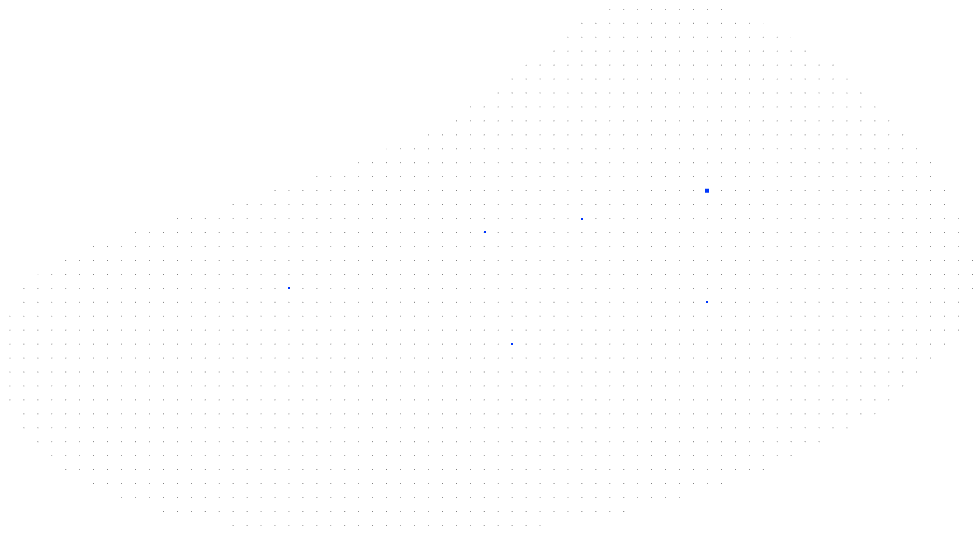
4Real
Real-time urban pluvial flood forecasting

Abstract
We aim to develop a forecasting model for urban floods, by tightly integrating physical modelling with data-driven deep learning. Our primary application scenario are urban pluvial floods that occur when precipitation cannot be fully absorbed by the drainage system, therefore causing flooding and substantial damages, as well as disruption to socio-economic activities. Structural engineering solutions to cope with urban flooding are large and expensive to build and maintain, and it is important to also advance non-structural solutions, and in particular real-time flood prediction. The fast occurrence and relatively short duration of urban floods (“flash floods”) means that physically-based models are of limited use, due to their long run times. In this project, we will develop new Machine Learning (ML) methods to generate flood predictions with sufficient lead time, such that they can be used to alert the population and to plan mitigation and rescue actions. Our core research question in this project is: how can deep learning and physically-based hydraulic models be combined? Our hypothesis is that by exploiting hydraulic modelling knowledge, deep learning need not “ statistically learn physics from scratch”. By tightly integrating the two modelling approaches, we aim to get the best of both worlds: interpretability and adherence to physical constraints; outputs with well-calibrated uncertainty estimates; and the predictive power and speed of neural networks. The input data for the ML flood forecasting model will be rainfall forecasts provided by meteorological services (e.g. from weather radar), images and digital surface models. The ML-based flood model will produce spatially explicit, two-dimensional flood hazard maps with water depth, flood extent and flow velocity information.
People
Collaborators
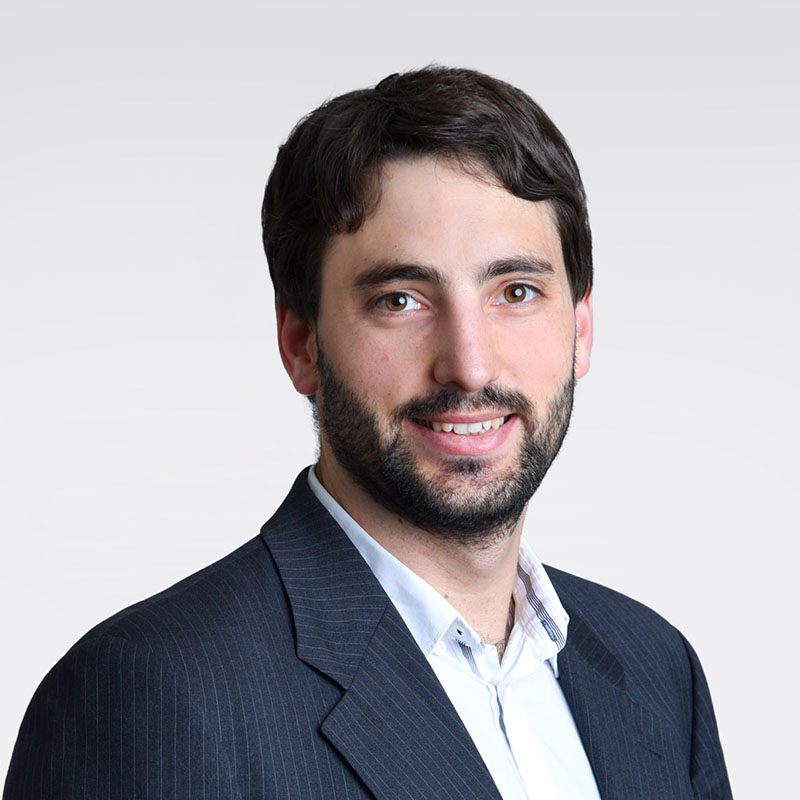
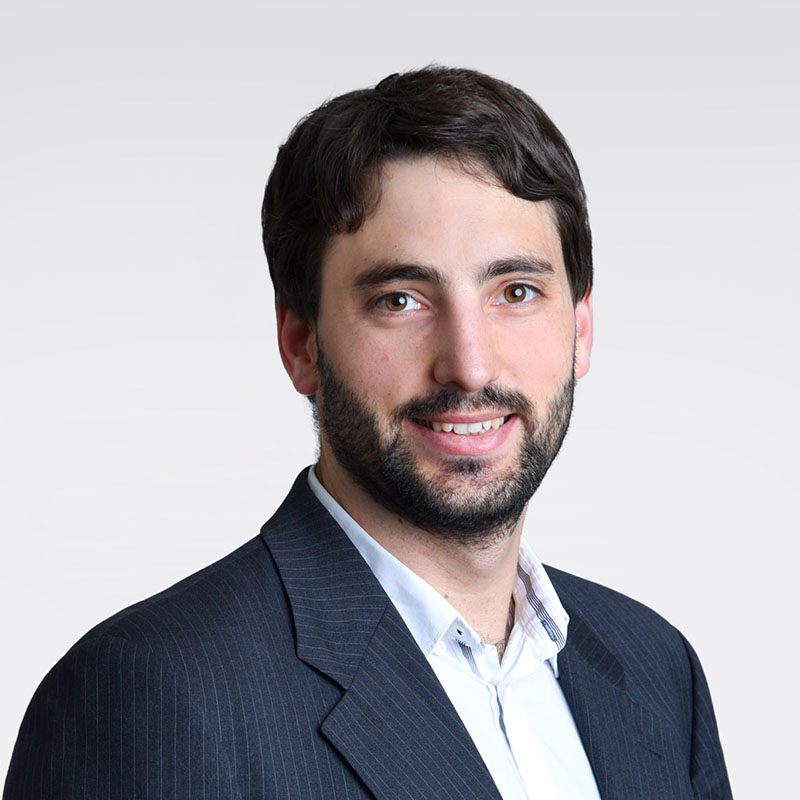
After finishing his Master in electrical engineering at the Ecole Fédérale de Lausanne (EPFL), Nathanaël worked as a researcher in the Acoustic Research Institute (ARI) in Vienna. In 2013, he returned to EPFL for a PhD, where he specialized himself in different fields of data science: signal processing, machine learning, graph theory and optimization. Furthermore, he created two open source libraries for optimization (UNLocBoX) and graph signal processing (GSPBOX). Since 2017, Nathanaël Perraudin is a Research Data Scientist at the Swiss Data Science Center in the ETH Zurich. He focuses on different aspects of deep learning in the area of generative models (VAE and GAN), recursive architectures and convolutional neural network for irregular domains. Outside office hours, he is passionate by tango dancing, tandem bike touring, skiing and rock climbing.
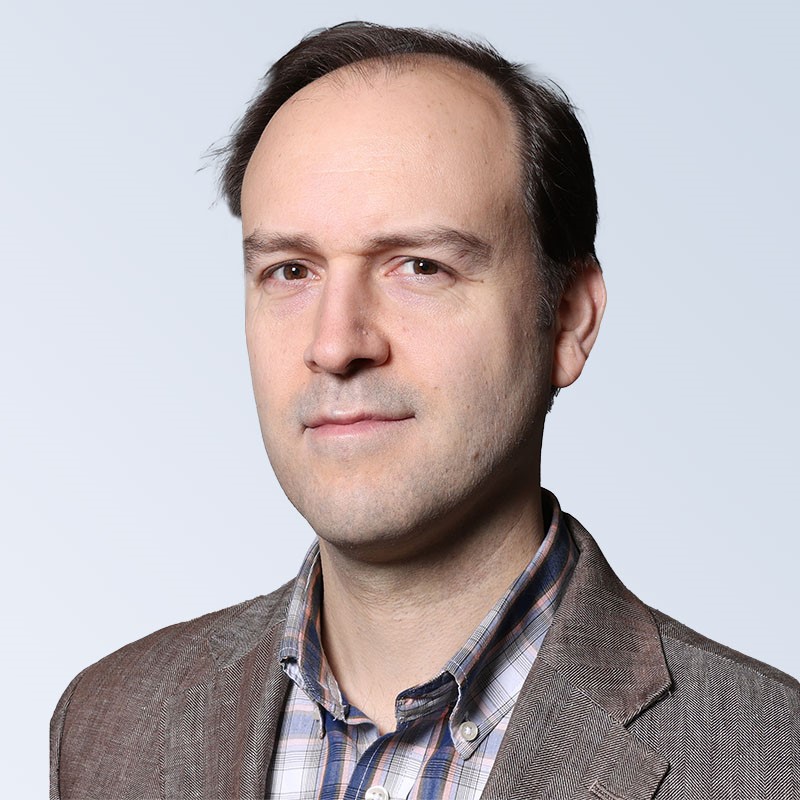
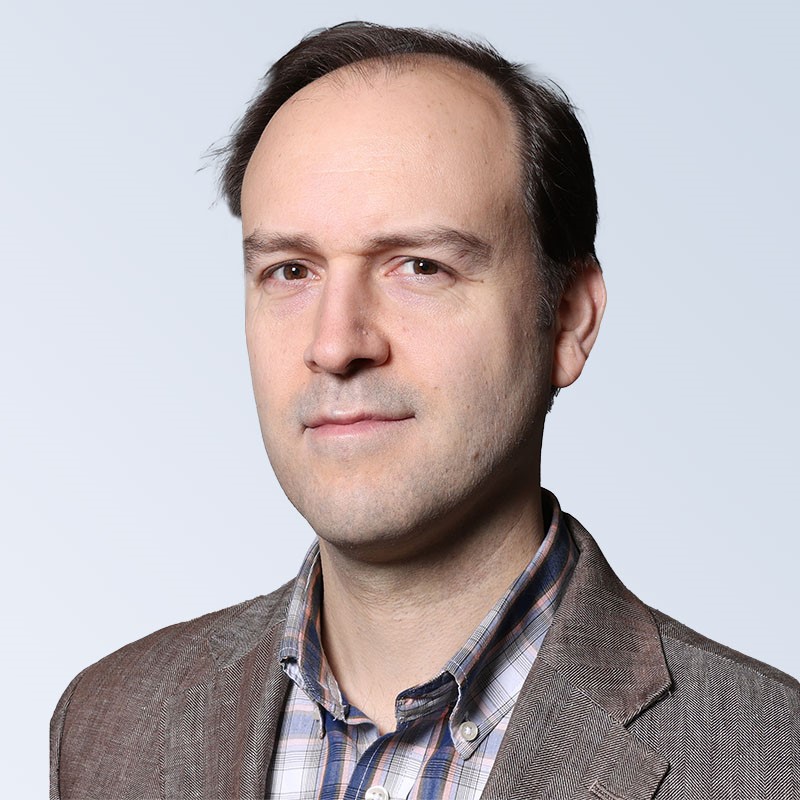
Guillaume Obozinski graduated with a PhD in Statistics from UC Berkeley in 2009. He did his postdoc and held until 2012 a researcher position in the Willow and Sierra teams at INRIA and Ecole Normale Supérieure in Paris. He was then Research Faculty at Ecole des Ponts ParisTech until 2018. Guillaume has broad interests in statistics and machine learning and worked over time on sparse modeling, optimization for large scale learning, graphical models, relational learning and semantic embeddings, with applications in various domains from computational biology to computer vision.
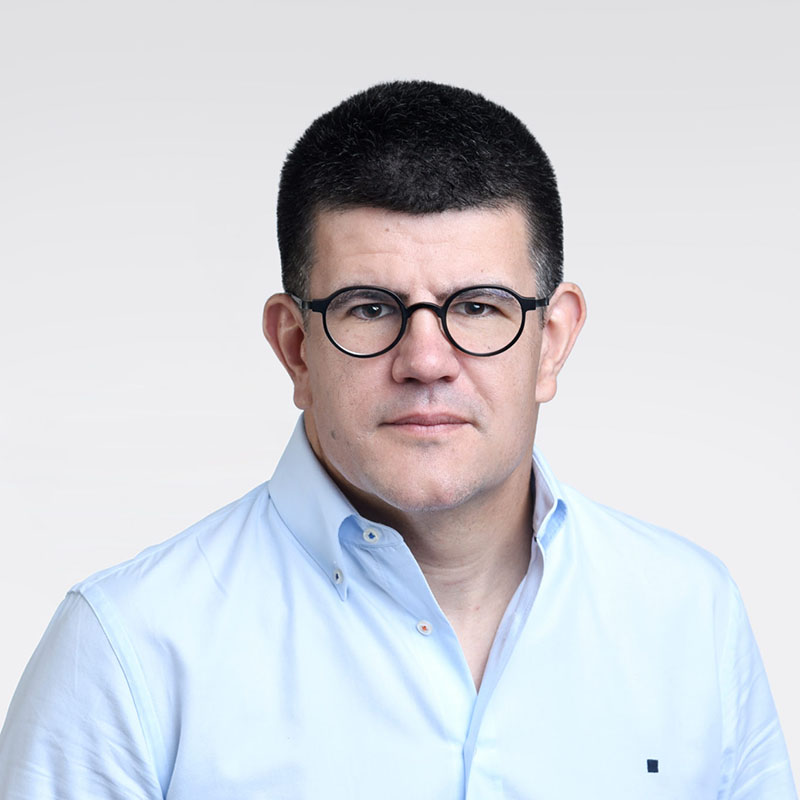
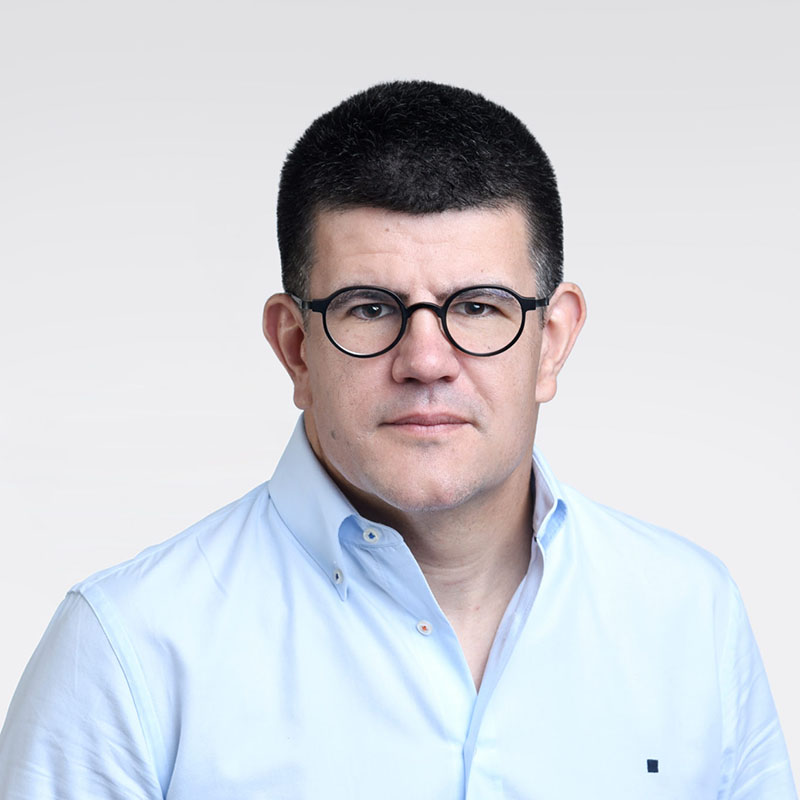
Fernando Perez-Cruz received a PhD. in Electrical Engineering from the Technical University of Madrid. He is Titular Professor in the Computer Science Department at ETH Zurich and Head of Machine Learning Research and AI at Spiden. He has been a member of the technical staff at Bell Labs and a Machine Learning Research Scientist at Amazon. Fernando has been a visiting professor at Princeton University under a Marie Curie Fellowship and an associate professor at University Carlos III in Madrid. He held positions at the Gatsby Unit (London), Max Planck Institute for Biological Cybernetics (Tuebingen), and BioWulf Technologies (New York). Fernando Perez-Cruz has served as Chief Data Scientist at the SDSC from 2018 to 2023, and Deputy Executive Director of the SDSC from 2022 to 2023
description
Problem:
Urban pluvial floods, occurring when precipitation cannot be fully absorbed by the drainage system, cause flooding and substantial damages, as well as disruption to socio-economic activities. Their fast occurrence and relatively short duration mean that physically-based models for flood prediction are of limited use, due to their long computational runtime.
Goal:
This project goal is to develop new Deep Learning (DL) methods to generate real-time flood predictions, such that they can be used to alert the population and to plan mitigation and rescue actions. Additionally, by exploiting hydraulic modelling knowledge and tightly integrating it with DL models, we aim to produce DL-based flood models which return spatially explicit, two-dimensional flood hazard maps with water depth, flood extent and flow velocity information. Tightly coupling the underlying hydraulic equations with a DL-framework will provide both, interpretability and adherence to physical constraints. The input data for the DL flood forecasting model will be rainfall forecasts provided by meteorological services (MeteoSwiss), images and 3D digital surface city models.
Presentation
Gallery
Annexe
Additional resources
Bibliography
- Kim, B., Azevedo, V.C., Thuerey, N., Kim, T., Gross, M., Solenthaler, B.: Deep Fluids (2019). A Generative Network for Parameterized Fluid Simulations. Eurographics, 38(2)
- Chaudhary, P., d‘Aronco, S., Moy de Vitry, M., L eitão, J.P., Wegner, J.D.: Flood-water level estimation from social media images. ISPRS Annals of the Photogrammetry, Remote Sensing and Spatial Information Sciences, 2019, vol. IV-2/W5, 5 – 12.
- Ladický, L., Jeong, S., Solenthaler, B., Pollefeys, M., Gross, M. (2015). Data-Driven Fluid Simulations using Regression Forests. Transactions on Graphics (SIGGRAPH Asia)
- Leitão, J.P., Simões, N. E., Maksimović, Č., Ferreira, F., Prodanović, D., Matos, J.S., Sá Marques, A. (2010). Real-time forecasting urban drainage models: full or simplified networks? Water Science and Technology, 62 (9), 2106–2114
- Winkler, D., Zischg, J. Rauch, W. Virtual reality in urbanwater management: communicating urban flooding with particle- based CFD simulations. Water Science and Technology, 77(2), 518-524
- Kendall, A., Gal, Y. (2017). What uncertainties do we need in bayesian deep learning for computer vision? Advances in neural information processing systems, 5574-5584
- Leitão, J.P., Zaghloul, M.,Moosavi, V. (2018). Modelling overland flow from local inflows in “almost no‐time” using Self‐ Organizing Maps. 2018 International Conferences in Urban Drainage Modelling
Publications
Related Pages
More projects
ML-L3DNDT
BioDetect
News
Latest news


Smartair | An active learning algorithm for real-time acquisition and regression of flow field data
Smartair | An active learning algorithm for real-time acquisition and regression of flow field data
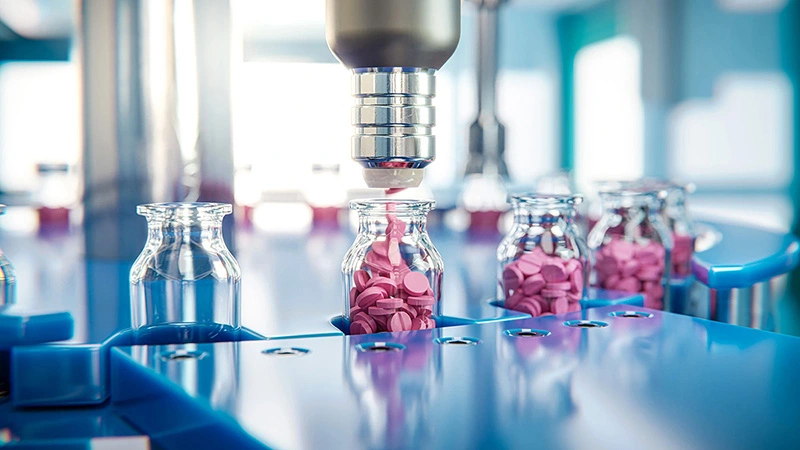
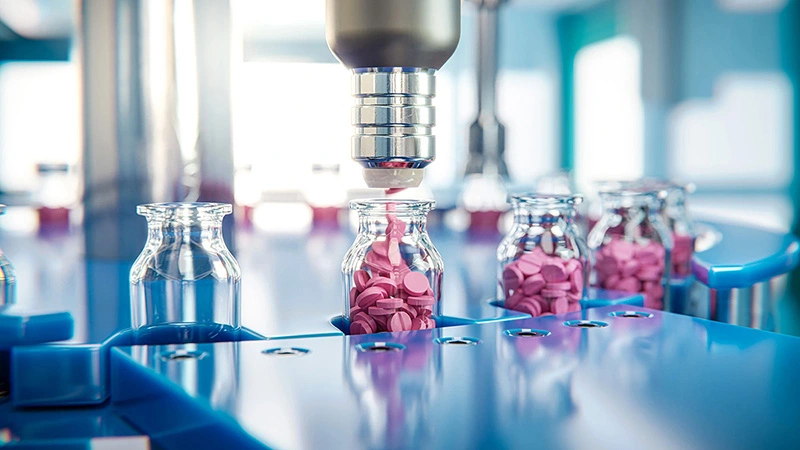
The Promise of AI in Pharmaceutical Manufacturing
The Promise of AI in Pharmaceutical Manufacturing


Efficient and scalable graph generation through iterative local expansion
Efficient and scalable graph generation through iterative local expansion
Contact us
Let’s talk Data Science
Do you need our services or expertise?
Contact us for your next Data Science project!