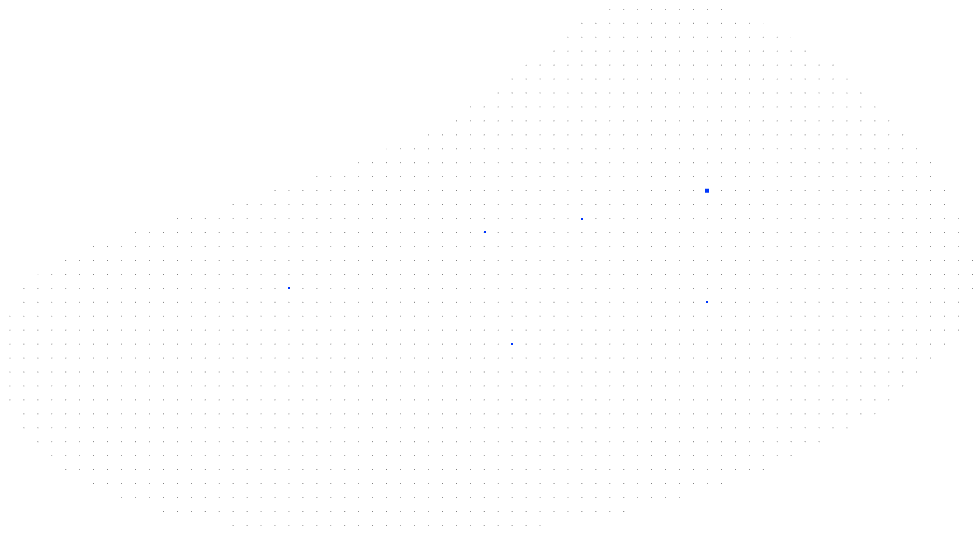
HighFEM
High Frequency Earthquake Modelling

Abstract
Earthquakes are among the most devastating natural hazards humanity is confronted with. Earthquake shaking spans a very wide range of frequencies, with classic sensors designed to record signals over four orders of magnitude in frequency. The low frequency part of seismic signals is readily used to study earthquake processes and the interior structure of the Earth. Using the high frequency parts of earthquake wavefields, on the other hand, is more challenging, because i) high frequency signals are hard to observe: seismometers are usually too far away and too sparse to record high frequency wavefields with the necessary resolution; ii) high frequency wavefields are hard to model: the computational cost of wavefield simulations grows with the 4th power of frequency, and we lack the detailed knowledge of underground geological structures that affect and modify the signals at this small scales. Next level underground laboratories, such as ETHZ’s Bedretto Lab or Utah Forge, however, strongly reduce the observational limitations, in that they facilitate earthquake monitoring from unusually close distances, and with high sensor densities. Here we propose to use this opportunity, and to jointly leverage high performance computing, generative deep modeling and the massive, near-field earthquake data sets that the novel laboratories provide, to model and study earthquake waveforms up to very high frequencies. We will use these modelling capabilities to achieve a range of science goals, including improved seismicity monitoring algorithms, and to study small scale earthquake source processes and ground motion site response.
People
Collaborators


Simon joined the SDSC as a senior data scientist in April 2022. He conducted his doctoral studies on statistical modeling of genetic data at ETH Zürich and obtained his MSc and BSc degrees at Technical University Munich in computer science. Before joining the SDSC, Simon worked as a freelance statistical consultant, and as an ML scientist at an AI startup in Lugano where he built experience in various topics ranging from generative modeling over Bayesian optimization to time series forecasting. Simon's research interests and expertise lie broadly in probabilistic machine and deep learning, causal inference, generative modeling, and their application in the natural sciences. Simon is an avid open-source software contributor and particularly enthusiastic about probabilistic programming languages, such as Stan.
PI | Partners:
ETH Zurich, Department of Earth Sciences:
- Dr. Men-Andrin Meier
- Dr. Laura Ermert
- Dr. Maria Koroni
In close collaboration with our SDSC intern Andreas Bergmeister
description
Motivation
The prime observation to study earthquake phenomena are seismic waveforms. Inferences
on earthquake sources and earth interior structure are made by comparing them to increasingly sophisticated numerical models. A current gap in our capabilities is the generation of realistic high
frequency (HF) synthetic seismic waveforms. The computational cost of deterministic physics-based
waveform simulations puts limits to the resolvable frequency range. Moreover, detailed modelling
of high-frequency waveforms requires considerable prior geologic knowledge, such as gradients and interfaces between rock types, cracks and shear zones, and fine-scale topography.
Proposed Approach / Solution
For the project HighFEM, we are developing generative models for synthesis of seismic waveforms. Since waveforms are long-range time series data sets, approaches based on forecasting methods, such as recurrent neural networks and autoregressive models (e.g., LSTMs or pixelCNNs), combined with adversarial training (e.g., GANs) pose a promising research direction.
Impact
The project will allow to better characterize and understand natural and induced earthquakes; to study earthquake rupture, including at small scales; and to improve site-specific seismic hazard estimates.
Presentation
Gallery
Annexe
Additional resources
Bibliography
- Florez, Manuel A., et al. "Data‐driven synthesis of broadband earthquake ground motions using artificial intelligence." Bulletin of the Seismological Society of America 112.4 (2022): 1979-1996.
Publications
Related Pages
More projects
ML-L3DNDT
BioDetect
News
Latest news


Smartair | An active learning algorithm for real-time acquisition and regression of flow field data
Smartair | An active learning algorithm for real-time acquisition and regression of flow field data
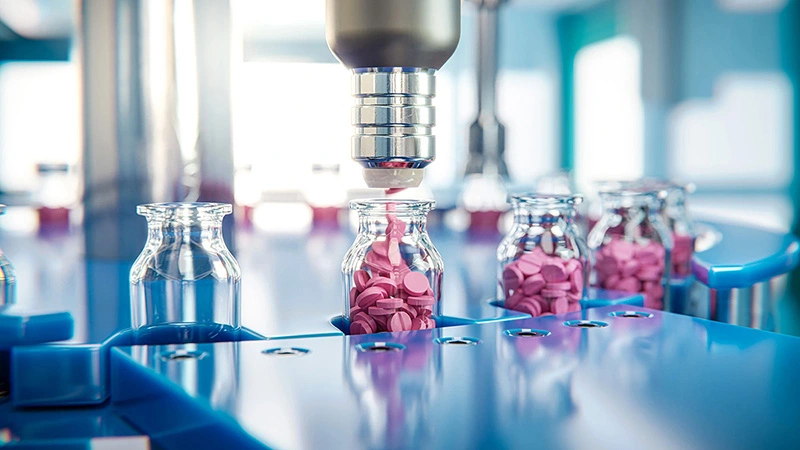
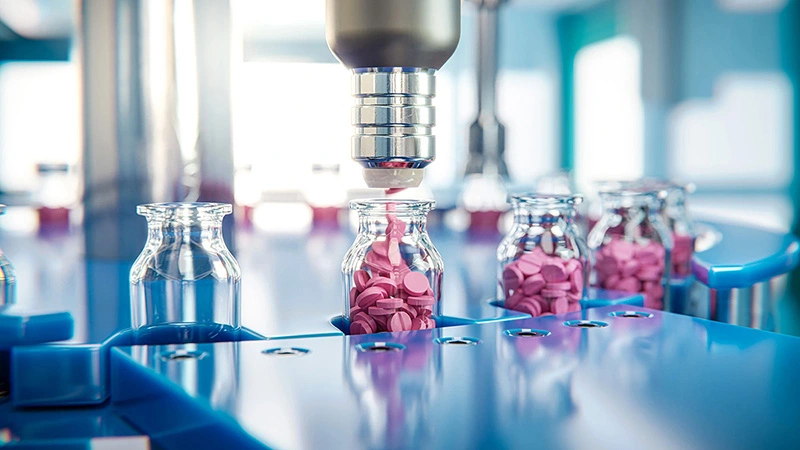
The Promise of AI in Pharmaceutical Manufacturing
The Promise of AI in Pharmaceutical Manufacturing


Efficient and scalable graph generation through iterative local expansion
Efficient and scalable graph generation through iterative local expansion
Contact us
Let’s talk Data Science
Do you need our services or expertise?
Contact us for your next Data Science project!