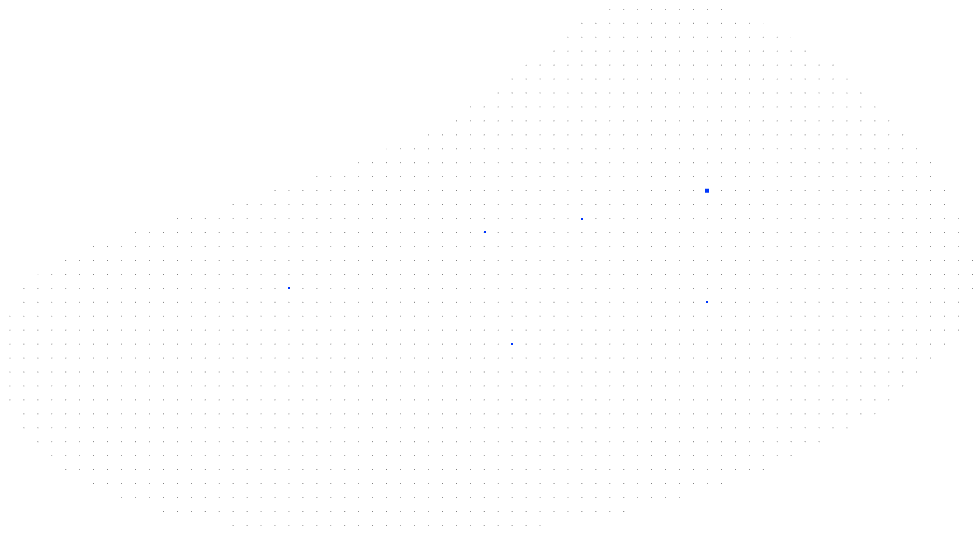
N2O-SSA
Combining measurements, modeling and machine learning to improve N2O accounting for sustainable agricultural development in sub-Saharan Africa

Abstract
Fertiliser use in sub-Saharan Africa is currently low, which results in lower agricultural crop yields than in many developed nations. In the coming decades, fertilizer use is predicted to increase, in order to drive increasing agricultural productivity. If fertilizer application is carefully controlled, emissions of greenhouse gases from agriculture soils can be minimized while ensuring high productivity and food security: Climate smart agriculture. The success of climate-smart agricultural practices depends on detailed knowledge of nutrient cycles in soils.
Nitrous oxide (N2O) is a strong greenhouse gas which makes a large contribution to global warming. In addition, it is the most important substance currently released that contributes to destruction of the ozone layer. N2O is produced by microbes in soils as they use nitrogen in two key biochemical reactions: Nitrification and denitrification. Agricultural fertilisation increases the amount of nitrogen available in soils, which leads to larger emissions of N2O. N2O emissions have primarily been studied in temperate ecosystems, but the causes and magnitude of emissions in sub-Saharan Africa are not well known. Expected changes in N2O emissions due to climate change and increasing fertilizer use are very poorly constrained, making it particularly difficult to drive investment in climate-smart agriculture in this region.
In this project, we will use a novel measurement technique – laser spectroscopy – to study N2O emissions from agricultural soils in Kenya. Laser spectroscopy allows us to directly monitor the isotopic composition of N2O emitted from soils, and thus infer the microbial N2O production and consumption pathways occurring in the soils. We will monitor N2O emissions and isotopic composition through a dry-wet seasonal transition to understand how climate and soil moisture control the production of N2O. We will relate our results to observations of N2O emissions made at other sites in sub-Saharan Africa, and use machine learning techniques to develop a new model of N2O emissions. This will allow us to understand and predict emissions and thus contribution to the development and uptake of sustainable agricultural practices.
People
Collaborators
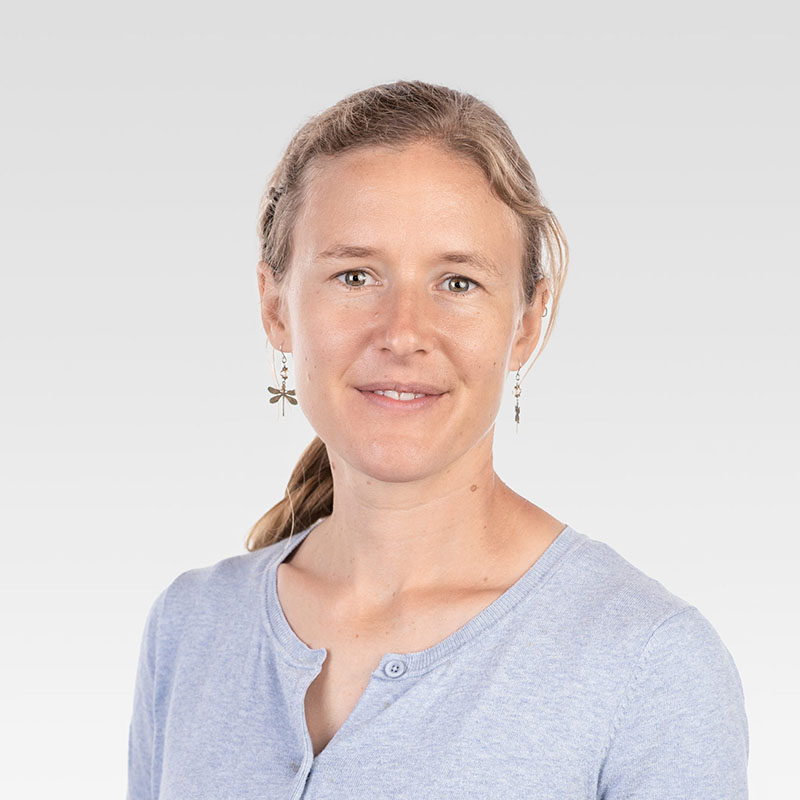
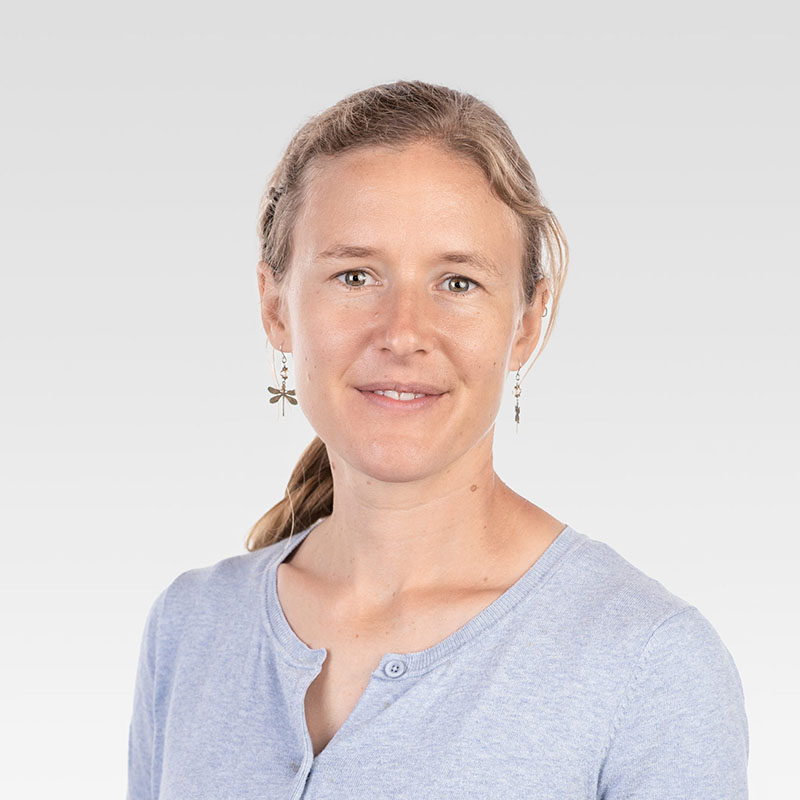
Eliza started at SDSC in March 2021, working as a Senior Scientist as part of the SDSC's academic team. She had previously worked as a postdoctoral researcher at the Massachusetts Institute of Technology (2012-2013), Empa (2013-2017), and the University of Innsbruck (2017-2020). Eliza had received her PhD in Atmospheric Science from the Max Planck Institute for Chemistry in 2012, and her Bachelor’s degree with Honours in Antarctic Science from the University of Tasmania in 2008. Her previous research had centered around the use of novel isotopic measurements and modeling approaches in atmospheric and biogeosciences, particularly the nitrogen cycle. Her research at SDSC was to focus on data analytics and machine learning approaches in environmental and natural sciences. Eliza's mission with SDSC ended in September 2024.
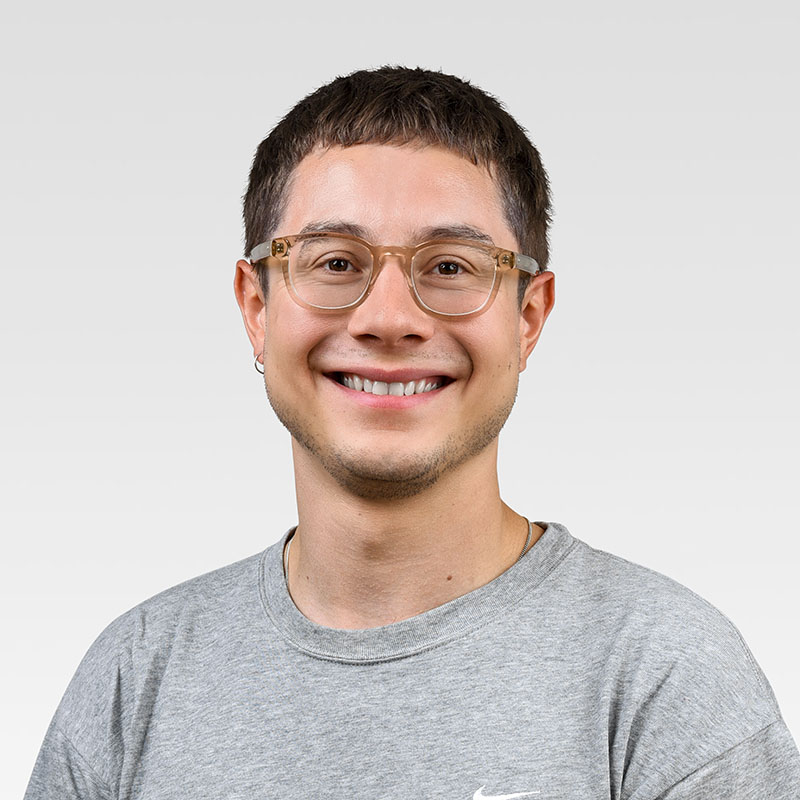
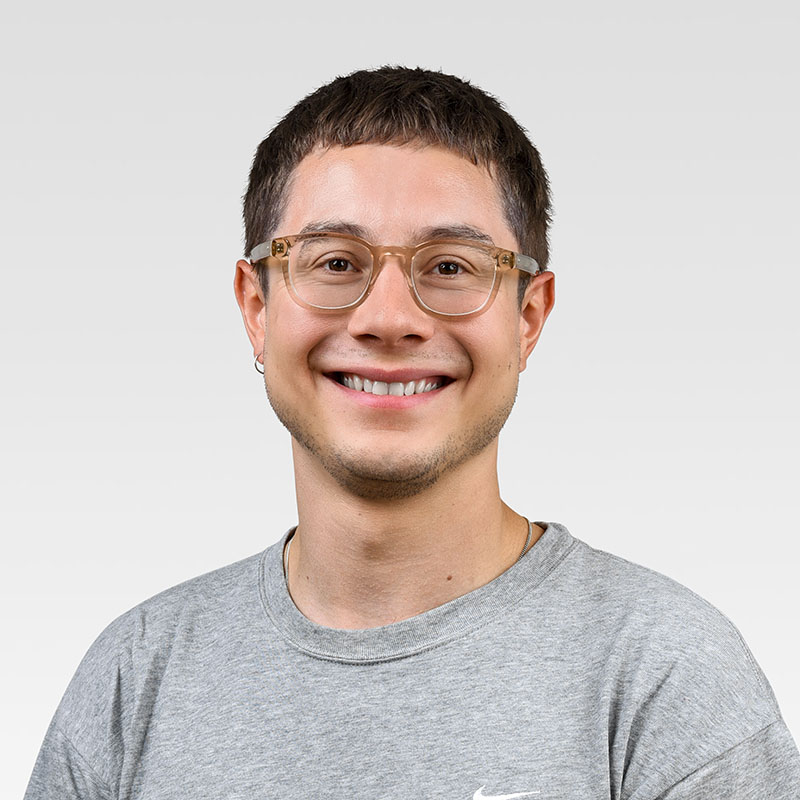
Phillip received his PhD in Environmental Science from the University of Reading in 2023, his Master’s by Research in Earth Sciences from the University of Manchester in 2019, and his Bachelor's degree in Environmental Science from the University of Manchester in 2018.
His previous research investigated biogeochemical cycling in wetland environments, in particular the nitrogen and carbon cycles.
Phillip’s research at SDSC will focus on novel nitrogen isotope measurements from agricultural soils and modelling, with a focus on data analytics and machine learning.
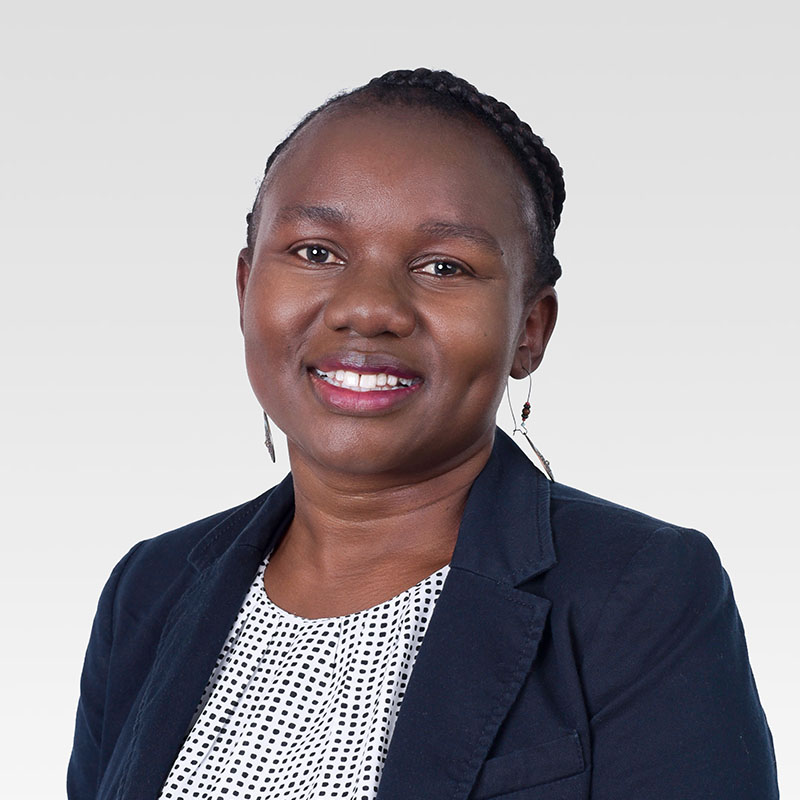
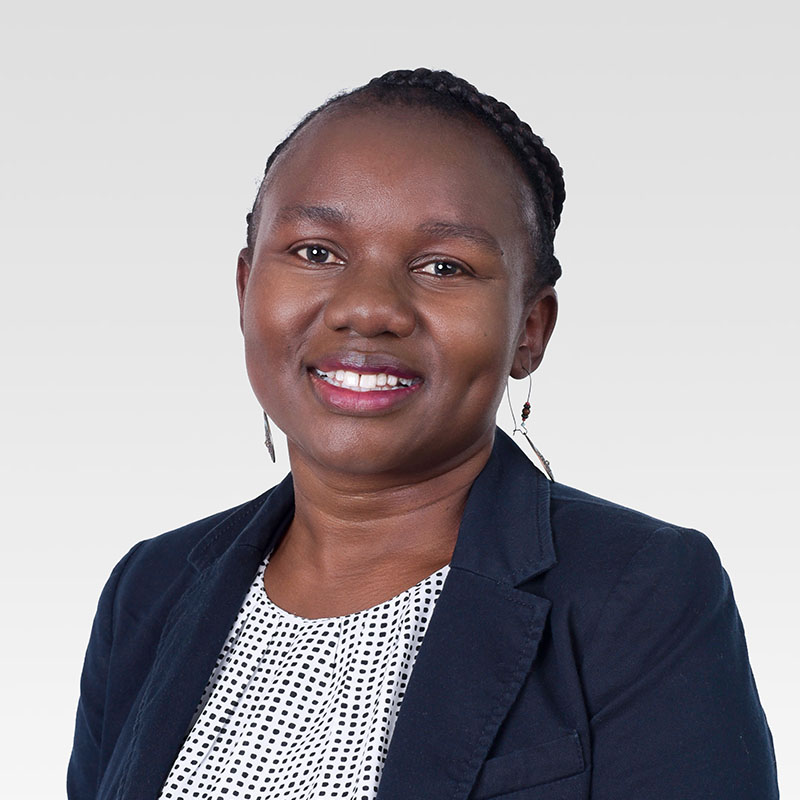
Turry joined the SDSC in July 2022 in a joint PhD position with ETH Zurich. Her research will be focused on the application of machine learning and data analytics in environmental science. Before her PhD program, Turry was a senior research associate in data science at the International Institute of Tropical Agriculture (IITA) within the sub-Saharan Africa region. Turry holds an Msc in Research Methodology with a focus on statistics and biometrics from the Jomo Kenyatta University of Agriculture and Technology (JKUAT).
description
Motivation
Measurements of greenhouse gases such as N2O in sub-Saharan Africa are sparse, leading to poor greenhouse gas accounting and a lack of investment in sustainable agriculture. Moreover, drivers of N2O emissions in tropical soils are poorly known, making it difficult to accurately predict emissions in a changing climate.
Proposed Approach / Solution
In N2O-SSA, we will use a combined measurement-data science-modelling approach to understand drivers of soil N2O emissions, in particular the influence of precipitation and soil moisture, and thus make improved predictions of N2O emission strength and variability in the coming decades.
Specific project goals include:
- Develop TREX-QCLAS-chamber system for high precision monitor of N2O fluxes and emitted N2O isotopic composition from soils
- Conduct a 6-month measurement campaign in Eldoret, Kenya, to understand soil N2O sources and sinks across a dry-wet seasonal transition
- Compile N2O flux and isotope data from studies in sub-Saharan Africa and other tropical regions, and - in combination with campaign measurements - analyse data to understand drivers of N2O production and consumption
- Use data science approaches for modelling and spatiotemporal upscaling, in order to predict future emissions and promote the development of targeted mitigation strategies
Impact
These results will help us understand and predict N2O emissions under current and future management and climate, and thus identify potential avenues of sustainable agricultural development.

Presentation
Gallery
Annexe
Additional resources
Bibliography
- Harris et al. (2022) Warming and redistribution of nitrogen inputs drive an increase in terrestrial nitrous oxide emission factor, Nature Communications, doi: 10.1038/s41467-022-32001-z
- Barthel et al. (2022) Low N2O and variable CH4 fluxes from tropical forest soils of the Congo Basin, Nature Communications, doi: 10.1038/s41467-022-27978-6
- Harris et al. (2021) Denitrifying pathways dominate nitrous oxide emissions from managed grassland during drought and rewetting, Science Advances, doi: 10.1126/sciadv.abb7118
Publications
Related Pages
- Further information at: N2O-SSA
- Funding information: SNSF Data Portal
More projects
MAGNIFY
News
Latest news


First National Calls: 50 selected projects to start in 2025
First National Calls: 50 selected projects to start in 2025
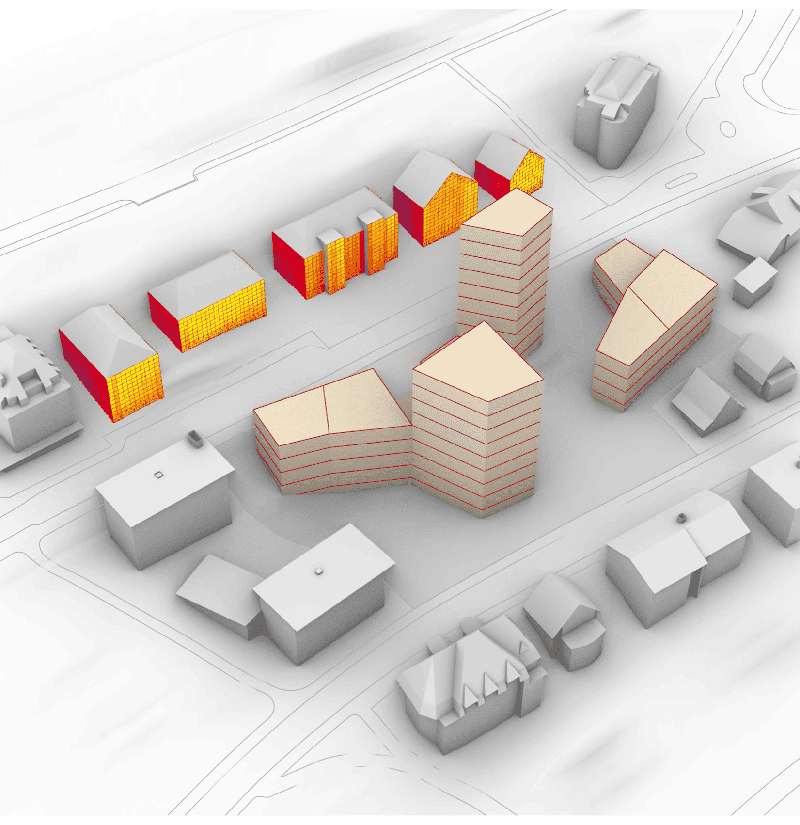
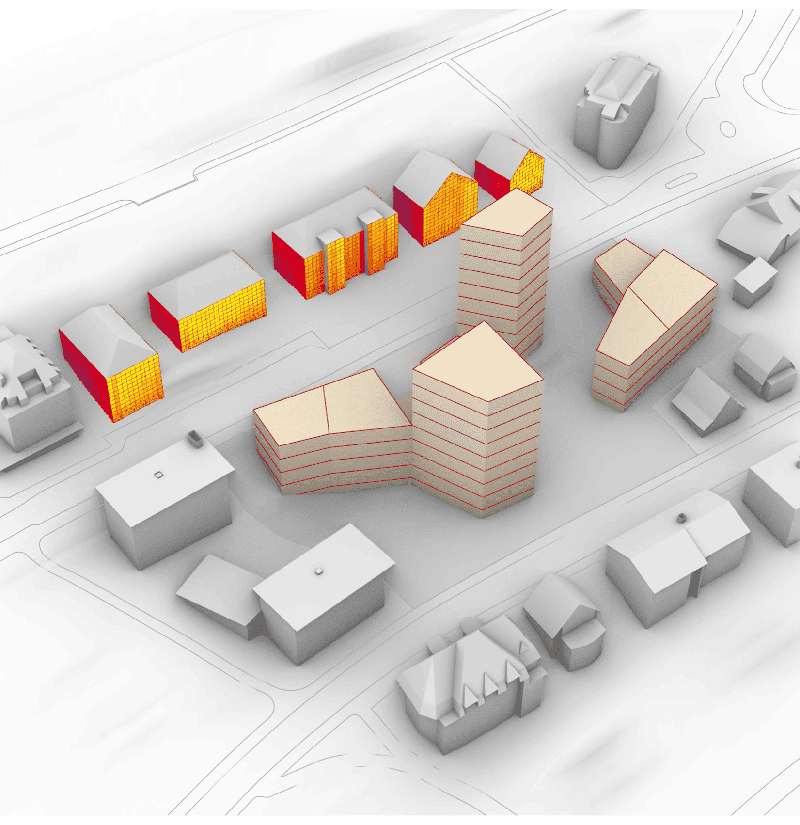
AIXD | Generative AI toolbox for architects and engineers
AIXD | Generative AI toolbox for architects and engineers


Smartair | An active learning algorithm for real-time acquisition and regression of flow field data
Smartair | An active learning algorithm for real-time acquisition and regression of flow field data
Contact us
Let’s talk Data Science
Do you need our services or expertise?
Contact us for your next Data Science project!