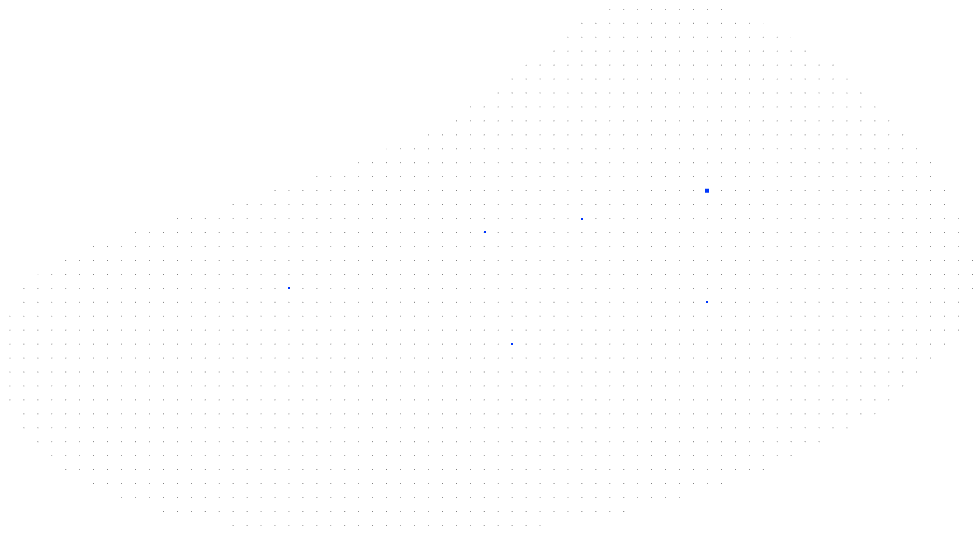
EconMultiplex
Multiplex Networks in International Trade

Abstract
This project addresses the issue of economic network interdependence at the level of firms in China and their connectedness among each other as well as with the rest of the world. China accounts for about one-fifth of the world’s population and is on the way to become the world’s largest exporting economy. Since the Economic and Financial Crisis of 2007/08, researchers have become aware that understanding economic network structures at the micro-level is vital for determining the exposure and resilience of firms, jobs, sectors, and countries to adverse shocks globally. With China’s importance as a supplier of intermediate and final goods, understanding this economy as well as its internal and external economic network structure is of global importance. This project will use modern methods in data science and statistics (network and spatial econometrics) to gauge insights into the nature of the domestic and foreign network structure of Chinese firms.
People
Collaborators


Corinne joined the SDSC as a senior data scientist in December 2020. She graduated with a PhD in Statistics from the University of Washington in 2020. Her doctoral research focused on representation learning for partitioning problems. Prior to her PhD, she obtained bachelor's degrees in Math, Statistics, and Economics, along with a master's degree in Economics, from Penn State University. Her research interests include deep learning and kernel-based methods, with applications in fields ranging from computer vision to oceanography.
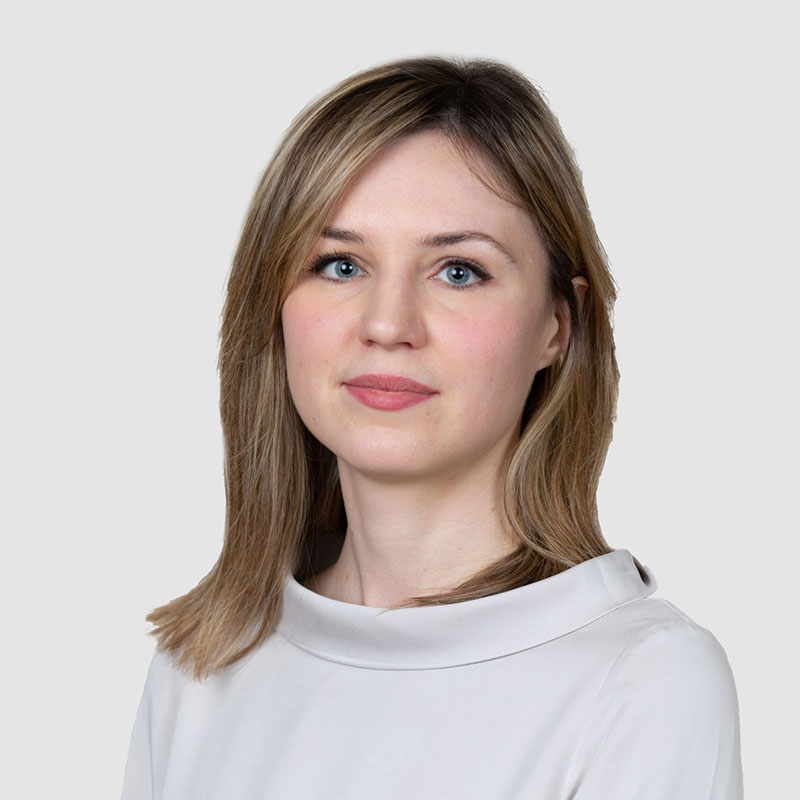
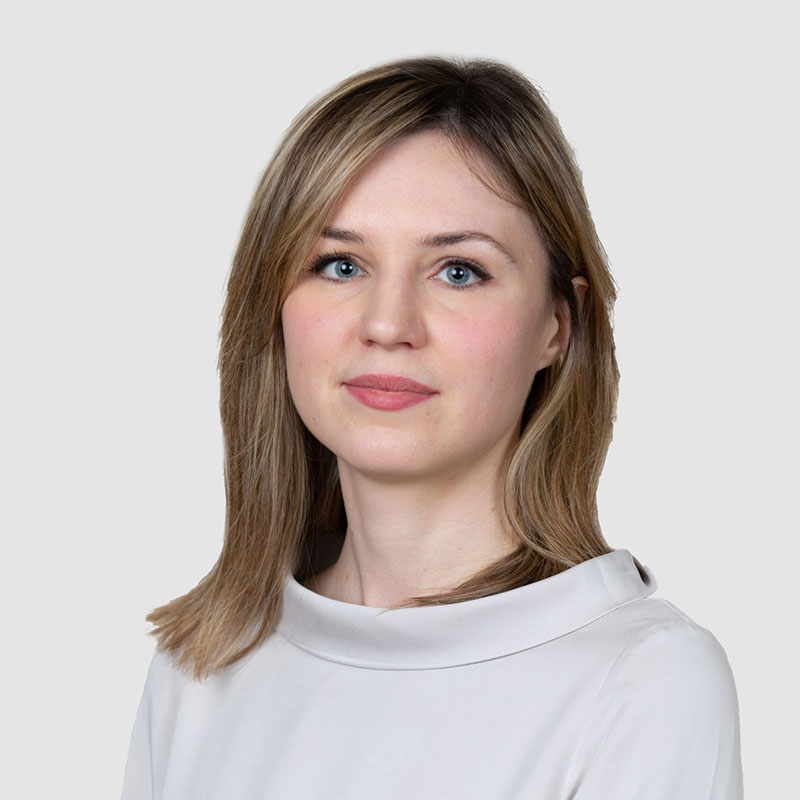
Ekaterina received her PhD in Computer Science from Moscow Institute for Physics and Technology, Russia. Afterwards, she worked as a researcher at the Institute for Information Transmission Problems in Moscow and later as a postdoctoral researcher in the Stochastic Group at the Faculty of Mathematics at University Duisburg-Essen, Germany. She has experience with various applied projects on signal processing, predictive modelling, macroeconomic modelling and forecasting, and social network analysis. She joined the SDSC in November 2019. Her interests include machine learning, non-parametric statistical estimation, structural adaptive inference, and Bayesian modelling.
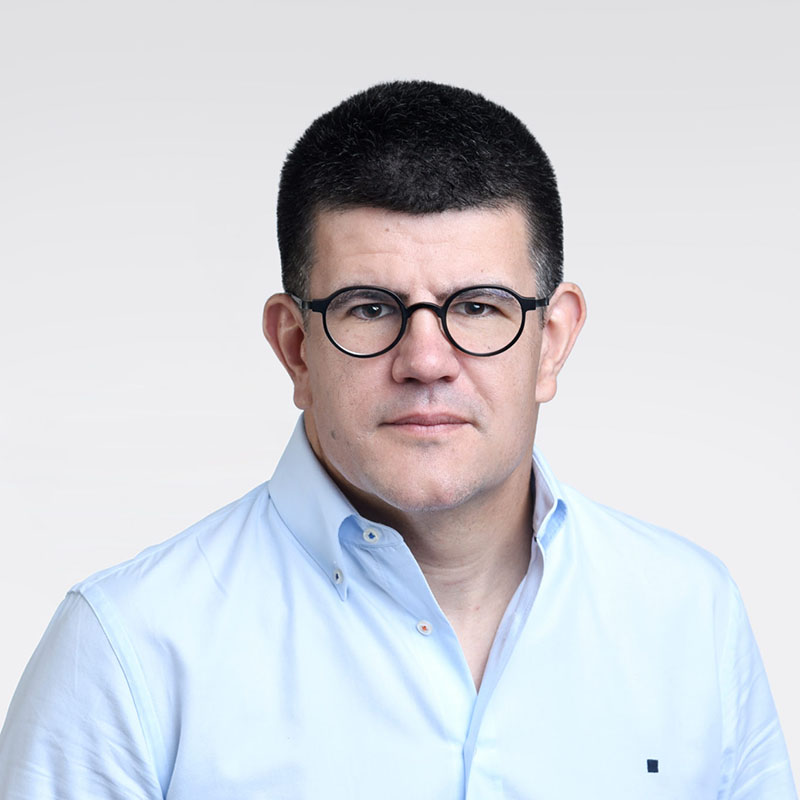
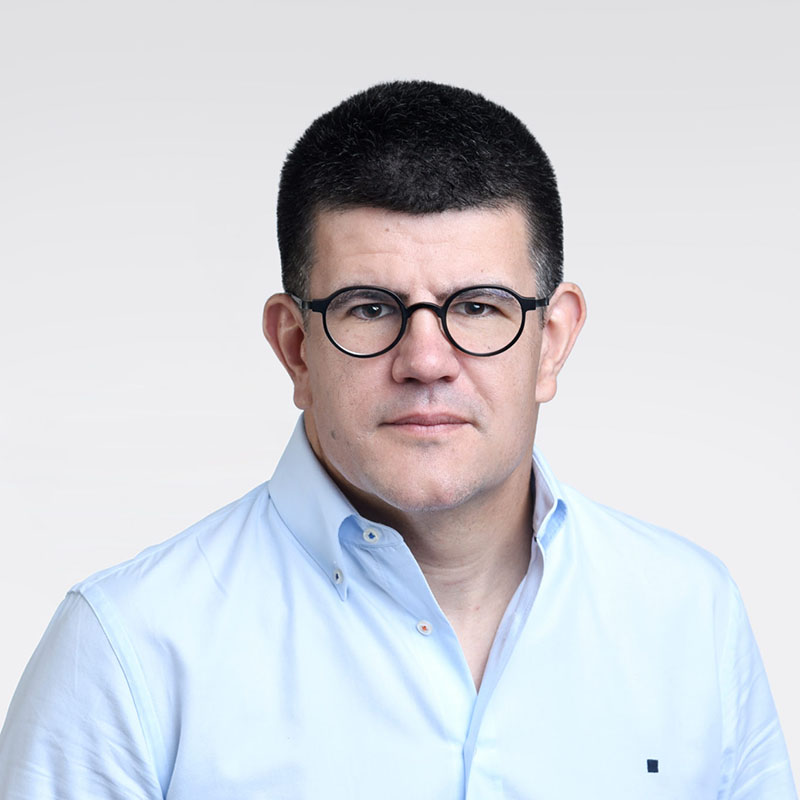
Fernando Perez-Cruz received a PhD. in Electrical Engineering from the Technical University of Madrid. He is Titular Professor in the Computer Science Department at ETH Zurich and Head of Machine Learning Research and AI at Spiden. He has been a member of the technical staff at Bell Labs and a Machine Learning Research Scientist at Amazon. Fernando has been a visiting professor at Princeton University under a Marie Curie Fellowship and an associate professor at University Carlos III in Madrid. He held positions at the Gatsby Unit (London), Max Planck Institute for Biological Cybernetics (Tuebingen), and BioWulf Technologies (New York). Fernando Perez-Cruz has served as Chief Data Scientist at the SDSC from 2018 to 2023, and Deputy Executive Director of the SDSC from 2022 to 2023
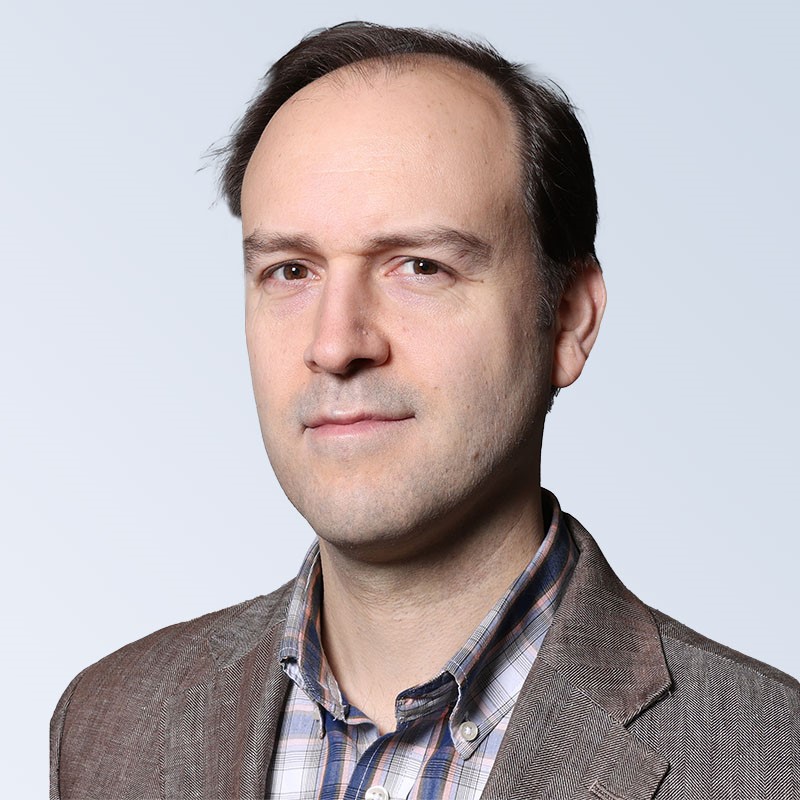
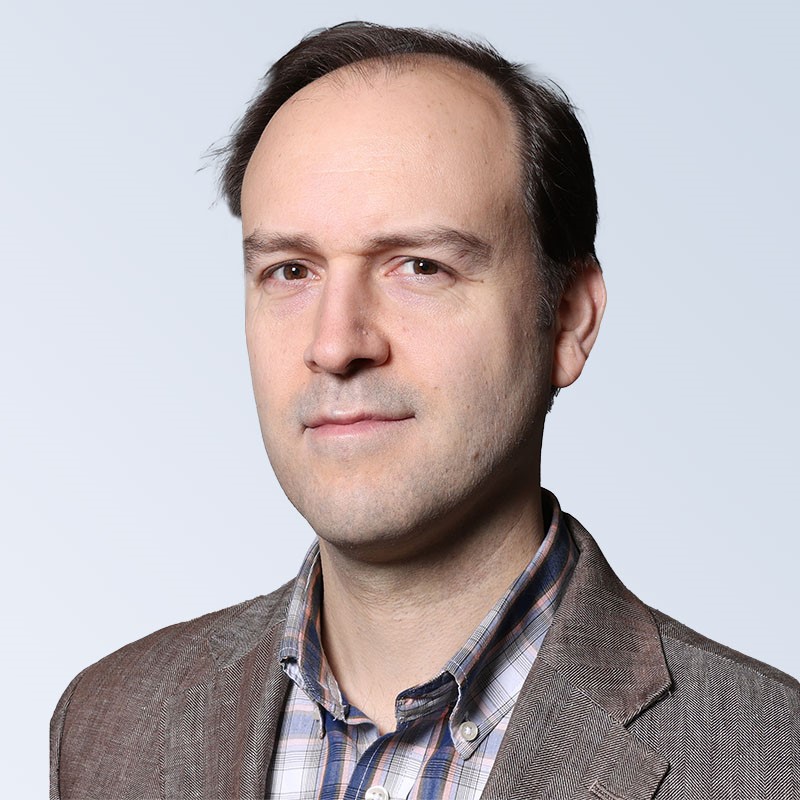
Guillaume Obozinski graduated with a PhD in Statistics from UC Berkeley in 2009. He did his postdoc and held until 2012 a researcher position in the Willow and Sierra teams at INRIA and Ecole Normale Supérieure in Paris. He was then Research Faculty at Ecole des Ponts ParisTech until 2018. Guillaume has broad interests in statistics and machine learning and worked over time on sparse modeling, optimization for large scale learning, graphical models, relational learning and semantic embeddings, with applications in various domains from computational biology to computer vision.
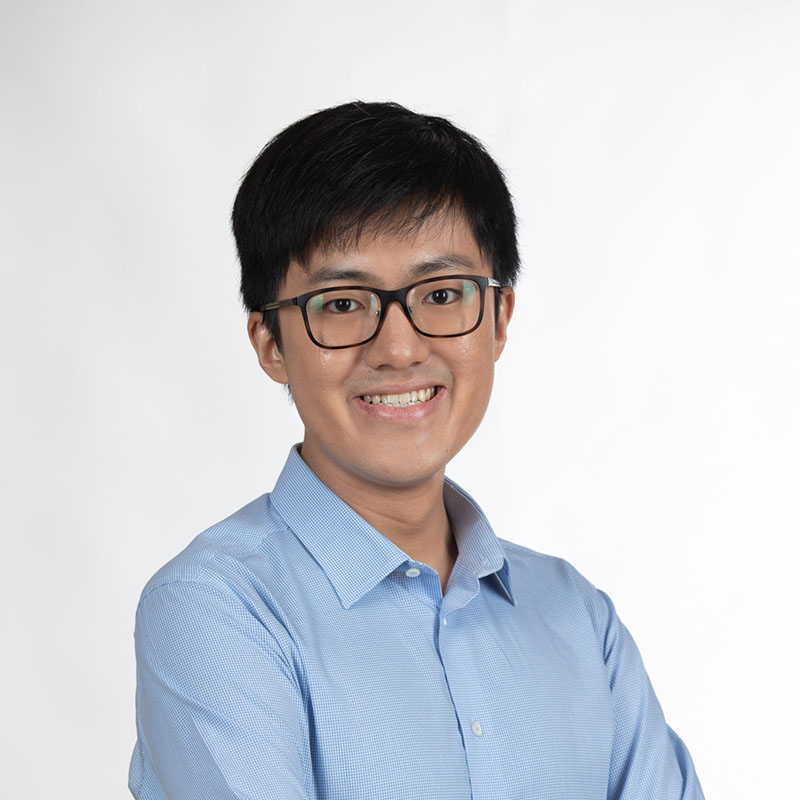
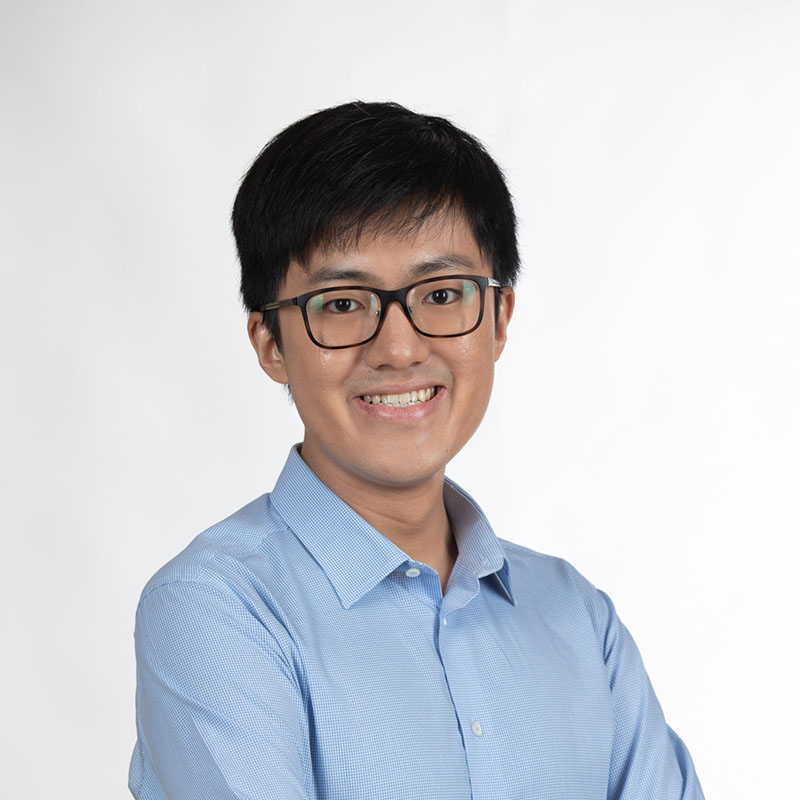
Tao Sun joined SDSC as a Computer Scientist in March 2020. Tao holds a MSc in Electrical & Electronic Engineering from EPFL, where he specialized in data analytics and developed a keen interest in machine learning. Before coming to Switzerland, he completed his undergraduate studies in Zhejiang University (Hangzhou, China) with a major in Electronic Information Engineering and a minor in Public Administration.
PI | Partners:
ETH Zurich, Chair of Applied Economics: Innovation and Internationalisation:
- Prof. Peter Egger
- Dr. Sophia Ding
- Dr. Nicole Loumeau
- Susie Xi Rao
- Vincent Lohmann
description
Problem:
Combine methods in data science, statistics/econometrics and economics to analyse:
- The evolution of large, multilayer domestic and trade networks of firms.
- Study their consequences for economic outcomes, focusing on one of the largest economies on the globe.
Proposed approach:
Perform Bayesian non-parametric modeling to understand the behavior of Chinese firms when exporting, and the behavior of consumer countries when importing, based on the firm/region-product-consumer-destination space.
Impact:
Advance probabilistic machine learning methods for network analysis and statistical/econometric methods in data with endogenous network structure.
Presentation
Gallery

Annexe
Publications
- Peter Egger and Corinne Jones. Co-Exportation of Products by Multi-Product Firms (18683). CEPR Discussion Paper, 2023.
- Peter Egger, Susie Xi Rao, and Sebastiano Papini. A new algorithm for matching ChineseNBS firm-level with customs data. China Economic Journal, 14(3):311–335, 2021.
Additional resources
Bibliography
Publications
Related Pages
More projects
ML-L3DNDT
BioDetect
News
Latest news
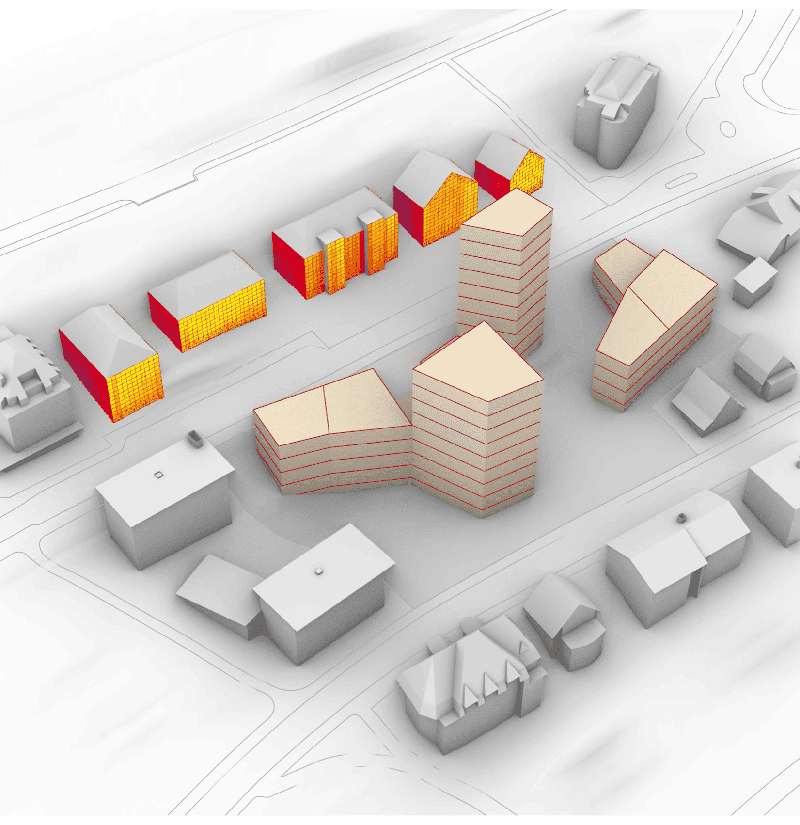
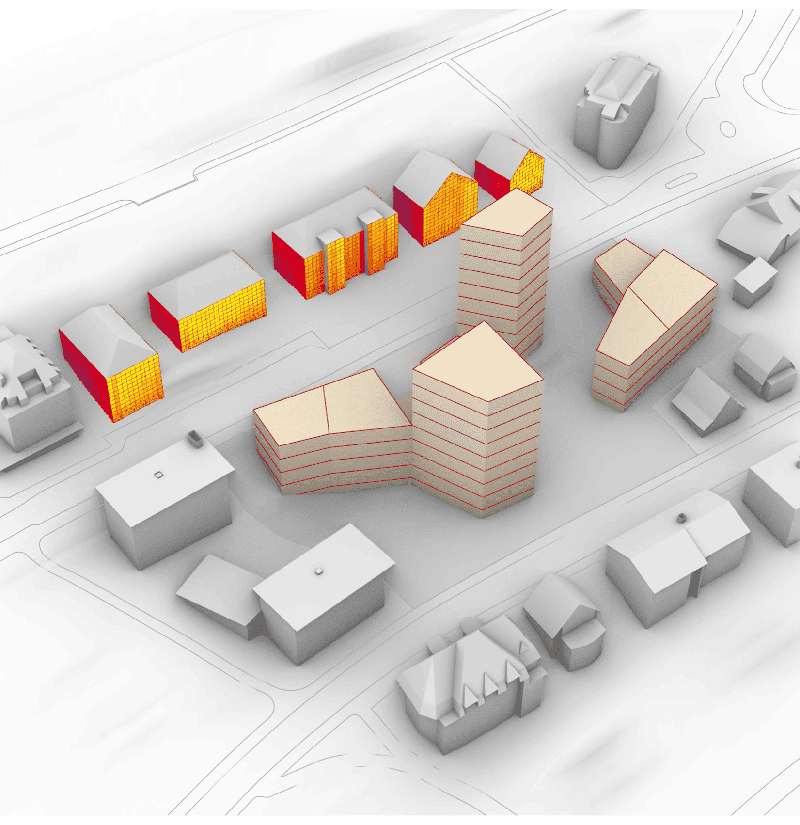
AIXD | Generative AI toolbox for architects and engineers
AIXD | Generative AI toolbox for architects and engineers


Smartair | An active learning algorithm for real-time acquisition and regression of flow field data
Smartair | An active learning algorithm for real-time acquisition and regression of flow field data
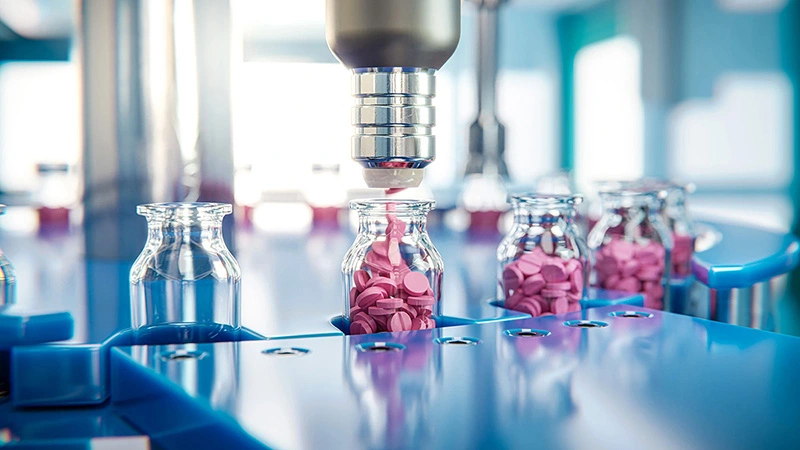
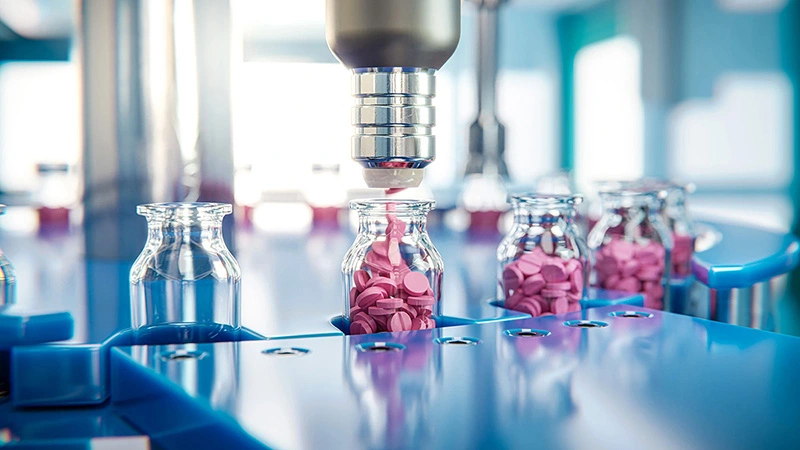
The Promise of AI in Pharmaceutical Manufacturing
The Promise of AI in Pharmaceutical Manufacturing
Contact us
Let’s talk Data Science
Do you need our services or expertise?
Contact us for your next Data Science project!