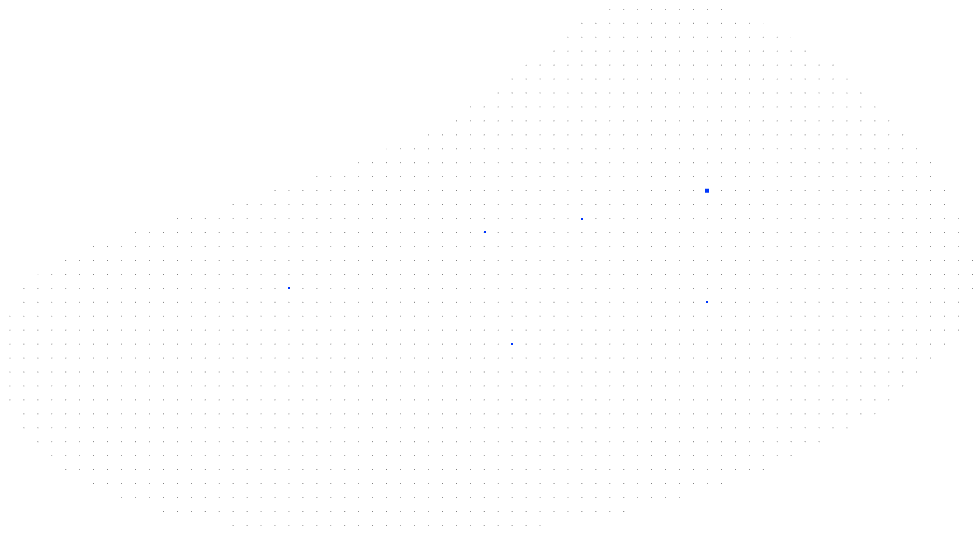
ArcticNAP
Arctic climate change: Exploring the Natural Aerosol baseline for improved model Predictions

Abstract
The Arctic is warming two to three times faster than the global average. Arctic amplification has repercussions for global climate, northern hemispheric weather and local livelihoods. Current models have difficulties to simulate Arctic change, making future scenarios uncertain. To improve model skill the factors contributing to Arctic amplification need to be better represented. We focus on the role of aerosols. Aerosols can interact with solar radiation directly, as well as indirectly through their incluence on cloud radiative properties. Considerable effort has gone into describing the role of anthropogenic aerosols in Arctic climate change. However, there has been much less focus on natural aerosols, which represents a large part of overall model uncertainty. This is partly because they are emitted and processed via complex pathways involving several environmental compartments, which are difficult to represent in models. To complicate things further, the natural state of the Arctic is changing rapidly thereby changing aerosol processes.
We propose to use an unprecedented combination of in situ measurements, satellite-based observations and numerical weather prediction data to reveal the processes controlling natural Arctic aerosols and driving climate-relevant aerosol properties. Aerosol data are available from eight Arctic observatories covering up to 30 years, and from two high Arctic drift expeditions. By developing a latent variable model, which accommodates the different data types, time series with gaps, and domain knowledge for constraints, we explore relationships between environmental variables, such as sea ice extent, chlorophyll-a concentrations, or meteorological conditions, and aerosol concentrations. After quantifying these relationships, we compare the output from five Earth System models (ESM) against the data driven model results, to identify where ESMs can be improved. This project is an important step in this research field, as the response of the Arctic environment to future climate change (including atmospheric and oceanic conditions) remains an open research question and provides an avenue for continuing research.
People
Collaborators
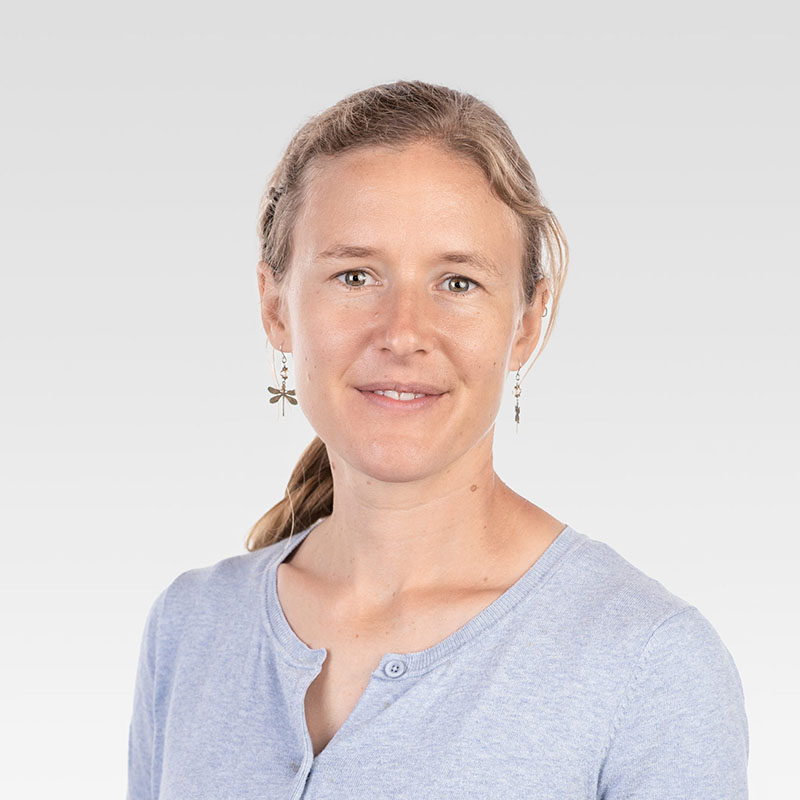
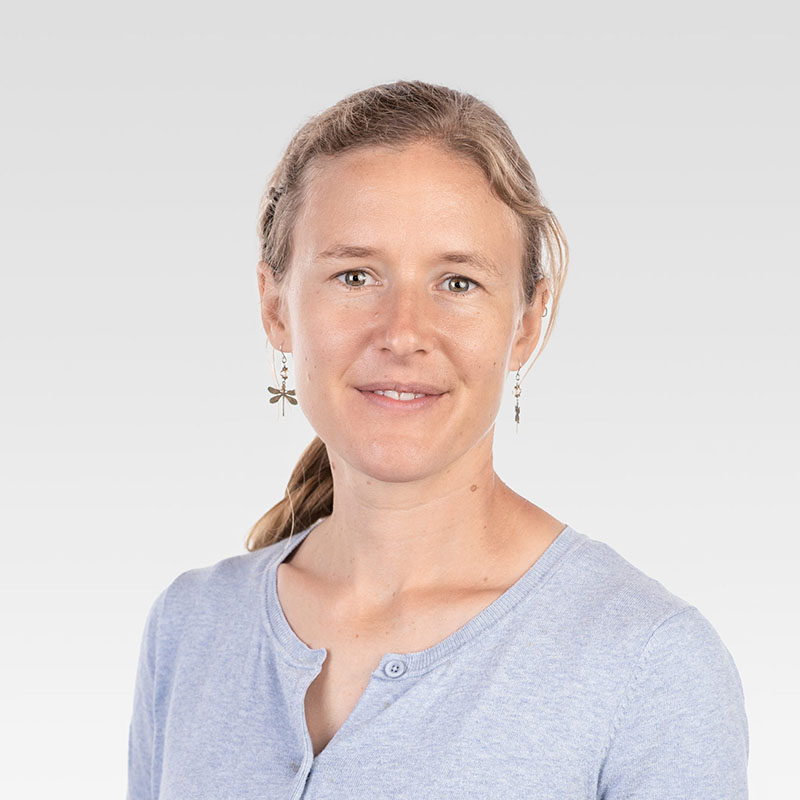
Eliza started at SDSC in March 2021, working as a Senior Scientist as part of the SDSC's academic team. She had previously worked as a postdoctoral researcher at the Massachusetts Institute of Technology (2012-2013), Empa (2013-2017), and the University of Innsbruck (2017-2020). Eliza had received her PhD in Atmospheric Science from the Max Planck Institute for Chemistry in 2012, and her Bachelor’s degree with Honours in Antarctic Science from the University of Tasmania in 2008. Her previous research had centered around the use of novel isotopic measurements and modeling approaches in atmospheric and biogeosciences, particularly the nitrogen cycle. Her research at SDSC was to focus on data analytics and machine learning approaches in environmental and natural sciences. Eliza's mission with SDSC ended in September 2024.


William obtained a PhD in Statistics in 2015 jointly from the University of Geneva and the University of Sydney. He then worked as a post-doctoral research fellow at Dalhousie University as part of a Canadian Statistical Sciences Institute collaborative research team. He was an Assistant Professor of Statistics at Stevens Institute of Technology in Hoboken, New Jersey, before joining the SDSC in September 2020. His research interests include robust statistics, non-parametric methods, and spatio-temporal modeling. His recent cross-disciplinary collaborations involve applications in marine biology, volcanology, and fisheries science.
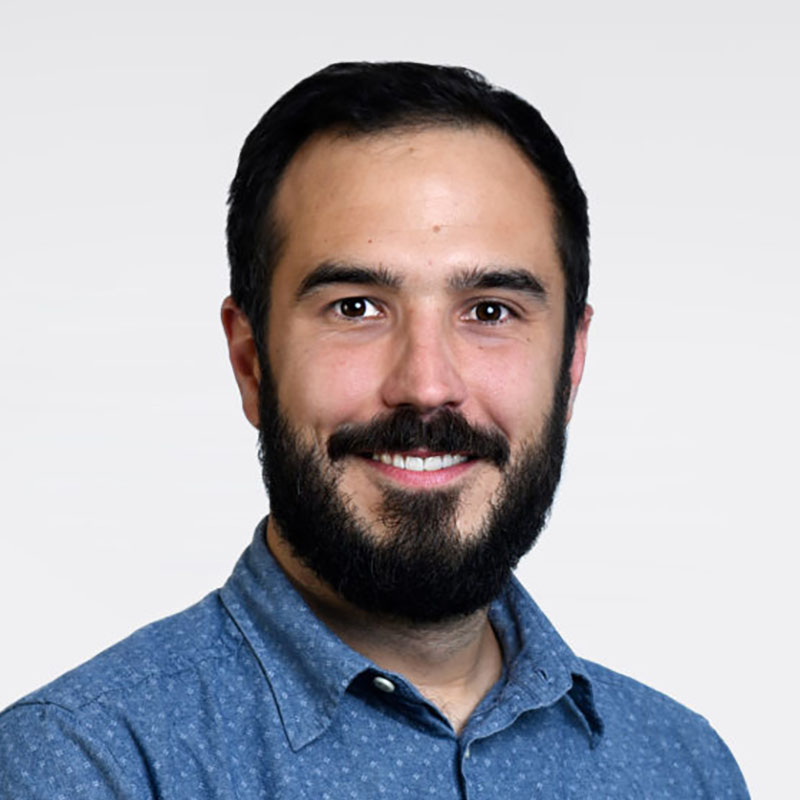
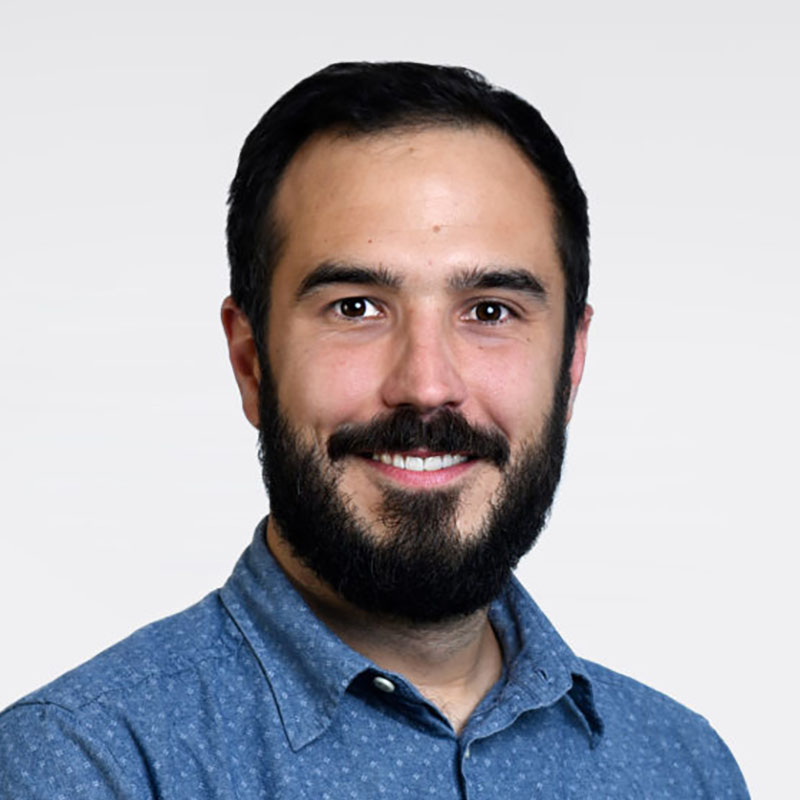
Michele received a Ph.D. in Environmental Sciences from the University of Lausanne (Switzerland) in 2013. He was then a visiting postdoc in the CALVIN group, Institute of Perception, Action and Behaviour of the School of Informatics at the University of Edinburgh, Scotland (2014-2016). He then joined the Multimodal Remote Sensing and the Geocomputation groups at the Geography department of the University of Zurich, Switzerland (2016-2017). His main research activities were at the interface of computer vision, machine and deep learning for the extraction of information from aerial photos, satellite optical images and geospatial data in general.
PI | Partners:
description
Motivation
The natural production of aerosols in the Arctic plays a likely important role in climate change, although this role is not well understood, particularly in a changing climate. The main goal of the project is to construct models which can identify key environmental drivers of the key aerosol species methanesulfonic acid (MSA), and potentially describe processes that may evolve with climate change.
Proposed Approach / Solution
We combine many data sources in an unprecedented way, including in situ measurements of MSA (see Figure 1), remote sensing, and outputs from numerical weather prediction models. By pre-processing climatic and environmental features as residence-time weighted averages based on a particle dispersion model (FLEXPART) we can model and predict MSA concentrations in space-time. We propose two models: a random forest model and an additive model tailored for the task at hand. Both models have similar predictive performance in terms of MSA concentration forecasts, but they yield different inference as far as feature selection is concerned. In parallel to this work, using gradient-boosted trees with selected key features (Figure 2) we have identified the main source regions for MSA and project a seasonal shift over the next 50 years (Figure 3).
Impact
Our results, including the climatic and environmental drivers we identified in a fully data-driven way, can in principle directly inform global climate models in their capacity to represent aerosols in the Arctic. This can improve climate predictions at a global scale and thus have a substantial impact in certain medium- and long-term projections.


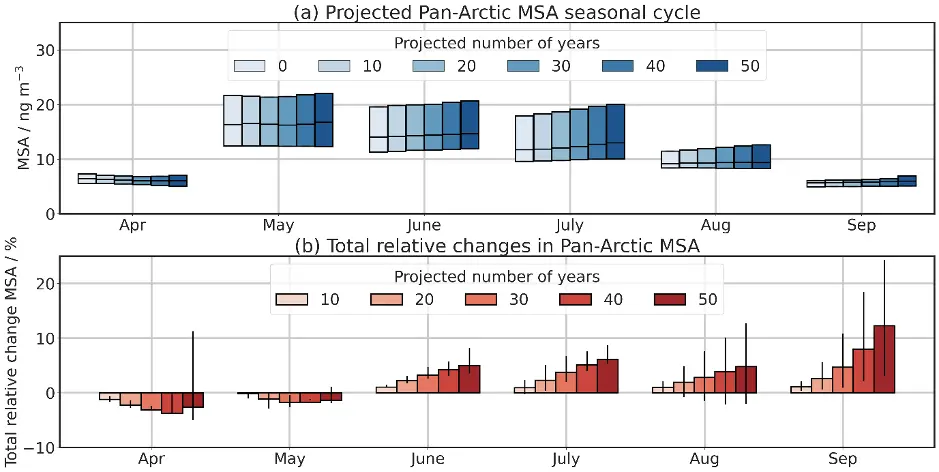
Presentation
Gallery
Annexe
Additional resources
Bibliography
- Pörtner, H.-O. et al. (2019). IPCC Special Report on the Ocean and Cryosphere in a Changing Climate. Report Home
- Schmale, J., Zieger, P., and Ekman, A. M. L. (2021). Aerosols in current and future Arctic climate. Nature Climate Change 11, 95-105. https://doi.org/10.1038/s41558-020-00969-5
Publications
Related Pages
More projects
ML-L3DNDT
BioDetect
News
Latest news


First National Calls: 50 selected projects to start in 2025
First National Calls: 50 selected projects to start in 2025
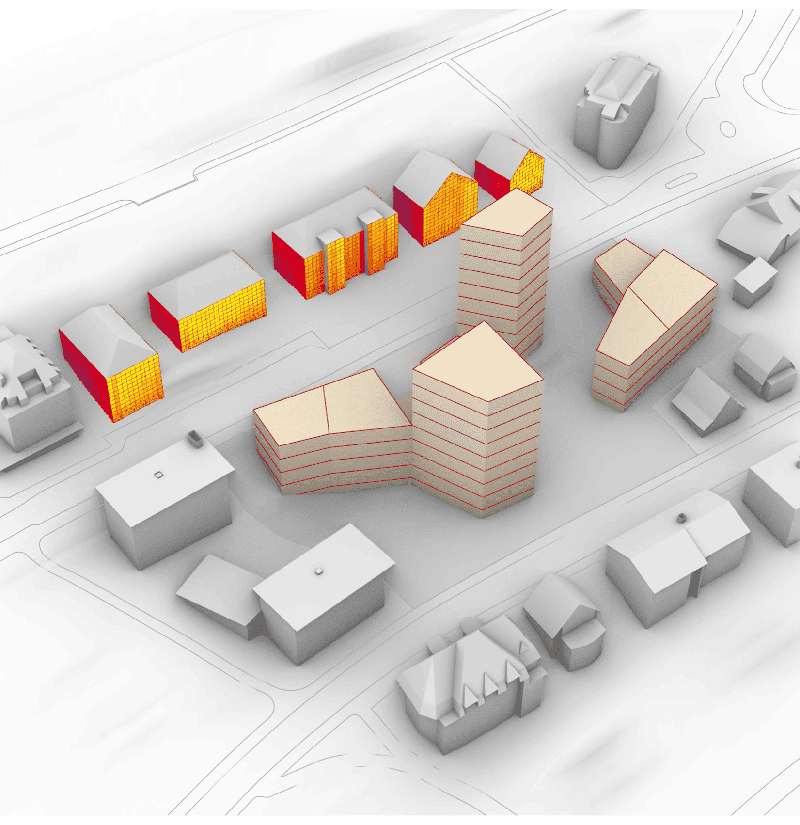
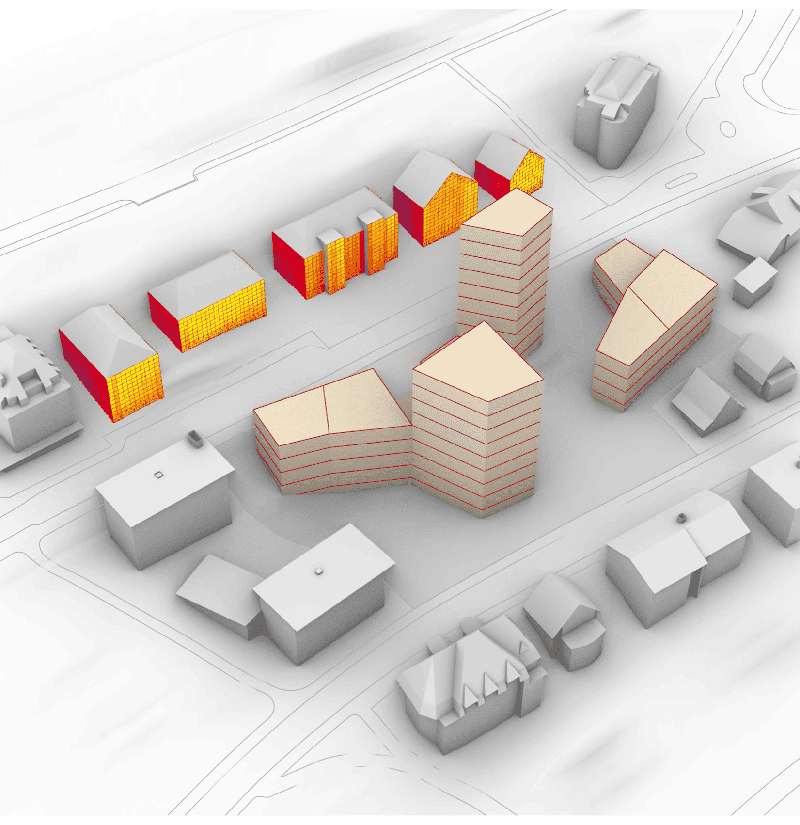
AIXD | Generative AI toolbox for architects and engineers
AIXD | Generative AI toolbox for architects and engineers


Smartair | An active learning algorithm for real-time acquisition and regression of flow field data
Smartair | An active learning algorithm for real-time acquisition and regression of flow field data
Contact us
Let’s talk Data Science
Do you need our services or expertise?
Contact us for your next Data Science project!