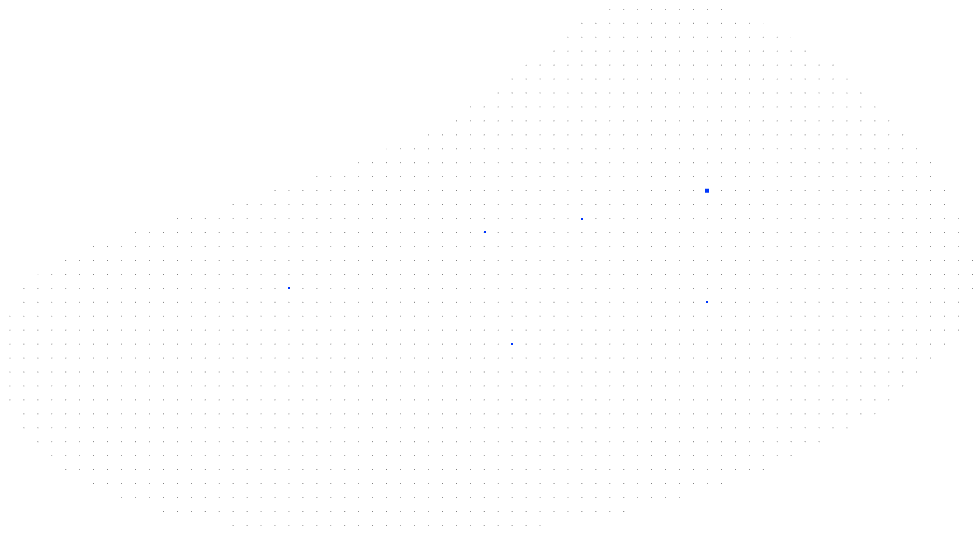
DNAi
High throughput eDNA processing using artificial intelligence for ecosystem monitoring

Abstract
The current biodiversity crisis demands novel approaches to monitor how human activities influence the biosphere. The rapid development of ‘omics’ tools, in particular the metabarcoding of environmental DNA (eDNA), have opened a new area of comprehensive biodiversity data generation across many regions of the world. Yet, the development of efficient data processing pipelines has not matched the exponential increase in size and quality of ‘omics’ data, which limits the application of eDNA for ecological monitoring. Currently, processing eDNA requires multiple expensive and error prone bioinformatic steps, with each step relying on poorly automatized disparate software, and many paths to choose with output results sensitive to subjective decisions.
Moving toward large-scale biodiversity monitoring requires a fast, objective, and automated processing pipeline that will transform eDNA data into meaningful information about ecosystems including (i) standardized taxonomic lists for each sampled location that guide species management, (ii) standardized classification of samples from their DNA composition which can guide ecosystem management. In this project, we propose to harness a combination of recent machine learning approaches that directly transforms raw eDNA metabarcoding data into informative ecological indicators that improves ecosystem monitoring and decision making.
People
Collaborators


Steven Stalder joined the SDSC in 2022 as a Data Scientist in the academia team. He received both his BSc and MSc in computer science from ETH Zürich, with a main focus on machine learning and high-performance computing. His first contact with the SDSC was during his master’s thesis, where he worked on explainable neural network models for image classification. Outside of work, Steven loves playing football, reading an interesting book, or watching a good movie.
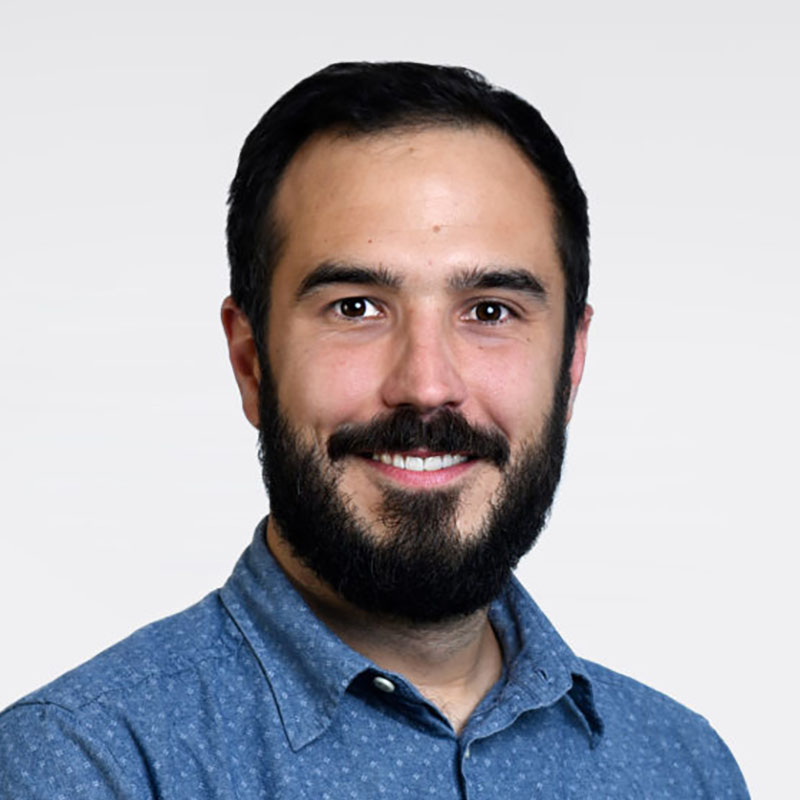
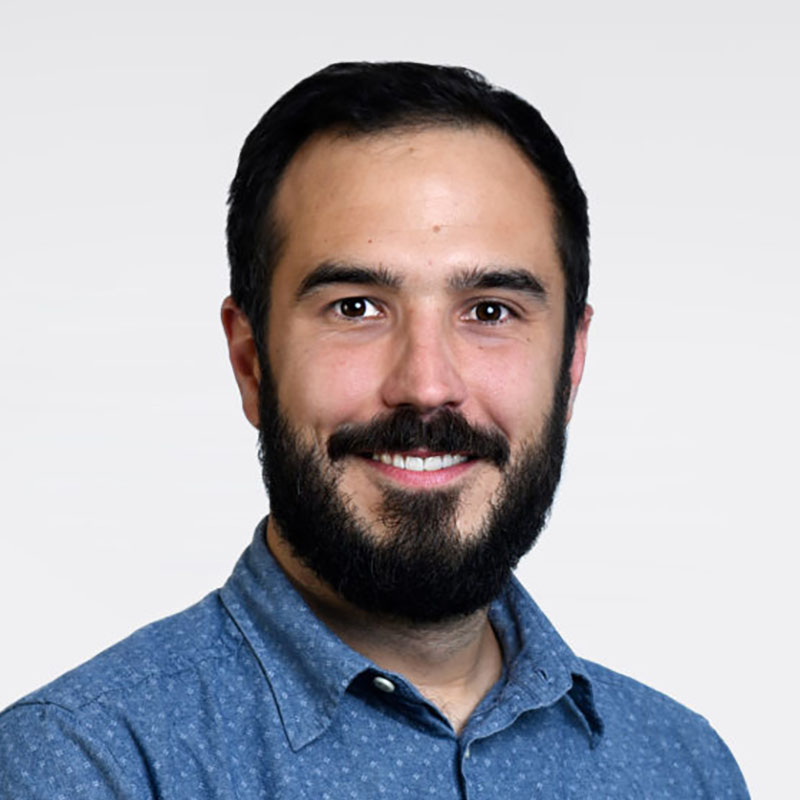
Michele received a Ph.D. in Environmental Sciences from the University of Lausanne (Switzerland) in 2013. He was then a visiting postdoc in the CALVIN group, Institute of Perception, Action and Behaviour of the School of Informatics at the University of Edinburgh, Scotland (2014-2016). He then joined the Multimodal Remote Sensing and the Geocomputation groups at the Geography department of the University of Zurich, Switzerland (2016-2017). His main research activities were at the interface of computer vision, machine and deep learning for the extraction of information from aerial photos, satellite optical images and geospatial data in general.
description
Motivation
Human-related disturbances are affecting all ecosystems of the world from terrestrial to marine habitats, threatening biodiversity and disrupting ecosystem services. Thus, monitoring ecosystems and how they respond to human influences, is crucial. eDNA has revolutionized biodiversity monitoring, offering non-invasive means to assess ecosystem health. However, the complexity of eDNA data poses significant challenges for conventional bioinformatics. This project aims to tackle some of these challenges by relying on novel machine learning methods.
Proposed Approach / Solution
The project first tackles the problem of ordination of uncurated eDNA samples, where the aim is to find low-dimensional representations of the data highlighting the main ecological gradients. Lacking ground truth data for this task, a contrastive self-supervised learning approach is paired with an attention-based neural network in order to extract the main distinguishing factors in eDNA samples consisting of large amounts of uncurated DNA strings. The learned latent representations of eDNA samples will later also be directly used as inputs for downstream estimations of various ecosystem properties of interest. Another problem tackled in DNAi is the identification of taxonomic compositions in eDNA samples, given the lack of complete reference databases. This project aims to utilize information from phylogenetic trees as well as species co-occurrence data. Here, we develop another method relying on neural networks to classify the DNA of species that do not yet have an entry in a reference database, in a zero-shot learning setting. Figure 1 provides a schematic overview of the different work packages.

Impact
The methods and models developed in this project have the potential to transform how eDNA data is parsed, processed and analyzed by ecologists and practitioners. This, in turn, affects the monitoring of environmental health - a crucial task considering the global biodiversity crisis.
Presentation
Gallery
Annexe
Additional resources
Bibliography
- Flück, B., Mathon, L., Manel, S., Valentini, A., Dejean, T., Albouy, C., ... & Pellissier, L. (2022). Applying convolutional neural networks to speed up environmental DNA annotation in a highly diverse ecosystem. Scientific Reports, 12(1), 10247. https://doi.org/10.1038/s41598-022-13412-w
- Cordier, T., Lanzén, A., Apothéloz-Perret-Gentil, L., Stoeck, T., & Pawlowski, J. (2019). Embracing environmental genomics and machine learning for routine biomonitoring. Trends in microbiology, 27(5), 387-397. https://doi.org/10.1016/j.tim.2018.10.012
- Gauch Jr, H. G. (1982). Noise reduction by eigenvector ordinations. Ecology, 63(6), 1643-1649. https://doi.org/10.2307/1940105
- Schroff, F., Kalenichenko, D., & Philbin, J. (2015). Facenet: A unified embedding for face recognition and clustering. In Proceedings of the IEEE conference on computer vision and pattern recognition (pp. 815-823). https://doi.org/10.1109/CVPR.2015.7298682
Publications
Related Pages
- Public source code for work package 2: DNAi / ORDNA · GitLab
More projects
ML-L3DNDT
BioDetect
News
Latest news


First National Calls: 50 selected projects to start in 2025
First National Calls: 50 selected projects to start in 2025
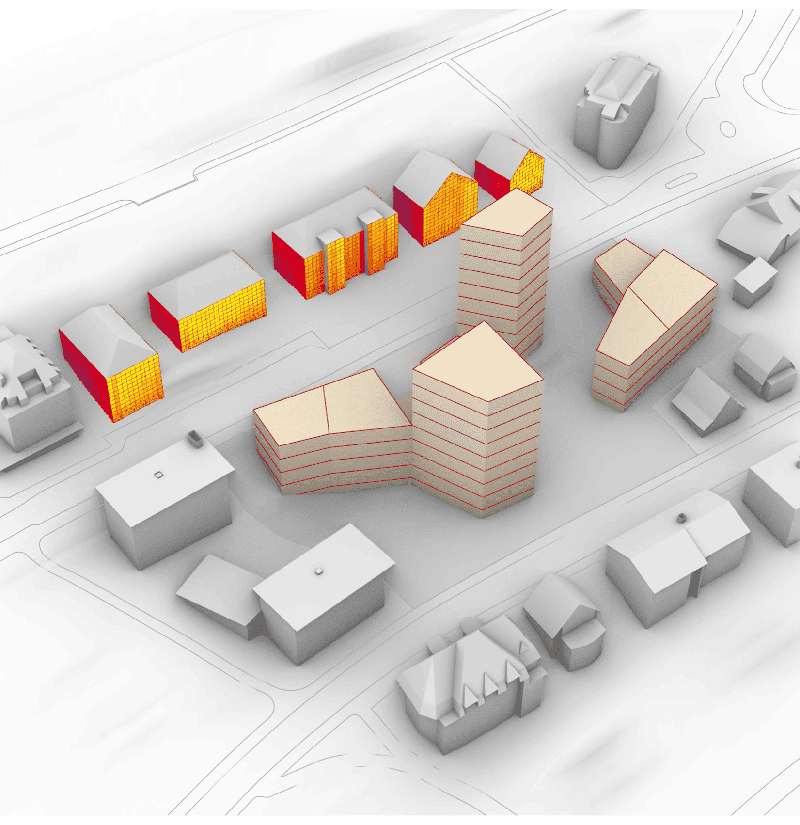
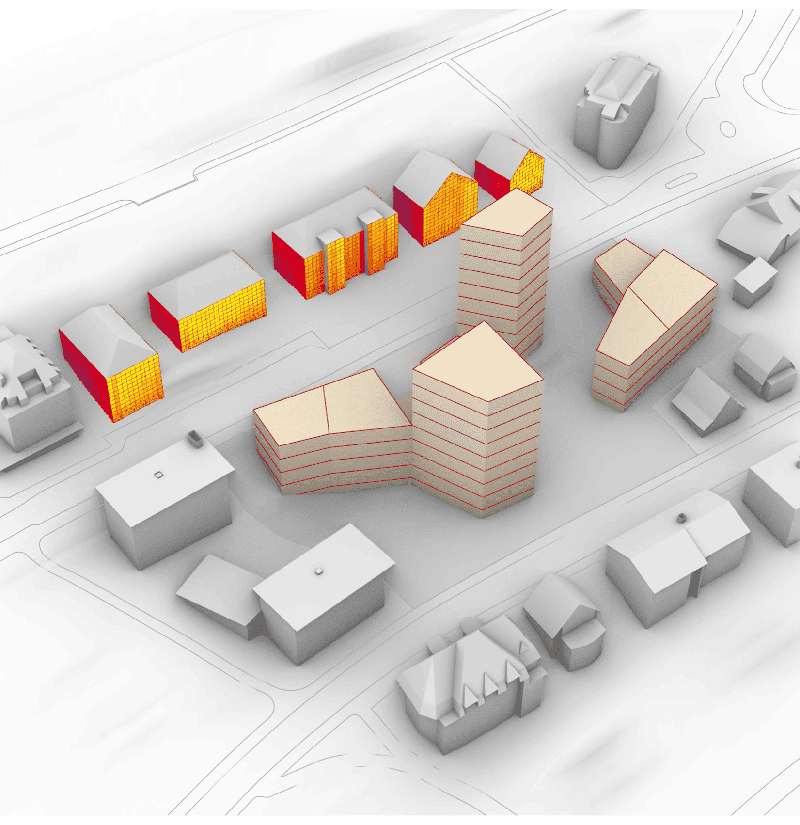
AIXD | Generative AI toolbox for architects and engineers
AIXD | Generative AI toolbox for architects and engineers


Smartair | An active learning algorithm for real-time acquisition and regression of flow field data
Smartair | An active learning algorithm for real-time acquisition and regression of flow field data
Contact us
Let’s talk Data Science
Do you need our services or expertise?
Contact us for your next Data Science project!