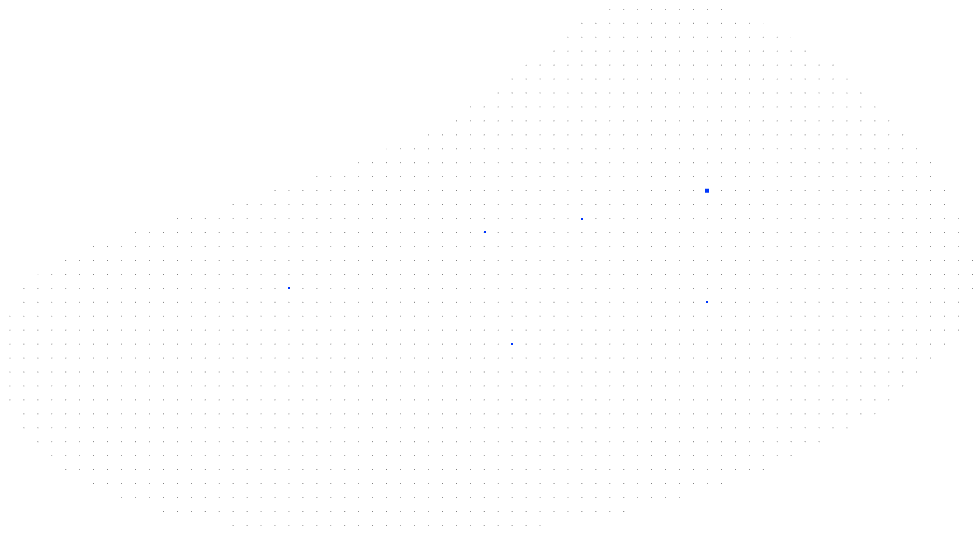
SPEED2ZERO
Sustainable pathways towards net zero Switzerland

Abstract
Climate change increasingly affects society and ecosystems, including biodiversity, and also impacts energy security in Switzerland. Climate-related weather extremes of unprecedented levels and increased weather variability on different timescales may lead to the intermittency of energy supply. Combined with changes in hydropower, these require the design of more resilient future energy systems that operate reliably even in extreme situations and years. In the scope of the SPEED2ZERO initiative, we use state-of-the-art machine learning approaches, like generative adversarial networks (GANs), variational autoencoders (VAEs), normalizing flows, and diffusion models, to name a few, as well as Earth System Model emulators to model spatially explicit temperature, precipitation, and climate extremes, combined with sampling approaches that enable the emulation of high-dimensional density distributions. These models can learn from high-resolution climate model simulations and generate new weather patterns at much lower computational cost. The project will provide crucial inputs for modeling platforms and enable the generation of climate storylines, i.e., realizations of specific extremes and compound extremes, e.g., periods that are exceptionally hot and dry at the same time.
People
Collaborators


Maxim joined the SDSC as a Senior Data Scientist in September 2023. He is interested in developing practical Machine Learning tools and deriving actionable insights for complex real-world challenges. He has worked on a variety of topics in generative AI, computer vision, and deep learning theory, with practical applications in different areas like object detection in environmental science or data-driven discovery of novel molecules in chemistry. Maxim holds a Ph.D. in computer science from the University of Basel and a B.Sc. as well as an M.Sc. in physics from ETH Zurich.
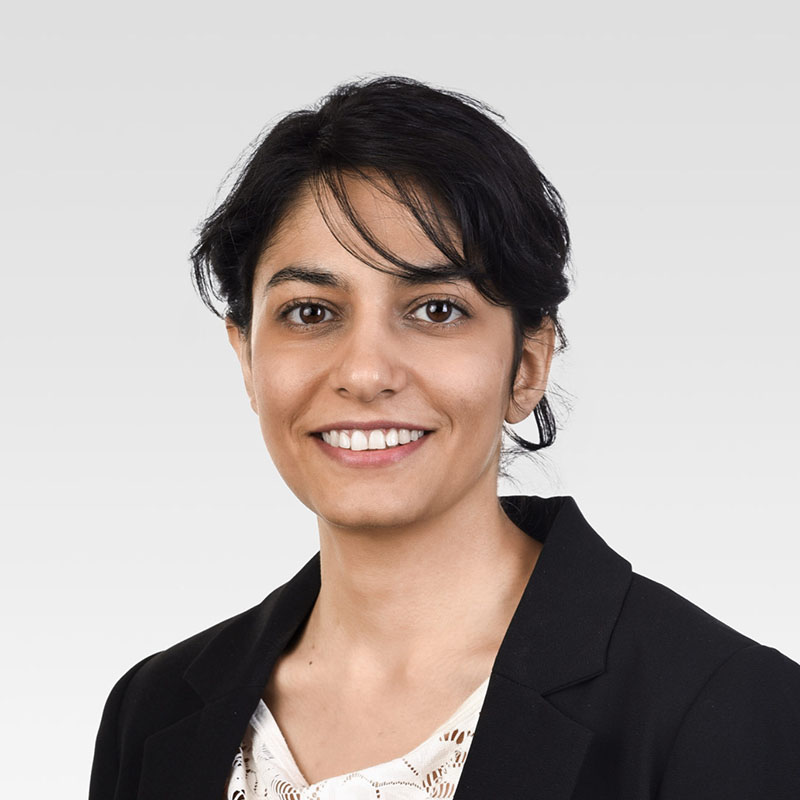
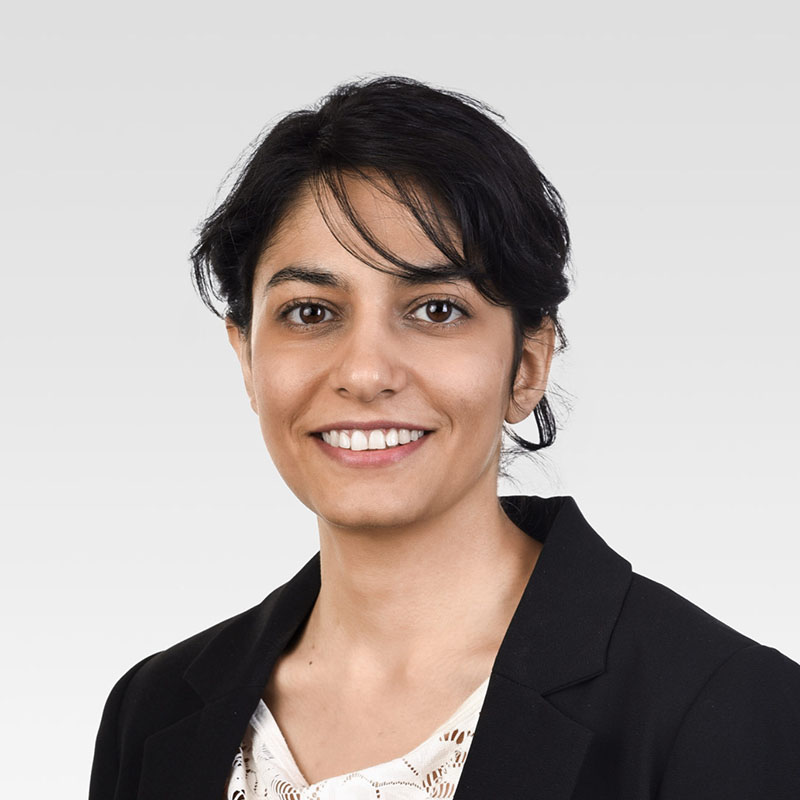
Shirin obtained her PhD in Data Science from ETH in 2024 where she focused on evaluation of high-dimensional probabilistic generative models with sequential sampling algorithms. As a Marie Skłodowska-Curie fellow, she had the opportunity to visit and collaborate with researchers at the Machine Learning groups at UC3M and Eurecom, gaining experience in parameteric and non-parameteric probabilistic models, stochastic differential equations, and optimal transport approximators. Shirin has a keen interest in studying complex stochastic dynamical systems and their application to climate and environmental sciences.
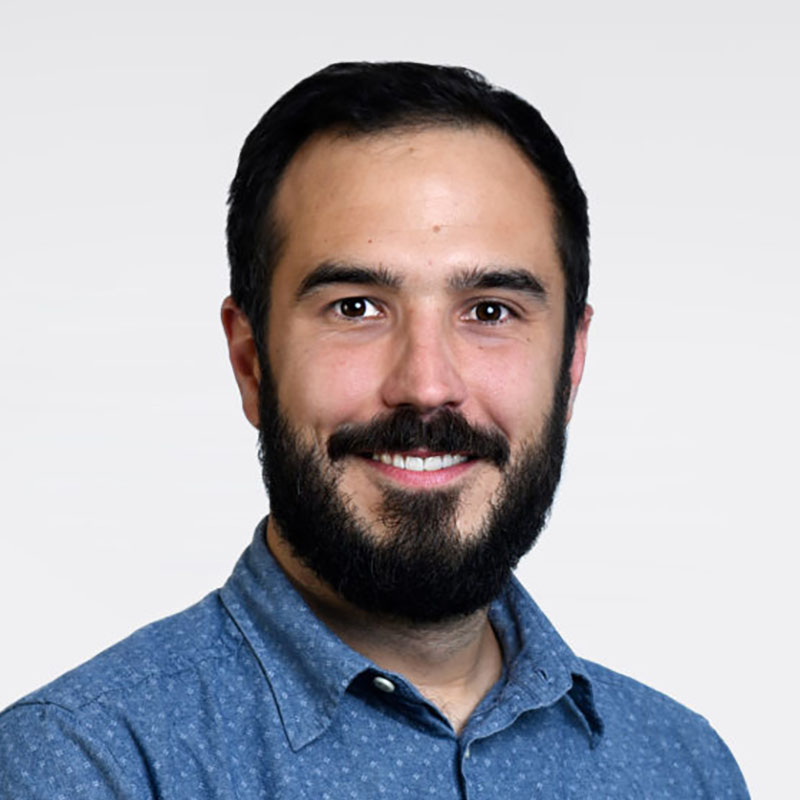
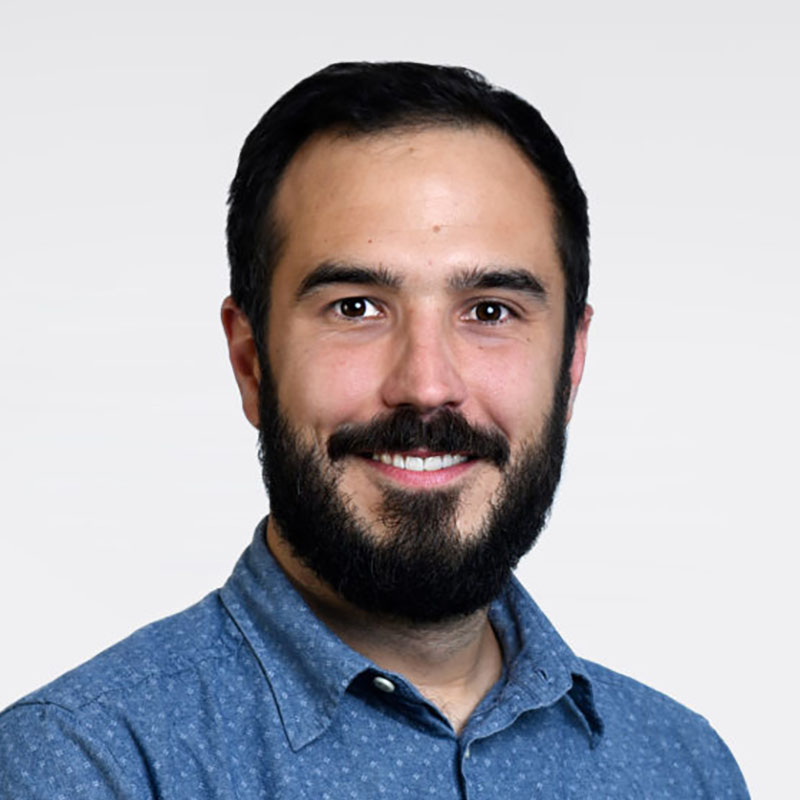
Michele received a Ph.D. in Environmental Sciences from the University of Lausanne (Switzerland) in 2013. He was then a visiting postdoc in the CALVIN group, Institute of Perception, Action and Behaviour of the School of Informatics at the University of Edinburgh, Scotland (2014-2016). He then joined the Multimodal Remote Sensing and the Geocomputation groups at the Geography department of the University of Zurich, Switzerland (2016-2017). His main research activities were at the interface of computer vision, machine and deep learning for the extraction of information from aerial photos, satellite optical images and geospatial data in general.
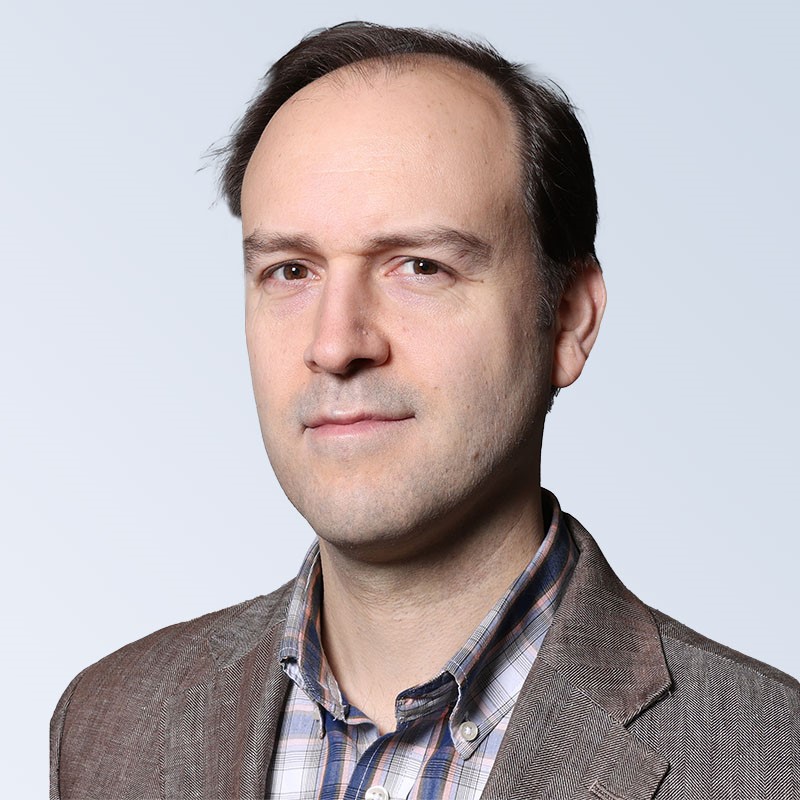
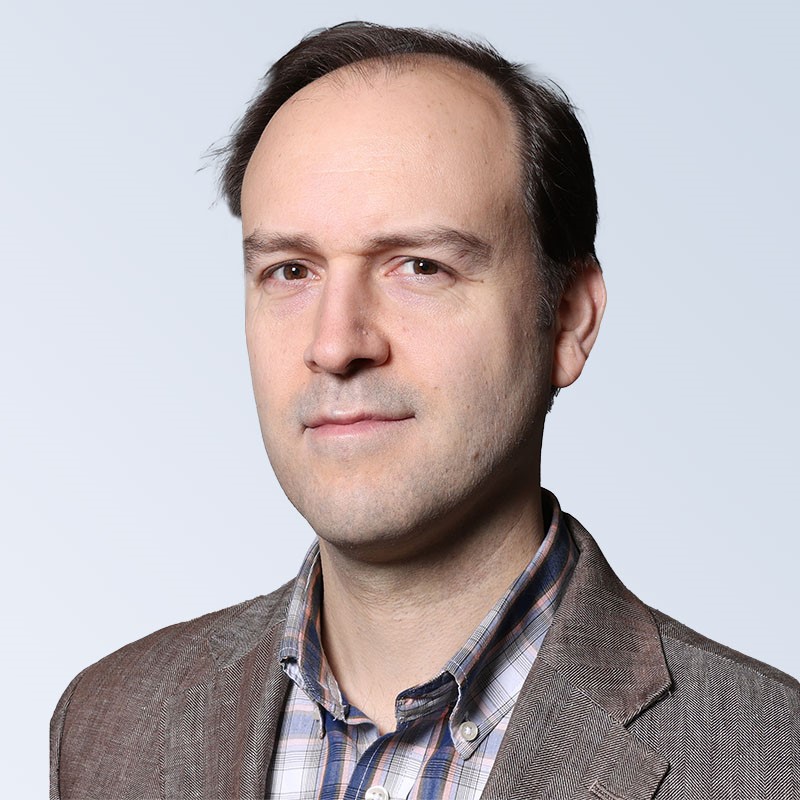
Guillaume Obozinski graduated with a PhD in Statistics from UC Berkeley in 2009. He did his postdoc and held until 2012 a researcher position in the Willow and Sierra teams at INRIA and Ecole Normale Supérieure in Paris. He was then Research Faculty at Ecole des Ponts ParisTech until 2018. Guillaume has broad interests in statistics and machine learning and worked over time on sparse modeling, optimization for large scale learning, graphical models, relational learning and semantic embeddings, with applications in various domains from computational biology to computer vision.
description
Motivation
Climate projections on regional scales are indispensable for deriving successful policies to address challenges related to energy, biodiversity, and climate change. However, typical global circulation models (GCMs) simulate climate variables like temperature or precipitation on spatial resolutions of 100 to 300 km and, thus, can only provide coarse estimates. To include regional-scale processes and local characteristics, regional climate models (RCMs) downscale outputs of GCMs to higher spatial resolutions of typically 10 to 30 km. Still, these RCM simulations can be computationally demanding and exhibit biases in the model prediction compared to actual temperature and other climate variable observations. To address these shortcomings, we develop multivariate generative downscaling approaches to generate regional-scale climate patterns from coarse-scale GCM inputs.
Proposed Approach / Solution
As a starting point for achieving the goals within the SPEED2ZERO initiative topic areas depicted in Figure 1, the SDSC's involvement focuses on developing multivariate generative downscaling approaches based on recent promising results in applying state-of-the-art architectures like GANs or diffusion models. As illustrated in Figure 2, the goal is to design an RCM emulator capable of providing several plausible climate pattern predictions, which are closer to actual observations of temperature and other climate variables.
Impact
Machine learning is increasingly becoming more important in climate modeling. In that regard, our projects will contribute state-of-the-art methods for working on challenges related to energy, biodiversity, and climate change. The SPEED2ZERO initiative will generate crucial scientific insight and develop technology, toolboxes, scenarios, and action plans with interactive visualizations to enable a sustainable transformation to a net zero greenhouse gases and biodiversity-positive Switzerland.


Presentation
Gallery
Annexe
Additional resources
Bibliography
- Sun, Y., Deng, K., Ren, K., Liu, J., Deng, C., & Jin, Y. (2024). Deep learning in statistical downscaling for deriving high spatial resolution gridded meteorological data: A systematic review. ISPRS Journal of Photogrammetry and Remote Sensing. https://doi.org/10.1016/j.isprsjprs.2023.12.011
- Ling, F., Ouyang, L., Larbi, B. R., Luo, J.-J., Han, T., Zhong, X., & Bai, L. (2024). Is artificial intelligence providing the second revolution for weather forecasting? ArXiv. https://arxiv.org/abs/2401.16669
- Fischer, E. M., Beyerle, U., Bloin-Wibe, L., Gessner, C., Humphrey, V., Lehner, F., Pendergrass, A. G., Sippel, S., Zeder, J., & Knutti, R. (2023). Storylines for unprecedented heatwaves based on ensemble boosting. Nature Communications, 14(4643). https://doi.org/10.1038/s41467-023-40112-4
- Lam, R., Sanchez-Gonzalez, A., Willson, M., Wirnsberger, P., Fortunato, M., Alet, F., Ravuri, S., Ewalds, T., Eaton-Rosen, Z., Hu, W., Merose, A., Hoyer, S., Holland, G., Vinyals, O., Stott, J., Pritzel, A., Mohamed, S., & Battaglia, P. (2023). Learning skillful medium-range global weather forecasting. Science, 382(1416-1421). https://doi.org/10.1126/science.adi2336
- Price, I., Sanchez-Gonzalez, A., Alet, F., Andersson, T. R., El-Kadi, A., Masters, D., Ewalds, T., Stott, J., Mohamed, S., Battaglia, P., Lam, R., & Willson, M. (2023). GenCast: Diffusion-based ensemble forecasting for medium-range weather. ArXiv. https://arxiv.org/abs/2312.15796
Publications
Related Pages
More projects
ML-L3DNDT
BioDetect
News
Latest news
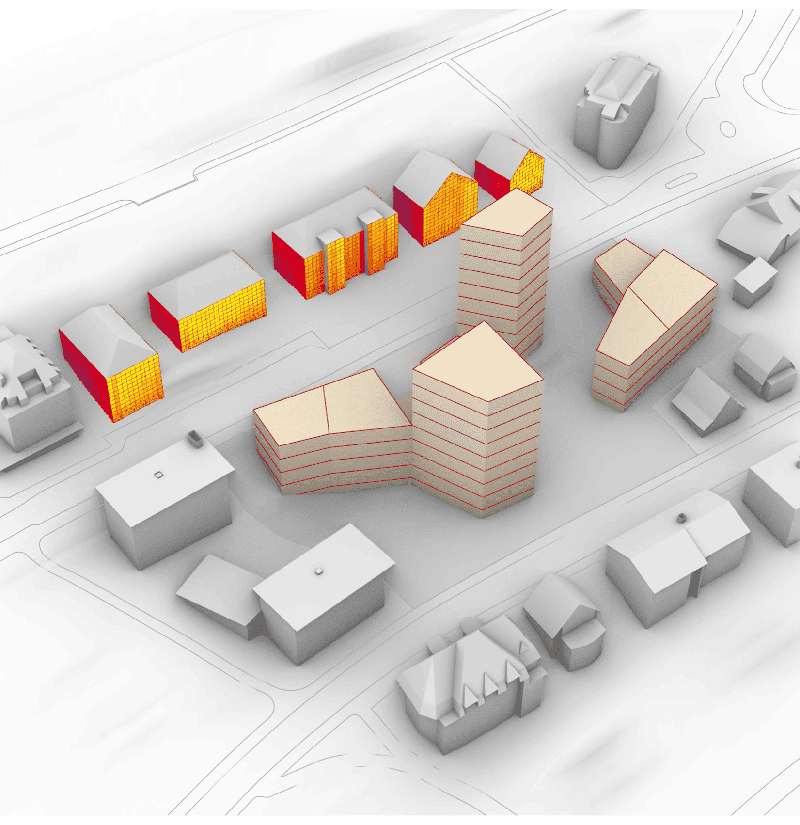
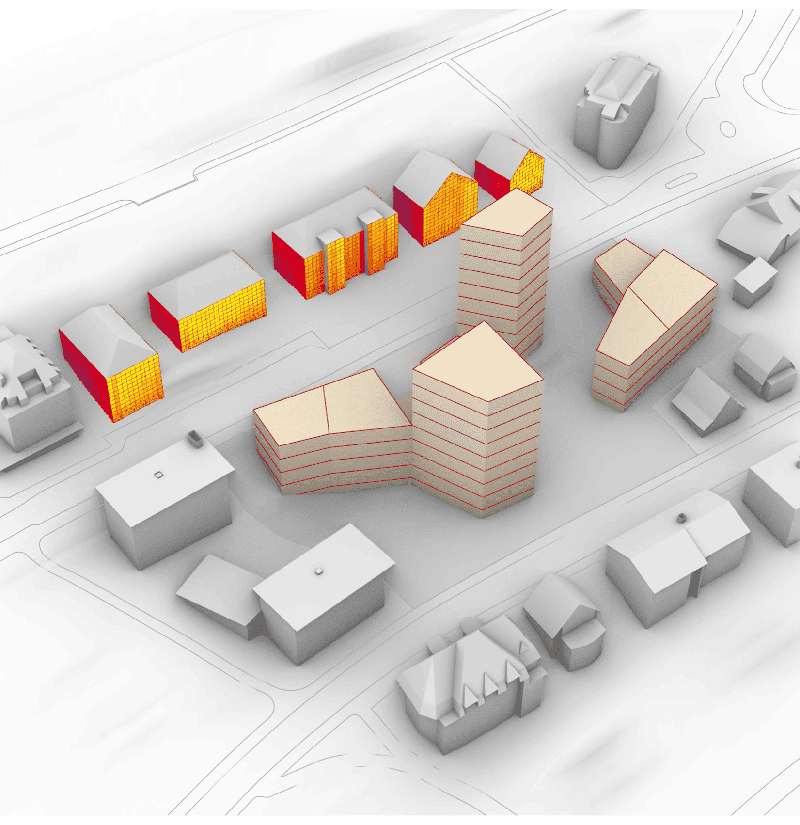
AIXD | Generative AI toolbox for architects and engineers
AIXD | Generative AI toolbox for architects and engineers


Smartair | An active learning algorithm for real-time acquisition and regression of flow field data
Smartair | An active learning algorithm for real-time acquisition and regression of flow field data
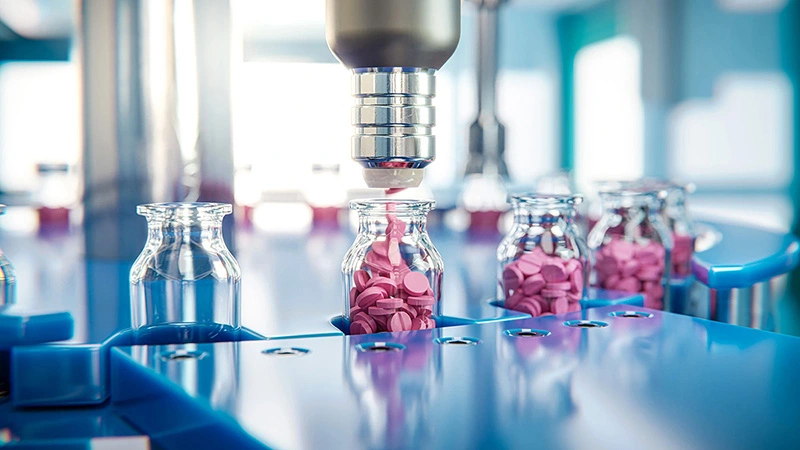
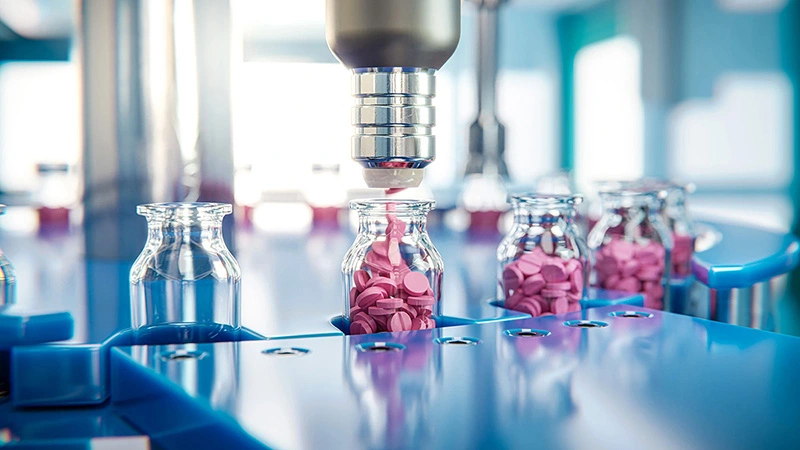
The Promise of AI in Pharmaceutical Manufacturing
The Promise of AI in Pharmaceutical Manufacturing
Contact us
Let’s talk Data Science
Do you need our services or expertise?
Contact us for your next Data Science project!