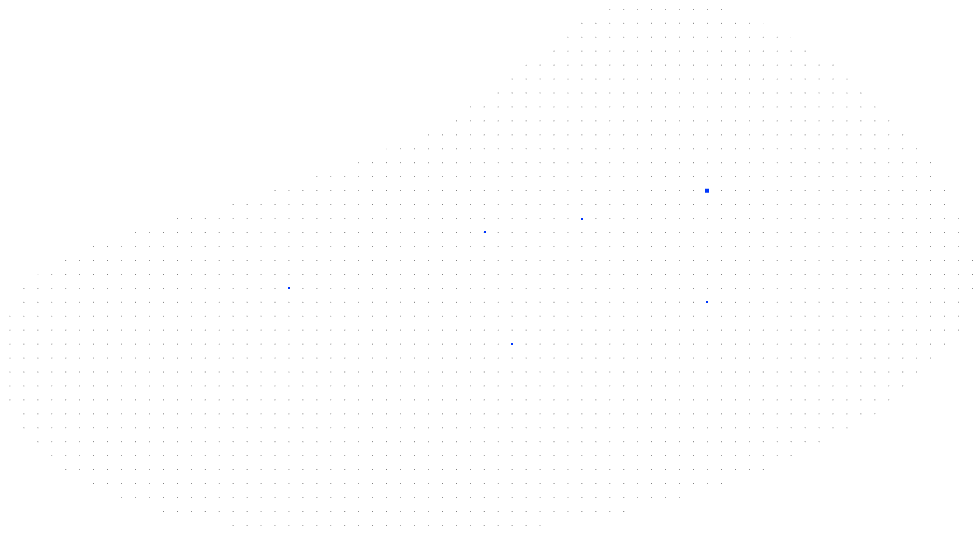
LEAP
LEArning to Print – towards data-driven real-time predictions for additive manufacturing

Abstract
Laser Powder Bed Fusion (LPBF) is the dominating additive manufacturing process for high-performance metal components. This process builds 3D parts by repeatedly spreading and selectively melting a thin layer of powder. In each layer, the 2D cross section of the geometry is melted by a moving laser spot and then solidifies and bonds to the underlying layer. For a part of ~100 mm height, roughly 1000-2000 layers are needed. On each layer, the laser spot follows a certain trajectory, i.e., a set of hundreds of scan vectors that melt the powder on suitable points of the layer. The quality of the printed part strongly depends on its geometry and the scan strategy. Nowadays, since no method is available to find an optimal scan pattern for a given part geometry, scan strategies are found empirically. However, for a technology capable of producing single parts from digital data, it is prohibitive to count on experience and trial-and-error optimization. A plethora of numerical process models has been developed to simulate the process. When entire parts are simulated fast, simplified approaches are used, that cannot account for the scan strategy. High-fidelity (HF) simulations of the interaction of the laser and the material are feasible, but only for a limited number of scan vectors. They can neither be used to simulate entire layers, nor for entire 3D geometries. A source of information not yet exploited rigorously are data that can be monitored during the process. High resolution optical and thermal imaging as well as pyrometry allow to measure the temperature and the melt pool shape, and create several hundred GB of data per hour of printing. These data code the thermal history at each point of the top layer as a function of the scan vector sequence and laser settings, but they are subject to systematic measurement errors and noise. In this project, we address the research questions whether it is possible (1) to generate fast predictive process models from a combination of experimentally measurable process data and HF numerical simulations to enhance the resolution and accuracy of the experimental data and (2) to identify geometrical features and scan vector patterns in shapes to be printed that are prone to developing defects along with strategies to avoid such defects. We thus would like to develop an ‘LPBF learning process’, which is fully in line with the scope of the call.
People
Collaborators
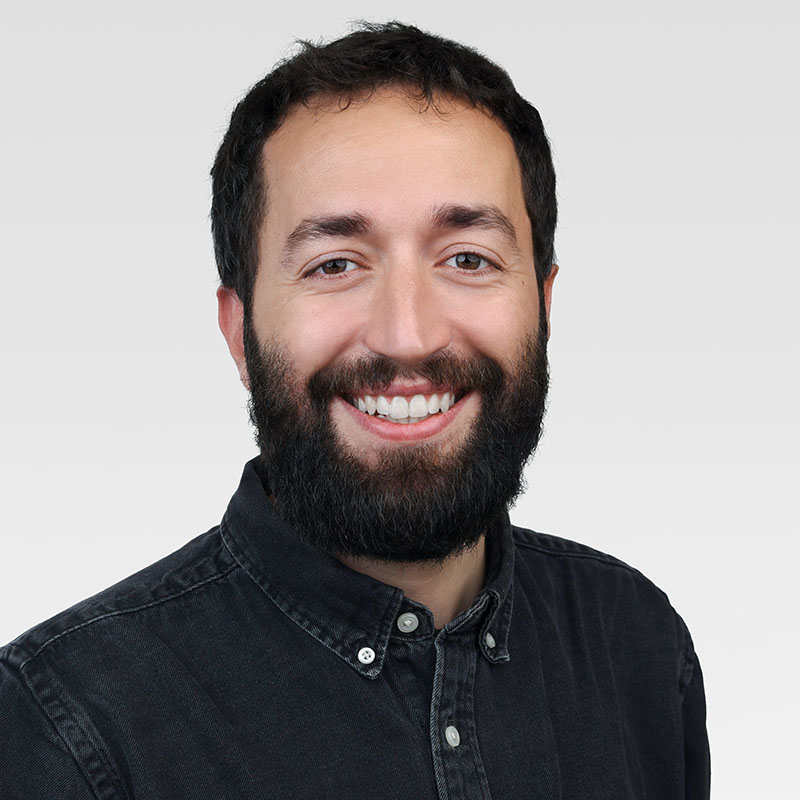
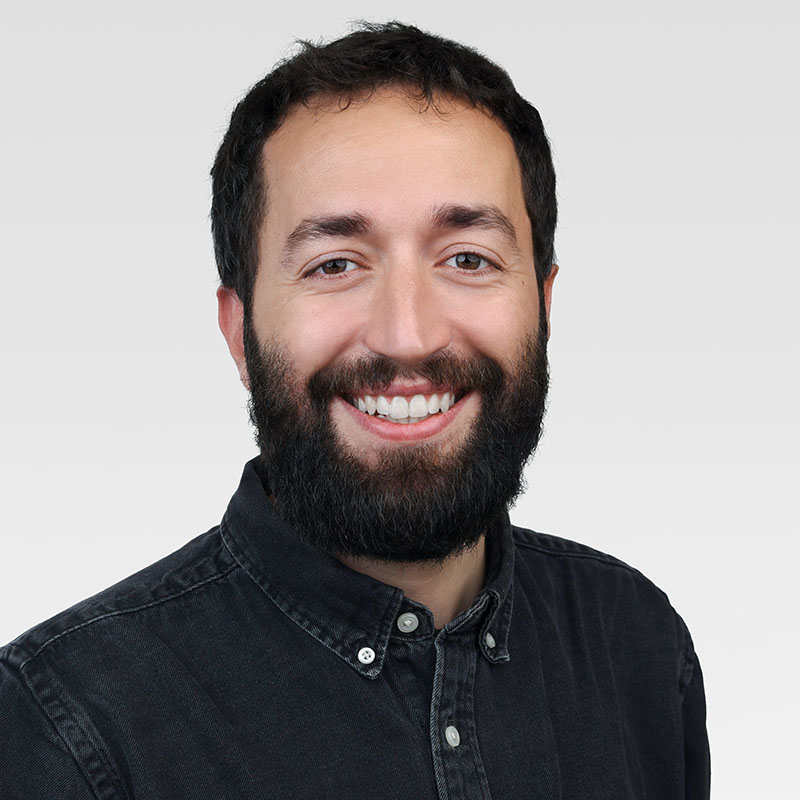
Konstantinos obtained a Master's degree in Mechanical Engineering from the Technical University of Delft, Netherlands and conducted his doctoral studies in the Chair of Structural Mechanics and Monitoring at ETH Zurich with a focus on the fusion of physics-based and data-driven models for vibration-based monitoring of structural and mechanical systems. Before joining SDSC, he was a postdoctoral researcher at ETH Zurich. His research interests revolve around machine learning, uncertainty quantification, inference of probabilistic models, time series forecasting and Bayesian modeling.
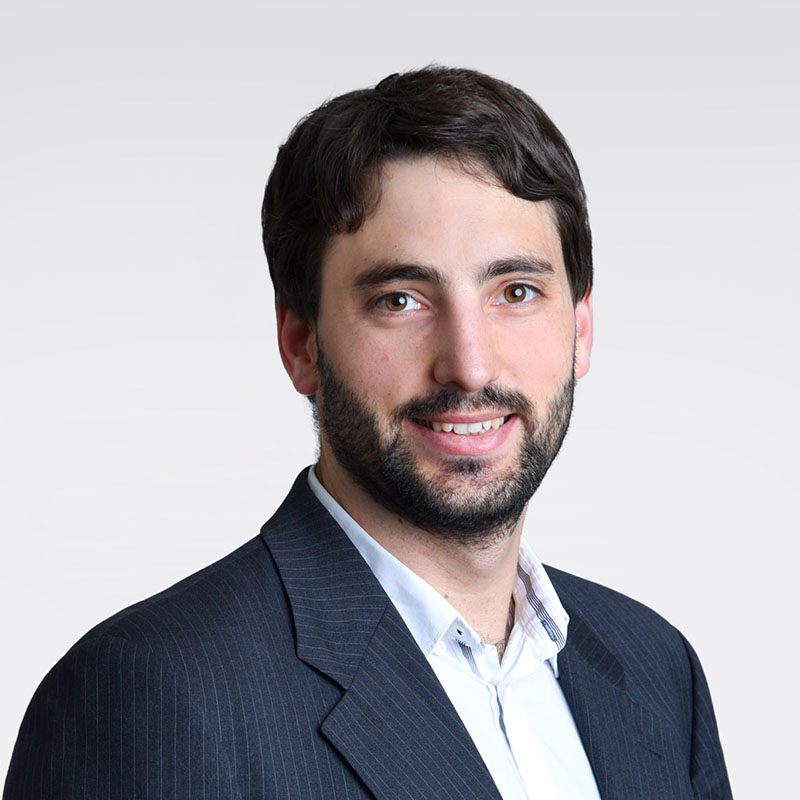
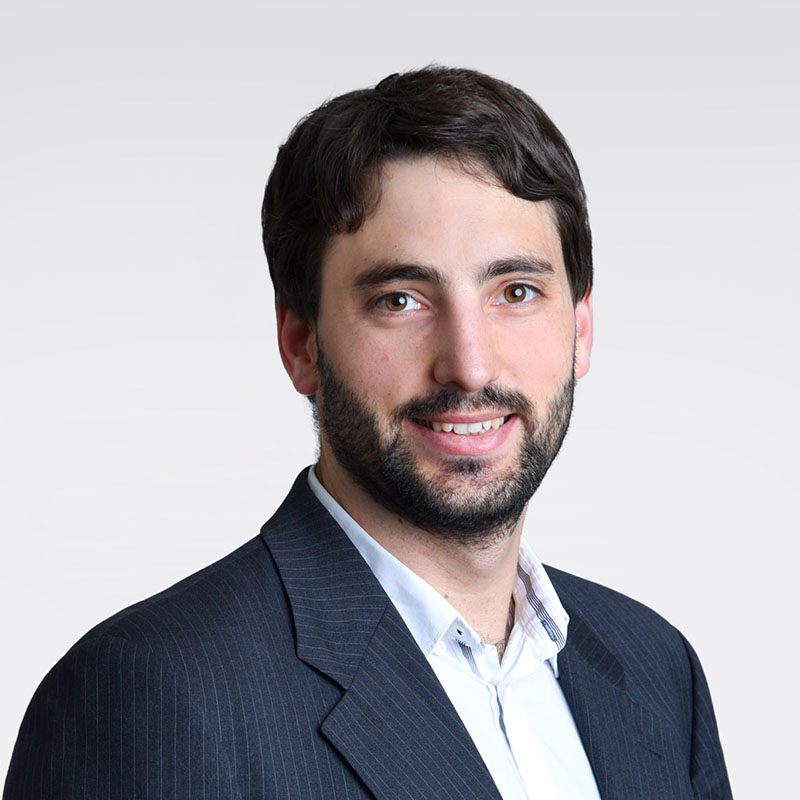
After finishing his Master in electrical engineering at the Ecole Fédérale de Lausanne (EPFL), Nathanaël worked as a researcher in the Acoustic Research Institute (ARI) in Vienna. In 2013, he returned to EPFL for a PhD, where he specialized himself in different fields of data science: signal processing, machine learning, graph theory and optimization. Furthermore, he created two open source libraries for optimization (UNLocBoX) and graph signal processing (GSPBOX). Since 2017, Nathanaël Perraudin is a Research Data Scientist at the Swiss Data Science Center in the ETH Zurich. He focuses on different aspects of deep learning in the area of generative models (VAE and GAN), recursive architectures and convolutional neural network for irregular domains. Outside office hours, he is passionate by tango dancing, tandem bike touring, skiing and rock climbing.


Luis is originally from Spain, where he completed his bachelor's studies in Electrical engineering, and the Ms.C. on signal theory and communications, both at the University of Seville. During his Ph.D. he started focusing on machine learning methods, more specifically message passing techniques for channel coding, and Bayesian methods for channel equalization. He carried it out between the University of Seville and the University Carlos III in Madrid, also spending some time at the EPFL, Switzerland, and Bell Labs, USA, where he worked on advanced techniques for optical channel coding. When he completed his Ph.D. in 2013, he moved to the Luxembourg Center on Systems Biomedicine, where he switched his interest to neuroscience, neuroimaging, life sciences, etc., and the application of machine learning techniques to these fields. During his 4 and a half years there as a Postdoc, he worked on many different problems as a data scientist, encompassing topics such as microscopy image analysis, neuroimaging, single-cell gene expression analysis, etc. He joined the SDSC in April 2018. As Lead Data Scientist, Luis coordinates projects in various domains. Several projects focus on the application of natural language processing and knowledge graphs to the study of different phenomena in social and political sciences. In the domains of architecture and engineering, Luis is responsible for projects centered on the application of novel generative methods to parametric modeling. Finally, Luis also coordinates different projects in robotics, ranging from collaborative robotic construction to deformable object manipulation.
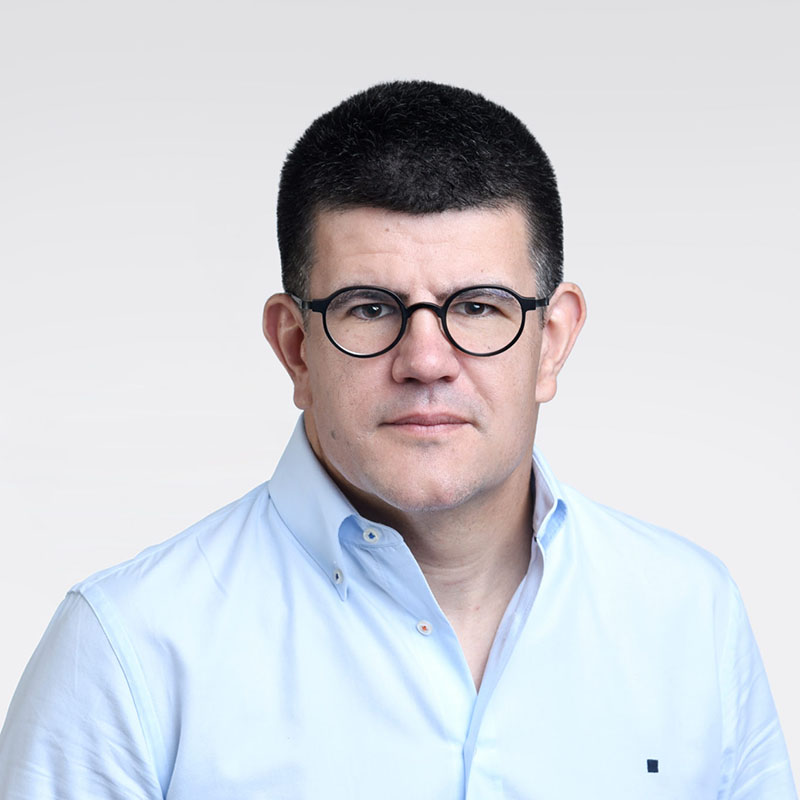
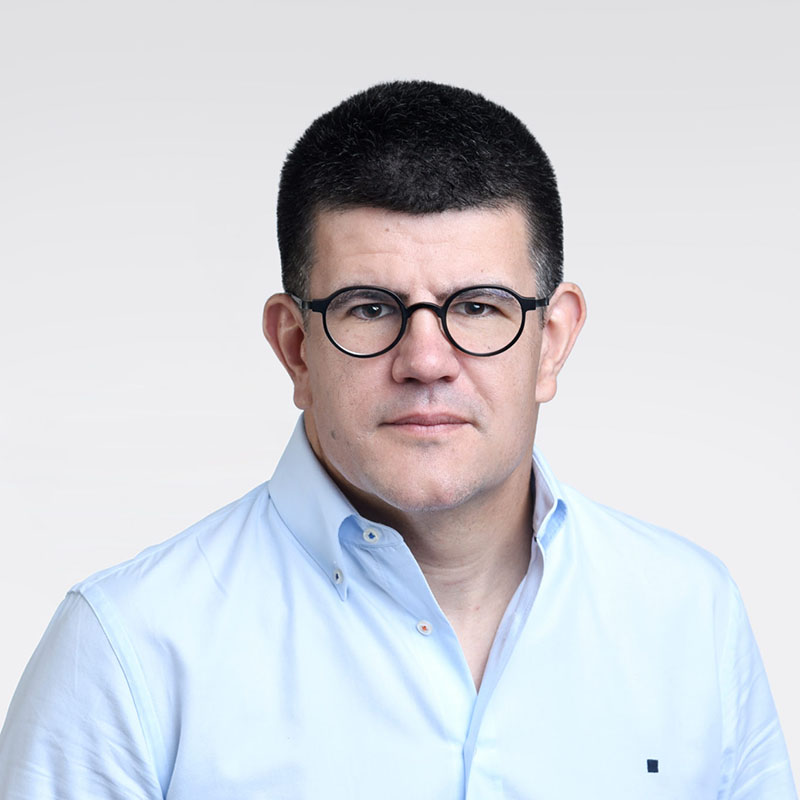
Fernando Perez-Cruz received a PhD. in Electrical Engineering from the Technical University of Madrid. He is Titular Professor in the Computer Science Department at ETH Zurich and Head of Machine Learning Research and AI at Spiden. He has been a member of the technical staff at Bell Labs and a Machine Learning Research Scientist at Amazon. Fernando has been a visiting professor at Princeton University under a Marie Curie Fellowship and an associate professor at University Carlos III in Madrid. He held positions at the Gatsby Unit (London), Max Planck Institute for Biological Cybernetics (Tuebingen), and BioWulf Technologies (New York). Fernando Perez-Cruz has served as Chief Data Scientist at the SDSC from 2018 to 2023, and Deputy Executive Director of the SDSC from 2022 to 2023
PI | Partners:
description
Motivation
The Laser Powder Bed Fusion (LPBF) 3D printing process faces a significant challenge in accurately inputting the correct laser commands to achieve the desired object shape with the desired properties. While simulations exist, their computational complexity restricts their practical usability. The aim of this project it to enhance the LPBF 3D printing process with the use of data-driven surrogate models, which can replace the computationally expensive simulators for the prediction of the melting pool properties.
Proposed Approach / Solution
The proposed solution is based on the development of Deep Learning (DL) surrogate models using High Fidelity (HF) simulation data, as shown in Fig. 1. These models will be used for space and time predictions during the LPBF process, as well as for the optimization of the scanning process, with the aim of minimising thermal concentrations and delivering the target object with the desired microstructure properties.
Impact
One of the key promises of additive manufacturing in general, and LPBF specifically, is the possibility to manufacture new and highly individualized designs with fine and complicated structures, which have not been possible to manufacture traditionally at a rather low cost. As such, the integration of Deep Learning (DL) models in the loop will enhance the LPBF process and will enable the manufacturing of complicated designs.

Presentation
Gallery
Annexe
Additional resources
Bibliography
- Yap, C. Y., Chua, C. K., Dong, Z. L., Liu, Z. H., Zhang, D. Q., Loh, L. E., & Sing, S. L. (2015). Review of selective laser melting: Materials and applications. Applied physics reviews, 2(4), 041101.
- Mozaffar, M., Liao, S., Xie, X., Saha, S., Park, C., Cao, J., & Gan, Z. (2022). Mechanistic artificial intelligence (mechanistic-AI) for modeling, design, and control of advanced manufacturing processes: Current state and perspectives. Journal of Materials Processing Technology, 302, 117485.
Publications
Related Pages
More projects
ML-L3DNDT
BioDetect
News
Latest news
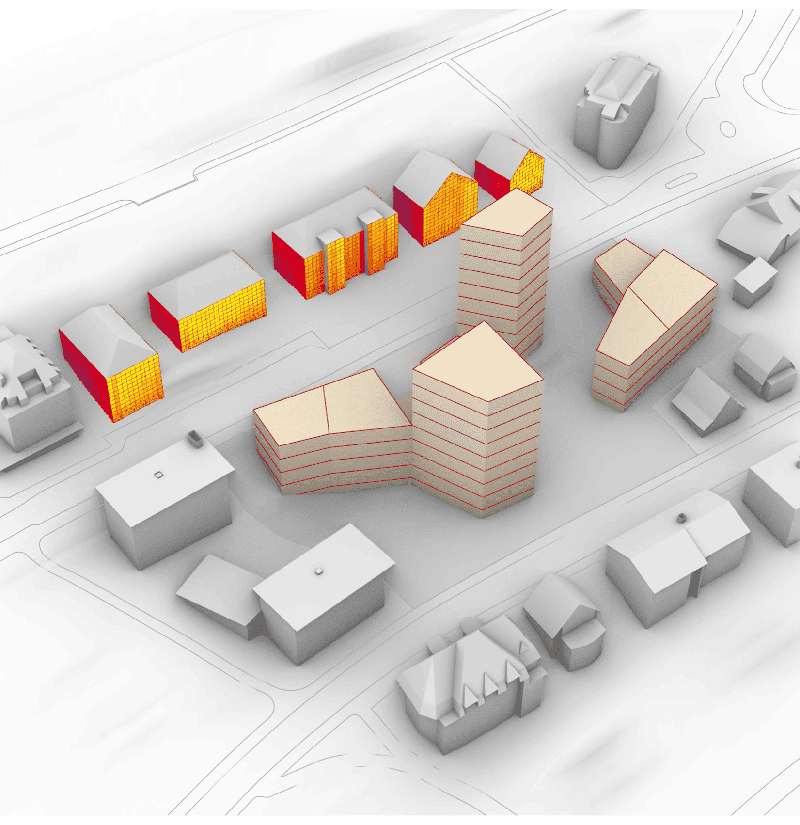
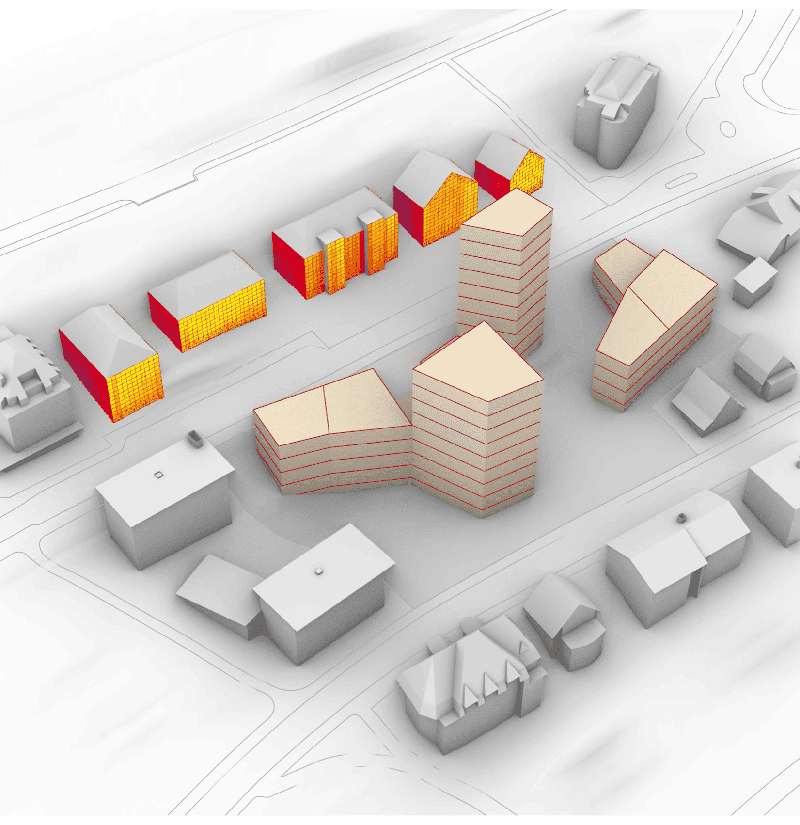
AIXD | Generative AI toolbox for architects and engineers
AIXD | Generative AI toolbox for architects and engineers


Smartair | An active learning algorithm for real-time acquisition and regression of flow field data
Smartair | An active learning algorithm for real-time acquisition and regression of flow field data
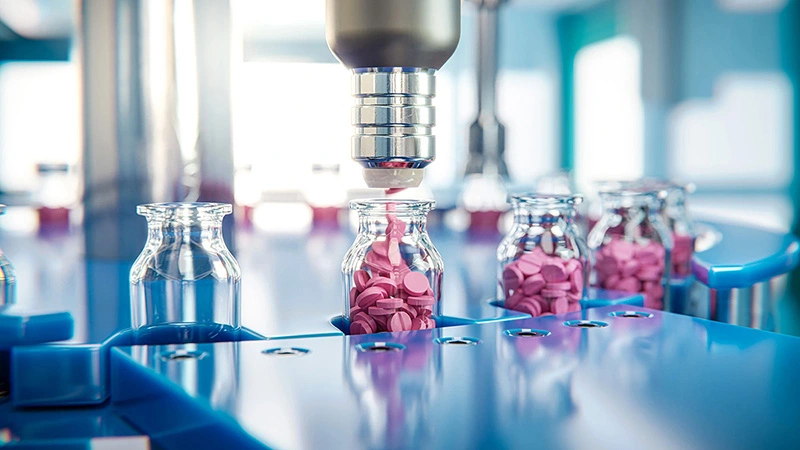
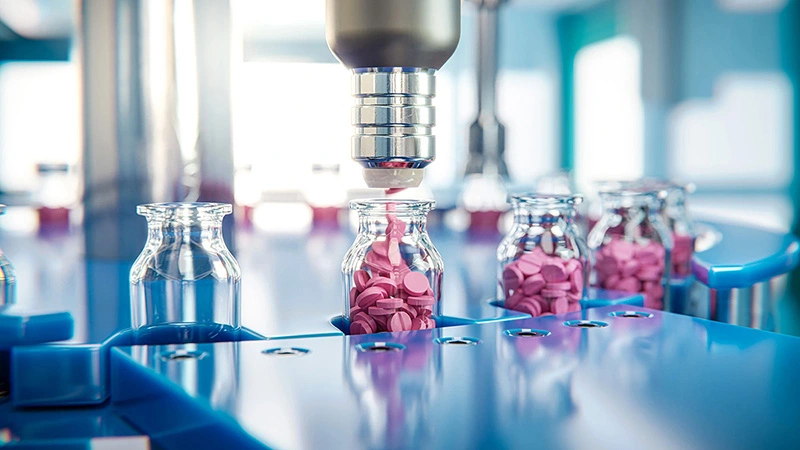
The Promise of AI in Pharmaceutical Manufacturing
The Promise of AI in Pharmaceutical Manufacturing
Contact us
Let’s talk Data Science
Do you need our services or expertise?
Contact us for your next Data Science project!