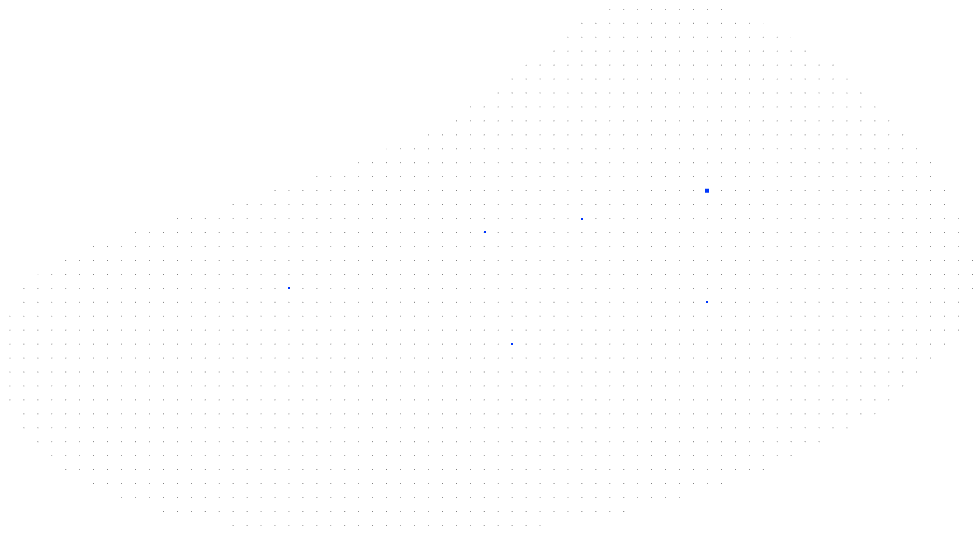
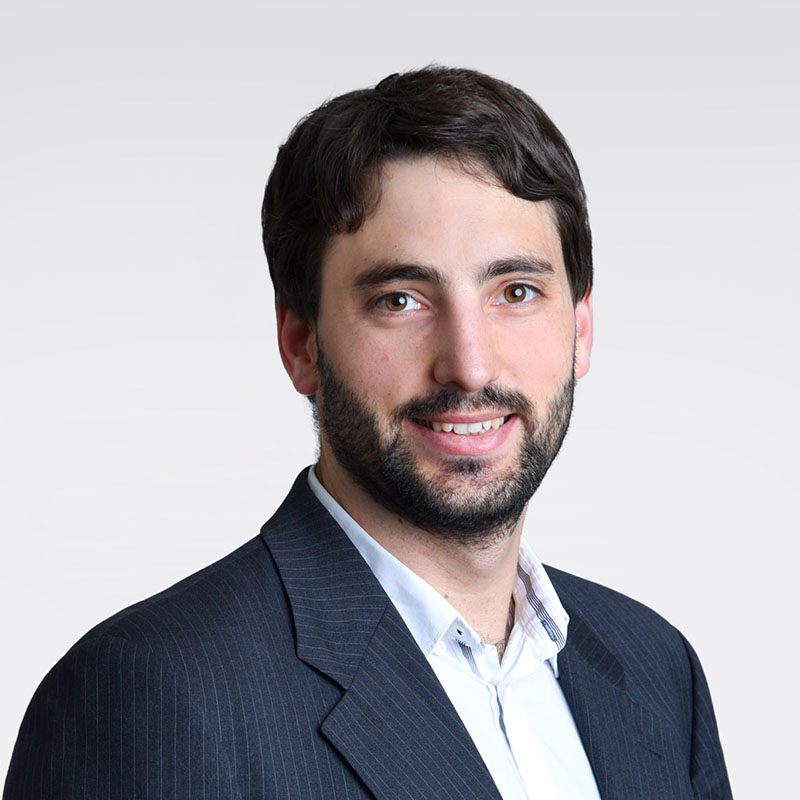
Nathanaël Perraudin
After finishing his Master in electrical engineering at the Ecole Fédérale de Lausanne (EPFL), Nathanaël worked as a researcher in the Acoustic Research Institute (ARI) in Vienna. In 2013, he returned to EPFL for a PhD, where he specialized himself in different fields of data science: signal processing, machine learning, graph theory and optimization. Furthermore, he created two open source libraries for optimization (UNLocBoX) and graph signal processing (GSPBOX). Since 2017, Nathanaël Perraudin is a Research Data Scientist at the Swiss Data Science Center in the ETH Zurich. He focuses on different aspects of deep learning in the area of generative models (VAE and GAN), recursive architectures and convolutional neural network for irregular domains. Outside office hours, he is passionate by tango dancing, tandem bike touring, skiing and rock climbing.
Projects
LEAP
DATSSFLOW
MLATEM
Publications
Mentioned in
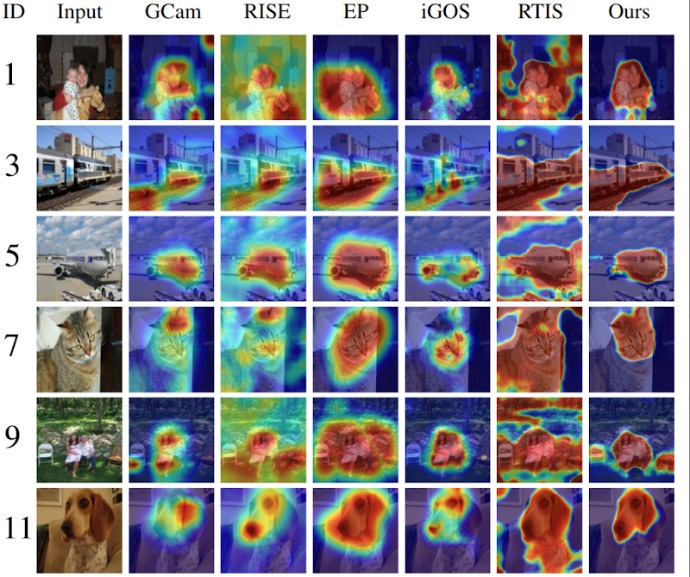
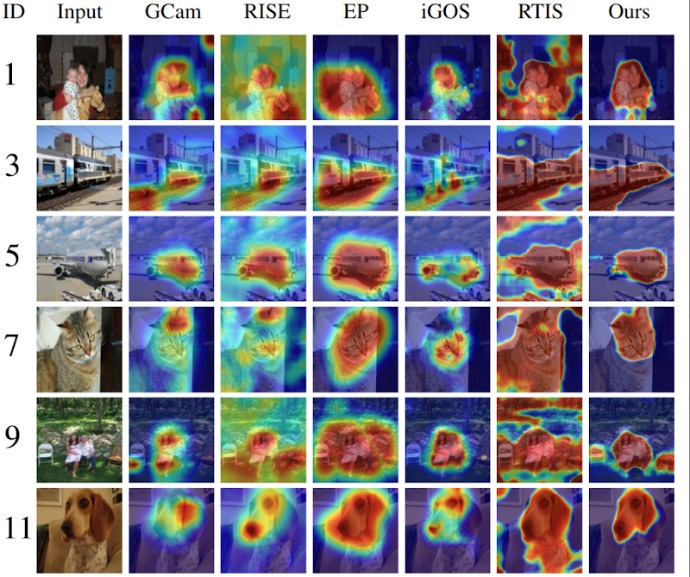
What you see is what you classify: black box attributions
What you see is what you classify: black box attributions
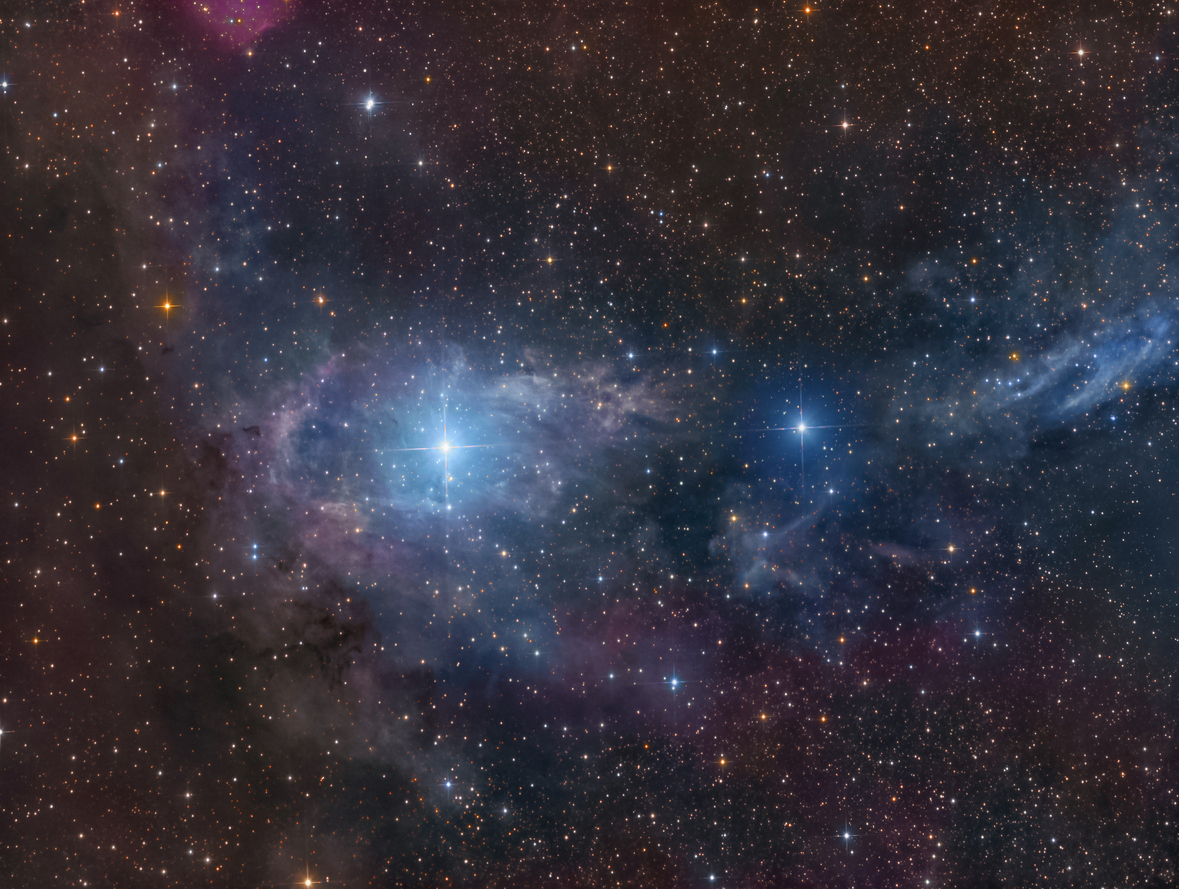
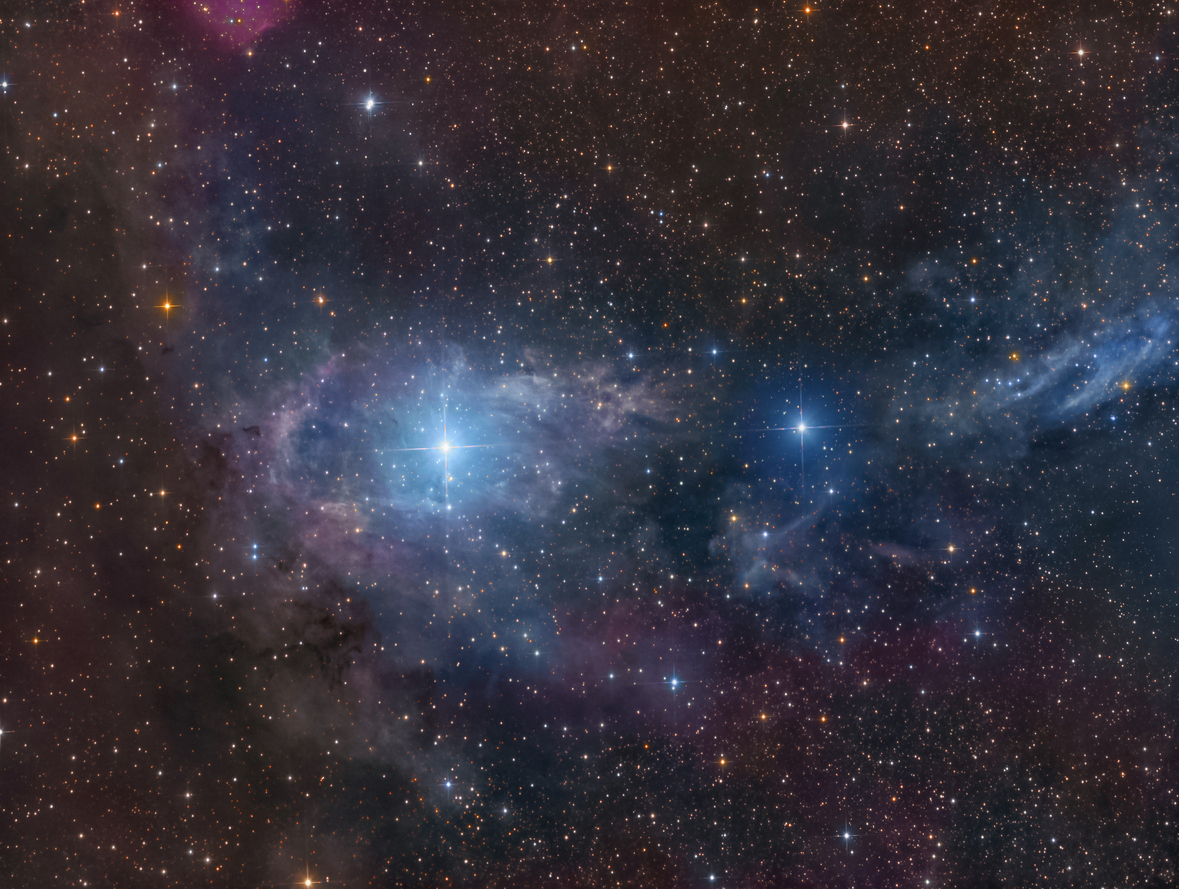
Deepsphere | A neural network architecture for spherical data
Deepsphere | A neural network architecture for spherical data
Case Studies
Contact us
Let’s talk Data Science
Do you need our services or expertise?
Contact us for your next Data Science project!