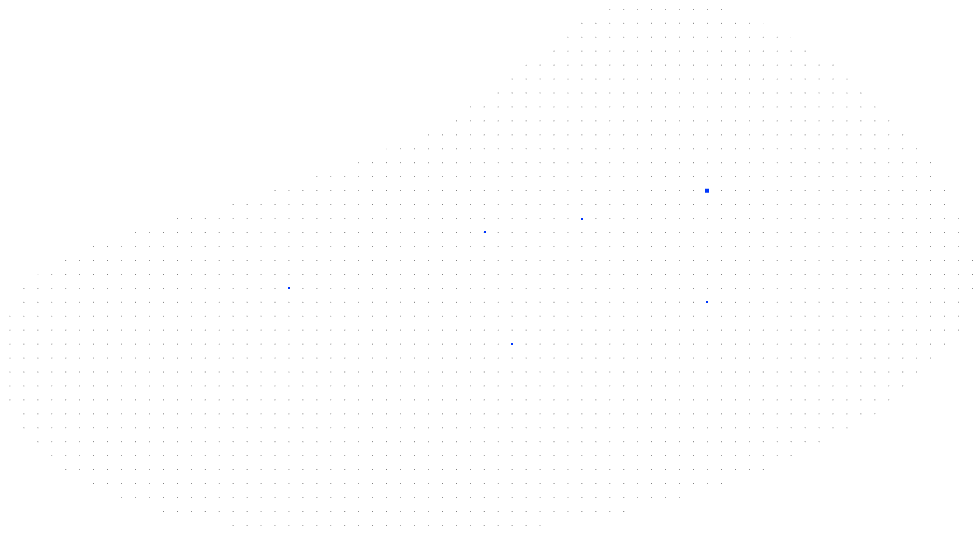
DrSCS
Predicting subclonal drug response from single-cell sequencing for precision oncology
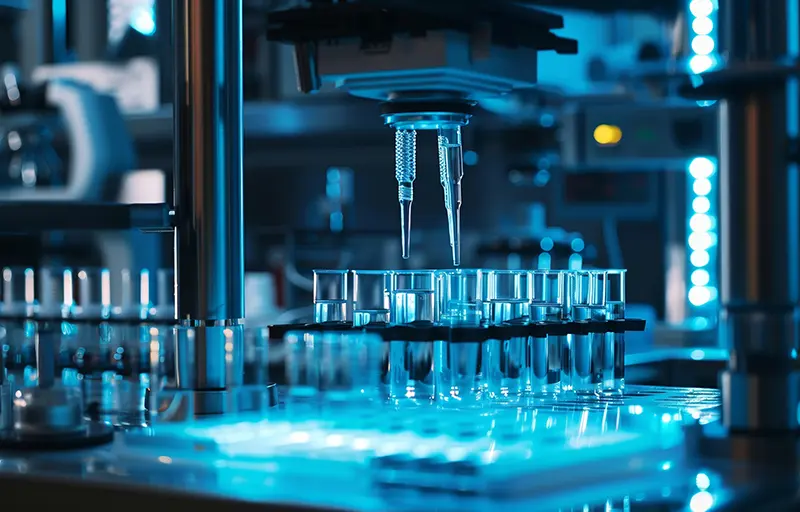
Abstract
Tumours evolve as heterogeneous populations of cells, which may be distinguished by different genomic aberrations and transcriptomic differences. The resulting intra-tumour heterogeneity plays an important role in cancer patient relapse and treatment failure, so that obtaining a clear understanding of each patient's tumour composition and evolutionary history is key for personalised therapies. Single-cell DNA sequencing now provides the possibility to resolve tumour heterogeneity at the highest resolution of individual tumour cells, while single-cell RNA sequencing offers expression profiling of tumour subpopulations as well as characterisation of the immune compartment of the tumourmicroenvironment. In parallel, ex-vivo drug screening gives a direct measurement of the aggregate response of tumour cells to various compounds.
With 136 samples profiled in a recent collaboration, we aim to develop and incorporate new prediction models for the drug response of the individual subclones in each patient's tumour. This approach will account for the tumour heterogeneity and for potentially different responses of constituent tumour parts to treatment. To integrate the different data modalities, we aim to first integrate the single-cell DNA and RNA information with joint modelling of the tumour evolution to more accurately understand its composition in terms of the existing subpopulations and their expression and copy number profiles. By additionally integrating drug response information, we can, for the first time, build and test drug prediction models at the level of tumour subclones. The potential of understanding the effect of different treatments on the heterogeneous parts of a tumour and thereby designing treatments for the full composite tumour could have great impact in aiding precision oncology.
This project is co-funded by PHRT.
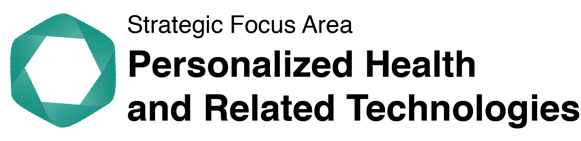
People
Collaborators


Quentin graduated with an engineering degree in mathematics and computer science from École des Ponts ParisTech in 2019. After a 6-month experience at the Center for Data Science of the New York University working on applied Machine Learning for medical imaging, he did a PhD in Statistics at Gustave Eiffel University (Paris). During his PhD, Quentin worked on random graphs and selective inference. His recent cross-disciplinary collaborations involve applications in biology and hydrology.
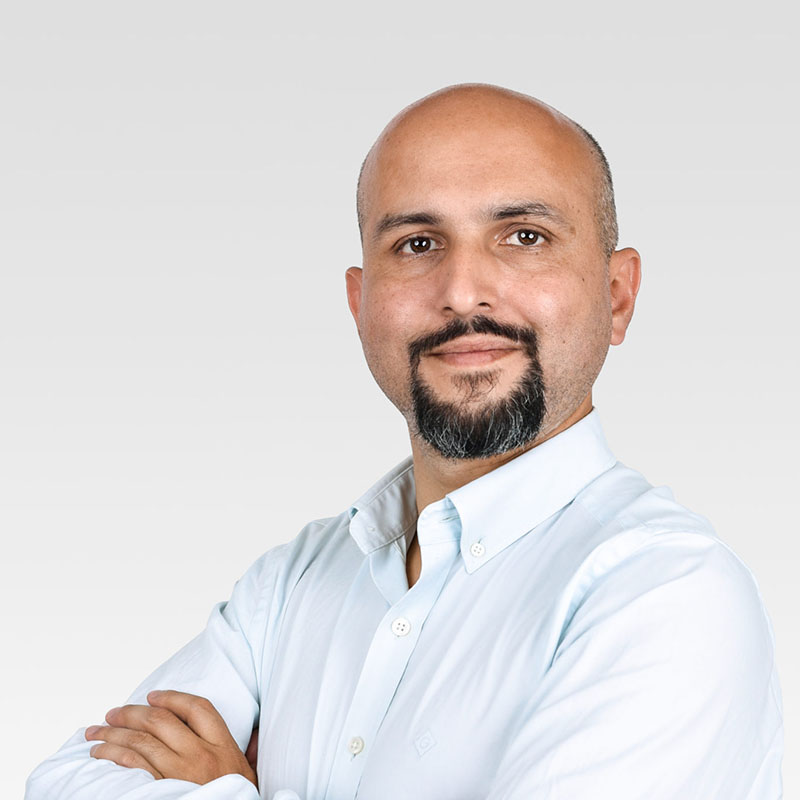
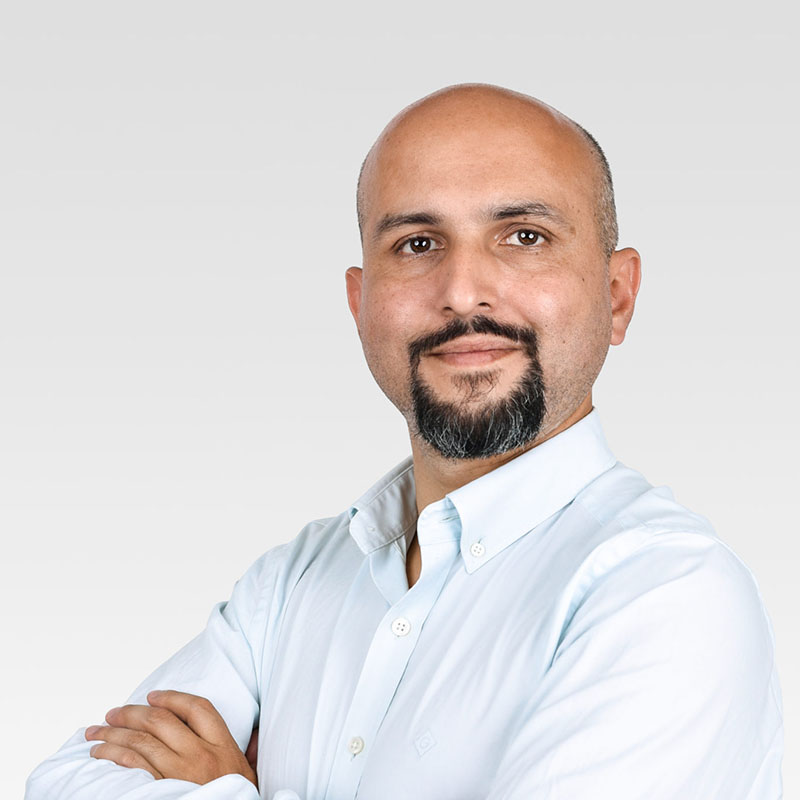
Daniel worked as a postdoctoral researcher on critical event prediction for the University Hospital in Zurich. In addition, Daniel has worked as a postdoctoral researcher in Lausanne, delivering algorithms for Bayesian inference in big panel data. Previously in Paris, he developed models for automated scientific discovery. He obtained a Ph.D. from the University of Edinburgh, funded by a Microsoft Research scholarship. His interest relates primarily to attacking applied biomedicine problems from different angles, frequentist statistics, Bayesian statistics, and Machine Learning.
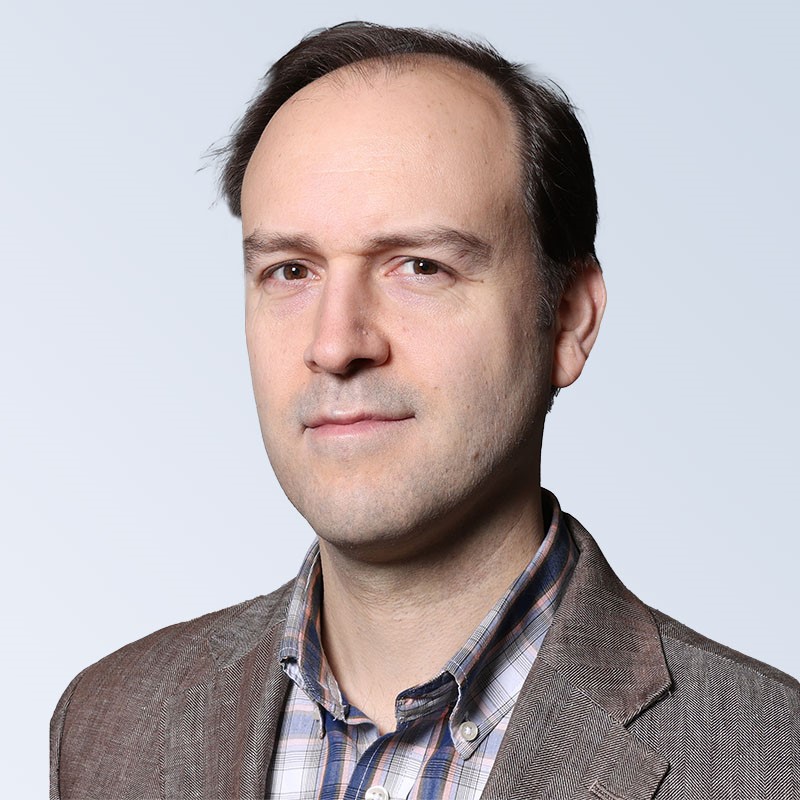
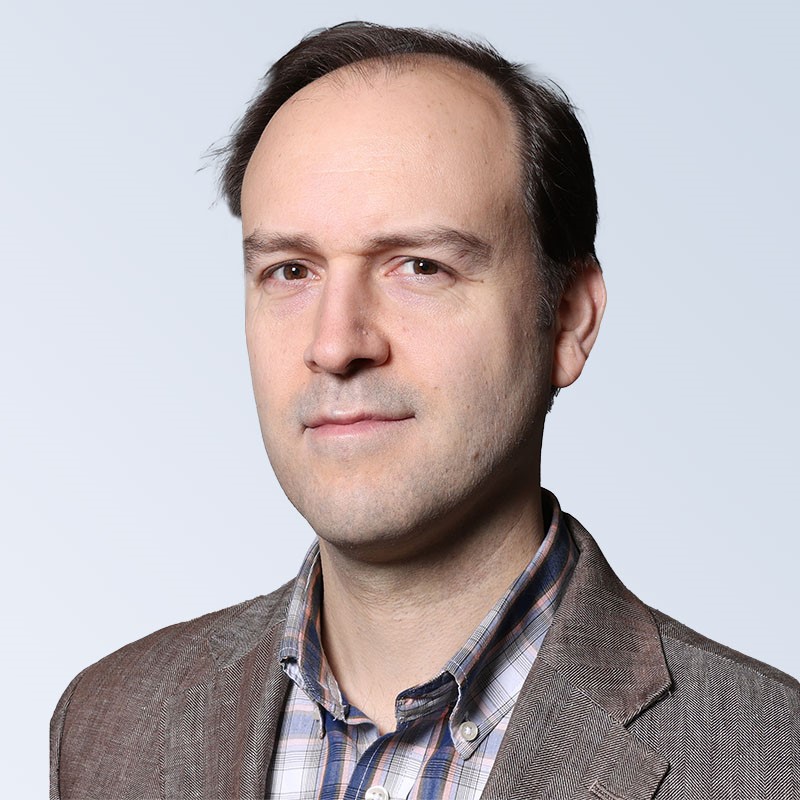
Guillaume Obozinski graduated with a PhD in Statistics from UC Berkeley in 2009. He did his postdoc and held until 2012 a researcher position in the Willow and Sierra teams at INRIA and Ecole Normale Supérieure in Paris. He was then Research Faculty at Ecole des Ponts ParisTech until 2018. Guillaume has broad interests in statistics and machine learning and worked over time on sparse modeling, optimization for large scale learning, graphical models, relational learning and semantic embeddings, with applications in various domains from computational biology to computer vision.
description
Motivation
During tumour progression, cancers may evolve into a complex system of heterogeneous
subpopulations of cells harbouring different genomic aberration. Diversity and heterogeneity within a tumour is a considerable cause of treatment failure and relapse, since subclonal cell populations may be resistant to treatment and therefore progress. Effective treatment should target all subpopulations, or evolve with the tumour to adapt as new clones become dominant. Uncovering the genetic makeup and evolutionary history of each tumour and linking these molecular alterations to the chances of reatment success is thus key to developing targeted therapies.
Proposed Approach / Solution
The aim of this project is to combine knowledge about the tumour architecture uncovered through single-cell DNA and RNA sequencing with the measured drug response of the tumour cells (cf. Figure 1). In particular we aim to integrate copy number and expression data from the same tumour to best describe the different tumour subpopulations and their aberrations, along with characterising the immune component. From this, and comparing to the aggregate drug responses of the tumour, we aim to build multitask learning predictors of drug sensitivity at the subclonal level. The mission of the SDSC in the project is to successfully integrate single-cell data modalities and implement the subclonal prediction model of drug response from single-cell DNA and RNA data.
Impact
This project offers the potential to determine which treatments could control the full tumour for precision oncology, allowing to set up protocols suitable for translating drug predictions to clinical usage.

Presentation
Gallery
Annexe
Additional resources
Bibliography
- Kuipers, J., Tuncel, M. A., Ferreira, P., Jahn, K., & Beerenwinkel, N. (2020). Single-cell copy number calling and event history reconstruction. doi:10.1101/2020.04.28.065755
- Ferreira, P. F., Kuipers, J., & Beerenwinkel, N. (2021). Mapping single-cell transcriptomes to copy number evolutionary trees. doi:10.1101/2021.11.04.467244
- Bertolini, A., Prummer, M., Tuncel, M. A., Menzel, U., Rosano-González, M. L., Kuipers, J., … Singer, F. (2022). scAmpi-A versatile pipeline for single-cell RNA-seq analysis from basics to clinics. PLoS Computational Biology, 18(6), e1010097. doi:10.1371/journal.pcbi.1010097
- Irmisch, A., Bonilla, X., Chevrier, S., Lehmann, K.-V., Singer, F., Toussaint, N. C., … Levesque, M. P. (2021). The Tumor Profiler Study: integrated, multi-omic, functional tumor profiling for clinical decision support. Cancer Cell, 39(3), 288–293. doi:10.1016/j.ccell.2021.01.004
Publications
Related Pages
More projects
ML-L3DNDT
BioDetect
News
Latest news


First National Calls: 50 selected projects to start in 2025
First National Calls: 50 selected projects to start in 2025
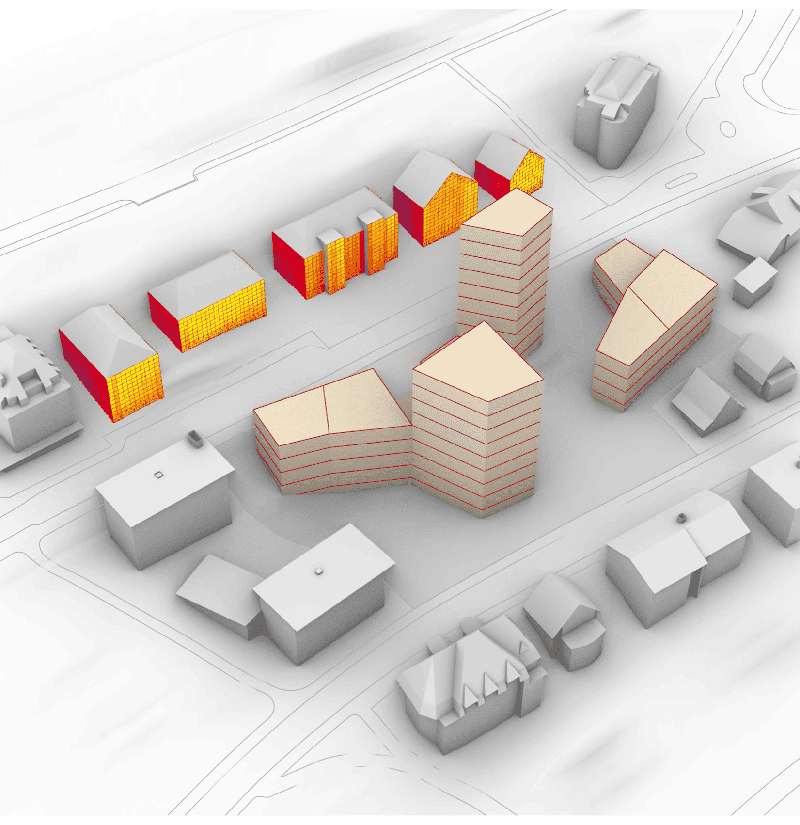
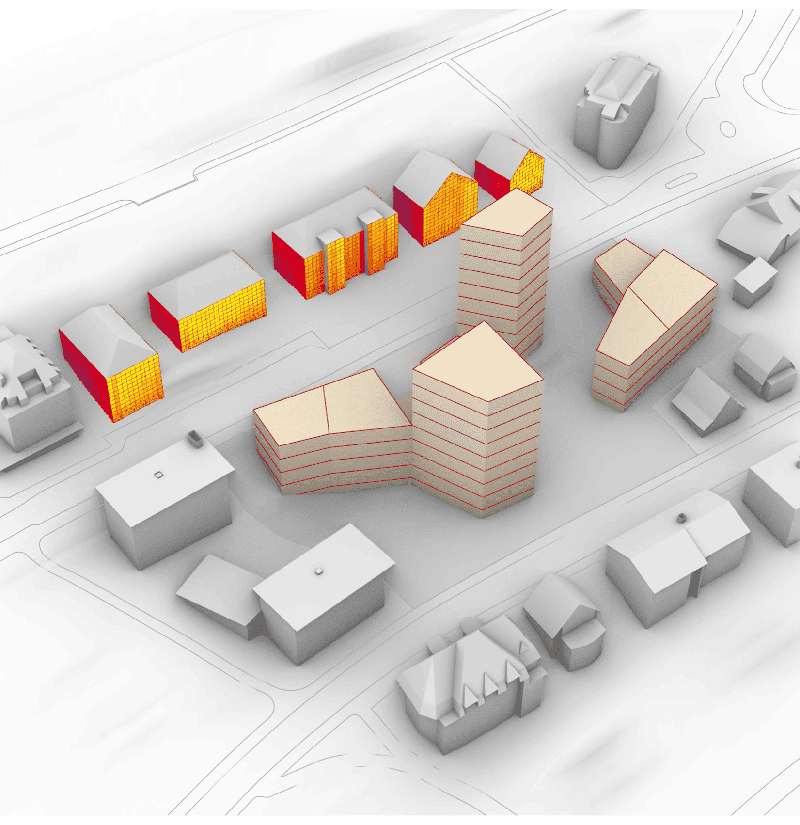
AIXD | Generative AI toolbox for architects and engineers
AIXD | Generative AI toolbox for architects and engineers


Smartair | An active learning algorithm for real-time acquisition and regression of flow field data
Smartair | An active learning algorithm for real-time acquisition and regression of flow field data
Contact us
Let’s talk Data Science
Do you need our services or expertise?
Contact us for your next Data Science project!