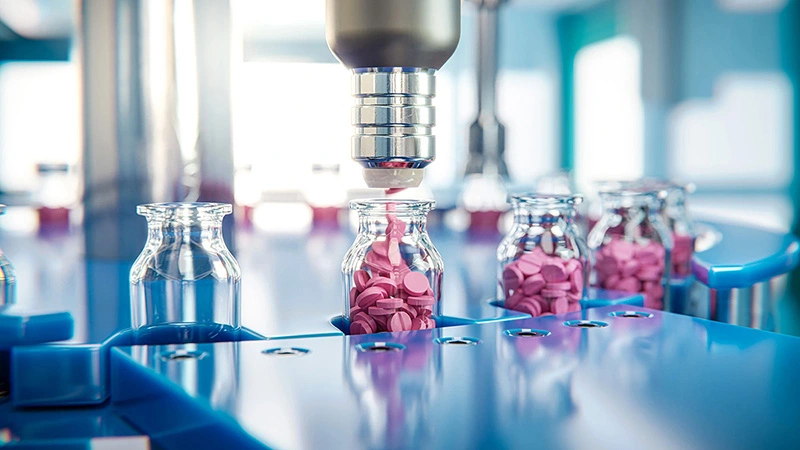
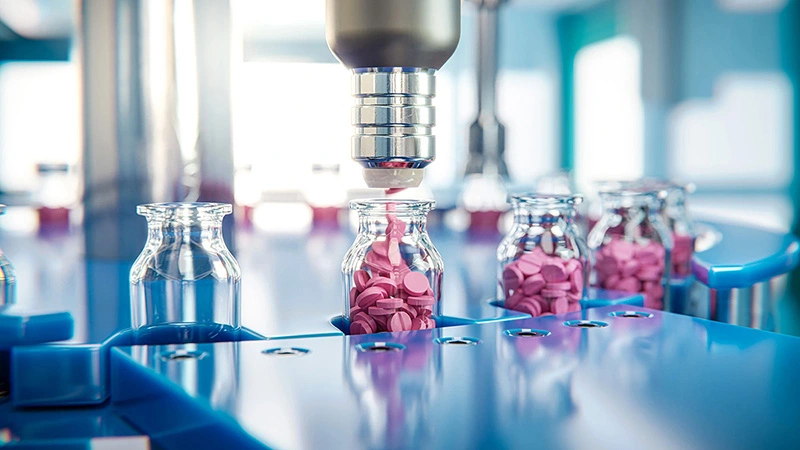
The Promise of AI in Pharmaceutical Manufacturing
The Promise of AI in Pharmaceutical Manufacturing
Contact us
Let’s talk Data Science
Do you need our services or expertise?
Contact us for your next Data Science project!