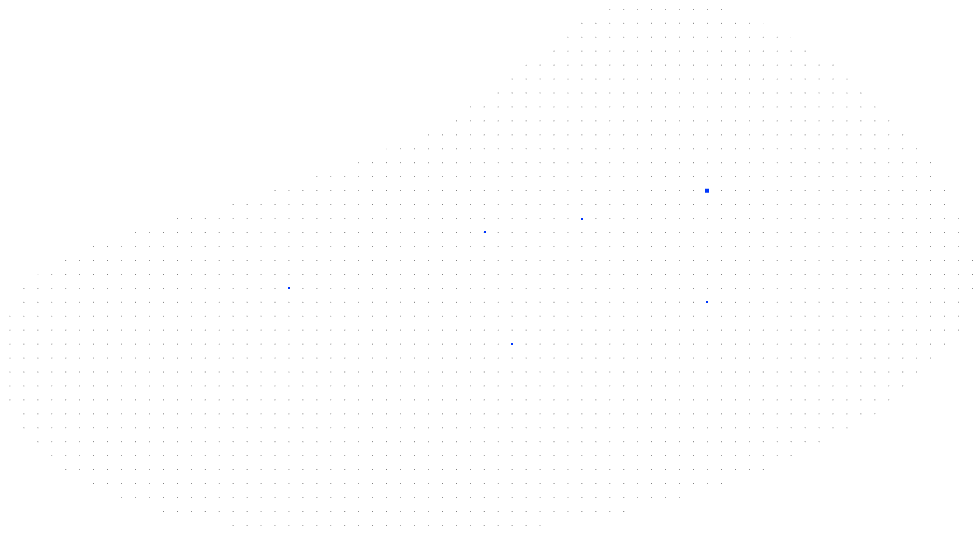
VOCIM
Directed Imitation During Vocal Learning
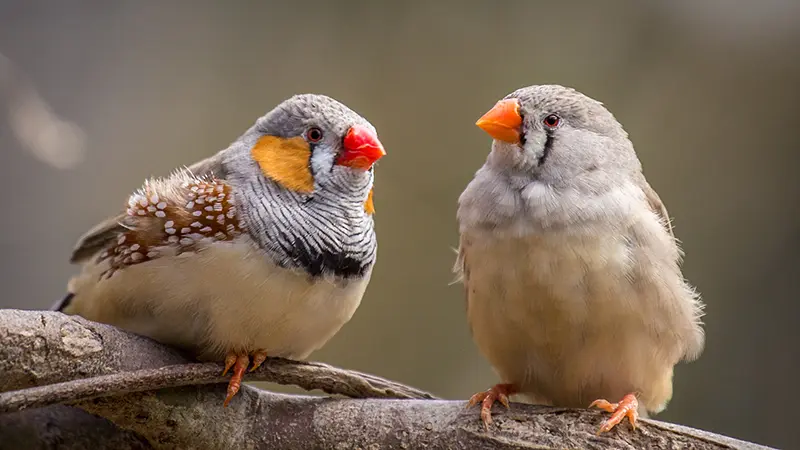
Abstract
Vocal learning is one of the great achievements of evolution, contributing to humans’ capacity of cumulative culture. How did vocal learning evolve and which are its underlying biological mechanisms? These are some of the pressing questions in language sciences and ethology. Many similarities exist between the developmental learning strategies in humans and in songbirds. In both, vocal learning depends on the interaction with experienced vocalizers. During the first year of an infant’s life, interactions with adults enable the acquisition of vocal units such as words. Similarly, in juvenile songbirds, interactions with adult singers shape the acquisition of songs. However, there are important knowledge gaps in either species about these interactions and about the extent to which they constitute attempts of immediate vocal imitations where either the tutor or the tutee tries to imitate the other. We seek to understand whether imitation attempts during tutor-tutee interactions shape the developmental learning of vocal units. Our interaction-imitation-learning (IIL) hypothesis states that the learning of a vocal unit is driven by directed speech/song and by tutee imitations, in a mechanism shared among humans and songbirds. Longitudinal data has been collected during the developmental song learning in zebra finches and humans. On the songbirds, with the video data, we aim to determine whether two songbirds are interacting with each other and what behavior they are exhibiting, and then with audio data, we learn embedded features to identify imitation events and understand how the songs develop over time by analyzing the learn embeddings. The approach can later be modified and extended to data in humans.
People
Collaborators


Xiaoran Chen joined SDSC as a senior data scientist in July 2022. Prior to this, she received her PhD at ETH Zurich in 2021. Her research was focused on unsupervised learning and anomaly detection on magnetic resonance imaging (MRI) scans. She also holds a master’s degree in bioinformatics and bachelor’s degree in biological science. Her research interest includes self-supervised learning, representation learning and general applications using machine learning methods.


Mathieu Salzmann is the Deputy Chief Data Scientist of the Swiss Data Science Center and a Senior Scientist and Lecturer at EPFL. He received his PhD from EPFL in 2009 and, between 2009 and 2015, has held post-doctoral and researcher positions at UC Berkeley, TTI-Chicago, and NICTA and the Australian National University. From May 2020 to February 2024, he was also a part-time Senior GNC Engineer at ClearSpace. Mathieu Salzmann has broad interests in machine learning and deep learning, with a particular focus on computer vision. He has published over 100 articles at top-tier peer-reviewed machine learning and computer vision venues and is a strong believer in collaborative work.
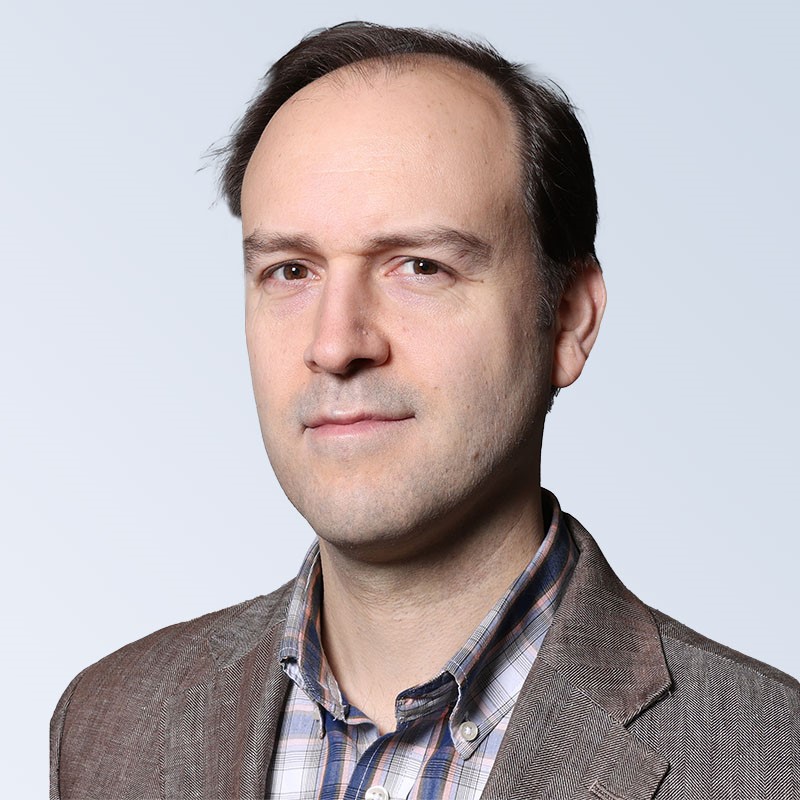
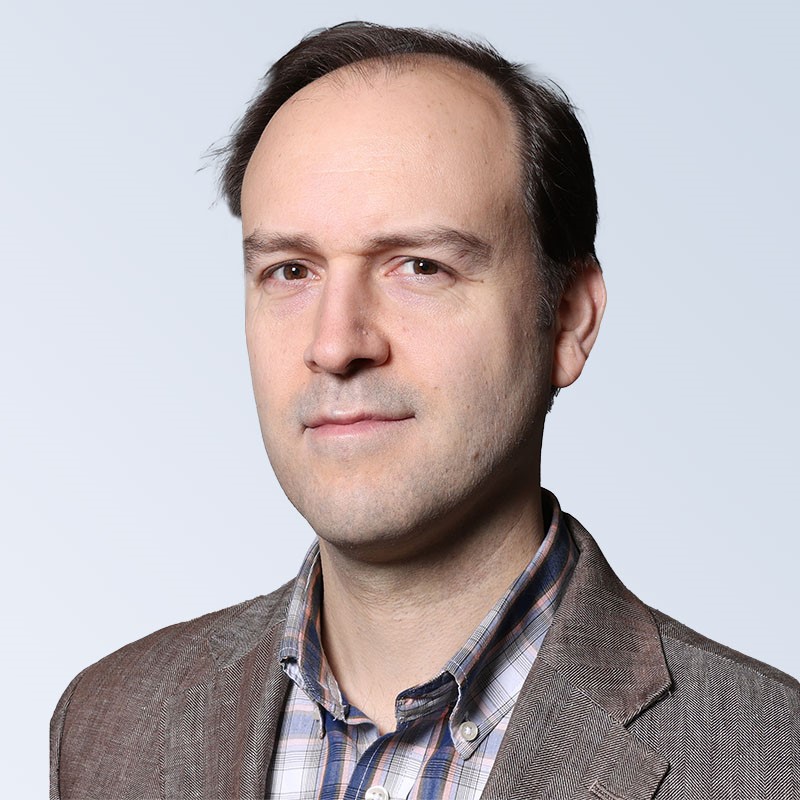
Guillaume Obozinski graduated with a PhD in Statistics from UC Berkeley in 2009. He did his postdoc and held until 2012 a researcher position in the Willow and Sierra teams at INRIA and Ecole Normale Supérieure in Paris. He was then Research Faculty at Ecole des Ponts ParisTech until 2018. Guillaume has broad interests in statistics and machine learning and worked over time on sparse modeling, optimization for large scale learning, graphical models, relational learning and semantic embeddings, with applications in various domains from computational biology to computer vision.
PI | Partners:
description
Motivation
The project aims to understand how young individuals learn vocal communication through imitating the sounds made by adults. The Interaction-Imitation-Learning (IIL) hypothesis assumes that young individuals learn to compose sentences or songs by acquiring vocal units through imitation. With a multi-modal dataset containing sounds, videos, and transcripts, the research approaches the problem by first analyzing bird behavior and bird songs, then moving towards understanding more complex learning processes in young children.

Proposed Approach / Solution
SDSC leads the work packages of bird directness estimation and feature-based imitation prediction. By determining the direction in which a bird is facing, one can estimate whether two birds are facing each other to interact. This is critical for identifying whether the juvenile is interacting with an adult bird and determining with which bird the juvenile is interacting. We trained a keypoint prediction model on videos captured from three different viewpoints to obtain a set of 2D coordinates for each individual. Utilizing the geometry relations, measured reference points and camera calibration videos, we estimated 3D coordinates from 2D coordinates of multiple views. Directedness can then be calculated by the distance and angles between the head/beak positions of a pair of individuals.
To predict imitation with a feature-based approach, embeddings of sounds produced by both juvenile and adult individuals will be learned, considering prior knowledge on which sounds are generated during interaction. These embeddings will then be used to estimate imitation events by calculating the similarity using a metric also developed in this project.

Impact
The project will provide scientific insights on language learning through imitation by young individuals as well as empirical evidence for how imitation plays a role in this process.
Presentation
Gallery
Annexe
Additional resources
Bibliography
- Nath T, Mathis A, Chen AC, Patel A, Bethge M, Mathis MW. Using DeepLabCut for 3D markerless pose estimation across species and behaviors. BioRxiv. 2018 Nov 24
- Rychen J, Rodrigues DI, Tomka T, Rüttimann L, Yamahachi H, Hahnloser RHR. A system for controlling vocal communication networks. Sci Rep. 2021 May 27;11(1):11099.
- Oord A van den, Dieleman S, Zen H, Simonyan K, Vinyals O, Graves A, et al. WaveNet: A Generative Model for Raw Audio. arXiv. 2016;
- Lipkind D, Zai AT, Hanuschkin A, Marcus GF, Tchernichovski O, Hahnloser RHR. Songbirds work around computational complexity by learning song vocabulary independently of sequence. Nat Commun. 2017 Nov 1;8(1):1247.
Publications
Related Pages
More projects
ML-L3DNDT
BioDetect
News
Latest news


Smartair | An active learning algorithm for real-time acquisition and regression of flow field data
Smartair | An active learning algorithm for real-time acquisition and regression of flow field data
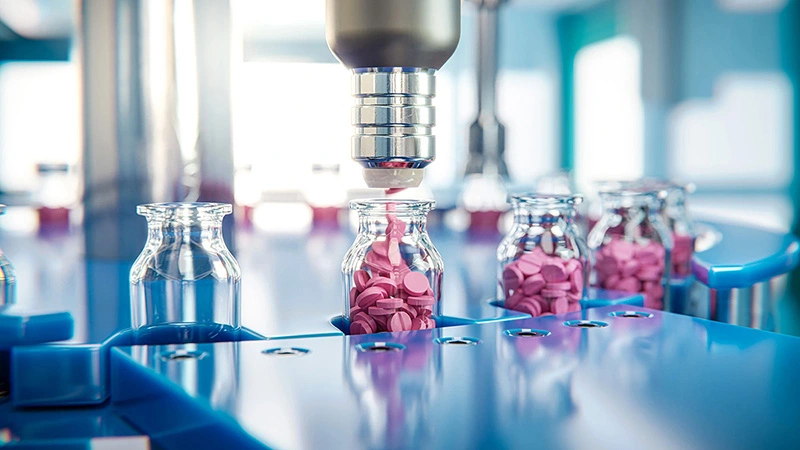
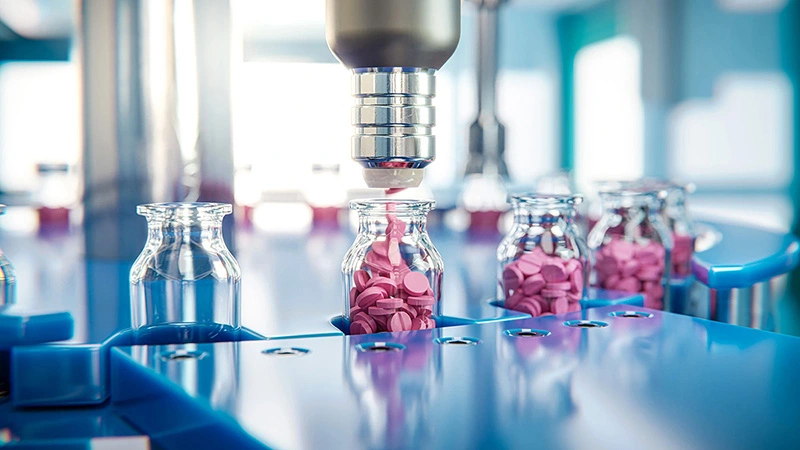
The Promise of AI in Pharmaceutical Manufacturing
The Promise of AI in Pharmaceutical Manufacturing


Efficient and scalable graph generation through iterative local expansion
Efficient and scalable graph generation through iterative local expansion
Contact us
Let’s talk Data Science
Do you need our services or expertise?
Contact us for your next Data Science project!