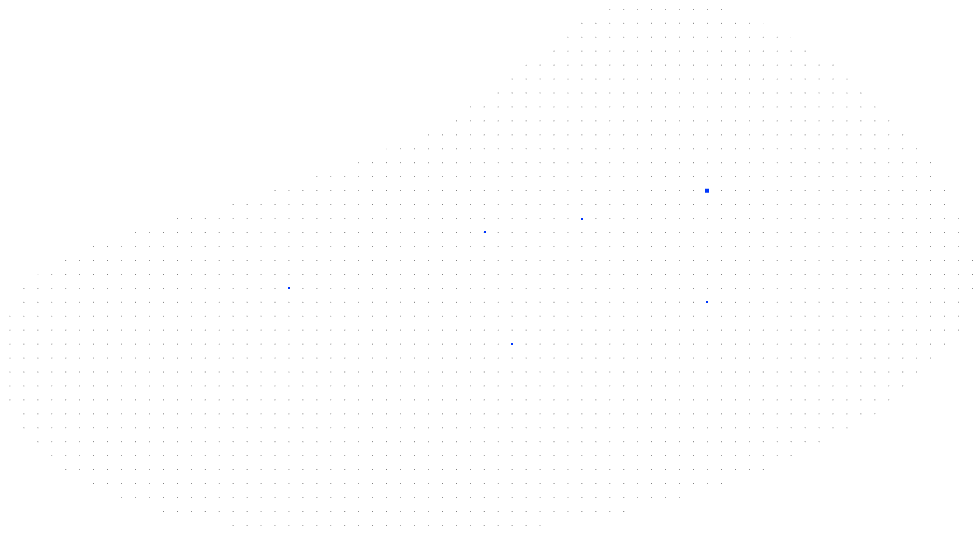
PolyNet
Exploring disease trajectories and outcome prediction using novel methods in network analysis and machine learning
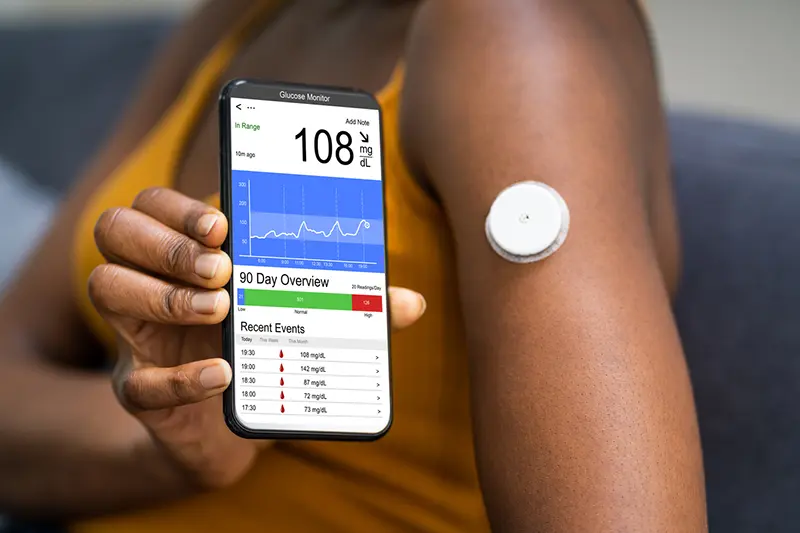
Abstract
T2DM is the fasting growing chronic disease worldwide and poses a substantial burden to the patient and healthcare system. Thus, the clinical management of T2DM is of global concern. However, due to the complex nature of the disease progression, the relationship between comorbidities and glycemic control remain poorly understood. The ability to improve our understanding of the common disease trajectories starting from diagnosis would provide new insights into disease phenotypes, risk factors, and provide opportunities to develop personalized treatment plans. Additionally, one clinical area of concern within diabetes management is the risk of fragility fractures. While patients with T2DM often have normal or even increased BMD, studies consistently show these patients have an increased risk of fragility fracture. While a number of studies have examined common fracture risk factors for fracture, observational and animal studies are conflicting. Thus, we aim to explore new methods to capture the complex and dynamic nature of patient trajectories. To achieve this aim, this collaboration grant will bring together experts in pharmacoepidemiology, real-world data analytics, social network analysis, and machine learning to develop interpretable models that will serve as an important step towards identifying high-risk patients and subsequently prevent adverse health outcomes. In particular, PolyNet has two primary research objectives to solve the above identified gaps in T2DM care: 1) To explore new methodologies to characterize and visualize common disease and comorbidity trajectories in patients, and 2) To develop longitudinal models to address important clinical questions in T2DM – predicting glycemic control changes and fragility fracture risk. All projects will leverage data from the world’s largest primary care database, the UK Clinical Practice Research Datalink.
People
Collaborators


Izabela holds a PhD degree in Computer Science from University of Rennes 1, France and the National French Institute for Research in Computer Science and Automatics (INRIA), France. Before joining the SDSC, she was a postdoctoral researcher at the Chair of Computational Social Science at ETH Zurich and a lecturer for the “Data Science in Techno-Socio-Economic Systems” course at ETH Zurich. Her main research focus is on big data analytics, tools and platforms, machine learning and data mining, large scale network analysis, in the particular setting of social data mining.


Victor has joined the SDSC in 2020 to design solutions for data-driven optimization problems. His research interests lie at the crossroad of machine learning and decision-making. This contains several topics such as stochastic optimization, reinforcement learning, combinatorial optimization, and probabilistic graphical models. Victor received a PhD in operations research and machine learning from Ecole des Ponts Paristech in 2020. Before that, he completed a master degree in Operation Research and Machine learning at Ecole des Ponts Paristech and a bachelor degree in Applied Mathematics and Computer Sciences.


Anna joined the SDSC as a Senior Data Scientist in 2020. She is a statistician by training with a Master’s degree with Honors in Mathematical Statistics from Lomonosov Moscow State University. Anna has graduated with a PhD from ETH Zurich in 2018, where she worked on causal structure learning for protein signaling pathways. During her studies she did an internship at Facebook AI Research in New York working on discovery of hierarchies from data using hyperbolic geometry. Later she joined Facebook AI Research in Paris as a postdoctoral researcher, where she worked on a problem of out-of-distribution prediction of unseen drug combinations. Broadly her research interests are in unsupervised and self-supervised learning, domain adaptation and generalisation.


Alessandro holds an M.Sc. in Applied Mathematics from EPFL with a minor in Data Science. After his studies, he joined the SDSC as Data Scientist in April 2022, where he closely works with the academic community to enlarge and support the use of data science. Over the years he worked on a variety of topics, from extreme events modeling to time series representation. His main interest lies in the application of machine learning to the energy sector.
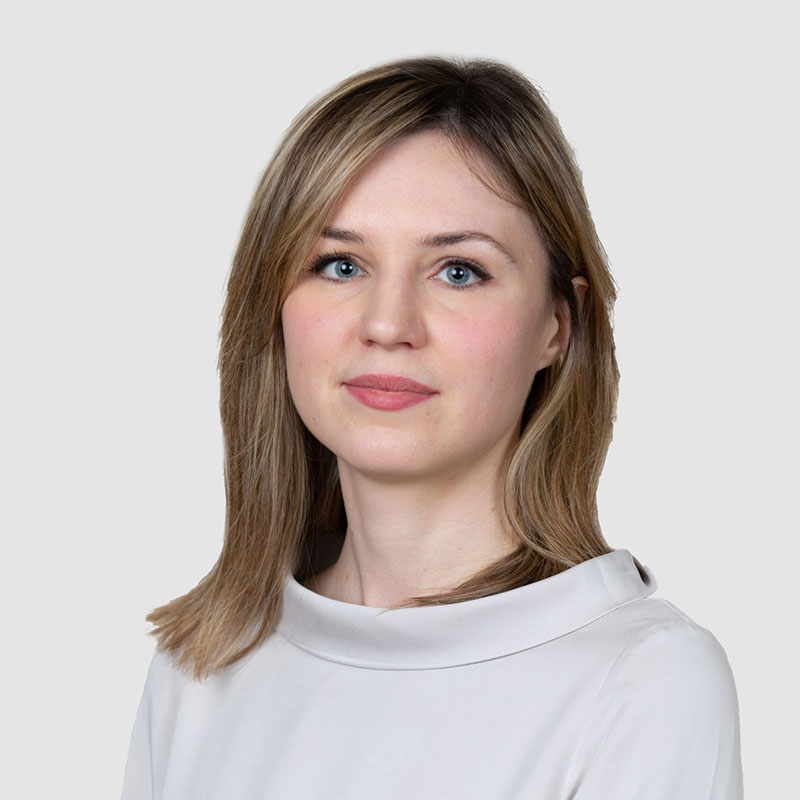
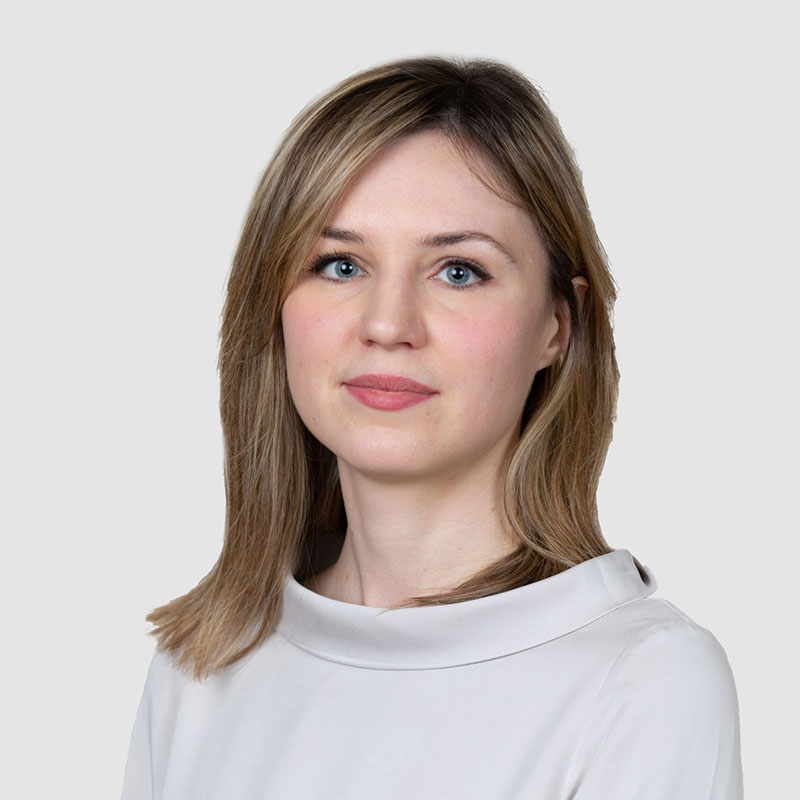
Ekaterina received her PhD in Computer Science from Moscow Institute for Physics and Technology, Russia. Afterwards, she worked as a researcher at the Institute for Information Transmission Problems in Moscow and later as a postdoctoral researcher in the Stochastic Group at the Faculty of Mathematics at University Duisburg-Essen, Germany. She has experience with various applied projects on signal processing, predictive modelling, macroeconomic modelling and forecasting, and social network analysis. She joined the SDSC in November 2019. Her interests include machine learning, non-parametric statistical estimation, structural adaptive inference, and Bayesian modelling.
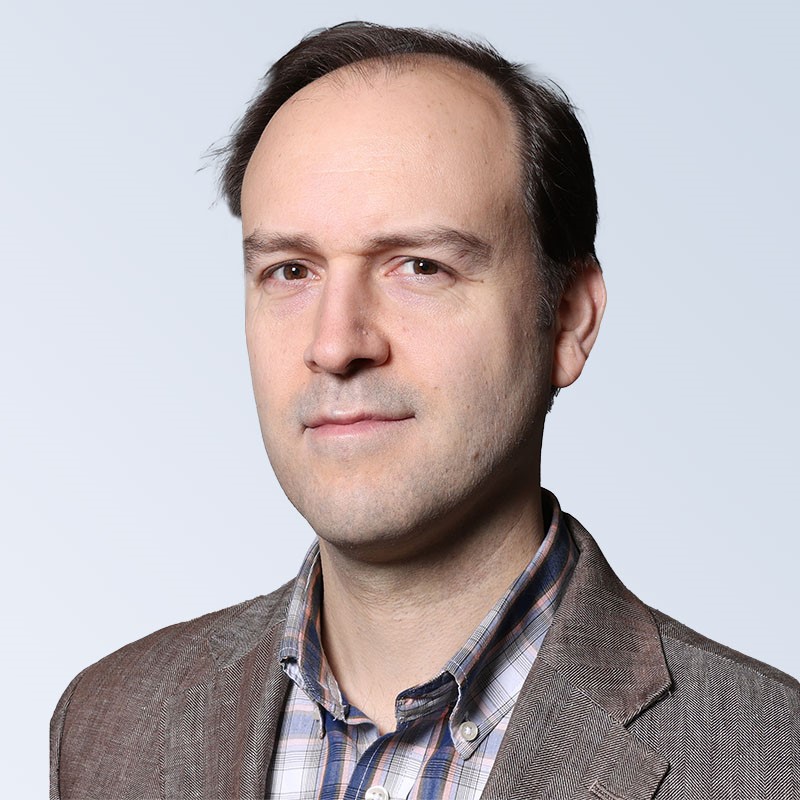
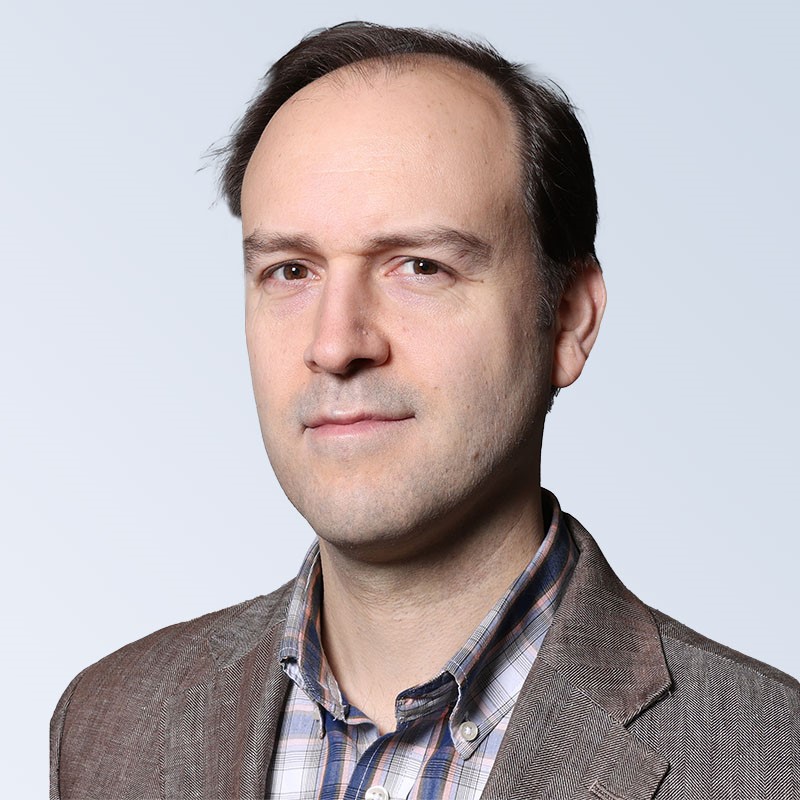
Guillaume Obozinski graduated with a PhD in Statistics from UC Berkeley in 2009. He did his postdoc and held until 2012 a researcher position in the Willow and Sierra teams at INRIA and Ecole Normale Supérieure in Paris. He was then Research Faculty at Ecole des Ponts ParisTech until 2018. Guillaume has broad interests in statistics and machine learning and worked over time on sparse modeling, optimization for large scale learning, graphical models, relational learning and semantic embeddings, with applications in various domains from computational biology to computer vision.
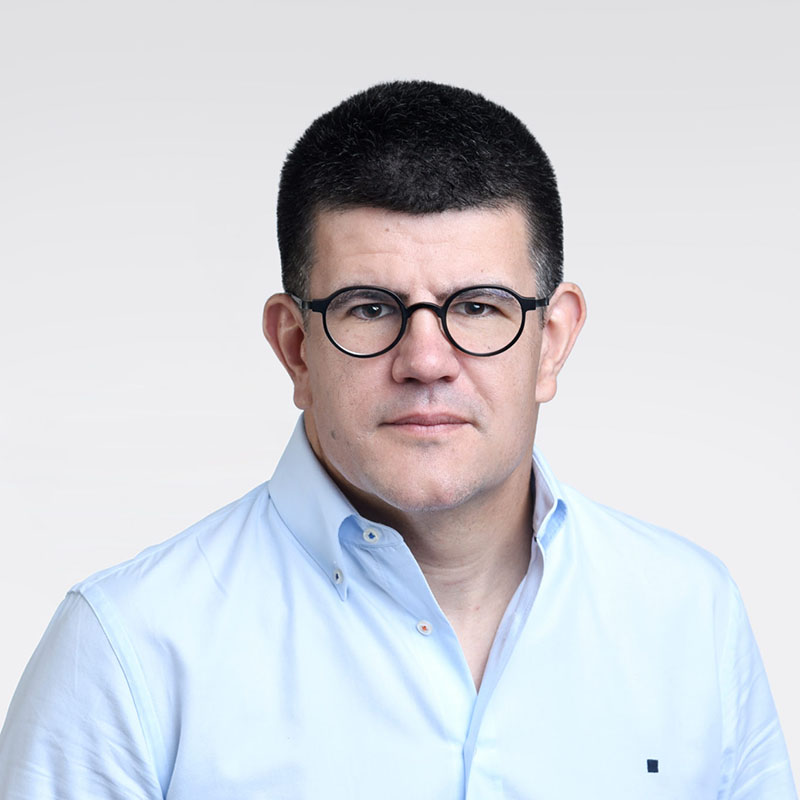
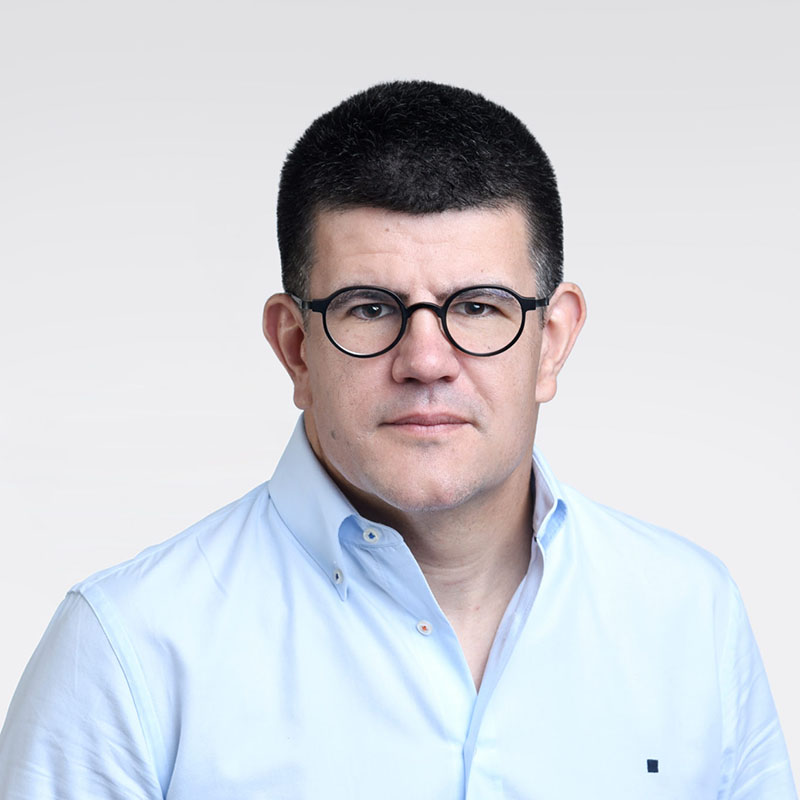
Fernando Perez-Cruz received a PhD. in Electrical Engineering from the Technical University of Madrid. He is Titular Professor in the Computer Science Department at ETH Zurich and Head of Machine Learning Research and AI at Spiden. He has been a member of the technical staff at Bell Labs and a Machine Learning Research Scientist at Amazon. Fernando has been a visiting professor at Princeton University under a Marie Curie Fellowship and an associate professor at University Carlos III in Madrid. He held positions at the Gatsby Unit (London), Max Planck Institute for Biological Cybernetics (Tuebingen), and BioWulf Technologies (New York). Fernando Perez-Cruz has served as Chief Data Scientist at the SDSC from 2018 to 2023, and Deputy Executive Director of the SDSC from 2022 to 2023
description
Motivation
The goal above all is to address the question: Can we better understand, and ultimately prevent, the development of complex comorbidities and adverse health events in T2DM? To accomplish this overarching goal, we identified two primary goals. First, to identify common trajectories of TD2M progression, comorbidity development and medication use over time. The second goal is to understand the interactions, to develop machine learning models predicting changes in glycemic control and fragility fracture risk.
Solution
We selected 58 chronic comorbidities of interest and used Bayesian nonparametric models to identify disease clusters. The latent feature models was able to automatically infer the number of binary latent features from the data. Further analysis of the clusters showed that presence of the certain comorbidities can lead to a dramatic increase in chances of developing other conditions. For modeling the progression of comorbidities over time we proposed the structural FHMM, which allowed to analyze the disease trajectories.
Impact
Our models identified established T2DM complications and previously unknown connections, thus, highlighting the potential for ML models to characterize complex comorbidity patterns.
Presentation
Gallery
Annexe
Additional resources
Bibliography
- Sonnenberg, F. A., & Beck, J. R. (1993). Markov models in medical decision making: A practical guide.
Medical Decision Making: An International Journal of the Society for Medical Decision Making,
13(4), 322–338. https://doi.org/10.1177/0272989X9301300409 - Wang, X., Sontag, D., & Wang, F. (2014). Unsupervised Learning of Disease Progression Models. Proceedings of the 20th ACM SIGKDD International Conference on Knowledge Discovery and Data Mining, 85–94. https://doi.org/10.1145/2623330.2623754
- P. Dworzynski, M. Aasbrenn, K. Rostgaard, M. Melbye, T. A. Gerds, H. Hjalgrim, and T. H. Pers. Nationwide prediction of type 2 diabetes comorbidities. Nature Scientific Report, vol. 10, 2019.
- M. Ravaut, H. Sadeghi, K. K. Leung, M. Volkovs, K. Kornas, V. Harish, T. Watson, G. F. Lewis, A. Weisman, T. Poutanen, and L. C. Rosella. Predicting adverse outcomes due to diabetes complications with machine learning using administrative health data.npj Digital Medicine, 4:1-12, 2021.
Publications
Related Pages
More projects
ML-L3DNDT
BioDetect
News
Latest news


First National Calls: 50 selected projects to start in 2025
First National Calls: 50 selected projects to start in 2025
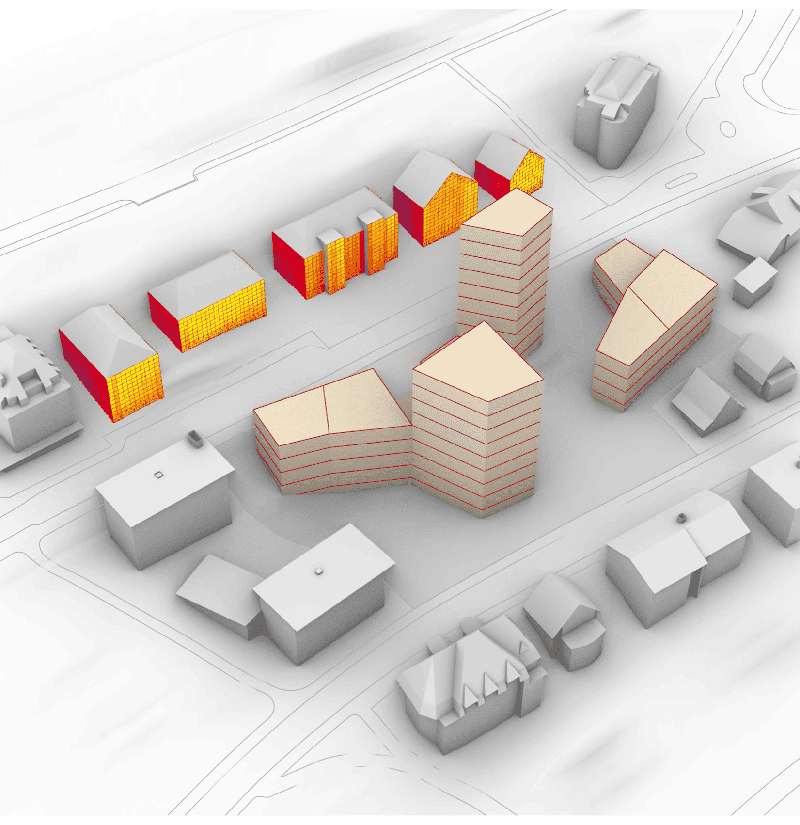
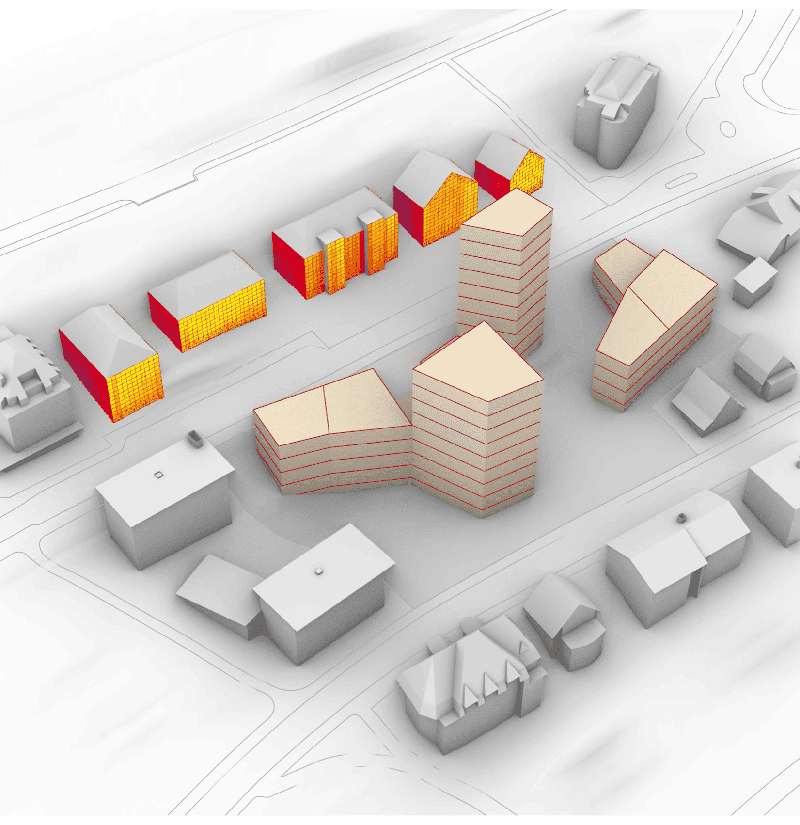
AIXD | Generative AI toolbox for architects and engineers
AIXD | Generative AI toolbox for architects and engineers


Smartair | An active learning algorithm for real-time acquisition and regression of flow field data
Smartair | An active learning algorithm for real-time acquisition and regression of flow field data
Contact us
Let’s talk Data Science
Do you need our services or expertise?
Contact us for your next Data Science project!