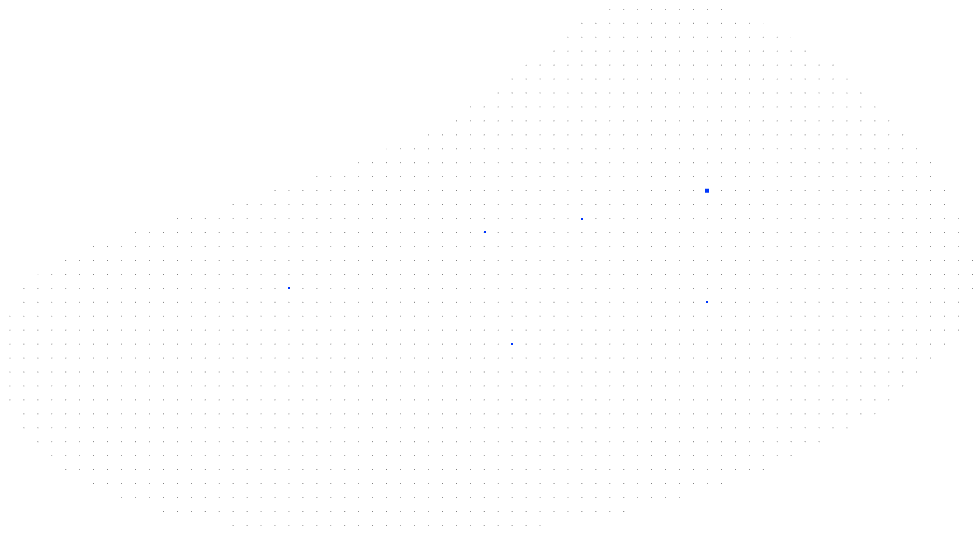
DATALAKES
Heterogeneous data platform for operational modelling and forecasting of Swiss lakes

Abstract
The objective of this project is to advance the forecasting capabilities of the data-driven hydrological and ecological lake modeling algorithms using methodologies inspired by data science and accelerated by high performance computing.
We aim to develop a parallel framework interfacing high resolution 3D numerical solvers for the underlying lake dynamics with modern numerical Markov Chain Monte Carlo sampling methods for Bayesian inference, with particular interest in investigating particle filtering and multi-level variance reduction methodologies. The resulting framework aims at accurate data assimilation and uncertainty quantification in both model parameters and the associated forecasts.
DATALAKES project is a collaboration with the Swiss Data Science Center (SDSC), EPF Lausanne and ETH Zurich, aiming at a sensor-to-frontend data platform providing and analyzing the dynamics of lake ecosystems at high spatial and temporal resolutions. Current version of the existing framework can be found at meteolakes.ch.
People
Collaborators


Eric received his PhD degree in Electrical Engineering from Columbia University, New York, in June 1999. Eric Bouillet has been working at IBM T.J. Watson Research Center, Hawthorne, NY since June 2004, and at the IBM Smarter City Technical Centre, Dublin from October 2010 to August 2016. While at IBM he has been working on scalable data stream analytics applied to a number of fields, including finances, law-enforcement, telecommunications, environmental monitoring, intelligent transport systems, and aircraft reliability control systems. Before joining IBM Research, Eric Bouillet was at Tellium, Oceanport, NJ where he was part of the research team who invented and designed the first commercial optical mesh restoration network (deployed nationwide and documented in their book Path Routing in Mesh Optical Networks), and at Lucent Technologies’ Mathematical Science Center in the department of Mathematics of Networks and System Research department where he worked on the design optimization and sizing of circuit and packet switched networks.
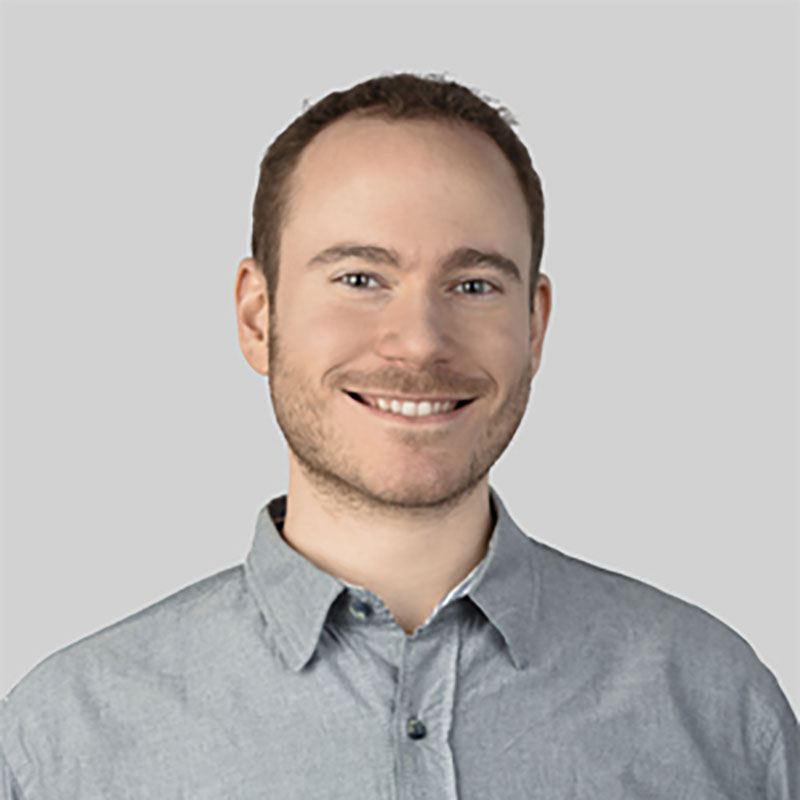
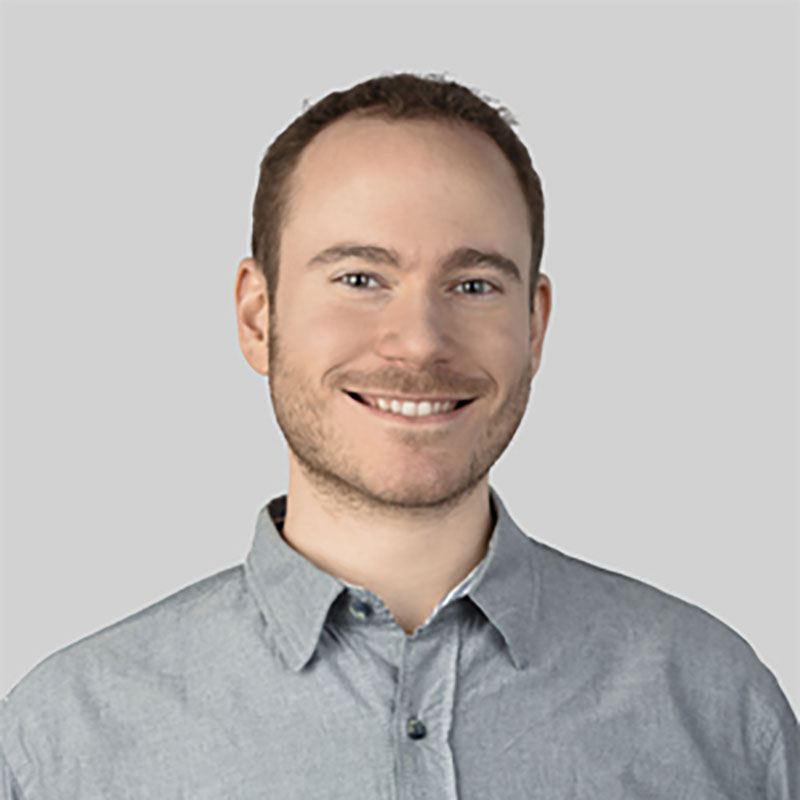
Firat completed his undergraduate studies in Electronics Engineering at Sabanci University. He later received his MSc. in Electrical and Electronics Engineering from EPFL. He conducted his doctoral studies on medical image segmentation in Computer Vision Lab at ETH Zurich. In between, he visited INRIA (Sophia Antipolis, France) and ABB Corporate Research Center (Baden, Switzerland). His research interests revolve around computer vision and machine learning, with a focus on the medical domain. He has been with SDSC since 2019.
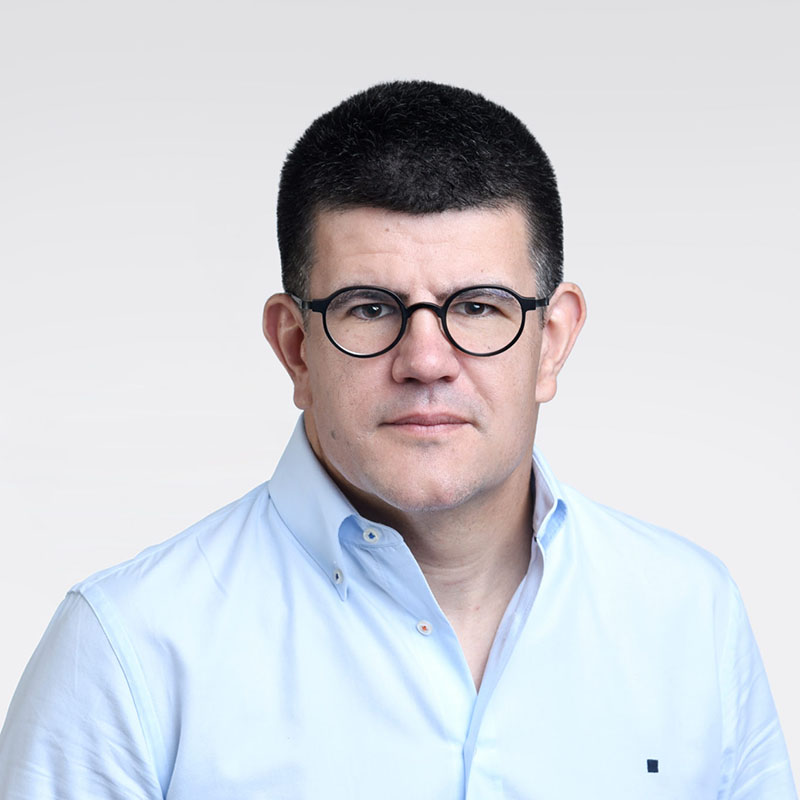
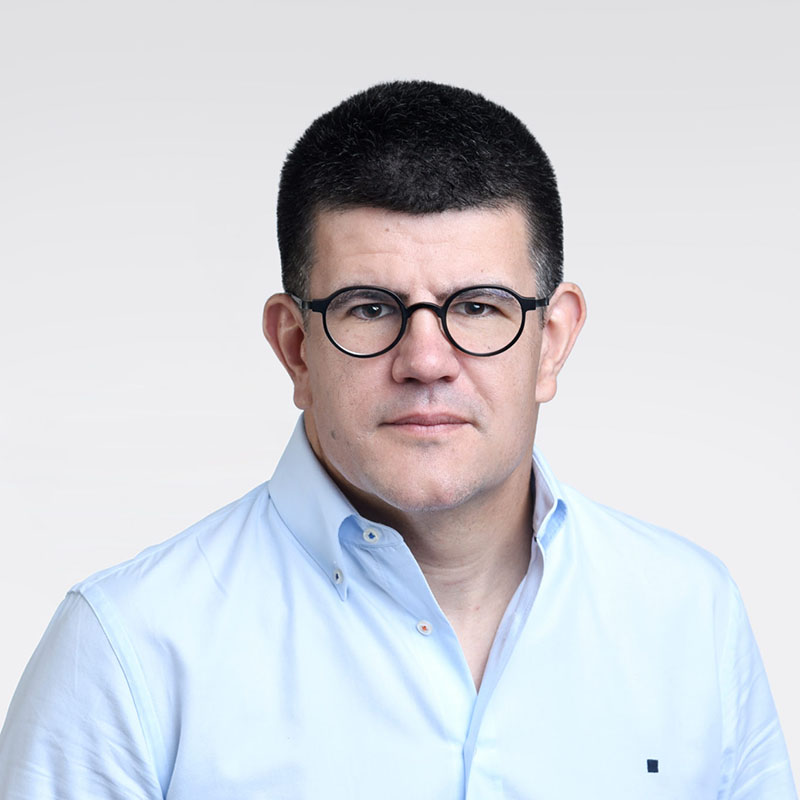
Fernando Perez-Cruz received a PhD. in Electrical Engineering from the Technical University of Madrid. He is Titular Professor in the Computer Science Department at ETH Zurich and Head of Machine Learning Research and AI at Spiden. He has been a member of the technical staff at Bell Labs and a Machine Learning Research Scientist at Amazon. Fernando has been a visiting professor at Princeton University under a Marie Curie Fellowship and an associate professor at University Carlos III in Madrid. He held positions at the Gatsby Unit (London), Max Planck Institute for Biological Cybernetics (Tuebingen), and BioWulf Technologies (New York). Fernando Perez-Cruz has served as Chief Data Scientist at the SDSC from 2018 to 2023, and Deputy Executive Director of the SDSC from 2022 to 2023


Fotis has joined SDSC as Sr. Systems Engineer. Before SDSC's Engineering team, Fotis has been delivering HPC platforms and large scale services across several countries, with varying technical complexity. Even with multiple projects, ranging from global ISPs TCP/IP delay measurements, several clusters for CERN's Large Hadron Collider physics experiment, developing in EasyBuild while automating HPC software builds and contracted for professional documentation thereof, up to delivering in 2015 and running the system that has led globally for processing most human DNA for clinical use ever, 100K Genome Project, Fotis always found the time and enthusiam for training hundreds of scientists and systems administrators to fulfill their mission. Finally, Fotis is an active promoter of Open Source Software and open standards and has been spearheading several impactful HPC/OSS hackathons.


Emma graduated from the University of Pennsylvania, PA, USA, with a BA in physics and computer science in 2013. Most recently since then, she has built systems to facilitate computational molecular dynamics research at D. E. Shaw Research and worked on exoplanet climate modeling in the astrobiology group at NASA GISS, both in New York City. Her research interests include networks and complexity as applied to life in the universe and also to the flow of scientific information through academia and society. The latter research interest has motivated her to volunteer at the World Science Festival in NYC, volunteer-teach computer science, attend open science hackathons. She is now attending a PhD program in History of Science at UC San Diego, CA, USA.
description
Problem:
- Increasing pressure on lakes needs scientific support
- 3D numerical simulations of lakes require input data – uncertainty quantification in parameters & forecast
- New L’EXPLORE platform in Lake Geneva – increasing availability of high resolution data
Solution:
- Sensor-to-frontend open data platform
- Physics-driven hydrodynamic models
- Data-driven modeling of input data processes
- Parallel Bayesian inference – MCMC with ABC or PF
- Multi-level speedup – hierarchical numerical models
- Powered by Renku, the SDSC-developed platform for transparency and reproducibility in science
- A neural network with uncertainty quantification properties in order to more accurately aggregate data from satellite imagery into the Bayesian inference
Impact
- Real time monitoring & future forecast of lakes
- Platform for large-scale interdisciplinary collaborations
- Research in hydrological / ecological lake modeling
- Scientifically grounded water resources management
Presentation
Gallery
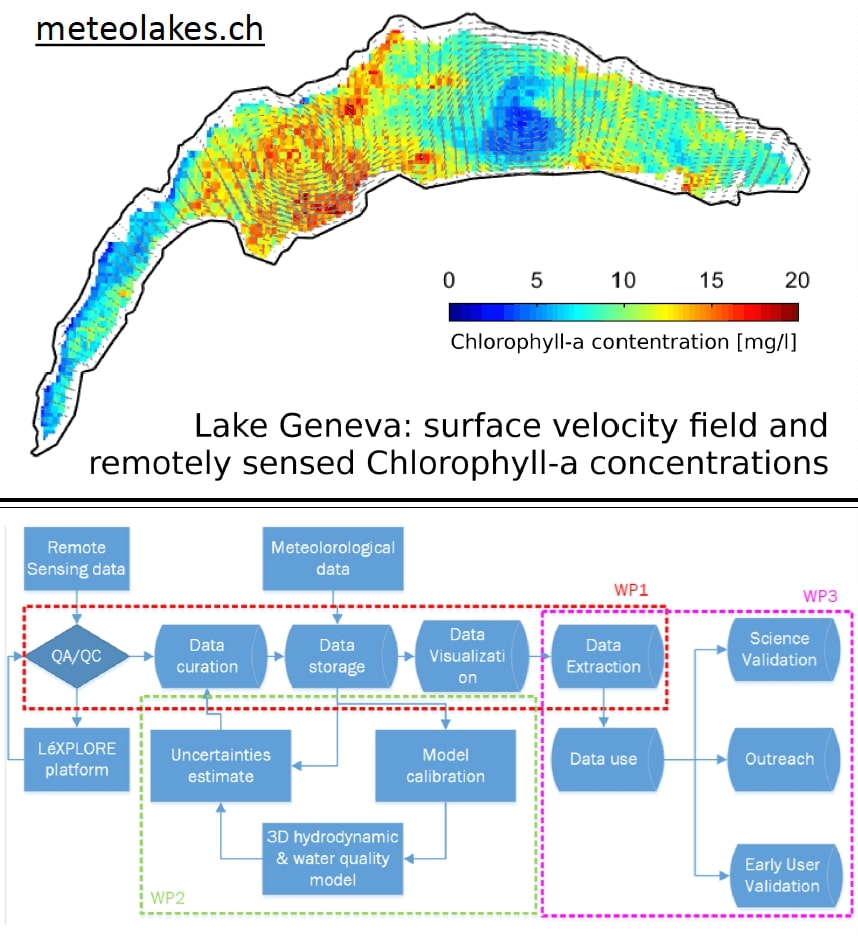
Annexe
Publications
- D. Bouffard, J Runnalls, T Baracchini, E Bouillet, H E Chmiel, T Doda, B Fernández Castro, F Georgatos, S Lavanchy, C Minaudo, F Ozdemir, D Odermatt, M-E Perga, P Perolo, S Piccolroaz, M Plüss, L Råman Vinnå, M Schmid, A Safin, J Šukys, V Tran-Khac, H N. Ulloa, C L. Ramón, A Wüest. Datalakes, a data platform for Swiss lakes. In prep for Earth System Data Science
- Safin, A., Bouffard, D., Ozdemir, F., Ramón, C. L., Runnalls, J., Georgatos, F., Minaudo, C., and Šukys, J.: A Bayesian data assimilation framework for lake 3D hydrodynamic models with a physics-preserving particle filtering method using SPUX-MITgcm v1, Geosci. Model Dev., 15, 7715–7730, A Bayesian data assimilation framework for lake 3D hydrodynamic models with a physics-preserving particle filtering method using SPUX-MITgcm v1 , 2022
Additional resources
Bibliography
Publications
Related Pages
More projects
ML-L3DNDT
BioDetect
News
Latest news


Smartair | An active learning algorithm for real-time acquisition and regression of flow field data
Smartair | An active learning algorithm for real-time acquisition and regression of flow field data
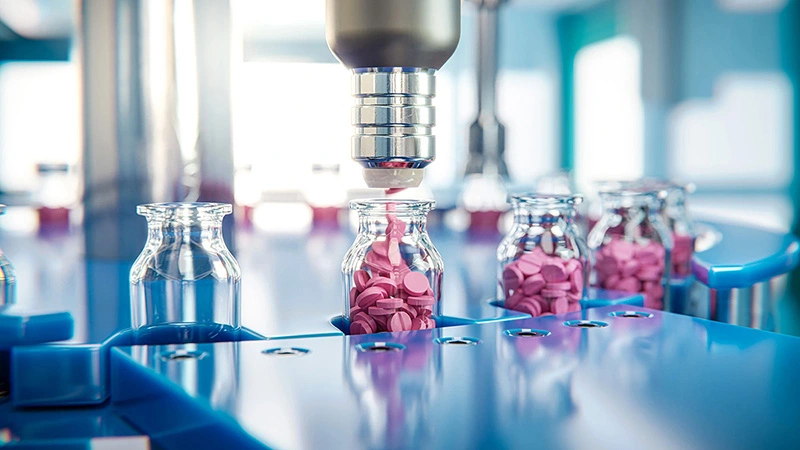
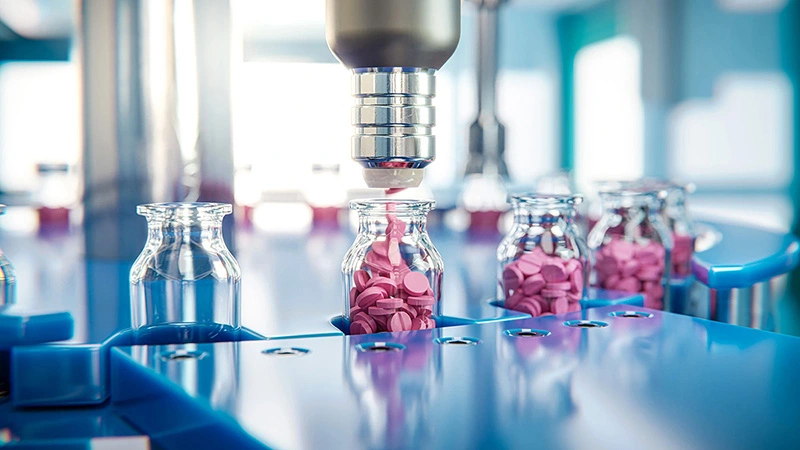
The Promise of AI in Pharmaceutical Manufacturing
The Promise of AI in Pharmaceutical Manufacturing


Efficient and scalable graph generation through iterative local expansion
Efficient and scalable graph generation through iterative local expansion
Contact us
Let’s talk Data Science
Do you need our services or expertise?
Contact us for your next Data Science project!