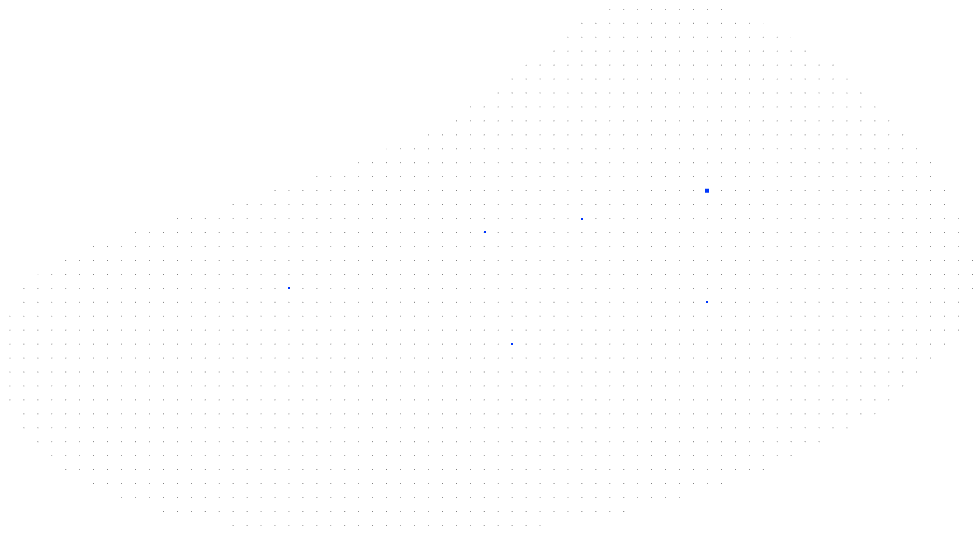
MLTox
Enhancing toxicological testing through machine learning
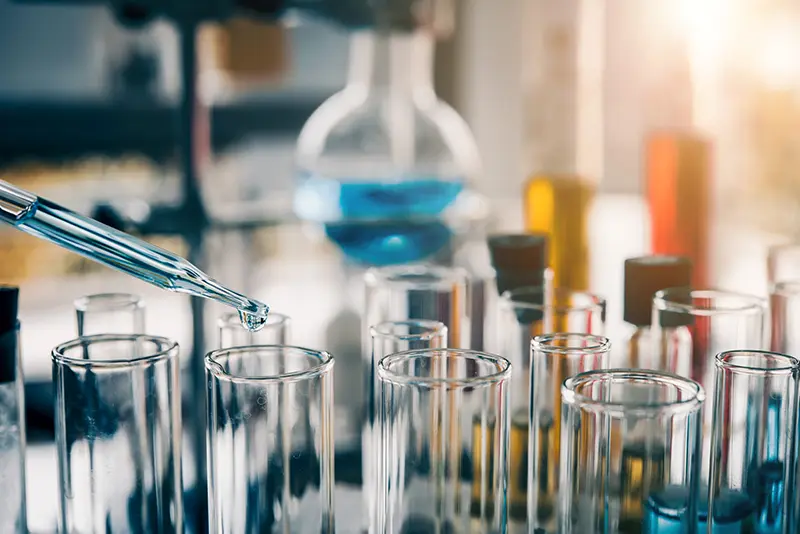
Abstract
We use machine learning (ML) methods to predict the effects of chemicals on aquatic species. Our main goal is to use data from in vivo (whole organisms) experiments to infer the effects of chemicals on organisms for which no testing data is available (both for the chemical and for the organism). In the literature, this kind of problem is also known as across-chemical (and across-species) extrapolation. Usually, extrapolation across chemicals is performed using measures of chemical similarity under the assumption that similar chemicals are similarly toxic to the same species. Extrapolation across species can be performed based on measured chemical effects on some species and the similarity between species, either by phylogenetic distance or sequence/structure similarity of known molecular targets of the chemicals, if at all available, or through similarity in physiological traits. Given the enormous number of chemicals and of potentially affected species, extrapolation of chemical by chemical or species by species is a daunting task. In an interdisciplinary effort by ecotoxicologists and ML experts, we combine thus far unconnected data to obtain predictions of toxicity across chemicals and species. We use a variety of data sources and types, all available in different publicly available tools and databases, combining chemical structure, and data on chemical testing on different organisms.
People
Collaborators
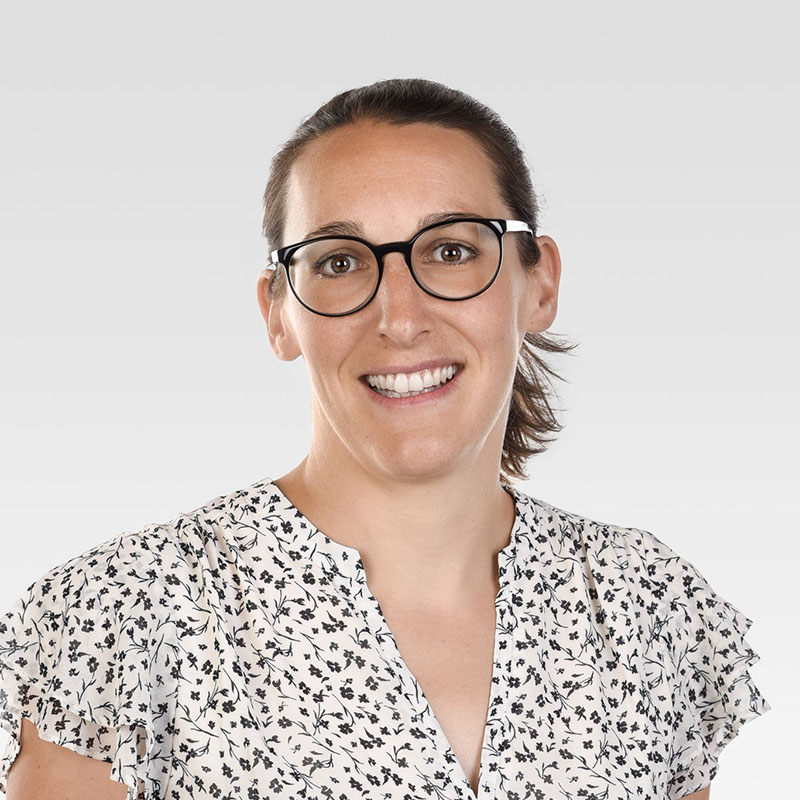
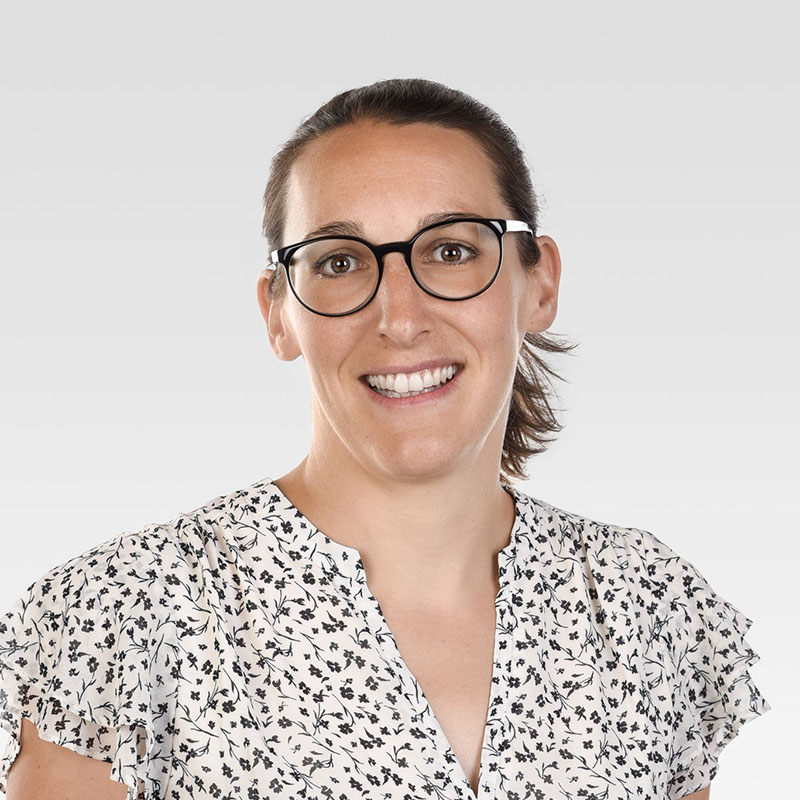
Lili obtained the MSc in Statistics from ETH in 2018. She wrote her Master thesis at the Swiss Data Science Center applying topic modelling to political data. She rejoined the SDSC in May 2020 after a year as a statistical consultant at the Seminar for Statistics at ETH. With her MSc in Chemical Engineering, she worked as a process engineer in the glass industry for several years. She is interested in interdisciplinary projects where data science can help uncover new insights.


Quentin graduated with an engineering degree in mathematics and computer science from École des Ponts ParisTech in 2019. After a 6-month experience at the Center for Data Science of the New York University working on applied Machine Learning for medical imaging, he did a PhD in Statistics at Gustave Eiffel University (Paris). During his PhD, Quentin worked on random graphs and selective inference. His recent cross-disciplinary collaborations involve applications in biology and hydrology.
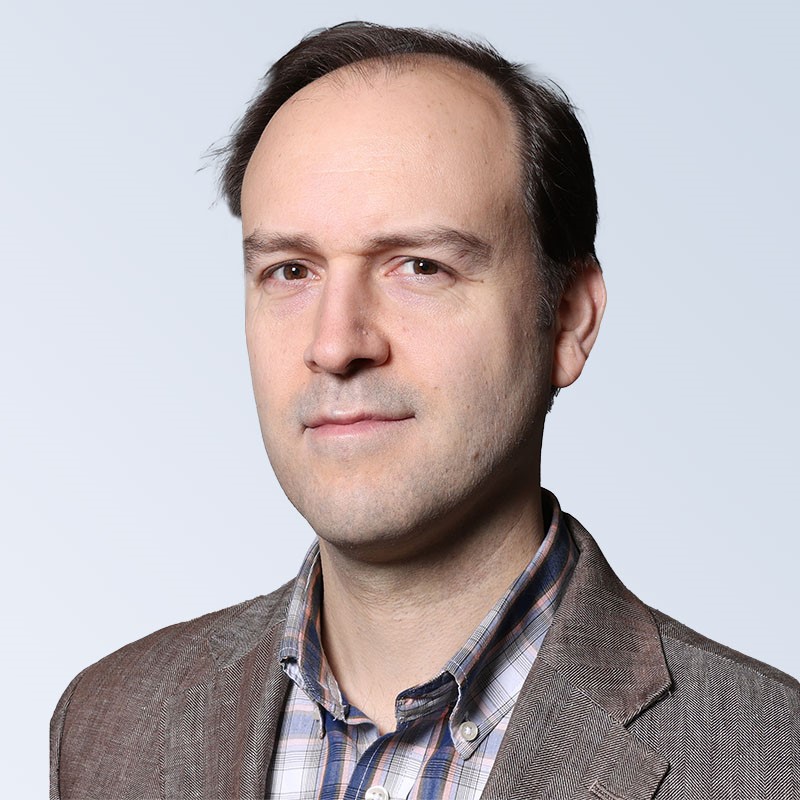
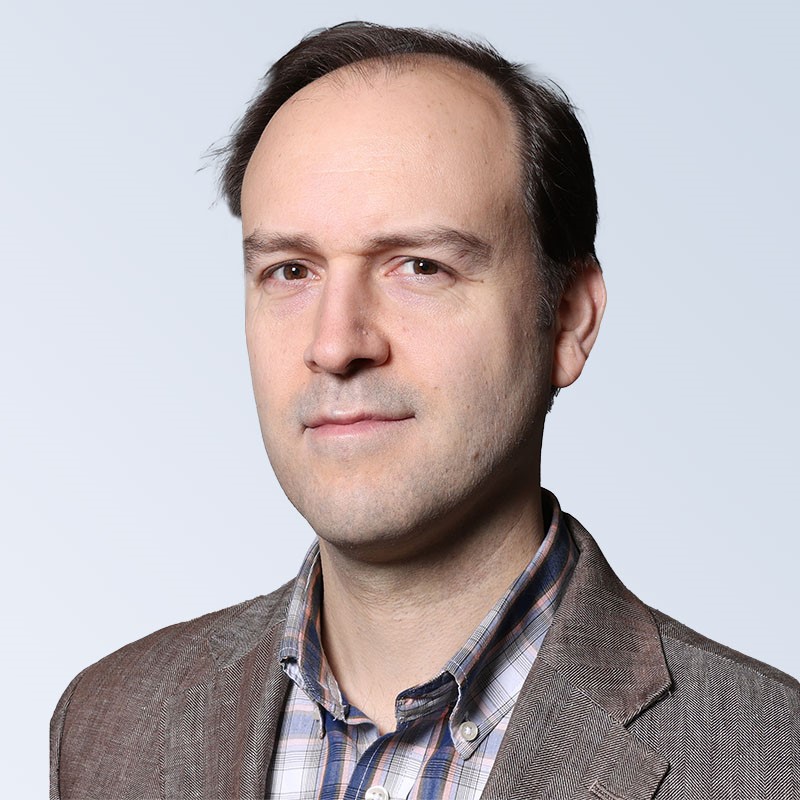
Guillaume Obozinski graduated with a PhD in Statistics from UC Berkeley in 2009. He did his postdoc and held until 2012 a researcher position in the Willow and Sierra teams at INRIA and Ecole Normale Supérieure in Paris. He was then Research Faculty at Ecole des Ponts ParisTech until 2018. Guillaume has broad interests in statistics and machine learning and worked over time on sparse modeling, optimization for large scale learning, graphical models, relational learning and semantic embeddings, with applications in various domains from computational biology to computer vision.
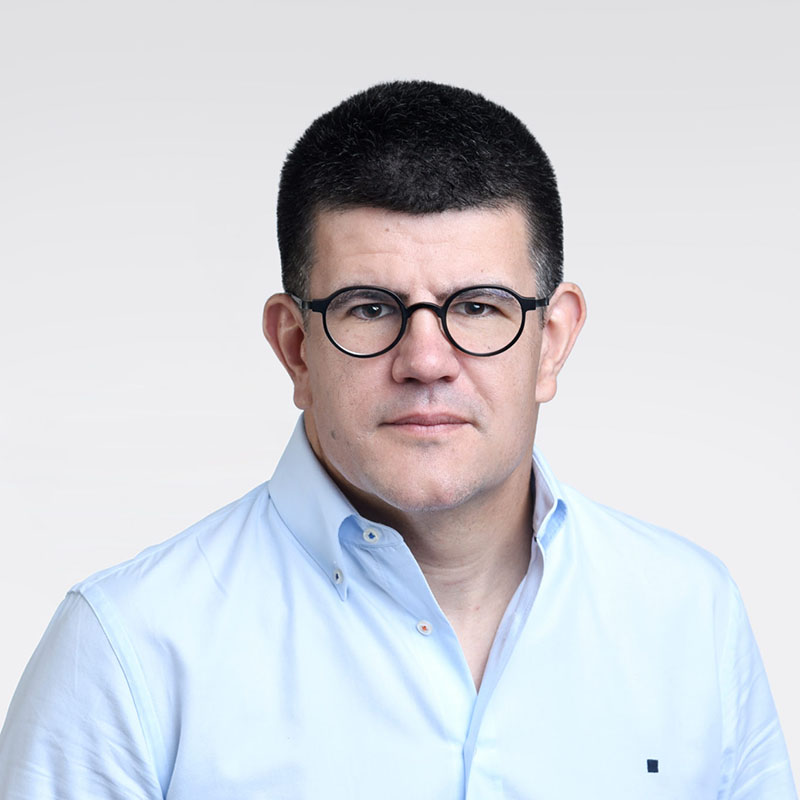
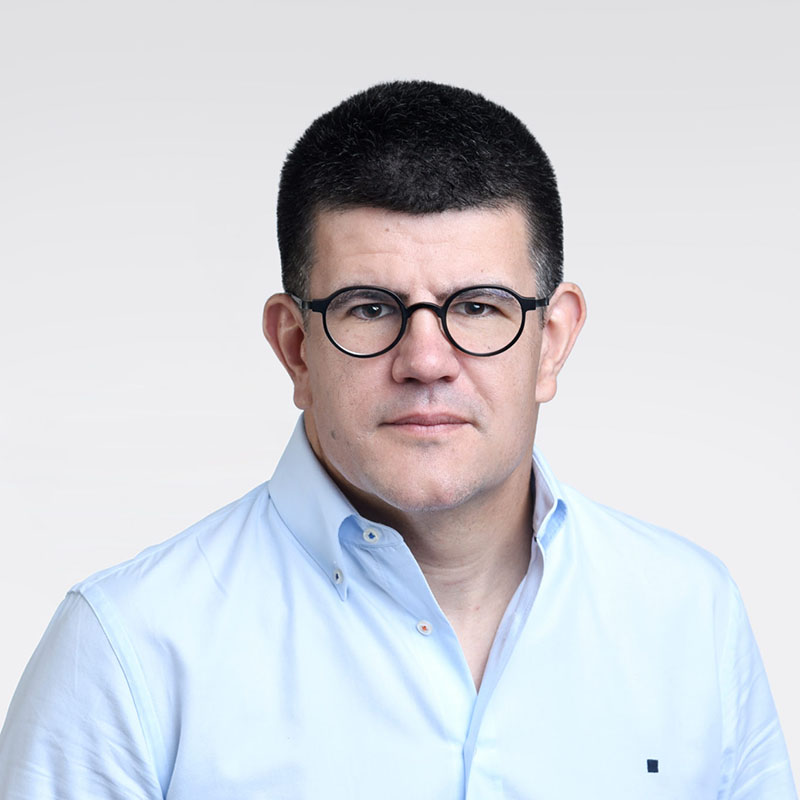
Fernando Perez-Cruz received a PhD. in Electrical Engineering from the Technical University of Madrid. He is Titular Professor in the Computer Science Department at ETH Zurich and Head of Machine Learning Research and AI at Spiden. He has been a member of the technical staff at Bell Labs and a Machine Learning Research Scientist at Amazon. Fernando has been a visiting professor at Princeton University under a Marie Curie Fellowship and an associate professor at University Carlos III in Madrid. He held positions at the Gatsby Unit (London), Max Planck Institute for Biological Cybernetics (Tuebingen), and BioWulf Technologies (New York). Fernando Perez-Cruz has served as Chief Data Scientist at the SDSC from 2018 to 2023, and Deputy Executive Director of the SDSC from 2022 to 2023
description
Motivation
Ecotoxicological testing requires investing large amounts of money, workforce, and time, in addition to the animal suffering from in vivo tests. There are global efforts to reduce or replace animal testing for human and environmental risk assessment for both ethical and feasibility concerns.
Proposed Approach / Solution
As a first step, we have compiled a benchmark dataset on acute mortality of the taxonomic groups fish, crustaceans, and algae, which is intended to be used to train, compare, and benchmark models on. The ADORE dataset has been compiled from several sources and contains data subsets of varying complexity, starting with single-species data, to data within a taxonomic group, to data across taxonomic groups (Figure 1). It has been published as a Nature data descriptor and is openly available.
From the challenges provided in ADORE, we have first focused on fish acute mortality by training standard ML models such as LASSO, random forest and XGBoost and comparing them to more elaborate models. Simultaneously, we have usedour models to gain better understanding of the nonlinear relationships that connect species, chemicals, and the related toxicity.
Impact
We provide new means to protect the environment from toxicants by combining ML and in vivo data. This is valuable in the discussion between toxicologist, regulators, and data scientists to progress in reducing experiments on animals.
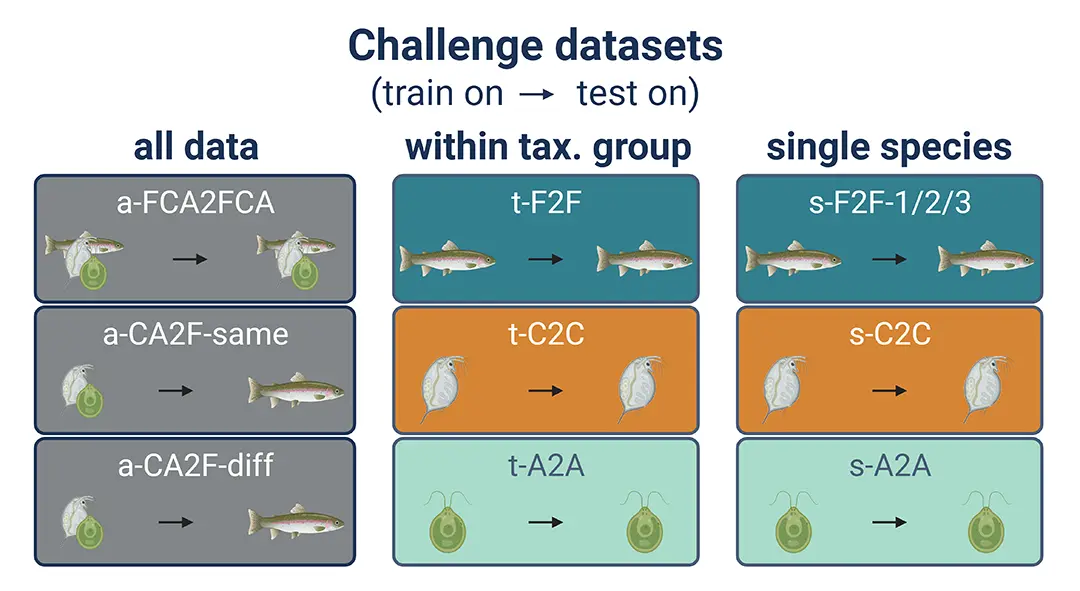
Presentation
Gallery
Annexe
Dataset, posters and talks
- Dataset: ADORE Reproducible Data Science | Open Research | Renku
- Poster: Schür C., Gasser L., Wu J., Perez-Cruz F., Schirmer K., Baity-Jesi M. (2022) Preparation and characterization of a benchmark data set for machine learning in ecotoxicology, Swiss Society of Toxicology, SST Annual Meeting 2022, 17. November, Basel
- Poster: Schür C., Gasser L., Perez-Cruz F., Schirmer K., Baity-Jesi M. (2023) A Benchmark Dataset for Machine Learning in Ecotoxicology. 33rd SETAC Europe Annual Meeting, Dublin, Ireland.
- Talk: Schür C., Gasser L., Perez-Cruz F., Schirmer K., Baity-Jesi M. (2023) Predicting Ecotoxicity across Taxa through Machine Learning. 33rd SETAC Europe Annual Meeting, Dublin, Ireland.
- Poster: Schür C., Gasser L., Wu J., Perez-Cruz F., Schirmer K., Baity-Jesi M. (2023) Machine learning for predictive ecotoxicology in fish, Swiss Society of Toxicology, SST Annual Meeting 2023, 16. November, Basel
Additional resources
Bibliography
- Luechtefeld, T., Marsh, D., Rowlands, C., & Hartung, T. (2018). Machine learning of toxicological big data enables read-across structure activity relationships (RASAR) outperforming animal test reproducibility. Toxicological Sciences, 165(1), 198-212. Machine Learning of Toxicological Big Data Enables Read-Across Structure Activity Relationships (RASAR) Outperforming Animal Test Reproducibility
Publications
Related Pages
- Official project page: Eawag: MLTox
More projects
MAGNIFY
News
Latest news
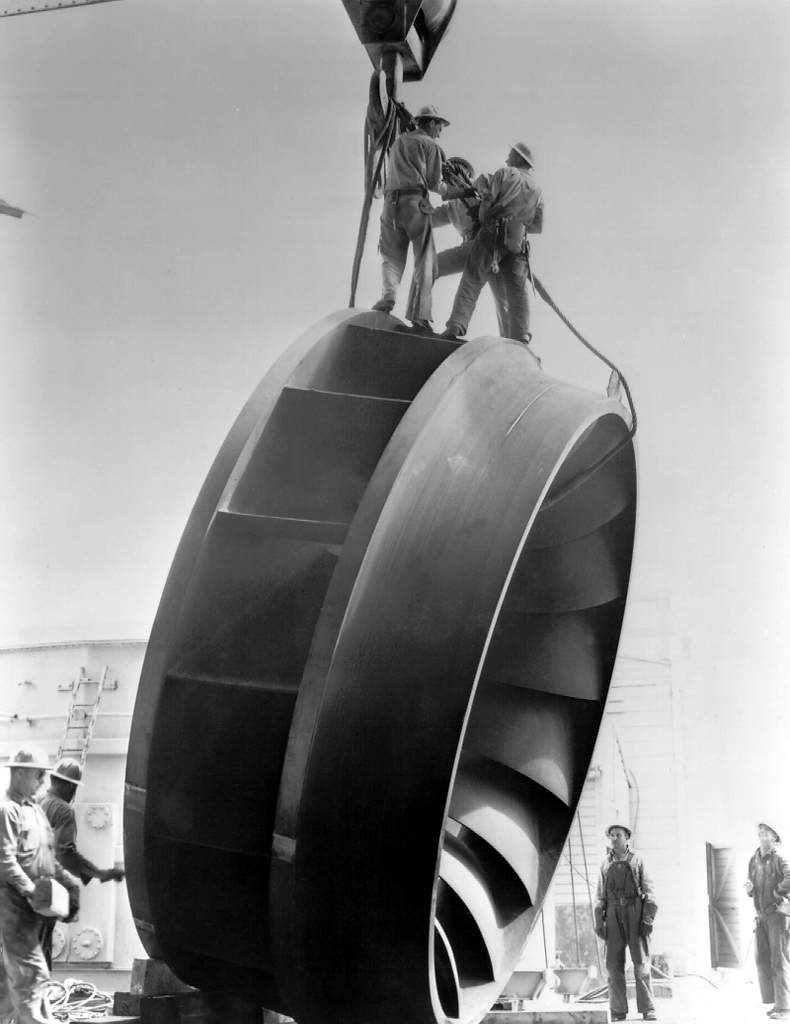
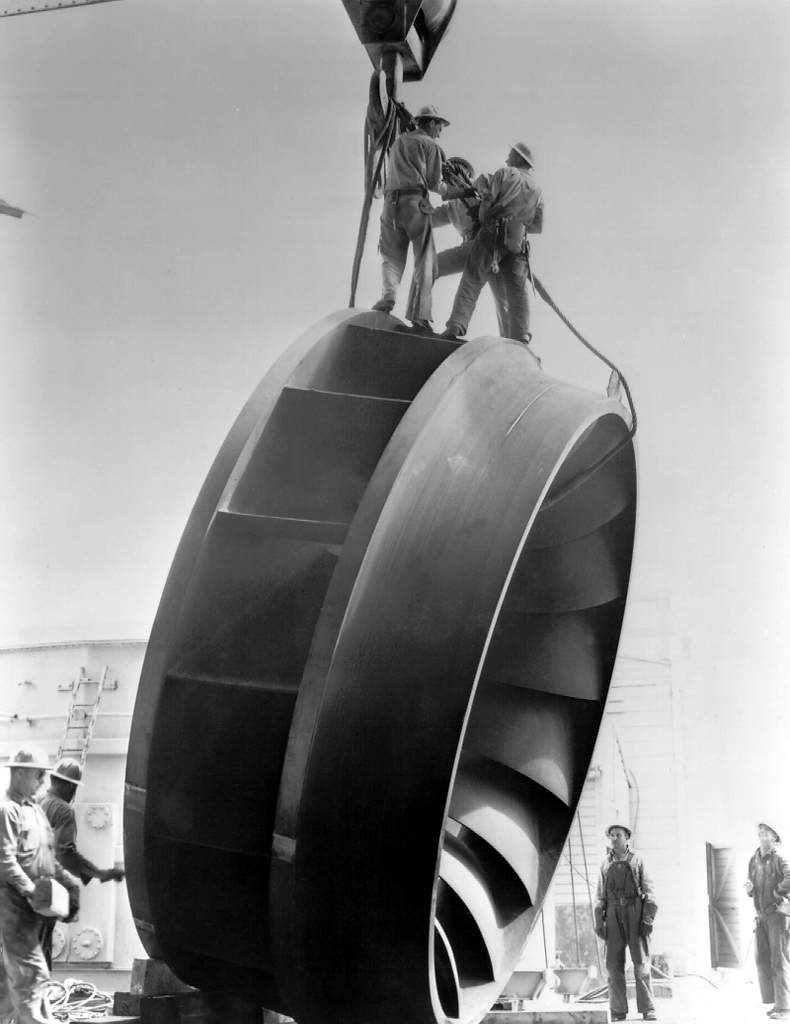
PAIRED-HYDRO | Increasing the Lifespan of Hydropower Turbines with Machine Learning
PAIRED-HYDRO | Increasing the Lifespan of Hydropower Turbines with Machine Learning


First National Calls: 50 selected projects to start in 2025
First National Calls: 50 selected projects to start in 2025
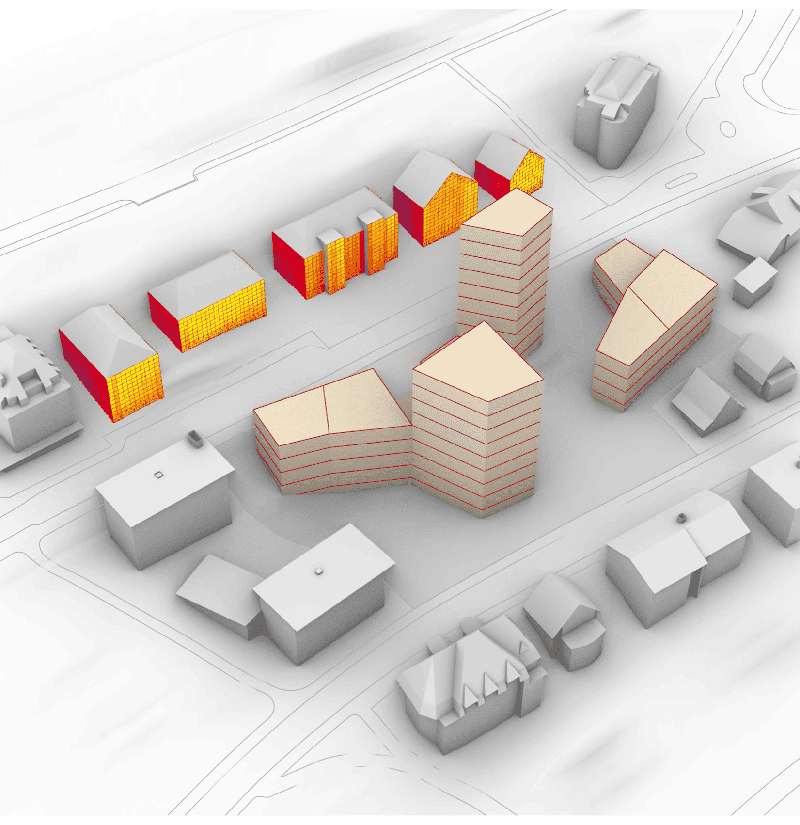
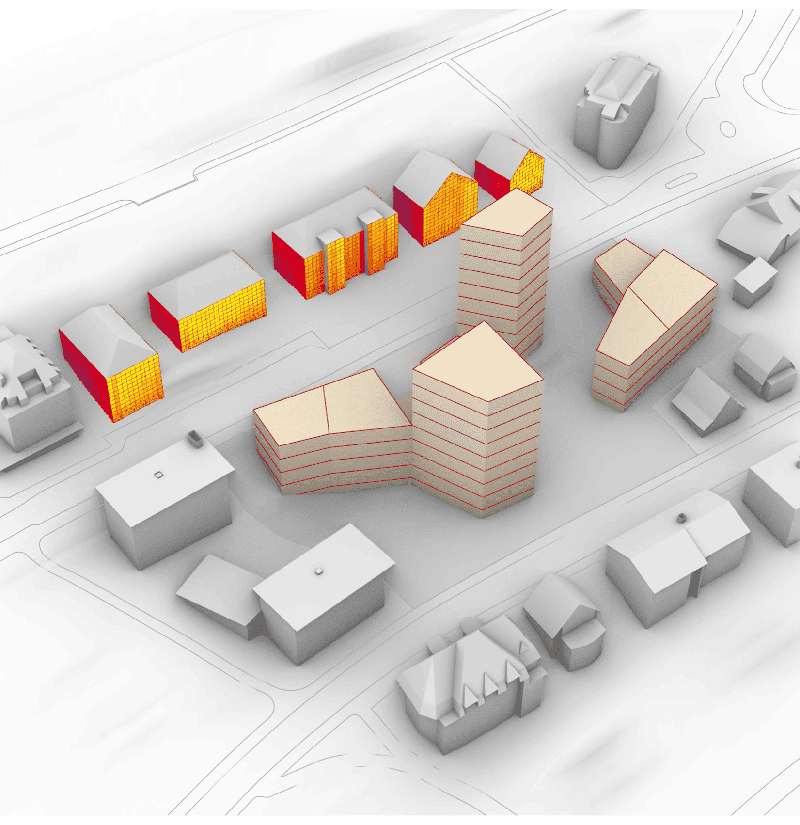
AIXD | Generative AI toolbox for architects and engineers
AIXD | Generative AI toolbox for architects and engineers
Contact us
Let’s talk Data Science
Do you need our services or expertise?
Contact us for your next Data Science project!