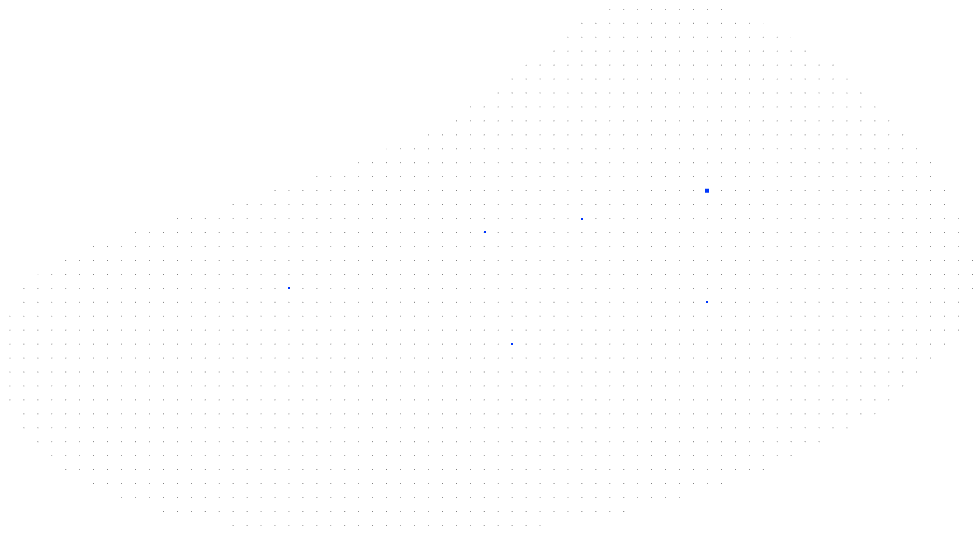
MACH-Flow
Machine learning for Swiss river flow estimation
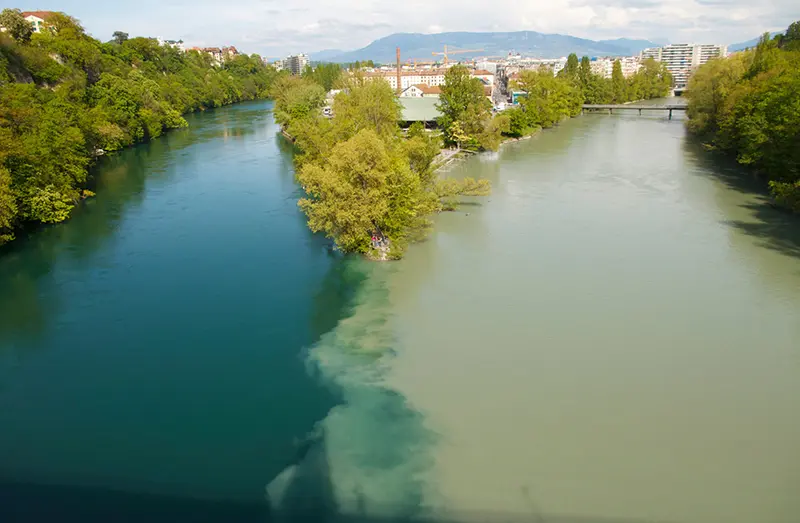
Abstract
River flow is a key component of the terrestrial water cycle and of special relevance for ecosystems and societies. Dependable information on river flow is essential for advancing both environmental research, and water resources management as well as for anticipating and mitigating floods and droughts.
To date, observations at gauging stations constitute the most accurate way to measure this quantity along river systems. However, observation networks only monitor a small number of locations, leaving many gaps on maps of regional river flow.
The proposed project MACH-Flow aims to bridge this gap by advancing our capabilities for estimating daily river flow at ungauged locations in Switzerland using data science methods. To this end, MACH-Flow will expand upon recent advances for modeling water-cycle variables by fusing sparse in situ observations with spatially continuous predictor variables using machine learning.
A special focus will be on making existing methods fit for application at the national scale, by tackling conceptual hurdles that arise when modeling daily river flow at the very high spatial resolution (i.e. 200×200 to 500×500 meters) that is relevant for water management. In particular, the MACH-Flow project will develop a machine-learning based reconstruction of spatially resolved daily river flow covering all of Switzerland. This product will be the first of its type and be relevant for a range of practical and scientific applications.
People
Collaborators


William obtained a PhD in Statistics in 2015 jointly from the University of Geneva and the University of Sydney. He then worked as a post-doctoral research fellow at Dalhousie University as part of a Canadian Statistical Sciences Institute collaborative research team. He was an Assistant Professor of Statistics at Stevens Institute of Technology in Hoboken, New Jersey, before joining the SDSC in September 2020. His research interests include robust statistics, non-parametric methods, and spatio-temporal modeling. His recent cross-disciplinary collaborations involve applications in marine biology, volcanology, and fisheries science.
description
Motivation
Accurately predicting river discharge, in particular at ungauged locations, is crucial for water resource management as well as drought and flood monitoring. Traditional hydrological models are generally calibrated on data from a single river catchment. They perform quite well when forecasting at this particular location (satisfactory temporal prediction) but they often generalize poorly at other locations (unsatisfactory spatial prediction). The main goal of this project was to develop models which can predict in space as well as in time. To this end we made use of data from many river gauging stations within a river network and modeled them jointly.
Proposed Approach / Solution
We developed two models in parallel. The first one is a recurrent neural network with long short-term memory (LSTM) cells, following the hydrological state-of-the-art architectures. Here, all gauging stations are considered independent and they share parameters (Figure 1). An extension we implemented in a follow-up paper involved a loss function based on a generalization of the continuous ranked probability score (negative energy score, tailored for multivariate probabilistic forecasts). This allowed to build ensemble predictions which quantify predictive uncertainty in a reliable way. The second model builds on first principles with an additive model with ridge-penalized cubic B-splines. This model includes engineered features for both short- and long-term effects of precipitation and temperature. We developed an additional independent module which can incorporated to this additive model. This module routes water through a river network, by encoding the latter as a directed graph. We have illustrated with a benchmark hydrological model that such spatio-temporal routing can only improve predictions, and this mainly for more downstream locations (Figure 2). A future research avenue, beyond MACH-Flow, is to combine this water routing module (as graph-based convolutions) with a recurrent neural network.
Impact
First, our LSTM neural network is on par with state-of-the-art hydrological models for Switzerland, albeit with much less inputs. This allowed us to reconstruct river discharge at a national scale back to the early 1960s, which is the earliest time for which precipitation and temperature data products are available. This reconstruction is important for the hydrological community as its low computation cost allows one to test various climate change scenarios. Second, the routing model, released as an independent module, is useful for the community as it can be easily added to any local prediction model to improve downstream predictions. Compared to other routing schemes in the hydrological literature, ours require minimal data inputs and accommodates any space discretization (regular grids or others). Also, it can improve predictions even with discharge data only available at a single station. Finally, a version of our additive model has been added to the ensemble of models used operationally on the drought monitoring platform drought.ch maintained by our partners at WSL.


Presentation
Gallery
Annexe
Additional resources
Bibliography
- Ghiggi, G., Humphrey, V., Seneviratne, S. I., and Gudmundsson, L. (2021). G-RUN ENSEMBLE: A multi-forcing observation-based global runoff reanalysis. Water Resources Research, 57 (5), 1-13. https://doi.org/10.1029/2020WR028787
- Jia, X. et al. (2021). Physics-Guided Recurrent Graph Model for Predicting Flow and Temperature in River Networks. Proceedings of the 2021 SIAM International Conference on Data Mining (SDM), 612-620. The Society for Industrial and Applied Mathematics: https://epubs.siam.org/doi/10.1137/1.9781611976700.69
- Asadi, P., Davison, A. C., and Engelke, S. (2015). Extremes on river networks. Ann. Appl. Stat. 9 (4), 2023-2050. Extremes on river networks: https://projecteuclid.org/journals/annals-of-applied-statistics/volume-9/issue-4/Extremes-on-river-networks/10.1214/15-AOAS863.full
Publications
Related Pages
More projects
MAGNIFY
News
Latest news
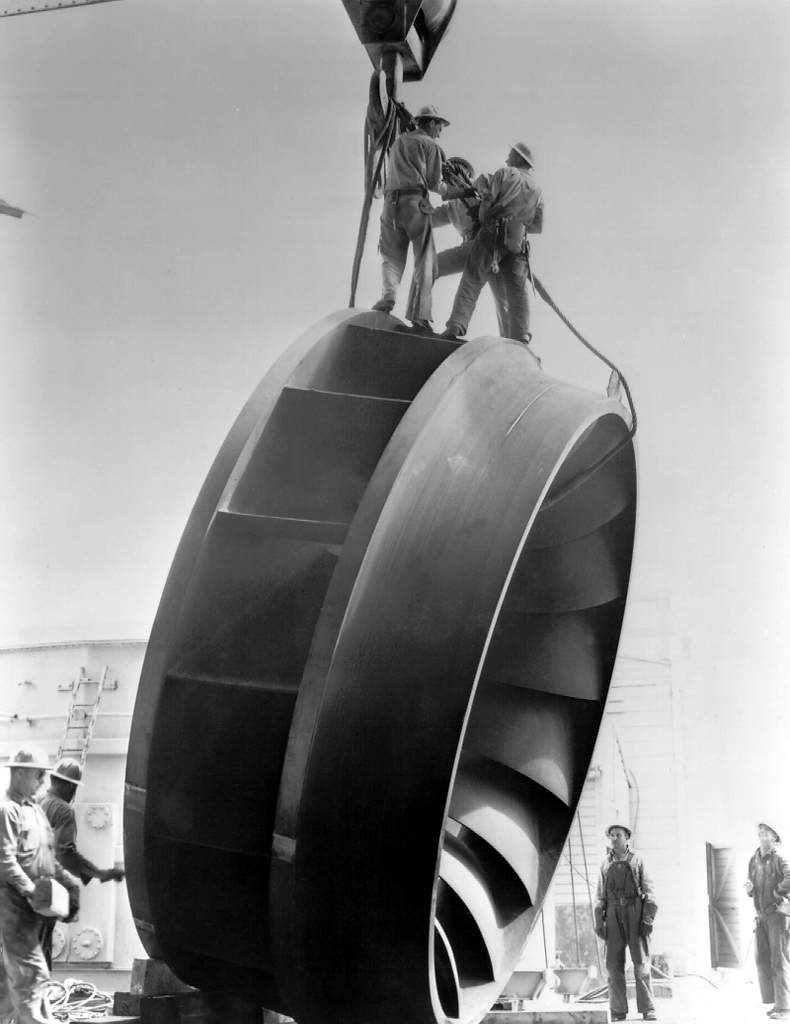
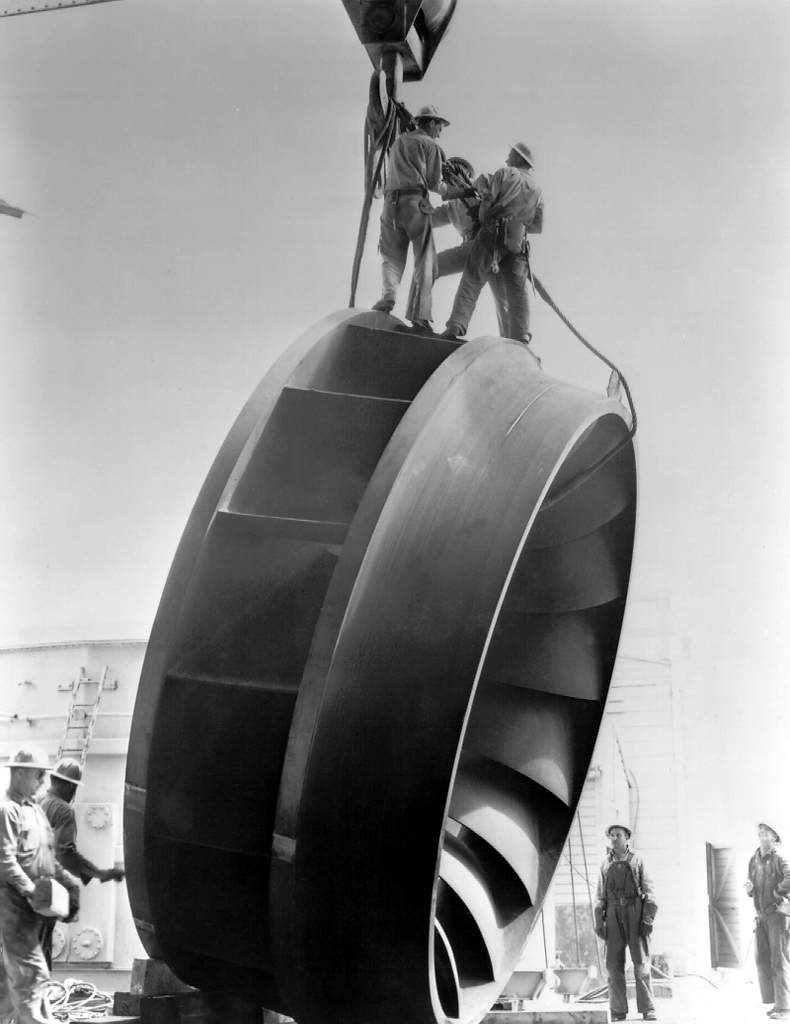
PAIRED-HYDRO | Increasing the Lifespan of Hydropower Turbines with Machine Learning
PAIRED-HYDRO | Increasing the Lifespan of Hydropower Turbines with Machine Learning


First National Calls: 50 selected projects to start in 2025
First National Calls: 50 selected projects to start in 2025
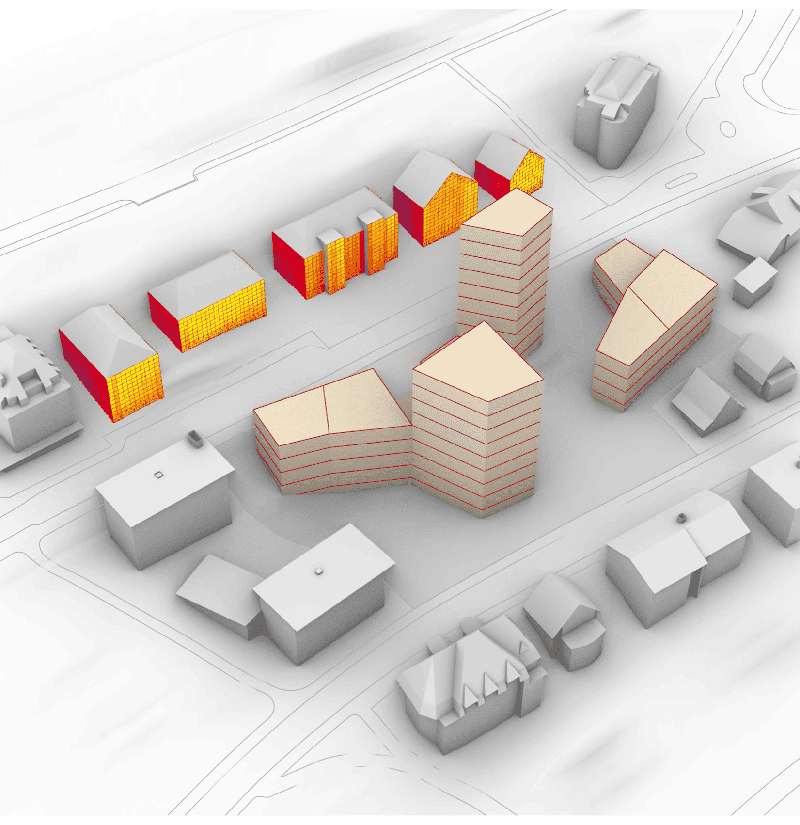
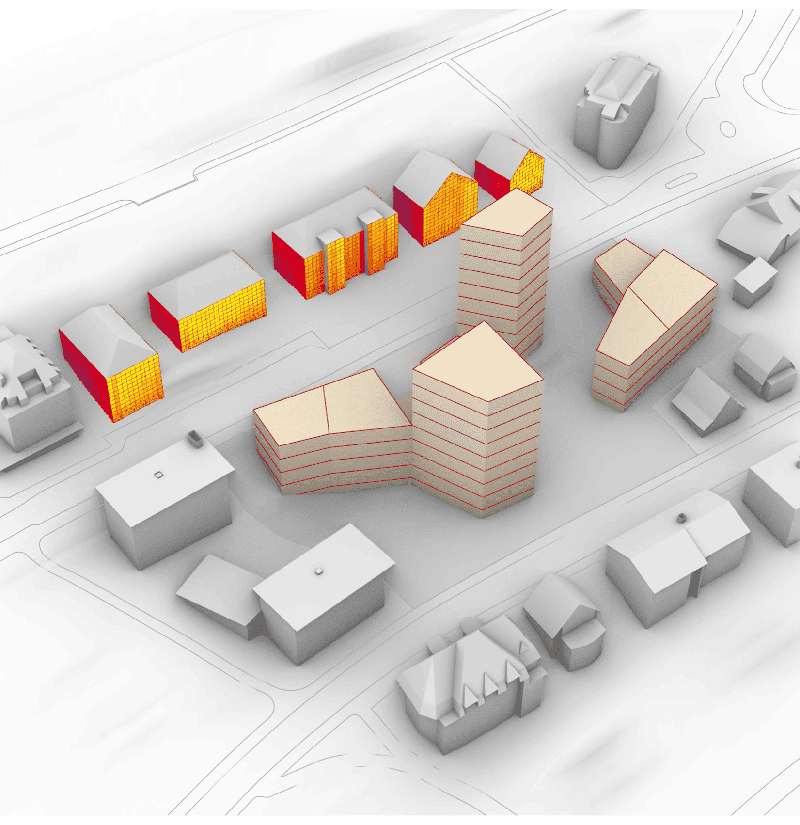
AIXD | Generative AI toolbox for architects and engineers
AIXD | Generative AI toolbox for architects and engineers
Contact us
Let’s talk Data Science
Do you need our services or expertise?
Contact us for your next Data Science project!