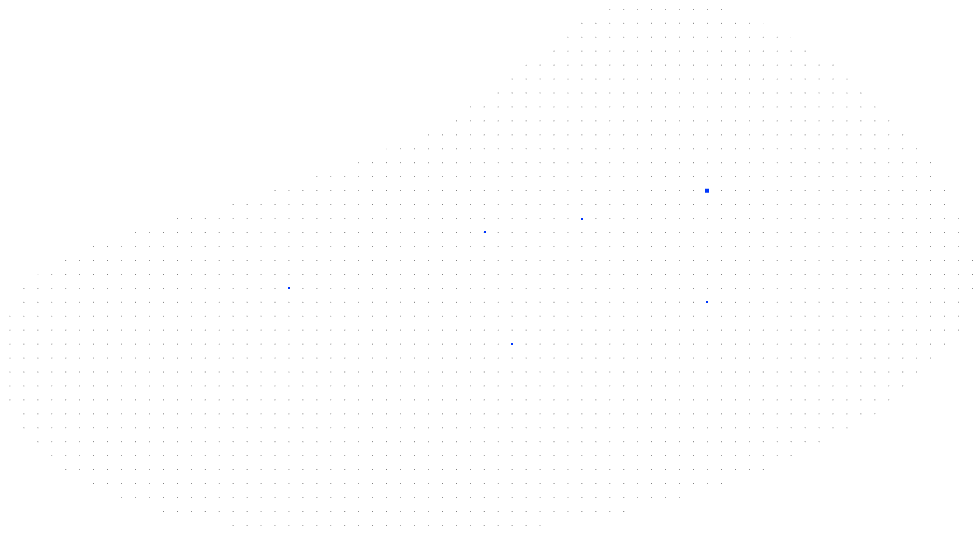
PACMAN HIPA
Particle Accelerators and Machine Learning
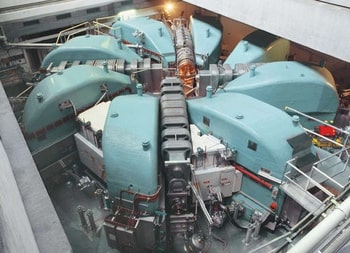
Abstract
The High Intensity Proton Accelerator (HIPA) at Paul Scherrer Institute (PSI) provides the primary beams to PSI’s versatile experimental facilities which in turn provide high intensity beams for research. In an accelerator control room several hundred of continuous sensor data are displayed in order to aid the operators in running the accelerator with maximal performance. We propose to bring Machine Learning (ML) to particle accelerator operation, in order to increase the performance. A more accurate parameter control based on the surrogate modelling will contribute to reliable and safe operation, and increase the accelerator efficiency. The immediate benefits will be: reducing the risks related to the high beam power by reducing the activation and beam losses, an action that will in turn, lead to fewer machine interruptions and possibly higher beam intensities. The project is likely to have a game-changing impact in howwe model and operate charged particle accelerators in the near future.
People
Collaborators
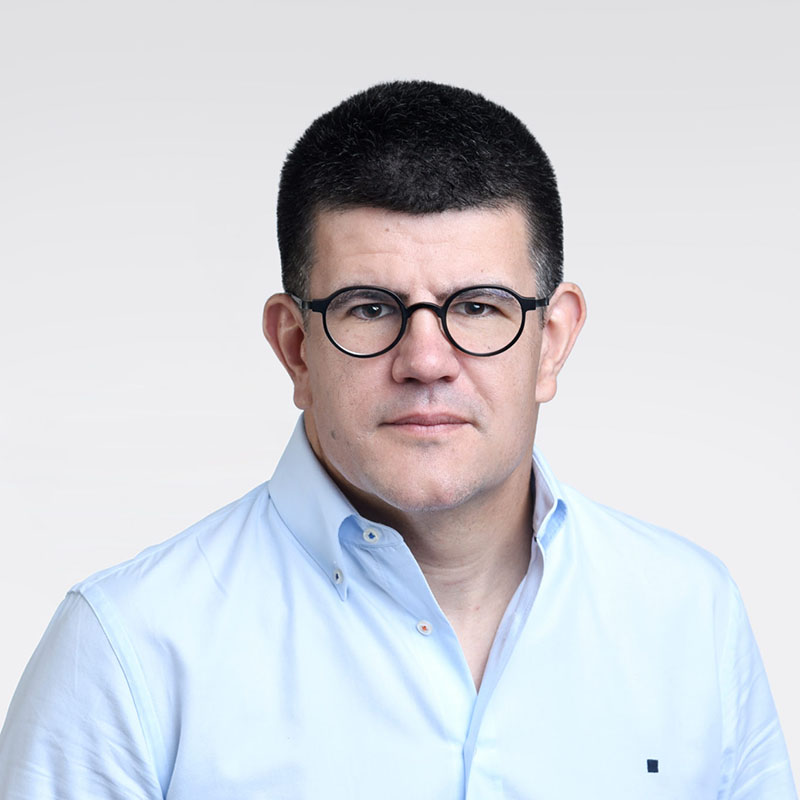
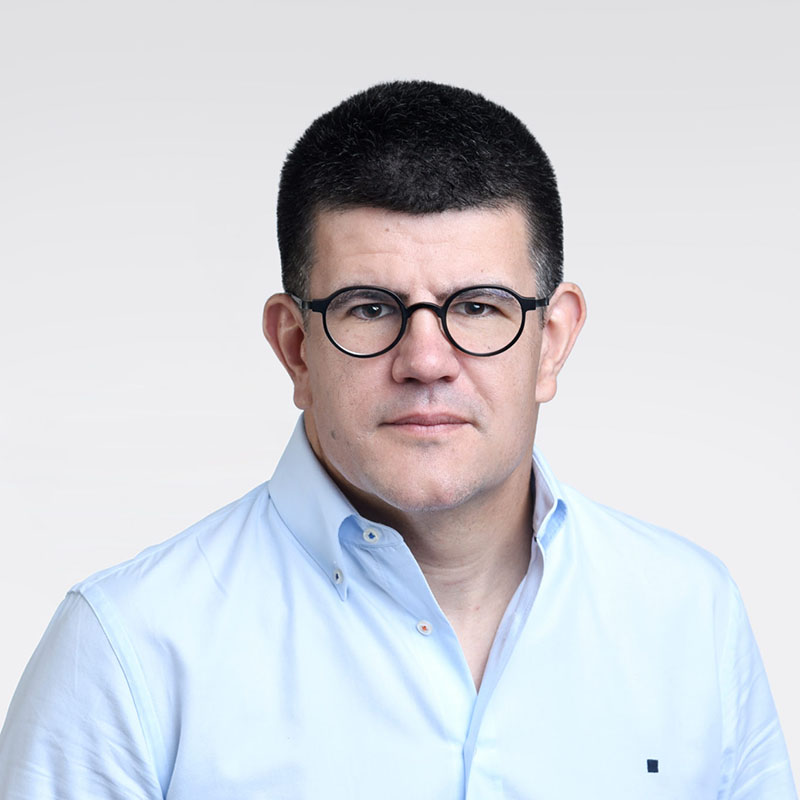
Fernando Perez-Cruz received a PhD. in Electrical Engineering from the Technical University of Madrid. He is Titular Professor in the Computer Science Department at ETH Zurich and Head of Machine Learning Research and AI at Spiden. He has been a member of the technical staff at Bell Labs and a Machine Learning Research Scientist at Amazon. Fernando has been a visiting professor at Princeton University under a Marie Curie Fellowship and an associate professor at University Carlos III in Madrid. He held positions at the Gatsby Unit (London), Max Planck Institute for Biological Cybernetics (Tuebingen), and BioWulf Technologies (New York). Fernando Perez-Cruz has served as Chief Data Scientist at the SDSC from 2018 to 2023, and Deputy Executive Director of the SDSC from 2022 to 2023
PI | Partners:
PSI, Laboratory for Scientific Computing and Modelling:
- Dr. Andreas Adelmann
- Dr. Jaime Coello de Portugal
- Sichen Li
ETH Zurich, Learning & Adaptive Systems Group:
- Prof. Andreas Krause
- Johannes Kirschner
- Mojmir Mutny
description
Goals:
- Minimise beam losses: To be able to predict the reaction of a knob, especially those at the first sections of the accelerator, a reliable machine model needs to be available.
- Better control of accelerator parameters: we will establish a fast on-line enhancement of the machine protection system with beam diagnostics data. The accelerator parameters will be predicted from the beam diagnostics data. Appropriate changes to the machine / beamline settings will be proposed as operational enhancements by the surrogate model.
- Prevent unnecessary machine interruptions: A surrogate model that captures fast responses from the machine can be used in the forecasting of machine interruptions. Here we would expand from research goal 1 and use results from the fusion community [10] (and the references therein).
Presentation
Gallery
Annexe
Publications
- Li, S., Zacharias, M., Snuverink, J., Coello de Portugal, J., Perez-Cruz, F., Reggiani, D., & Adelmann, A. (2021). A Novel Approach for Classification and Forecasting of Time Series in Particle Accelerators. Information, 12(3), 121.
- Kirschner, J., Mutný, M., Krause, A., Coello de Portugal, J., Hiller, N., & Snuverink, J. (2022). Tuning Particle Accelerators with Safety Constraints using Bayesian Optimization. Phys. Rev. Accel. Beams, arXiv:2203.13968v2.
Additional resources
Bibliography
Publications
Related Pages
More projects
ML-L3DNDT
BioDetect
News
Latest news


Smartair | An active learning algorithm for real-time acquisition and regression of flow field data
Smartair | An active learning algorithm for real-time acquisition and regression of flow field data
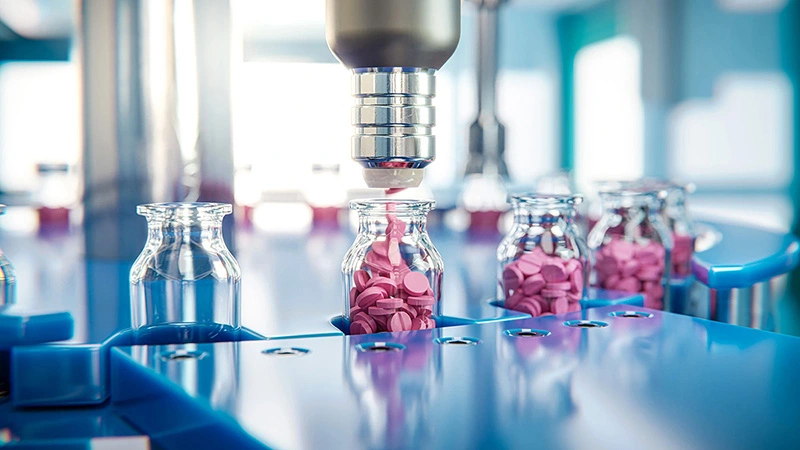
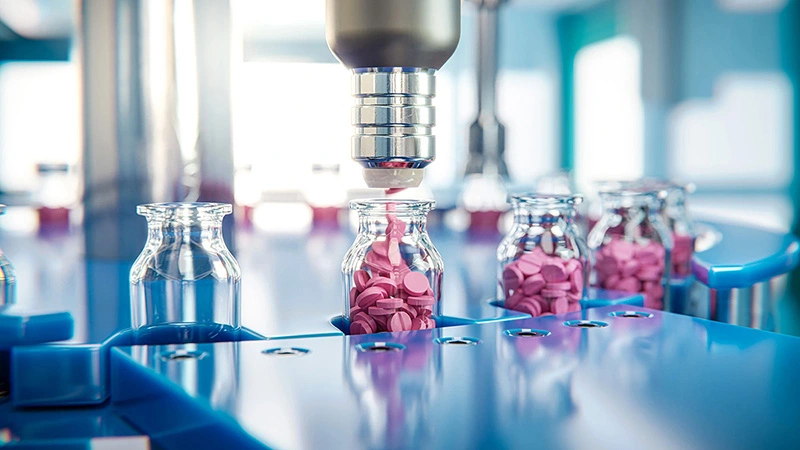
The Promise of AI in Pharmaceutical Manufacturing
The Promise of AI in Pharmaceutical Manufacturing


Efficient and scalable graph generation through iterative local expansion
Efficient and scalable graph generation through iterative local expansion
Contact us
Let’s talk Data Science
Do you need our services or expertise?
Contact us for your next Data Science project!