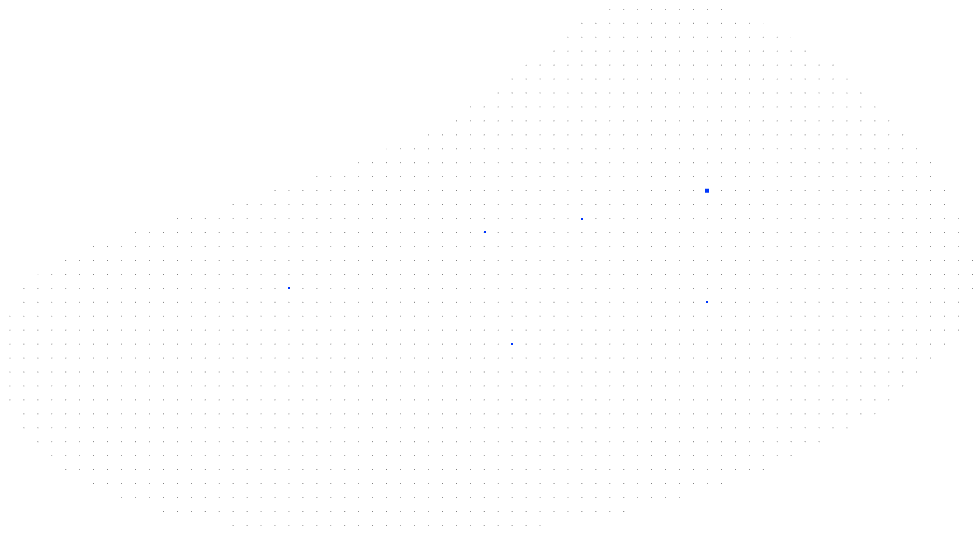
MLfusion
Machine Learning for Disruption Prediction in Tokamaks
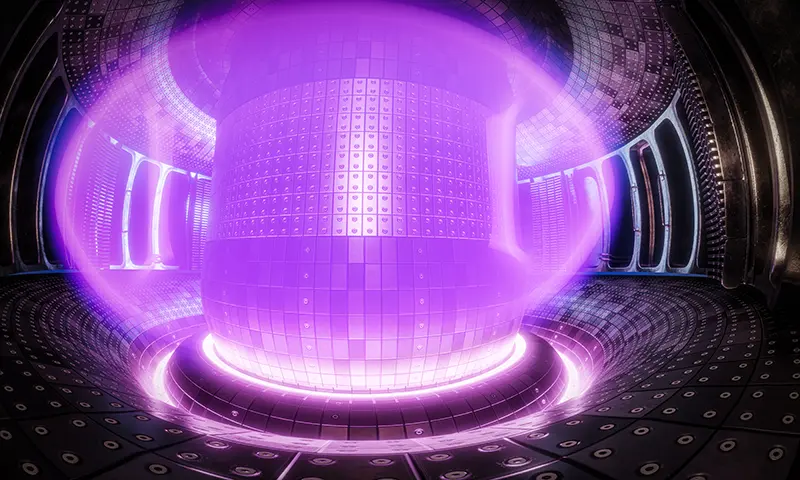
Abstract
Tokamak machines are promising technology for the ever-growing demand for clean energy. They confine plasma in a torus-shaped chamber in order to maintain prolonged fusion reactions. For Tokamaks' successful operation a critical phenomenon, known as disruptions (a catastrophic loss of plasma control), has to be avoided for the continuation of the fusion reaction and machine-damage mitigation. This project aims at leveraging Machine Learning models combined with efficient Bayesian Inference algorithms to detect the onset of plasma disruptions early on. For this purpose models shall project the high-dimensional sensor data, acquired during Tokamak operation, into a reduced latent space representation unveiling operation boundaries separating non-disruptive from disruptive regimes. Non-stationary models with the objective of tracking the transitions between different plasma regimes will be employed to track the versatile dynamics. This is important to inform the control system to avoid a disruption.
The capability of the model to generalize to unseen (or partially seen) domains is a critical issue that future large-scale reactors such as ITER must address before high-performance operation phases. At the second stage of the project, we wish to improve the generalization capabilities of the proposed models incorporating domain invariant prior knowledge extracted by the analysis of the physics mechanisms leading to disruption in different tokamaks and quantify the extrapolation uncertainty of the proposed model using a multi-machine database from three European tokamaks (JET, TCV, and AUG).
People
Collaborators


Christian joined SDSC in 2019 to design data-driven solutions to support academic research projects. His research interests comprise inference of probabilistic models, with a focus on nonparametric models, and stochastic processes. He holds a PhD in Computational Neuroscience from the Technical University Berlin, Germany, a MSc from the Bernstein Center for Computational Neuroscience Berlin, and a BSc in Cognitive Science from the University of Osnabrück, Germany. During the course of his studies, he additionally conducted research at Khalifa University (UAE), Strathclyde University (UK), and the RIKEN Brain Science Institute and the Kyoto University in Japan. Before joining SDSC, he was an intern at Amazon CoreAI in Berlin.


After earning a MSc in Theoretical Physics at University of Padua, Giulio graduated in Quantitative Finance from Bocconi University. Before joining the SDSC industry cell in June 2021, he spent a few years working in the financial sector, where he mainly dealt with the application of machine learning to financial risk management. When not coding, Giulio spends his free time playing bass guitar, hiking and cooking.
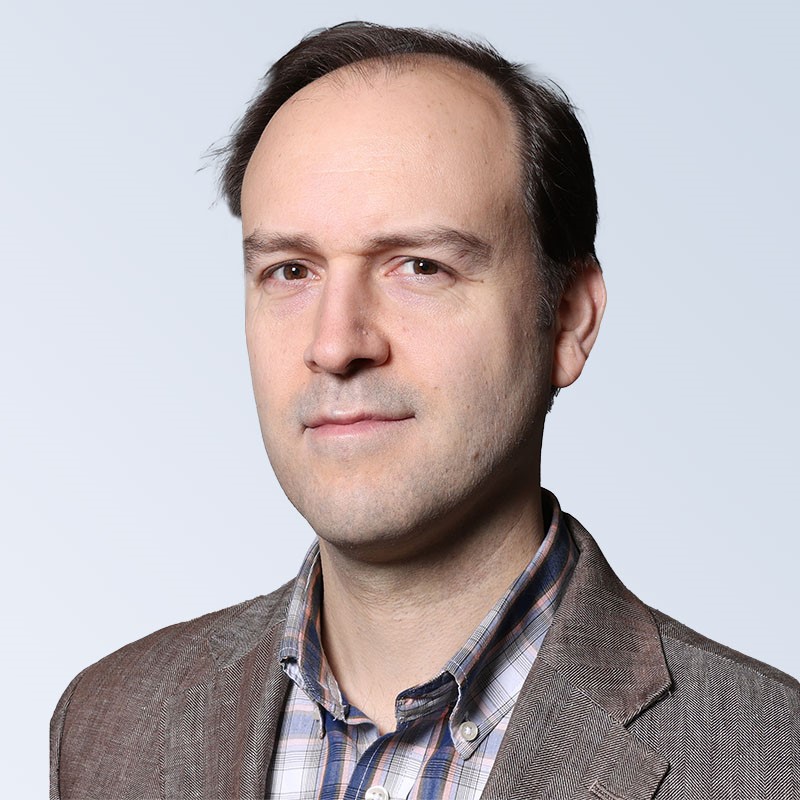
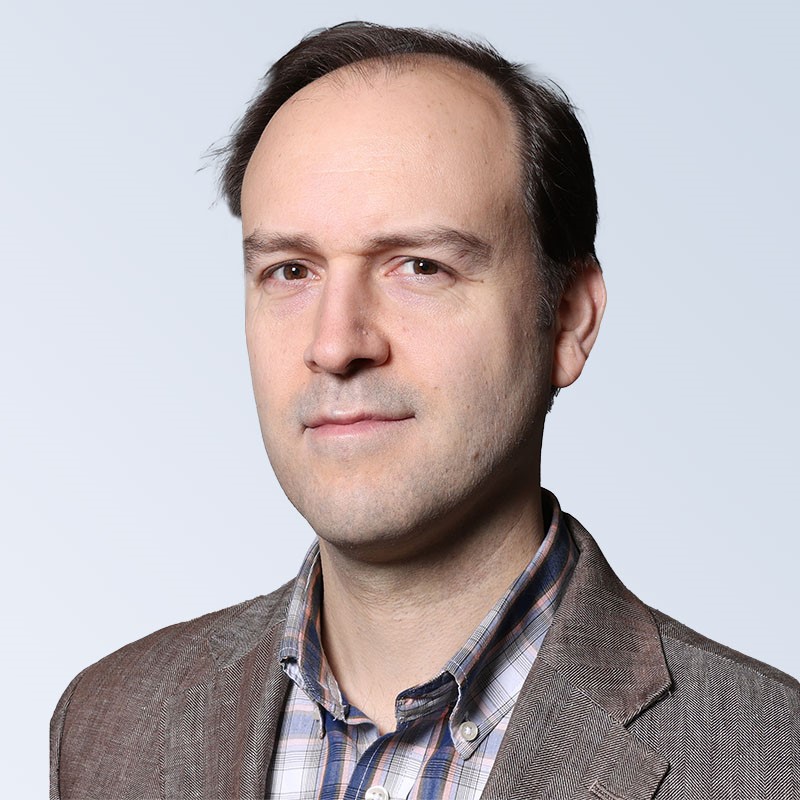
Guillaume Obozinski graduated with a PhD in Statistics from UC Berkeley in 2009. He did his postdoc and held until 2012 a researcher position in the Willow and Sierra teams at INRIA and Ecole Normale Supérieure in Paris. He was then Research Faculty at Ecole des Ponts ParisTech until 2018. Guillaume has broad interests in statistics and machine learning and worked over time on sparse modeling, optimization for large scale learning, graphical models, relational learning and semantic embeddings, with applications in various domains from computational biology to computer vision.
PI | Partners:
EPFL, Swiss Plasma Center:
- Prof. Olivier Sauter
- Dr. Alessandro Pau
- Yoeri Poels
- Cristina Venturini
description
Motivation
Tokamaks are devices that confine plasma allowing for fusion reaction. However, frequently fusion reaction is hampered by so-called disruptions - a sudden and uncontrolled loss of the plasma current and confinement, inducing severe thermal loads on plasma-facing components and large electromagnetic forces on the conductive structures surrounding the plasma. This can give rise to unacceptable damages, making the avoidance and mitigation (reduction of the detrimental effects) of disruptions, a critical need for the success of fusion energy in next-step fusion devices. Hence, it is crucial to design early warning strategies, that allow controlled shutdown, before a disruption occurs.
Proposed Approach / Solution
We attempt to learn data-driven approaches, that provide a real-time estimate of the disruption probability at any time. For this we use time-series models that learn dynamics in a latent space, which is also predictive for the danger of disruptions.
Impact
The resulting algorithm helps to predict disruption early and thereby prevent them, which is crucial for the new generation of fusion devices such as ITER, where disruption can cause severe damage to the machine.
Presentation
Gallery
Annexe
Additional resources
Bibliography
- Pau, A., Fanni, A., Carcangiu, S., Cannas, B., Sias, G., Murari, A., & Rimini, F. (2019). A machine learning approach based on generative topographic mapping for disruption prevention and avoidance at JET. Nuclear Fusion, 59(10), 106017. DOI: 10.1088/1741-4326/ab2ea9
- Degrave, J., Felici, F., Buchli, J., Neunert, M., Tracey, B., Carpanese, F., ... & Riedmiller, M. (2022). Magnetic control of tokamak plasmas through deep reinforcement learning. Nature, 602(7897), 414-419. DOI: 10.1038/s41586-021-04301-9
Publications
Related Pages
More projects
ML-L3DNDT
BioDetect
News
Latest news


Smartair | An active learning algorithm for real-time acquisition and regression of flow field data
Smartair | An active learning algorithm for real-time acquisition and regression of flow field data
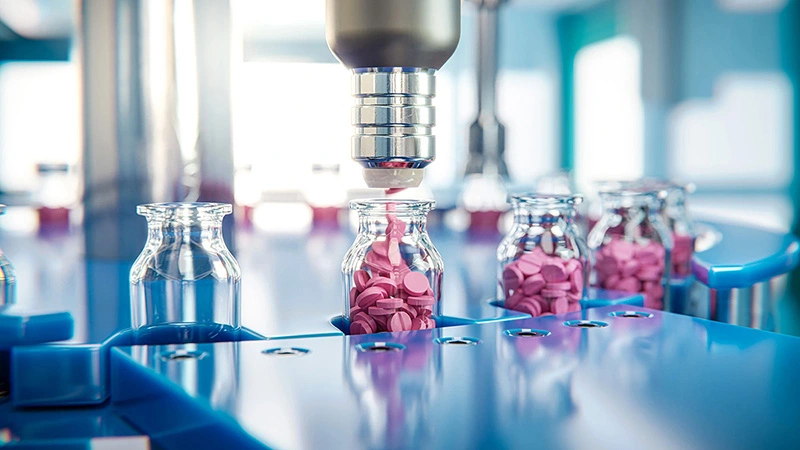
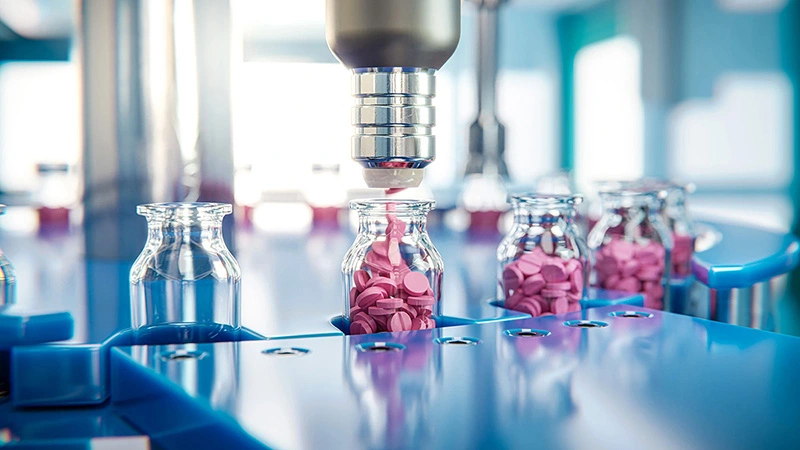
The Promise of AI in Pharmaceutical Manufacturing
The Promise of AI in Pharmaceutical Manufacturing


Efficient and scalable graph generation through iterative local expansion
Efficient and scalable graph generation through iterative local expansion
Contact us
Let’s talk Data Science
Do you need our services or expertise?
Contact us for your next Data Science project!