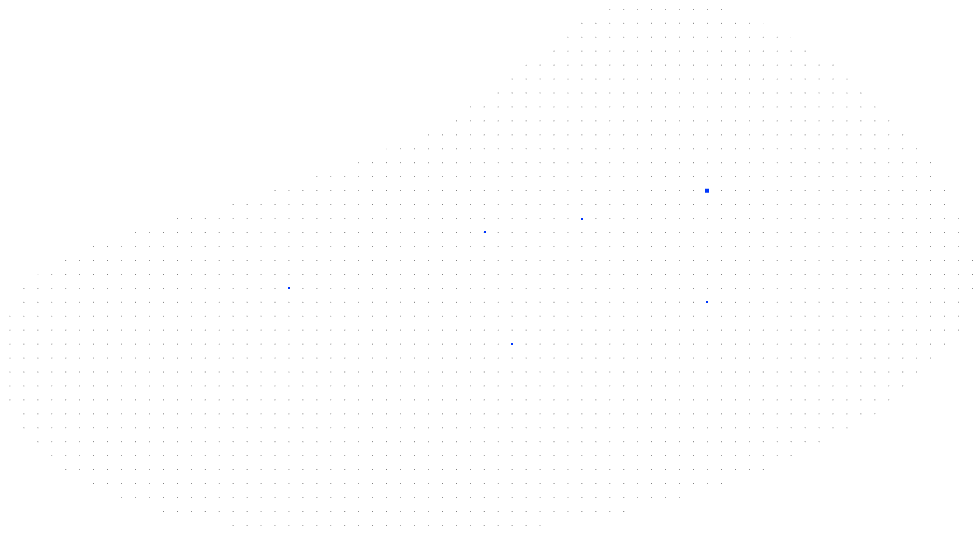
DASH
DAta Science-informed attribution of changes in the Hydrological cycle

Abstract
The main goal of the DASH (“DAta Science-informed attribution of changes in the Hydrologic cycle“) project is to improve the detection and attribution of climate change by incorporating data science and statistical learning methods. Detection and attribution (D&A) is a key methodology in climate science whose aim is to study the causal links between external drivers of the climate system and observed changes in climate variables (IPCC 2021). Improving our understanding of the factors that contribute to climate changes, such as anthropogenic emissions or natural variability, is of great relevance to society in order to anticipate and adapt to such changes, both on a global scale and regionally, for example in Switzerland. According to the IPCC standard terminology detection is the process of determining whether there is an observed change in a climate variable that cannot be explained by internal variability alone but without providing a reason for that change, while attribution is the process of providing a likely reason for the detected change. While it is well established that global-scale climate warming over the last decades and century is driven by human emissions of greenhouse gases and aerosols (e.g., see the most recent IPCC report: Sixth Assessment Report), important gaps of our understanding remain when it comes to climate changes on a regional scale (e.g., can warming or changes in the water cycle over Switzerland be attributed with high confidence to anthropogenic climate change?), or on short time scales (do extreme events, or daily patterns of climate variables, carry a signal of climate change?).
People
Collaborators
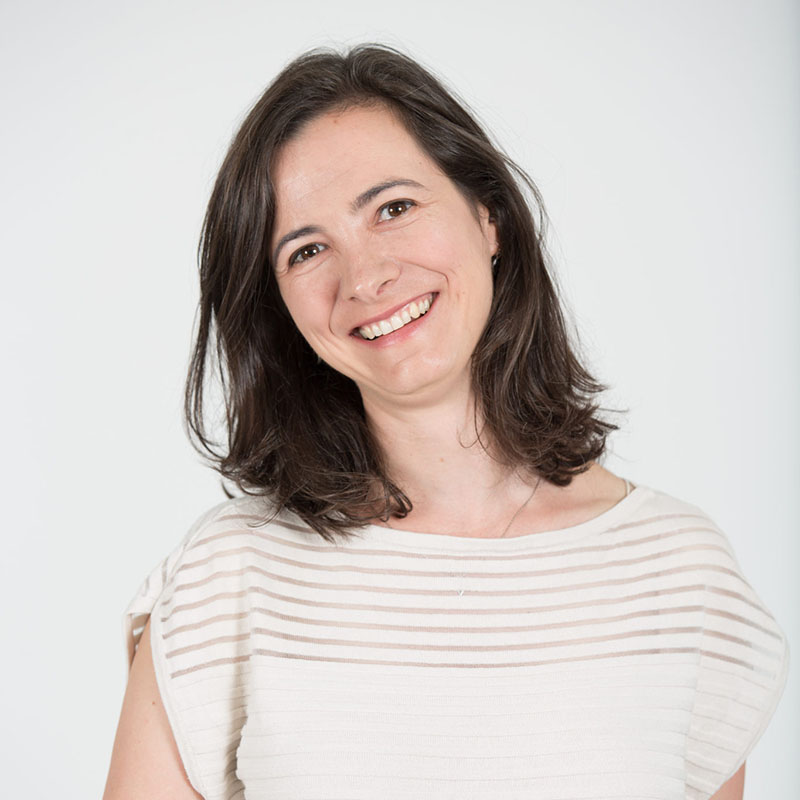
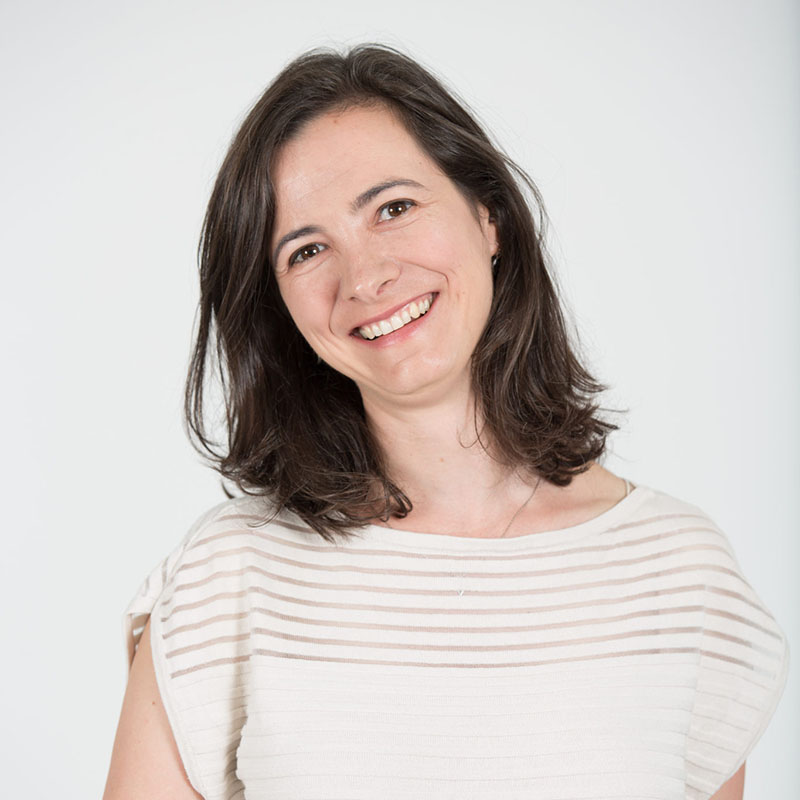
Eniko joined SDSC as a senior data scientist in September 2017. Previously, she was a postdoctoral researcher at the Courant Institute of Mathematical Sciences, New York University, working on machine learning for dynamical systems and climate science. She obtained her PhD in Computer Science from the University of Geneva, Switzerland (2011), her MSc from Telecom Bretagne, France (2006) and her BSc from the University of Timisoara, Romania (2005). Broadly she is interested in machine learning for nonlinear phenomena and high-dimensional data, and more recently she has been working on using machine learning approaches to advance our understanding of the climate.
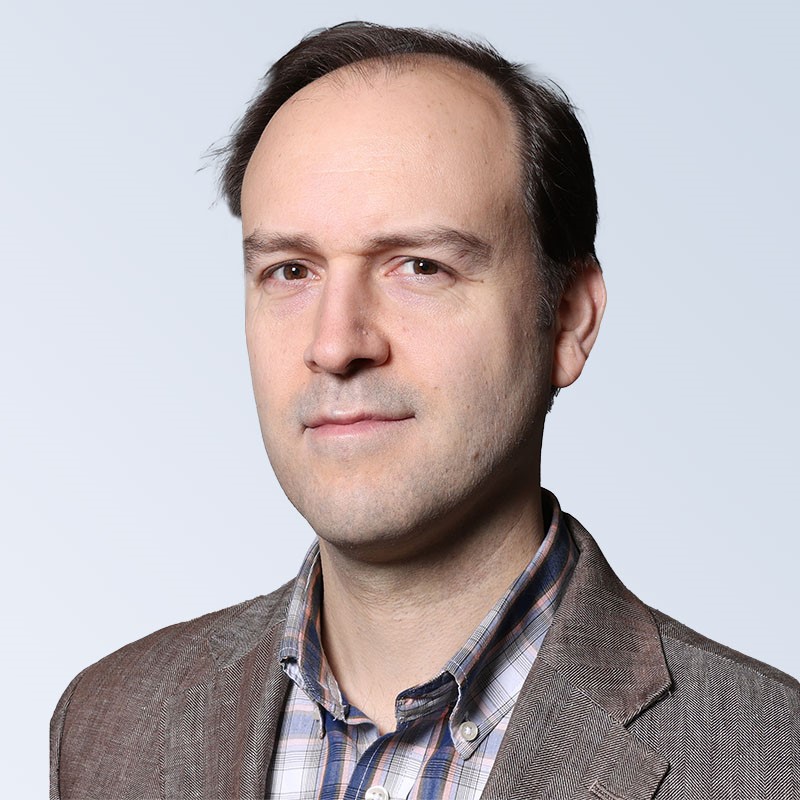
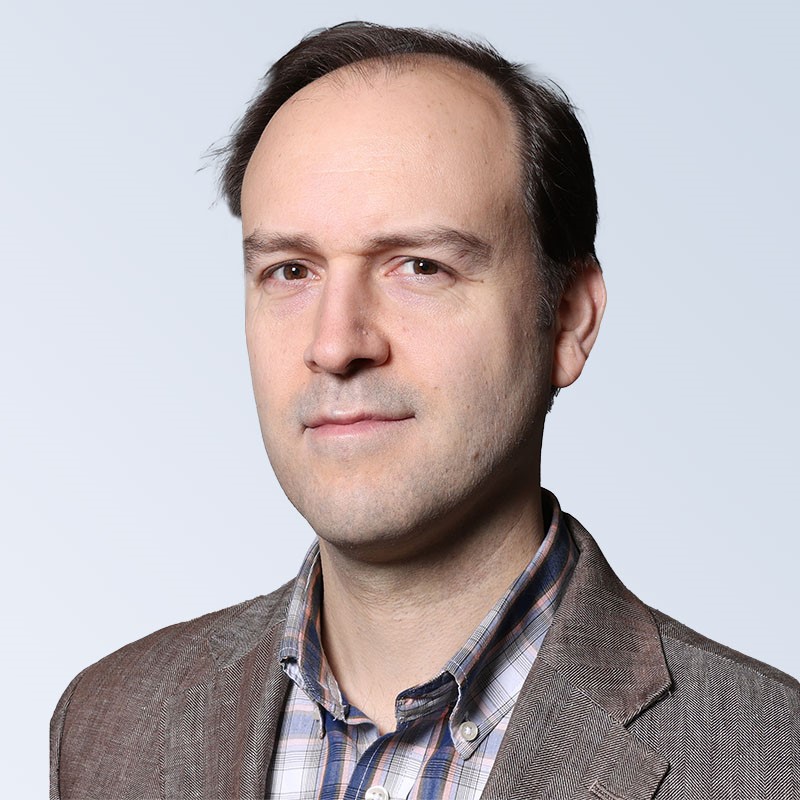
Guillaume Obozinski graduated with a PhD in Statistics from UC Berkeley in 2009. He did his postdoc and held until 2012 a researcher position in the Willow and Sierra teams at INRIA and Ecole Normale Supérieure in Paris. He was then Research Faculty at Ecole des Ponts ParisTech until 2018. Guillaume has broad interests in statistics and machine learning and worked over time on sparse modeling, optimization for large scale learning, graphical models, relational learning and semantic embeddings, with applications in various domains from computational biology to computer vision.
description
Goal:
Advance the detection and attribution (D&A) of climate change by incorporating advanced machine learning, statistical learning and data science methods.
Impact:
Advance the state-of-the-art on D&A techniques using data science, to finally guide climate model development based on the agreement or mismatch of models and observations, and improve the confidence in the detection and attribution statements.
Proposed approach:
Incorporate new statistical techniques for D&A using machine learning and causal inference to achieve robust predictions. We developed a novel detection and attribution methodology based on supervised statistical learning that uses explicitly the information contained in a target of interest to identify “fingerprints” of climate change (see Figure attached for a temperature fingerprint) that are representative of the target, such as anthropogenic or volcanic fingerprints. The methodology relies on a hypothesis testing framework and uses a statistical learning techniques inspired from causal inference that incorporates robustness constraints. These constraints help to increase the confidence in the detection and attribution statements. This methodology was used for both attribution of greenhouse gases and aerosols, and for detection to make it robust even under potentially large internal climate variability, a remaining long standing issue in climate science. In parallel work, we showed that a climate change signal can be detected from daily patterns of temperature and humidity at the global scale for every day since 2012. On a different line of work, we refined a traditional climate science method (“dynamical adjustment”) using data science, and we show that this allows to uncover the forced climate response as a residual from circulation-induced variability.
Presentation
Gallery

Annexe
Publications
- Sippel, S., Meinshausen, N., Székely, E., Fischer, E., Pendergrass, A. G., Lehner, F., and Knutti, R. (2021) Robust detection of forced warming in the presence of potentially large climate variability, Science Advances 7, eabh4429.
- Heinze-Deml, C., Sippel, S., Pendergrass, A. G., Lehner, F., and Meinshausen, N. (2020) Latent Linear Adjustment Autoencoders v1.0: A novel method for estimating and emulating dynamic precipitation at high resolution. Geoscientific Model Development 14, 4977-4999, doi:10.5194/gmd-2020-275
- Sippel, S., Fischer, E. M., Scherrer, S., Meinshausen, N., and Knutti, R. (2020) Late 1980s abrupt climatic change in Europe consistent with circulation variability and long-term warming, Environmental Research Letters 15, 094056, doi:10.1088/1748-9326/ab86f2
- Vignotto, E., Sippel, S., Lehner, F., and Fischer, E. M., (2020) Towards dynamical adjustment of the full temperature distribution. Proceedings of the 10th International Conference on Climate Informatics, 52-59, doi:10.1145/3429309.3429317
- Wills, R. J., Sippel, S., and Barnes, E. A. (2020) Separating forced and unforced components of climate change: The utility of pattern recognition methods in Large Ensembles and observations. CLIVAR Variations Newsletter 18(2), 1-8, doi:10.5065/0DSY-WH17
- Sippel, S., Meinshausen, N., Fischer, E. M., Szekely, E., and Knutti, R. (2020) Climate change now detectable from any single day of global weather. Nature Climate Change 10, 35-41, doi:10.1038/s41558-019-0666-7
See also News&Views: doi:10.1038/s41558-019-0670-y - Székely, E., Sippel, S., Knutti, R., Obozinski, G. and Meinshausen, N. (2019) A direct approach to detection and attribution of climate change. In Brajard, J., Charantonis, A., Chen, C., & Runge, J. (Eds.), Proceedings of the 9th International Workshop on Climate Informatics: CI 2019 (No. NCAR/TN-561+PROC), 119-124, doi:10.5065/y82j-f154. See also arXiv preprint, arXiv:1910.03346
- Sippel, S., Meinshausen, N., Merrifield, A., Lehner, F., Pendergrass, A. G., Fischer, E. M., and Knutti, R. (2019) Uncovering the forced climate response from a single ensemble member using statistical learning. Journal of Climate 32, 5677-5699, doi:10.1175/JCLI-D-18-0882.1
Media:
- ETH Zurich | Climate signals detected in global weather
- EPFL | Fingerprint of climate change detected in daily weather
- National Geographic | Weather shows evidence of climate change every single day since 2012
- Washington Post | The signal of human-caused climate change has emerged in everyday weather, study finds
- CBS News | Scientists detect ''fingerprint'' of human-induced climate change on weather
- Weather.com | Daily weather now reveals the global fingerprint of climate change, study says
- Tagesanzeiger | Klimawandel ist jetzt täglich messbar
- Spektrum | Klima und Wetter – Können wir den Klimawandel nun auch im täglichen Wetter feststellen?
- Spiegel Online | Klimawandel erstmals täglich im weltweiten Wetter nachweisbar
Code:
Videos
Additional resources
Bibliography
- Hasselmann, K. F. On the signal-to-noise problem in atmospheric response studies. In Joint Conference of Royal Meteorological Society, American Meteorological Society, Deutsche Meteorologische Gesellschaft and the Royal Society (ed. Shaw, D. B.) 251–259 (Royal Meteorological Society, 1979)
- C. Deser, R. Knutti, S. Solomon, and A. S. Phillips. Communication of the role of natural variability in future North American climate. Nature Climate Change, 2, 775-779, DOI: https://doi.org/10.1038/nclimate1562 (2012).
- D. Rothenhäusler, N. Meinshausen, P. Bühlmann, J. Peters. Anchor regression: Heterogeneous data meet causality. Journal of the Royal Statistical Society: Series B (Statistical Methodology), Vol. 83 (2), pages 215-246, https://rss.onlinelibrary.wiley.com/doi/abs/10.1111/rssb.12398 (2021)
Publications
Related Pages
More projects
ML-L3DNDT
BioDetect
News
Latest news
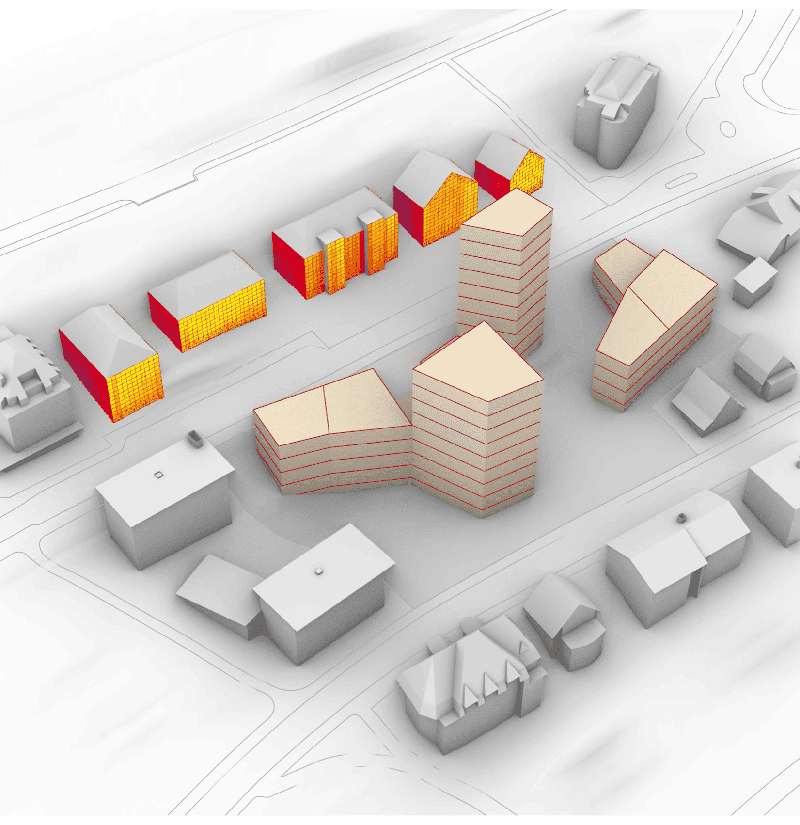
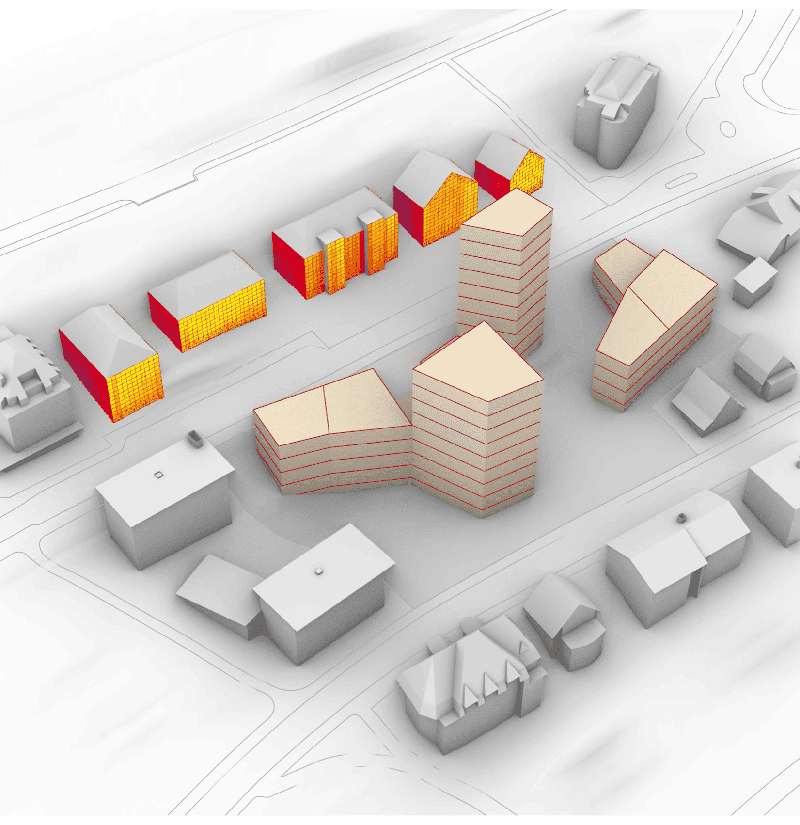
AIXD | Generative AI toolbox for architects and engineers
AIXD | Generative AI toolbox for architects and engineers


Smartair | An active learning algorithm for real-time acquisition and regression of flow field data
Smartair | An active learning algorithm for real-time acquisition and regression of flow field data
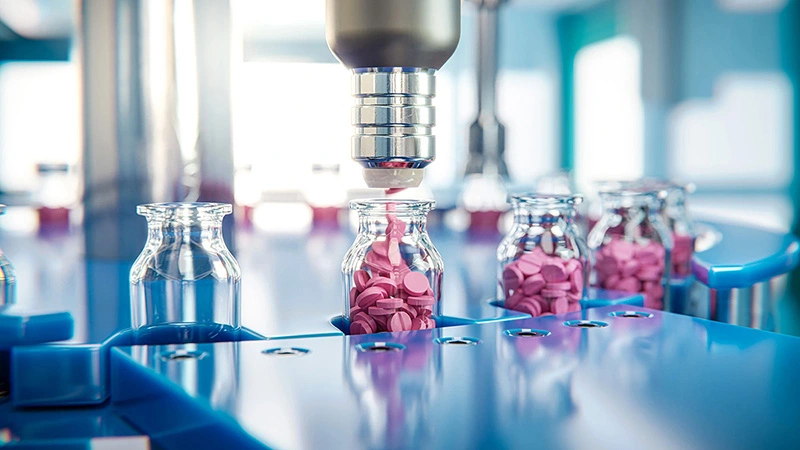
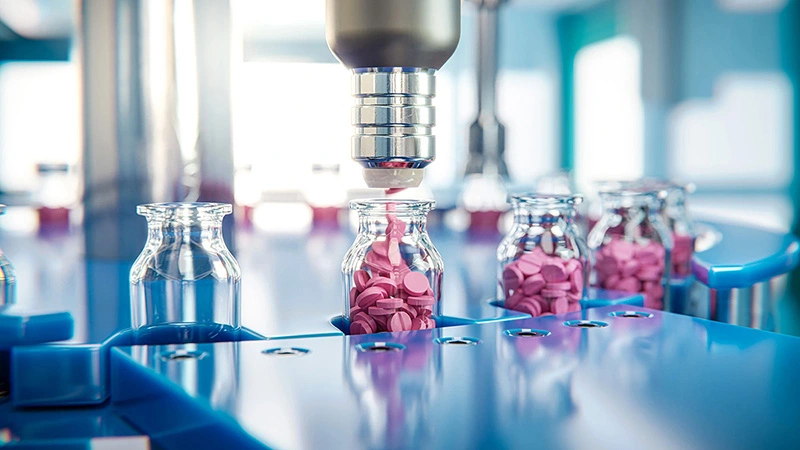
The Promise of AI in Pharmaceutical Manufacturing
The Promise of AI in Pharmaceutical Manufacturing
Contact us
Let’s talk Data Science
Do you need our services or expertise?
Contact us for your next Data Science project!