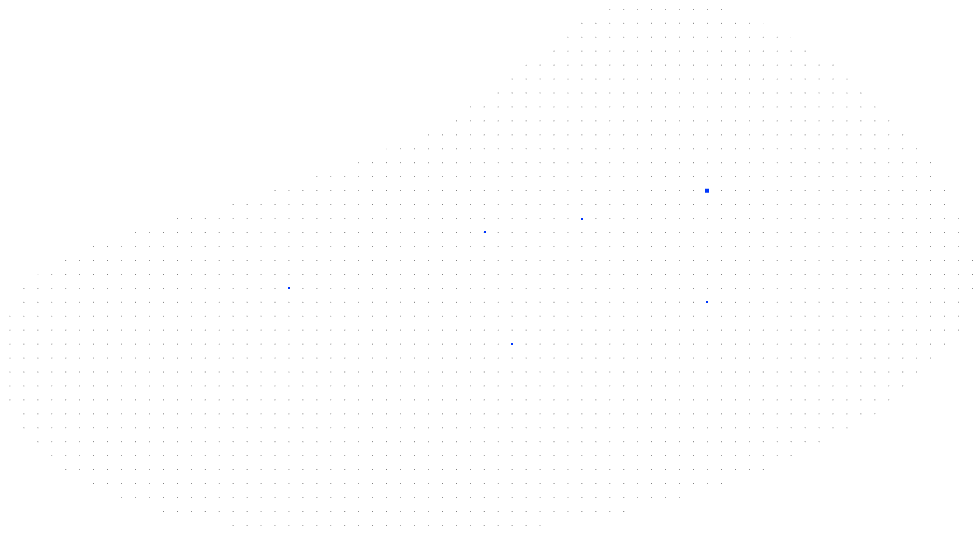
SPEEDMIND
Improving species biodiversity analyses and citizen science feedback through machine learning

Abstract
In order to conserve and manage biodiversity, we need an improved understanding of essential biodiversity drivers and improved predictions of resulting biodiversity patterns in space and time. Here, we propose a novel approach based on data mining and iterative machine learning to improve biodiversity models and to better exploit existing data as well as guide future data sampling efforts. Modern data mining techniques are destined to improve traditional species distribution modelling. On the one hand, massive amounts of biodiversity data are becoming available through citizen science and technical advances in monitoring, with increasing data of species occurrence, morphological traits, evolutionary history, and environmental variables. On the other hand, these data are often incomplete in that clear sampling designs are missing and information is not equally accurate or complete for all species. These data gaps can be filled by modern machine learning algorithms that are able to find a way through the maze of uncertainties in these data, in which scientists so easily get lost. Applicable to any species group world-wide, the project focuses on floristic data from Switzerland as a pilot system to set up and study the benefits of data mining and machine learning techniques for facilitating biodiversity assessments. Structured into four work packages, the project combines various data sources in a novel way and foster the link between ecological sciences and citizen science. By that, it paves the way towards automated quality checks in citizen science data, improved uncertainty analyses and identification of hidden information in large scale biodiversity inventories, and real-time guidance of observer efforts in citizen science based data collection. Key milestones of the project include: a) an operative framework linking real-time data streams and a citizen science interface; b) iteratively model the distribution of individual species and associated spatio-temporal uncertainty patterns using machine learning and data mining; c) a meta-learning to detect ecologically relevant, higher-level processes structuring biodiversity; and d) a model-based catalogue of criteria for guiding citizen scientists for improved data collection.
People
Collaborators


William obtained a PhD in Statistics in 2015 jointly from the University of Geneva and the University of Sydney. He then worked as a post-doctoral research fellow at Dalhousie University as part of a Canadian Statistical Sciences Institute collaborative research team. He was an Assistant Professor of Statistics at Stevens Institute of Technology in Hoboken, New Jersey, before joining the SDSC in September 2020. His research interests include robust statistics, non-parametric methods, and spatio-temporal modeling. His recent cross-disciplinary collaborations involve applications in marine biology, volcanology, and fisheries science.
PI | Partners:
Dynamic Macroecology Group:
- Prof. Niklaus Zimmermann
- Dr. Patrice Descombes
- Dr. Philipp Brun
- Dr. Damaris Zurell
description
Motivation
The SPEEDMIND project addresses some of the most important challenges in biodiversity monitoring at large scale. These include the preferential (or opportunistic) sampling aspect of presence-only data in the absence of full surveys (inventories), and the fact that species distribution maps (SDMs) are often constructed for one species at a time (no joint modeling of multiple species). The preferential biased sampling challenge arises from the plant species sightings provided by InfoFlora, the national data and information center of the Swiss flora, and the way it relies on citizen science/crowdsourcing for plant sightings (Figure 1).
Proposed Approach / Solution
Prior to the development of new models for plant SDMs, we have integrated large amounts of heterogeneous data sources (environmental data streams, maps, trait data, phylogenies, species occurrence data) in a standardized warehouse, representing an important contribution as it brings in one place disparate ecological, spatial and thematic information. In particular, SPEEDMIND developed new types of predictors sets at a very high spatial resolution (93 m), with improved precision, and which enable a better description of the species ecological niche. First, we generated highly computational demanding maps of climate (temperature and precipitation) by downscaling CHELSA climate layers (Karger et al., 2017) from 1 km to 93 m spatial resolution in Switzerland. Second, by combining a massive amount of plant data occurrences with expert-based ecological indicators of the plant ecology, we used random forests to generate eight ecologically meaningful predictors of plants (e.g., soil acidity, soil moisture, etc.). The resulting predictors outperformed traditional predictors used in ecology and increased our ability to predict the distribution of plant species in Switzerland (Figure 2).
While modelling rare plant species is a main challenge using traditional SDMs (because of low data availability), we aim at achieving this by jointly modelling rare species with the more widespread ones and by integrating information on species ecological and morphological similarities. More precisely, we are using two separate approaches to build joint species distribution models. The first is a hierarchical Poisson factorization approach, a form of recommender system where the most likely location-species pairs are identified and distinct latent weights represent preferences of locations and prevalence of species. The second approach is a particular spatial point process, a log-Gaussian Cox process, where environmental information is introduced as smooth non-linear effects. This point process is further enhanced by including predicted intensity fields from other species, which achieves a joint modeling.
Impact
The development of SDMs jointly for 3500+ plant species over Switzerland improves the monitoring of potentially invasive plant species, helps the study of rare species and their habitat, and can play a direct role in revising biodiversity management at the national scale, with possible implications for land use.


Presentation
Gallery
Annexe
Publications
- Descombes, P., Walthert, L., Baltensweiler, A., Meuli, R. G., Karger, D. N., Ginzler, C., Zurell, D., and Zimmermann, N. E. (2020). Spatial modelling of ecological indicator values improves predictions of plant distributions in complex landscapes. Ecography43, 1448-1463. https://doi.org/10.1111/ecog.05117
- Descombes, P., Chauvier, Y., Brun, P., Righetti, D., Wüest, R. O., Karger, D. N., Zurell, D., and Zimmermann, N. E. (2022). Strategies for sampling pseudo-absences for species distribution models in complex mountainous terrain. bioRxiv preprints. https://doi.org/10.1101/2022.03.24.485693
- Brun, P., Karger, D. N., Zurell, D., Descombes, P., de Witte, L. C., de Lutio, R., Wegner, J. D., and Zimmermann, N. E. (2024). Multispecies Deep Learning Using Citizen Science Data Produces More Informative Plant Community Models. Nature Communications 15, 4421. https://doi.org/10.1038/s41467-024-48559-9
- Wüest, R. O., Zimmermann, N. E., Zurell, D., Alexander, J. M., Fritz, S. A., Hof, C., Kreft, H., Normand, S., Cabral, J. S., Szekely, E., Thuiller, W., Wikelski, M., and Karger, D. N. (2019). Macroecology in the age of Big Data – Where to go from here? Journal of Biogeography 47, 1-12. https://doi.org/10.1111/jbi.13633
- Taylor, A., Zotz, G., Weigelt, P., Cai, L., Karger, D. N., König, C., and Kreft, H. (2021). Vascular epiphytes contribute disproportionately to global centres of plant diversity. Global Ecology and Biogeography 31, 62-74. https://doi.org/10.1111/geb.13411
- Karger, D. N., Wilson, A. M., Mahony, C., Zimmermann, N. E., and Jetz, W. (2021). Global daily 1 km land surface precipitation based on cloud cover-informed downscaling. Nature Scientific Data 8, 307, 1-12. https://doi.org/10.1038/s41597-021-01084-6
- Karger, D. N., Kessler, M., Lehnert, M., and Jetz, W. (2021). Limited protection and ongoing loss of tropical cloud forest biodiversity and ecosystems worldwide. Nature Ecology and Evolution 5, 854-862. https://doi.org/10.1038/s41559-021-01450-y
- Brun, P., Zimmermann, N. E., Hari, C., Pellissier, L. and Karger, D. N. (2022). Global climate-related predictors at kilometer resolution for the past and future. Earth System Science Data 14, 5573-5603. https://doi.org/10.5194/essd-14-5573-2022
- Karger, D. N., Lange, S., Hari, C., Reyer, C. P. O., Conrad, O., Zimmermann, N. E., and Frieler, K. (2023). CHELSA-W5E5: daily 1 km meteorological forcing data for climate impact studies. Earth System Science Data 15, 2445-2464. https://doi.org/10.5194/essd-15-2445-2023
- Karger, D. N., Saladin, B., Wüest, R. O., Graham, C. H., Zurell, D., Mo, L., and Zimmermann, N. E. (2023). Interannual climate variability improves niche estimates for ectothermic but not endothermic species. Nature Scientific Reports 13, 12538. https://doi.org/10.1038/s41598-023-39637-x
- Karger, D. N., Nobis, M. P., Normand, S., Graham, C. H., and Zimmermann, N. E. (2023). CHELSA-TraCE21k - high-resolution (1 km) downscaled transient temperature and precipitation data since the Last Glacial Maximum. Climate of the Past 19, 439-456. https://doi.org/10.5194/cp-19-439-2023
- Karger, D. N., Chauvier, Y., and Zimmermann, N. E. (2023). chelsa-cmip6 1.0: a python package to create high resolution bioclimatic variables based on CHELSA ver. 2.1 and CMIP6 data. Ecography 6, e06535. https://doi.org/10.1111/ecog.06535
Additional resources
Bibliography
Publications
Related Pages
More projects
ML-L3DNDT
BioDetect
News
Latest news


Smartair | An active learning algorithm for real-time acquisition and regression of flow field data
Smartair | An active learning algorithm for real-time acquisition and regression of flow field data
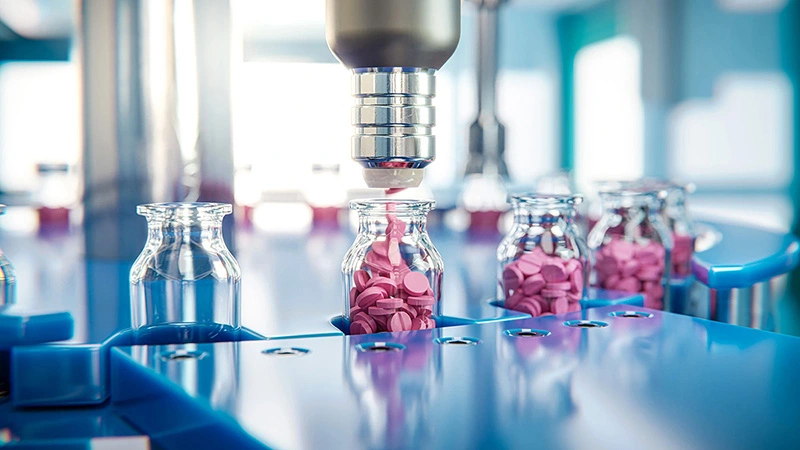
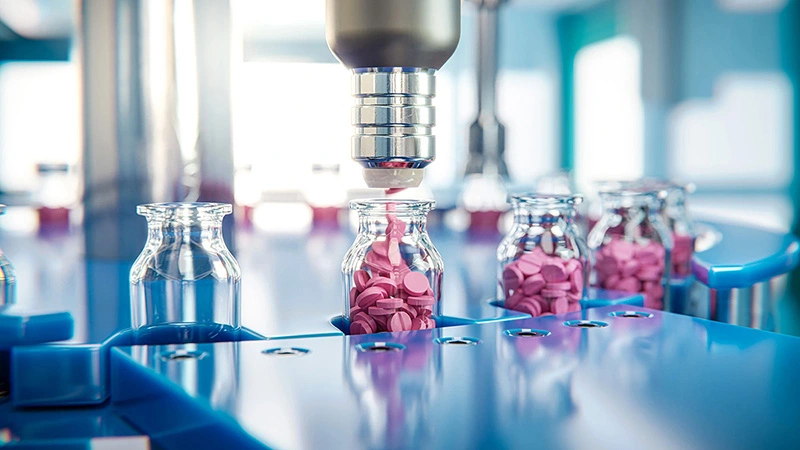
The Promise of AI in Pharmaceutical Manufacturing
The Promise of AI in Pharmaceutical Manufacturing


Efficient and scalable graph generation through iterative local expansion
Efficient and scalable graph generation through iterative local expansion
Contact us
Let’s talk Data Science
Do you need our services or expertise?
Contact us for your next Data Science project!