
NLP
Narratives in Law and Politics: A Computational Linguistics Approach

Abstract
The goal of this research is to leverage data science tools to the challenge of measuring narratives in legal and political texts, and then to analyze the causes and consequences of those narratives in the political economy. This proposal outlines a system for taking the plain text of documents, extracting the latent narrative structures in the text, and extracting the narratives that are causally related to some cause or outcome. The system will be applied to an analysis of prejudicial narratives in law, politics, and mass media. A data-driven approach that can extract text-based measures of narratives would mark a major step forward in this field. Many phenomena of interest to historians and social scientists are embedded in text, so the development and application of natural language processing methods to the analysis of narratives will be useful to researchers in a variety of fields and settings. In more specific reference to SDSC goals, this research seeks to make progress on an interdisciplinary data science problem (measuring narratives and analyzing associated causal links) and then focus on applications in a social-science domain (political economy of law and mass media). The deep learning models described below require significant computational infrastructure and expertise. To accelerate the empirical analysis by domain social scientists, we seek support from SDSC in data storage and management, implementation of statistical and machine learning models, and the development of compelling data visualizations. Our team share a core belief in reproducibility and open science initiatives.
People
Collaborators
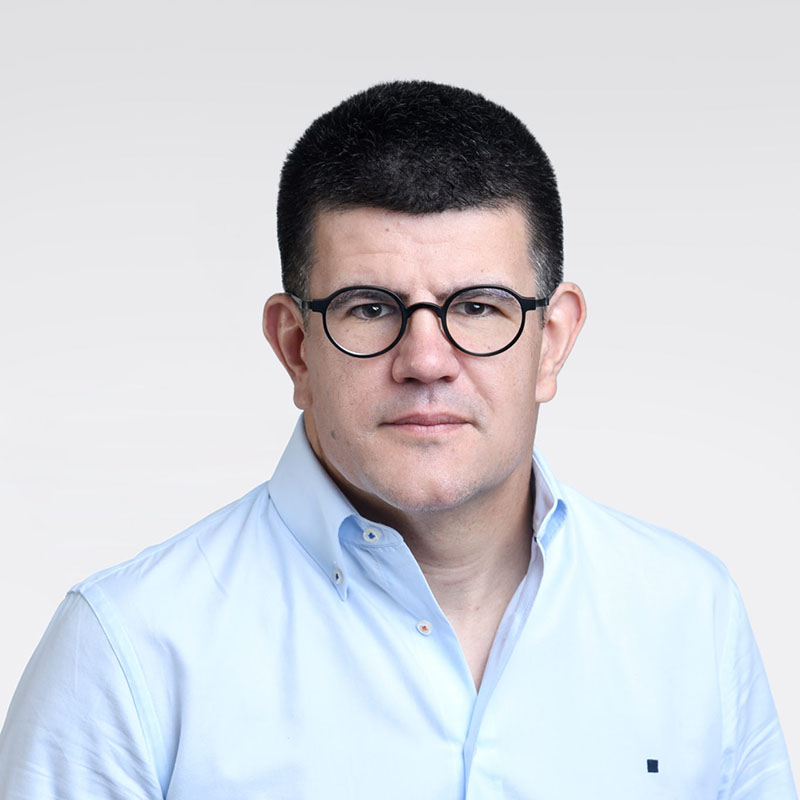
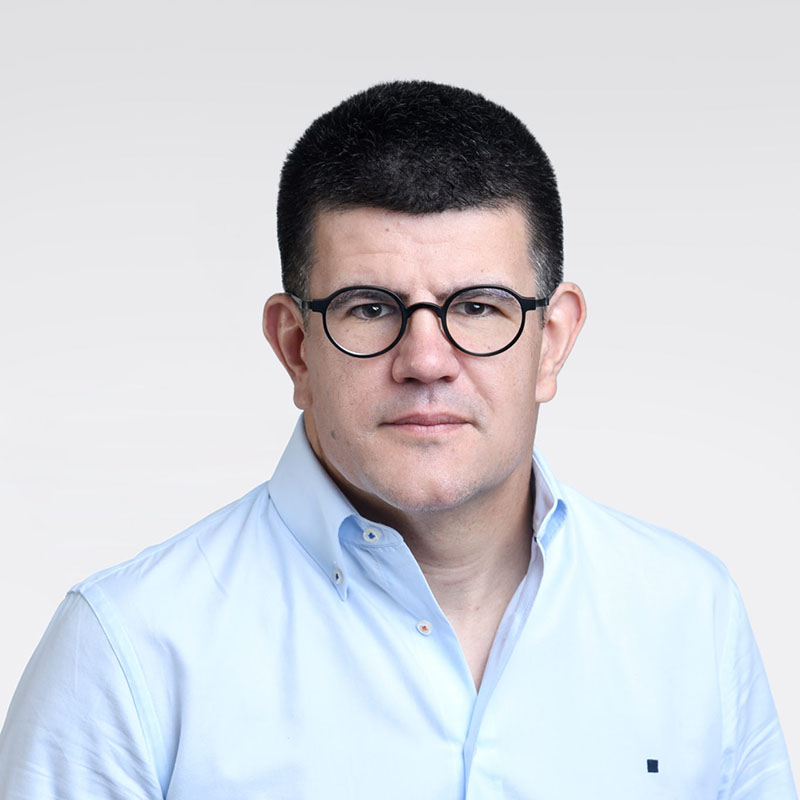
Fernando Perez-Cruz received a PhD. in Electrical Engineering from the Technical University of Madrid. He is Titular Professor in the Computer Science Department at ETH Zurich and Head of Machine Learning Research and AI at Spiden. He has been a member of the technical staff at Bell Labs and a Machine Learning Research Scientist at Amazon. Fernando has been a visiting professor at Princeton University under a Marie Curie Fellowship and an associate professor at University Carlos III in Madrid. He held positions at the Gatsby Unit (London), Max Planck Institute for Biological Cybernetics (Tuebingen), and BioWulf Technologies (New York). Fernando Perez-Cruz has served as Chief Data Scientist at the SDSC from 2018 to 2023, and Deputy Executive Director of the SDSC from 2022 to 2023
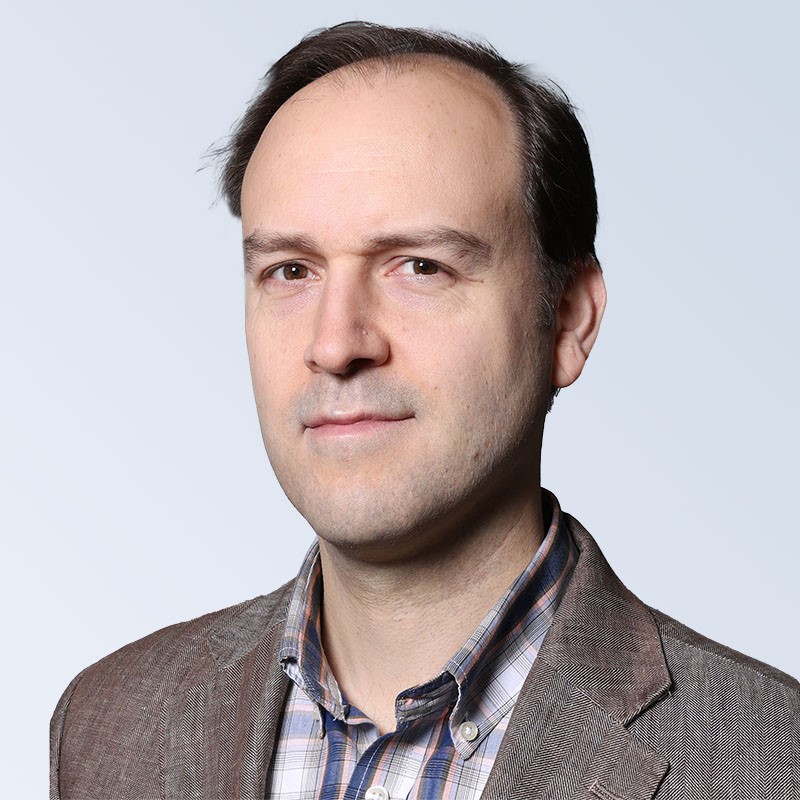
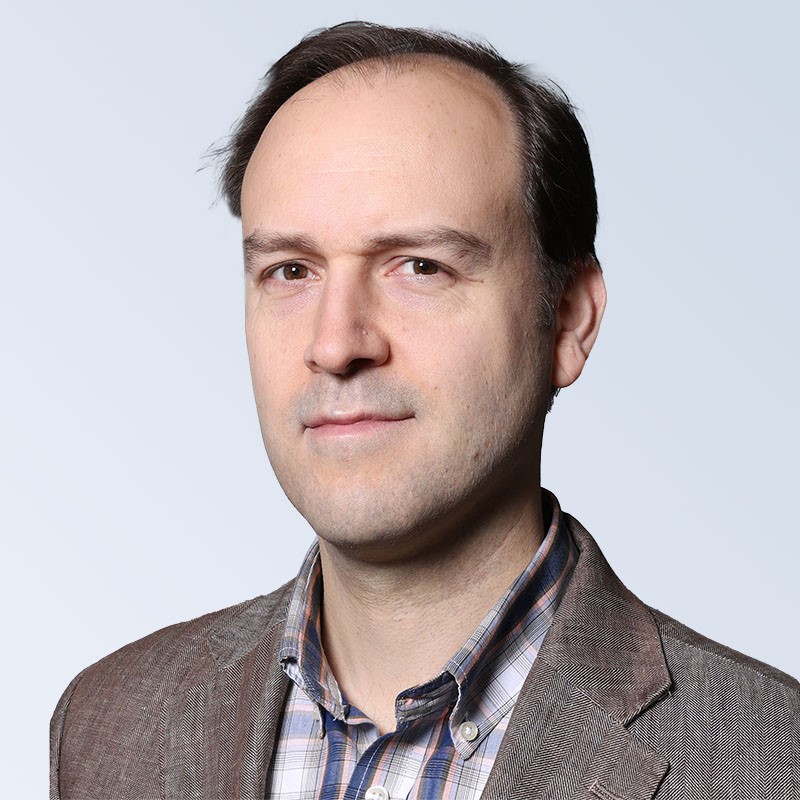
Guillaume Obozinski graduated with a PhD in Statistics from UC Berkeley in 2009. He did his postdoc and held until 2012 a researcher position in the Willow and Sierra teams at INRIA and Ecole Normale Supérieure in Paris. He was then Research Faculty at Ecole des Ponts ParisTech until 2018. Guillaume has broad interests in statistics and machine learning and worked over time on sparse modeling, optimization for large scale learning, graphical models, relational learning and semantic embeddings, with applications in various domains from computational biology to computer vision.
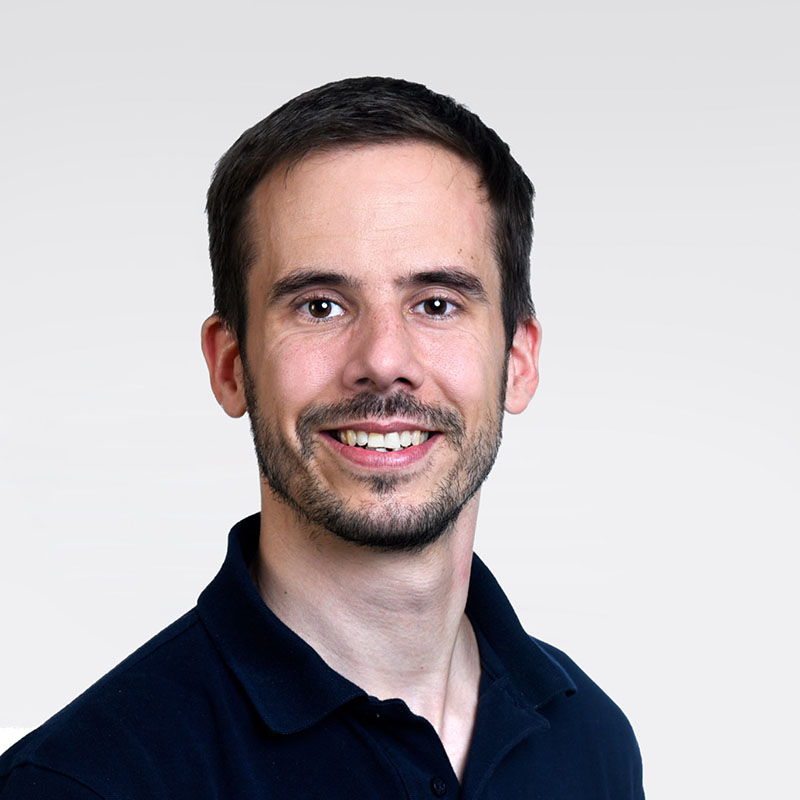
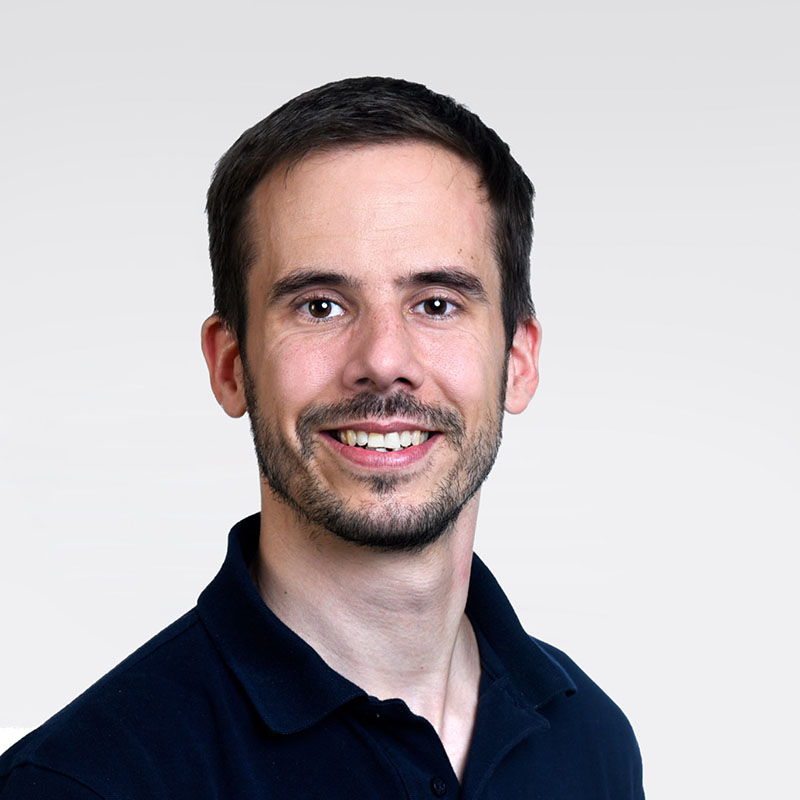
Luis is originally from Spain, where he completed his bachelor's studies in Electrical engineering, and the Ms.C. on signal theory and communications, both at the University of Seville. During his Ph.D. he started focusing on machine learning methods, more specifically message passing techniques for channel coding, and Bayesian methods for channel equalization. He carried it out between the University of Seville and the University Carlos III in Madrid, also spending some time at the EPFL, Switzerland, and Bell Labs, USA, where he worked on advanced techniques for optical channel coding. When he completed his Ph.D. in 2013, he moved to the Luxembourg Center on Systems Biomedicine, where he switched his interest to neuroscience, neuroimaging, life sciences, etc., and the application of machine learning techniques to these fields. During his 4 and a half years there as a Postdoc, he worked on many different problems as a data scientist, encompassing topics such as microscopy image analysis, neuroimaging, single-cell gene expression analysis, etc. He joined the SDSC in April 2018. As Lead Data Scientist, Luis coordinates projects in various domains. Several projects focus on the application of natural language processing and knowledge graphs to the study of different phenomena in social and political sciences. In the domains of architecture and engineering, Luis is responsible for projects centered on the application of novel generative methods to parametric modeling. Finally, Luis also coordinates different projects in robotics, ranging from collaborative robotic construction to deformable object manipulation.
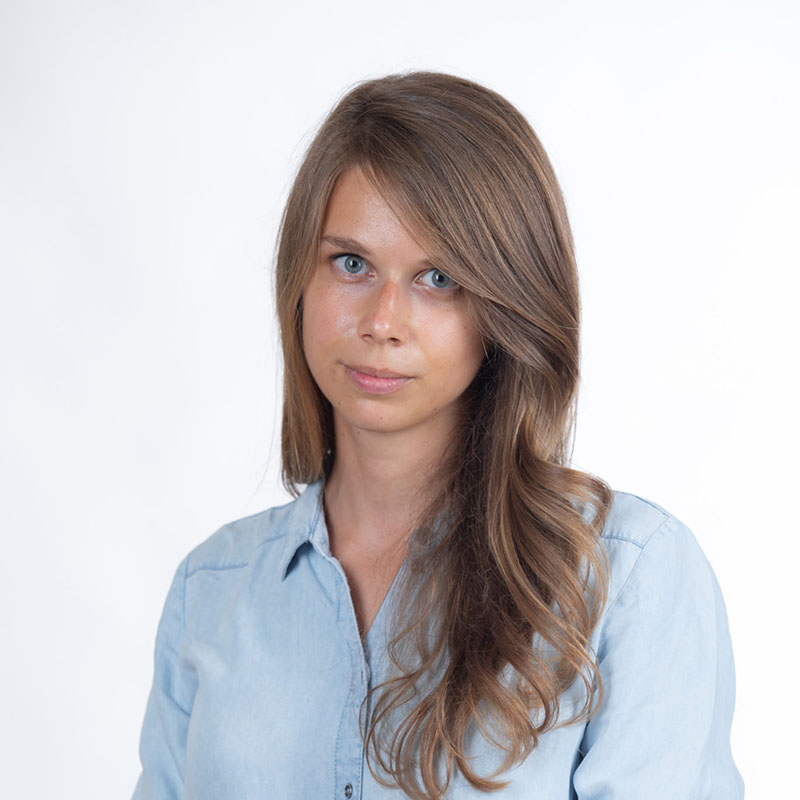
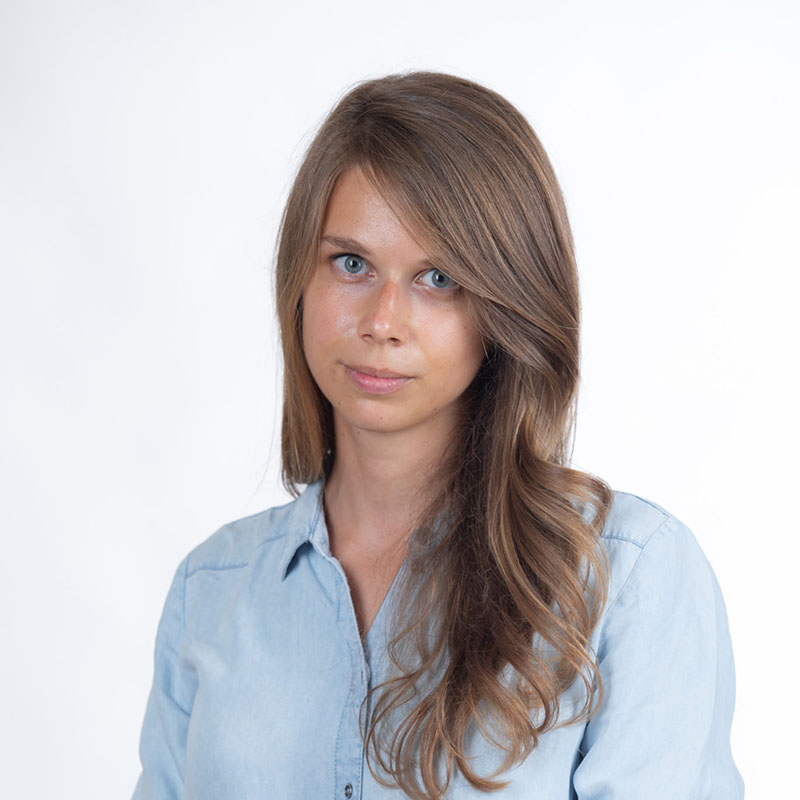
Natasa is a computer scientist by training, with a Bachelor degree in Computer Science and Engineering and Master degree in Embedded Systems from Ss. Cyril and Methodius University in Skopje, North Macedonia. She finished her doctoral studies in Information Systems at UNIL- HEC Lausanne, under the supervision of Valérie Chavez-Demoilun. During her studies she did an internship at NATO and Facebook AI Research. Her research interest focuses on causal inference, generative models, uncertainty and interpretability.
description
Problem:
- This project aims at leveraging data science approaches to (i) extract narratives in legal and political texts, and (ii) analyze the causes and consequences of those narratives in political economy. Such objectives require application and adoptation of natural language processing tools as well as data-driven techniques for causal discovery and inference in high dimensional data.
Impact:
- The findings – methods as well as estimates of the real-world impacts of narratives in the political economy – will be useful to social scientists and policymakers interested in the political and cultural factors underlying social disadvantage. Additionaly, working with text data introduces a challenge, leading to new research question to be tackled with inovative machine learning tools.
Presentation
Gallery
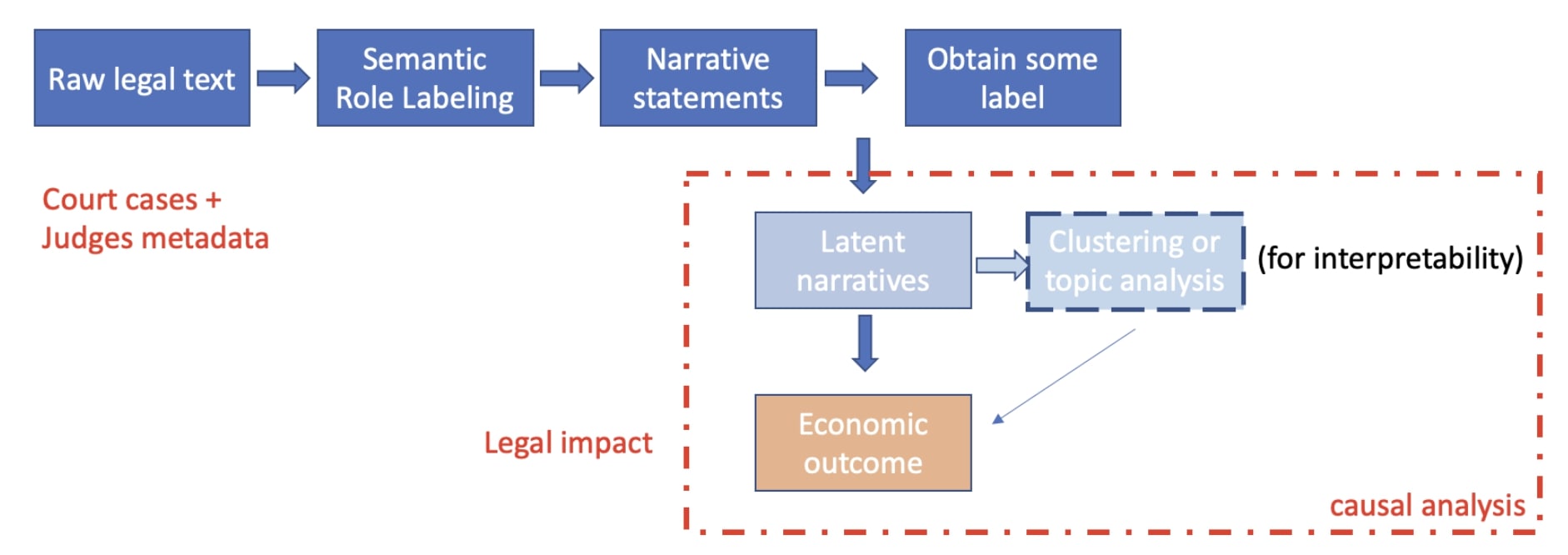
Annexe
Additionnal resources
Bibliography
- Angrist, J. and Pischke, J.-S. (2009). Mostly Harmless Econometrics: An empiricist’s companion. Princeton University Press, Princeton, NJ.
- Ash, E., Chen, D. L., and Naidu, S. (2017a). Ideas have consequences: The impact of law and economics on American justice. Technical report, NBER.
- Egami, N., Fong, C. J., Grimmer, J., Roberts, M. E., and Stewart, B. M. (2017). How to make causal inferences using texts.
- Galletta, S., Ash, E., and Chen, D. L. (2019). Do judicial sentiments affect social attitudes?
- Shiller, R. J. (2017). Narrative economics. American Economic Review, 107(4):967–1004.
Publications
Related Pages
More projects
CLIMIS4AVAL
News
Latest news
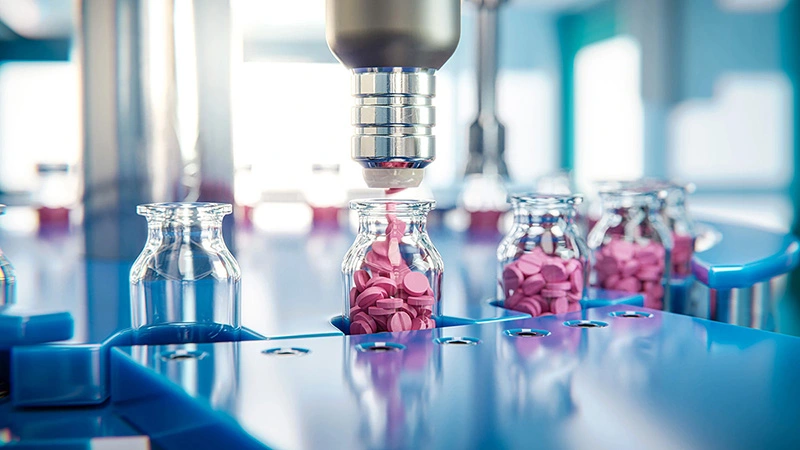
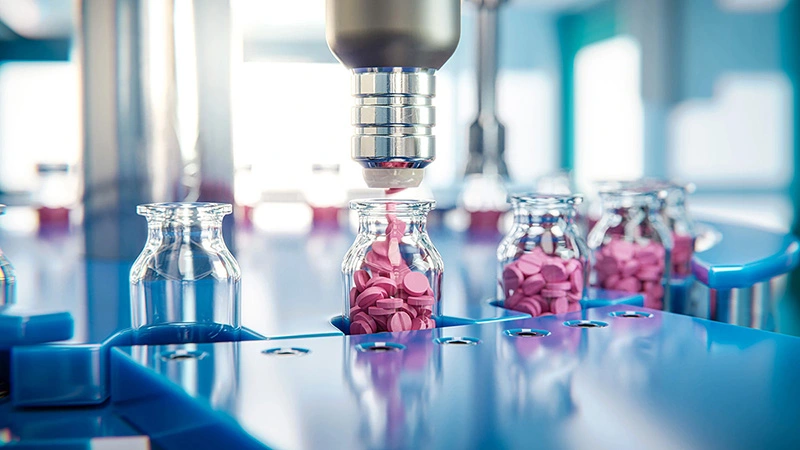
The Promise of AI in Pharmaceutical Manufacturing
The Promise of AI in Pharmaceutical Manufacturing
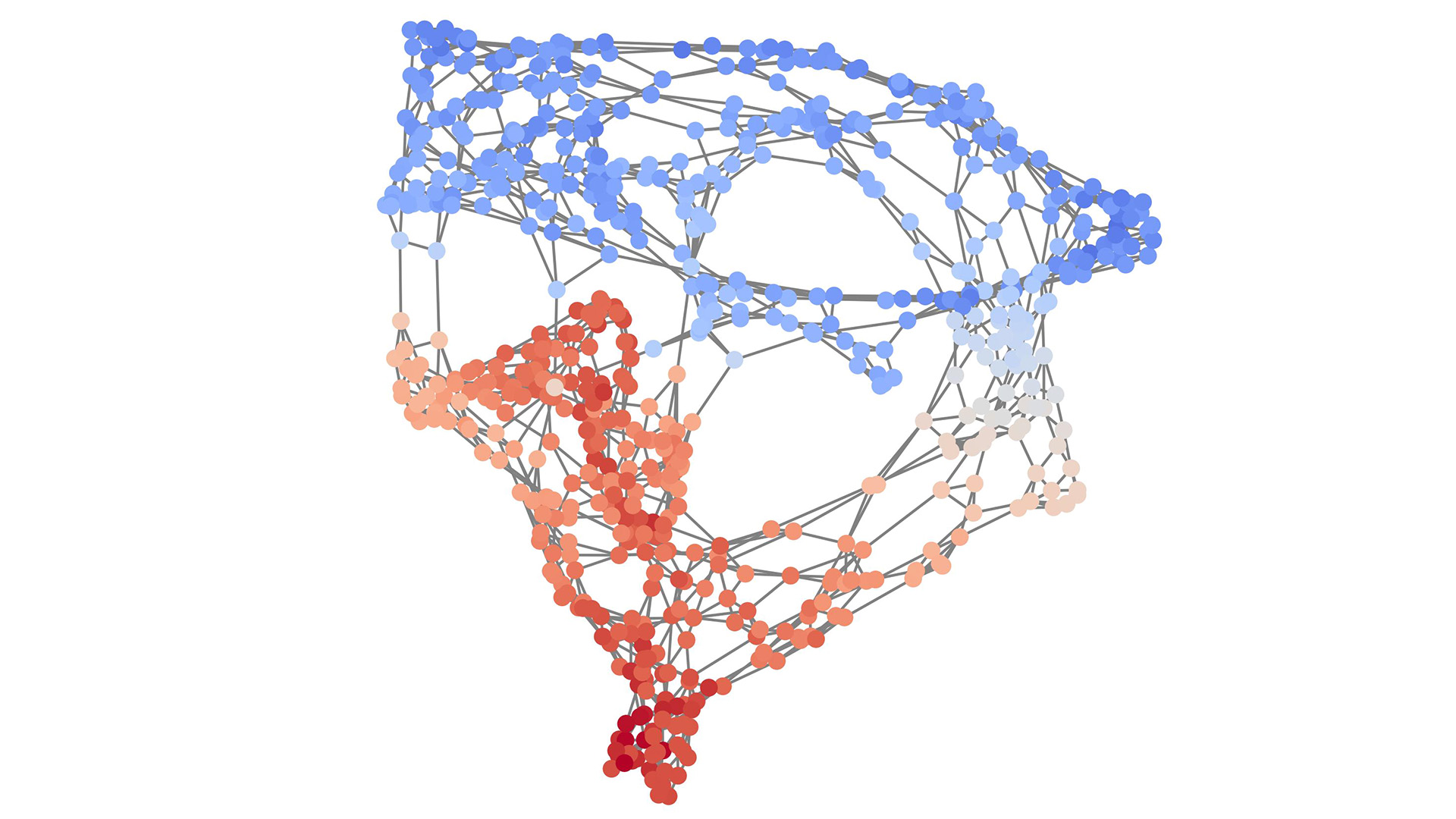
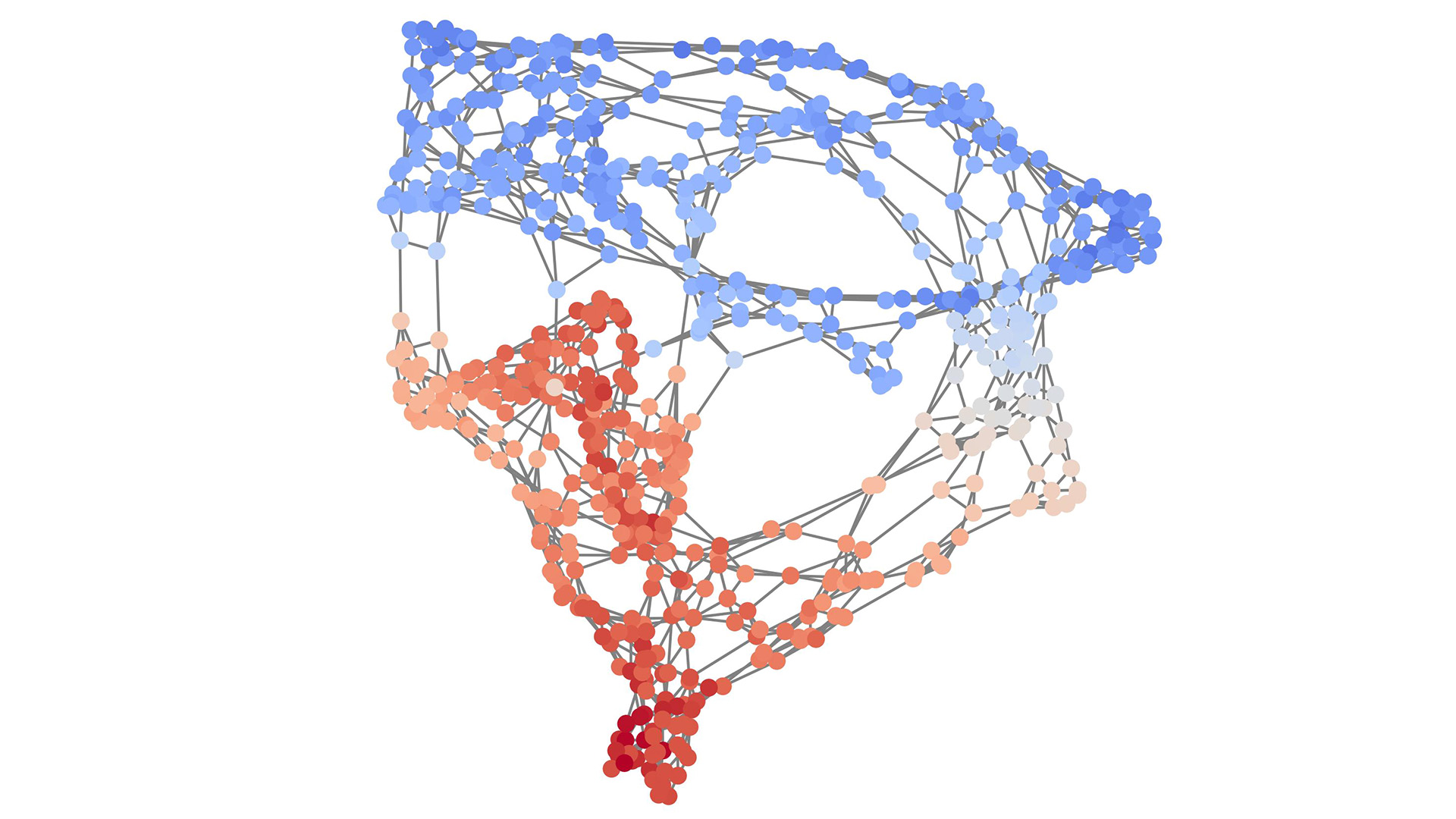
Efficient and scalable graph generation through iterative local expansion
Efficient and scalable graph generation through iterative local expansion


RAvaFcast | Automating regional avalanche danger prediction in Switzerland
RAvaFcast | Automating regional avalanche danger prediction in Switzerland
Contact us
Let’s talk Data Science
Do you need our services or expertise?
Contact us for your next Data Science project!